#difference between ai and data science
Explore tagged Tumblr posts
Text
Complete Excel, AI and Data Science mega bundle.
Unlock Your Full Potential with Our 100-Hour Masterclass: The Ultimate Guide to Excel, Python, and AI.
Why Choose This Course? In today’s competitive job market, mastering a range of technical skills is more important than ever. Our 100-hour comprehensive course is designed to equip you with in-demand capabilities in Excel, Python, and Artificial Intelligence (AI), providing you with the toolkit you need to excel in the digital age.
To read more click here <<
Become an Excel Pro Delve deep into the intricacies of Excel functions, formulae, and data visualization techniques. Whether you’re dealing with basic tasks or complex financial models, this course will make you an Excel wizard capable of tackling any challenge.
Automate Your Workflow with Python Scripting in Python doesn’t just mean writing code; it means reclaiming your time. Automate everyday tasks, interact with software applications, and boost your productivity exponentially.
If you want to get full course click here <<
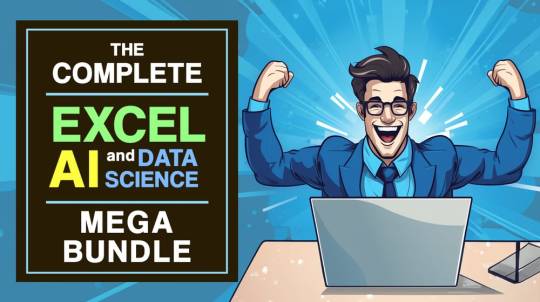
Turn Ideas into Apps Discover the potential of Amazon Honeycode to create custom apps tailored to your needs. Whether it’s for data management, content tracking, or inventory — transform your creative concepts into practical solutions.
Be Your Own Financial Analyst Unlock the financial functionalities of Excel to manage and analyze business data. Create Profit and Loss statements, balance sheets, and conduct forecasting with ease, equipping you to make data-driven decisions.
Embark on an AI Journey Step into the future with AI and machine learning. Learn to build advanced models, understand neural networks, and employ TensorFlow. Turn big data into actionable insights and predictive models.
Master Stock Prediction Gain an edge in the market by leveraging machine learning for stock prediction. Learn to spot trends, uncover hidden patterns, and make smarter investment decisions.
Who Is This Course For? Whether you’re a complete beginner or a seasoned professional looking to upskill, this course offers a broad and deep understanding of Excel, Python, and AI, preparing you for an ever-changing work environment.
Invest in Your Future This isn’t just a course; it’s a game-changer for your career. Enroll now and set yourself on a path to technological mastery and unparalleled career growth.
Don’t Wait, Transform Your Career Today! Click here to get full course <<
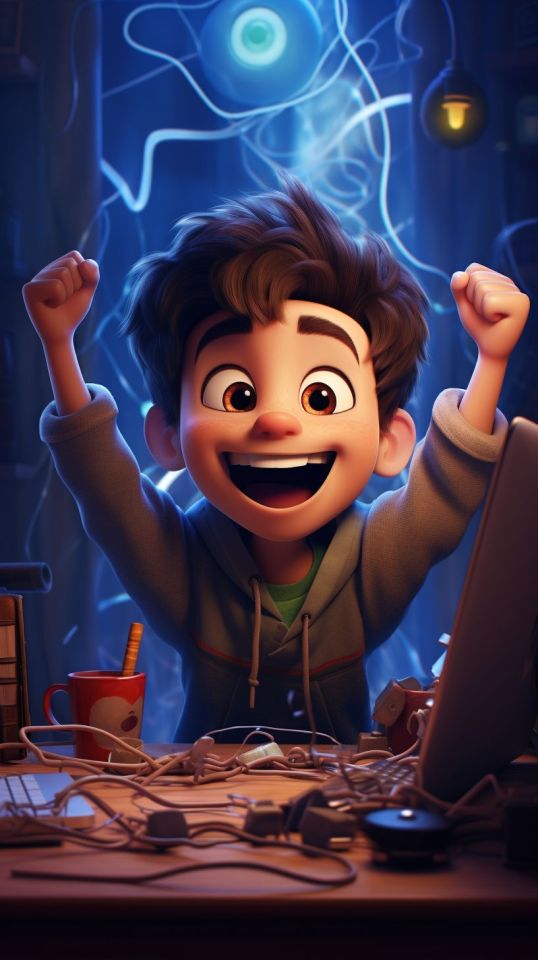
#data science#complete excel course#excel#data science and machine learning#microsoft excel#difference between ai and data science#learn excel#complete microsoft excel tutorial#difference between data science and data engineering#365 data science#aegis school of data science#advanced excel#excel tips and tricks#advanced excel full course#computer science#ms in data science#pgp in data science#python data science#python data science tutorial#Tumblr
1 note
·
View note
Text
This article highlights the key difference between Machine Learning and Artificial Intelligence based on approach, learning, application, output, complexity, etc.
#Difference between AI and ML#ai vs ml#artificial intelligence vs machine learning#key differences between ai and ml#artificial intelligence#machine learning#AI#ML#technology#data science#automation#robotics#neural networks#deep learning#natural language processing#computer vision#predictive analytics#big data#future trends.
2 notes
·
View notes
Text
Bubble findings could unlock better electrode and electrolyzer designs
New Post has been published on https://thedigitalinsider.com/bubble-findings-could-unlock-better-electrode-and-electrolyzer-designs/
Bubble findings could unlock better electrode and electrolyzer designs
Industrial electrochemical processes that use electrodes to produce fuels and chemical products are hampered by the formation of bubbles that block parts of the electrode surface, reducing the area available for the active reaction. Such blockage reduces the performance of the electrodes by anywhere from 10 to 25 percent.
But new research reveals a decades-long misunderstanding about the extent of that interference. The findings show exactly how the blocking effect works and could lead to new ways of designing electrode surfaces to minimize inefficiencies in these widely used electrochemical processes.
It has long been assumed that the entire area of the electrode shadowed by each bubble would be effectively inactivated. But it turns out that a much smaller area — roughly the area where the bubble actually contacts the surface — is blocked from its electrochemical activity. The new insights could lead directly to new ways of patterning the surfaces to minimize the contact area and improve overall efficiency.
The findings are reported today in the journal Nanoscale, in a paper by recent MIT graduate Jack Lake PhD ’23, graduate student Simon Rufer, professor of mechanical engineering Kripa Varanasi, research scientist Ben Blaiszik, and six others at the University of Chicago and Argonne National Laboratory. The team has made available an open-source, AI-based software tool that engineers and scientists can now use to automatically recognize and quantify bubbles formed on a given surface, as a first step toward controlling the electrode material’s properties.
Play video
Gas-evolving electrodes, often with catalytic surfaces that promote chemical reactions, are used in a wide variety of processes, including the production of “green” hydrogen without the use of fossil fuels, carbon-capture processes that can reduce greenhouse gas emissions, aluminum production, and the chlor-alkali process that is used to make widely used chemical products.
These are very widespread processes. The chlor-alkali process alone accounts for 2 percent of all U.S. electricity usage; aluminum production accounts for 3 percent of global electricity; and both carbon capture and hydrogen production are likely to grow rapidly in coming years as the world strives to meet greenhouse-gas reduction targets. So, the new findings could make a real difference, Varanasi says.
“Our work demonstrates that engineering the contact and growth of bubbles on electrodes can have dramatic effects” on how bubbles form and how they leave the surface, he says. “The knowledge that the area under bubbles can be significantly active ushers in a new set of design rules for high-performance electrodes to avoid the deleterious effects of bubbles.”
“The broader literature built over the last couple of decades has suggested that not only that small area of contact but the entire area under the bubble is passivated,” Rufer says. The new study reveals “a significant difference between the two models because it changes how you would develop and design an electrode to minimize these losses.”
To test and demonstrate the implications of this effect, the team produced different versions of electrode surfaces with patterns of dots that nucleated and trapped bubbles at different sizes and spacings. They were able to show that surfaces with widely spaced dots promoted large bubble sizes but only tiny areas of surface contact, which helped to make clear the difference between the expected and actual effects of bubble coverage.
Developing the software to detect and quantify bubble formation was necessary for the team’s analysis, Rufer explains. “We wanted to collect a lot of data and look at a lot of different electrodes and different reactions and different bubbles, and they all look slightly different,” he says. Creating a program that could deal with different materials and different lighting and reliably identify and track the bubbles was a tricky process, and machine learning was key to making it work, he says.
Using that tool, he says, they were able to collect “really significant amounts of data about the bubbles on a surface, where they are, how big they are, how fast they’re growing, all these different things.” The tool is now freely available for anyone to use via the GitHub repository.
By using that tool to correlate the visual measures of bubble formation and evolution with electrical measurements of the electrode’s performance, the researchers were able to disprove the accepted theory and to show that only the area of direct contact is affected. Videos further proved the point, revealing new bubbles actively evolving directly under parts of a larger bubble.
The researchers developed a very general methodology that can be applied to characterize and understand the impact of bubbles on any electrode or catalyst surface. They were able to quantify the bubble passivation effects in a new performance metric they call BECSA (Bubble-induced electrochemically active surface), as opposed to ECSA (electrochemically active surface area), that is used in the field. “The BECSA metric was a concept we defined in an earlier study but did not have an effective method to estimate until this work,” says Varanasi.
The knowledge that the area under bubbles can be significantly active ushers in a new set of design rules for high-performance electrodes. This means that electrode designers should seek to minimize bubble contact area rather than simply bubble coverage, which can be achieved by controlling the morphology and chemistry of the electrodes. Surfaces engineered to control bubbles can not only improve the overall efficiency of the processes and thus reduce energy use, they can also save on upfront materials costs. Many of these gas-evolving electrodes are coated with catalysts made of expensive metals like platinum or iridium, and the findings from this work can be used to engineer electrodes to reduce material wasted by reaction-blocking bubbles.
Varanasi says that “the insights from this work could inspire new electrode architectures that not only reduce the usage of precious materials, but also improve the overall electrolyzer performance,” both of which would provide large-scale environmental benefits.
The research team included Jim James, Nathan Pruyne, Aristana Scourtas, Marcus Schwarting, Aadit Ambalkar, Ian Foster, and Ben Blaiszik at the University of Chicago and Argonne National Laboratory. The work was supported by the U.S. Department of Energy under the ARPA-E program.
#Accounts#ai#aluminum#Analysis#bubbles#Capture#carbon#carbon capture#catalyst#catalysts#chemical#chemical reactions#chemicals#chemistry#Cleaner industry#climate change#Computer science and technology#contacts#data#deal#Department of Energy (DoE)#Design#designers#Difference Between#effects#efficiency#electricity#electrochemical#electrode#electrodes
0 notes
Text
weird/uncommon genres | dr ideas
date: december 16, 2024
Im never making a joke again 😭 after talking to my friend abt it, i feel better, but im still too scared. I thought poop jokes were childish and funny, like “your mom” 😭 regardless, nobody's seeing a joke from me ever again unless it’s on tiktok-- just to be clear tho, even if I found it funny, if the other party didn't, obviously the fault relies on me
I saw a guy get canceled for saying “your mom” too— though tbf it’s bc in Confucian countries it’s really bad to joke about your parents
sjfhdhsks I wanna cry…
Anyway, I haven't done these in awhile; I'm not sure if yall like my aethergarde academy posts more, these kinds of posts, or both (equally).
it's been awhile-- here's some weird ass genres you could make a DR from.
disclaimer: I used chatgpt (out of curiousity for some of these genres, those genres are made up and are not actual terms. Italicized ideas are ones from chatGPT. Guys it's unfair how good chatgpt is getting.. my brother told me that the goal of the current model is to have the AI simulate proper critical thinking instead of simply spitting out information.. isn't that crazy)
futuristic
cli-fi - this genre delves not only into climate change itself, but issues relating to the sun disappearing, or the world freezing. I remember seeing a shifter somewhere saying that she shifted here bc in her previous reality climate change was getting really really bad.
social sci-fi - focuses on how humans interact and behave in a futuristic setting.
planetary romance - exploration of different planets + romance, especially with an alien. Also characterized by distinctive extraterrestrial cultures and backgrounds.
data gothic - cyberpunk x gothic horror; characters encounter malevolent AI beings, digital ghosts, and corrupted data streams.
cosmic agriculture - genre focused on growing plant life in outer space or on different planets. Can also including breeding alien organisms (bacteria).
psychic noir - solve crimes in a world where memories, emotions, and thoughts could be hacked, manipulated, or weaponized. I think I'd make the memory thing extremely hard to do, since if it was too common I think it'd cause way too much havoc.
eco-metamorphosis - kinda like alien stage, but if you'd like, it could be less dark. This genre centers around earth being colonized by aliens, but the goal isn't to reject these changes, but rather to coexist with the other species.
liminal
slipstream - "speculative fiction that blends together science fiction, fantasy, and literary fiction or does not remain in conventional boundaries of genre and narrative"
abandoned intentions - explore incomplete worlds-- as if the world was abandoned mid-creation.
fantasy
lost world - discovering a hidden civilization, like atlantis or lumeria.
subterranean - a world that is primarily in an underground setting; similar to the hollow earth theory.
mythic/mythopeia - "fiction that is rooted in, inspired by, or that in some way draws from the tropes, themes, and symbolism of myth, legend, folklore, and fairy tales."; very similar to my wandering apocathary dr.
oceanic
nautical fiction - relationship between humans and the sea; "human relationship to the sea and sea voyages and highlights nautical culture in these environment".
wholesome/cute
furry sleuth - this is not about furries-- this is essentially a mystery where the main character is a household animal, typically a dog. Said animal would be the detective and solve mysteries.
cashier memoir - this genre always takes place in the head of a cashier. The goal is to come across as many different kinds of people as possible. This would be incredibly boring in this reality, but imagine if you were a barista in a fantasy or futuristic reality... you'd come across a lot of people without much effort or mental strain.
epistolary - a story told exclusively through fictional letters, newspaper articles, emails, and even texts. This isn't necessarily a genre of DR, but I think it'd be really interesting to guess/assume the plot of a DR through short snippets of letters or texts.
#shiftblr#reality shifting#shifting community#lalalian#shifting blog#desired reality#shifters#shifting diary#shifttok#scripting#shifting ideas
150 notes
·
View notes
Text
The Coprophagic AI crisis

I'm on tour with my new, nationally bestselling novel The Bezzle! Catch me in TORONTO on Mar 22, then with LAURA POITRAS in NYC on Mar 24, then Anaheim, and more!
A key requirement for being a science fiction writer without losing your mind is the ability to distinguish between science fiction (futuristic thought experiments) and predictions. SF writers who lack this trait come to fancy themselves fortune-tellers who SEE! THE! FUTURE!
The thing is, sf writers cheat. We palm cards in order to set up pulp adventure stories that let us indulge our thought experiments. These palmed cards – say, faster-than-light drives or time-machines – are narrative devices, not scientifically grounded proposals.
Historically, the fact that some people – both writers and readers – couldn't tell the difference wasn't all that important, because people who fell prey to the sf-as-prophecy delusion didn't have the power to re-orient our society around their mistaken beliefs. But with the rise and rise of sf-obsessed tech billionaires who keep trying to invent the torment nexus, sf writers are starting to be more vocal about distinguishing between our made-up funny stories and predictions (AKA "cyberpunk is a warning, not a suggestion"):
https://www.antipope.org/charlie/blog-static/2023/11/dont-create-the-torment-nexus.html
In that spirit, I'd like to point to how one of sf's most frequently palmed cards has become a commonplace of the AI crowd. That sleight of hand is: "add enough compute and the computer will wake up." This is a shopworn cliche of sf, the idea that once a computer matches the human brain for "complexity" or "power" (or some other simple-seeming but profoundly nebulous metric), the computer will become conscious. Think of "Mike" in Heinlein's *The Moon Is a Harsh Mistress":
https://en.wikipedia.org/wiki/The_Moon_Is_a_Harsh_Mistress#Plot
For people inflating the current AI hype bubble, this idea that making the AI "more powerful" will correct its defects is key. Whenever an AI "hallucinates" in a way that seems to disqualify it from the high-value applications that justify the torrent of investment in the field, boosters say, "Sure, the AI isn't good enough…yet. But once we shovel an order of magnitude more training data into the hopper, we'll solve that, because (as everyone knows) making the computer 'more powerful' solves the AI problem":
https://locusmag.com/2023/12/commentary-cory-doctorow-what-kind-of-bubble-is-ai/
As the lawyers say, this "cites facts not in evidence." But let's stipulate that it's true for a moment. If all we need to make the AI better is more training data, is that something we can count on? Consider the problem of "botshit," Andre Spicer and co's very useful coinage describing "inaccurate or fabricated content" shat out at scale by AIs:
https://papers.ssrn.com/sol3/papers.cfm?abstract_id=4678265
"Botshit" was coined last December, but the internet is already drowning in it. Desperate people, confronted with an economy modeled on a high-speed game of musical chairs in which the opportunities for a decent livelihood grow ever scarcer, are being scammed into generating mountains of botshit in the hopes of securing the elusive "passive income":
https://pluralistic.net/2024/01/15/passive-income-brainworms/#four-hour-work-week
Botshit can be produced at a scale and velocity that beggars the imagination. Consider that Amazon has had to cap the number of self-published "books" an author can submit to a mere three books per day:
https://www.theguardian.com/books/2023/sep/20/amazon-restricts-authors-from-self-publishing-more-than-three-books-a-day-after-ai-concerns
As the web becomes an anaerobic lagoon for botshit, the quantum of human-generated "content" in any internet core sample is dwindling to homeopathic levels. Even sources considered to be nominally high-quality, from Cnet articles to legal briefs, are contaminated with botshit:
https://theconversation.com/ai-is-creating-fake-legal-cases-and-making-its-way-into-real-courtrooms-with-disastrous-results-225080
Ironically, AI companies are setting themselves up for this problem. Google and Microsoft's full-court press for "AI powered search" imagines a future for the web in which search-engines stop returning links to web-pages, and instead summarize their content. The question is, why the fuck would anyone write the web if the only "person" who can find what they write is an AI's crawler, which ingests the writing for its own training, but has no interest in steering readers to see what you've written? If AI search ever becomes a thing, the open web will become an AI CAFO and search crawlers will increasingly end up imbibing the contents of its manure lagoon.
This problem has been a long time coming. Just over a year ago, Jathan Sadowski coined the term "Habsburg AI" to describe a model trained on the output of another model:
https://twitter.com/jathansadowski/status/1625245803211272194
There's a certain intuitive case for this being a bad idea, akin to feeding cows a slurry made of the diseased brains of other cows:
https://www.cdc.gov/prions/bse/index.html
But "The Curse of Recursion: Training on Generated Data Makes Models Forget," a recent paper, goes beyond the ick factor of AI that is fed on botshit and delves into the mathematical consequences of AI coprophagia:
https://arxiv.org/abs/2305.17493
Co-author Ross Anderson summarizes the finding neatly: "using model-generated content in training causes irreversible defects":
https://www.lightbluetouchpaper.org/2023/06/06/will-gpt-models-choke-on-their-own-exhaust/
Which is all to say: even if you accept the mystical proposition that more training data "solves" the AI problems that constitute total unsuitability for high-value applications that justify the trillions in valuation analysts are touting, that training data is going to be ever-more elusive.
What's more, while the proposition that "more training data will linearly improve the quality of AI predictions" is a mere article of faith, "training an AI on the output of another AI makes it exponentially worse" is a matter of fact.
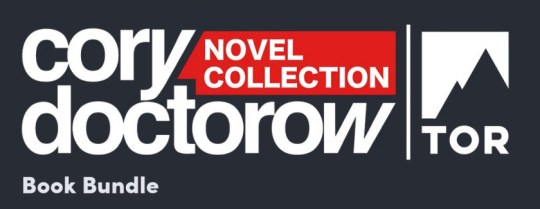
Name your price for 18 of my DRM-free ebooks and support the Electronic Frontier Foundation with the Humble Cory Doctorow Bundle.
If you'd like an essay-formatted version of this post to read or share, here's a link to it on pluralistic.net, my surveillance-free, ad-free, tracker-free blog:
https://pluralistic.net/2024/03/14/14/inhuman-centipede#enshittibottification
Image: Plamenart (modified) https://commons.wikimedia.org/wiki/File:Double_Mobius_Strip.JPG
CC BY-SA 4.0 https://creativecommons.org/licenses/by-sa/4.0/deed.en
#pluralistic#ai#generative ai#André Spicer#botshit#habsburg ai#jathan sadowski#ross anderson#inhuman centipede#science fiction#mysticism
554 notes
·
View notes
Text

Do Androids dream of electric sheep?

Pro:
Nurse Chapel episode! Interesting insight into her personal life and inner workings as well as her loyalties
Yes Dr. Korby we understand without you saying it. That's why the female Bot is wearing barely any clothes and is super beautiful. For sure.
Kirk on the Spinning wheel, woooooo!
DoubleKirk the second
I mean we have to mention the phallic stone right
unspoken Kirk and Spock communication and trust in each other! Kirks entire plan on the imposter-Kirk getting caught relies on Spock understanding what he's trying to say, and it works, I love that for them.
First real „Kirk is not the womanizer pop culture thinks he is“: he only kisses the woman / android to manipulate / get closer to his goal and not out of pleasure
The android design & clothes in the episode are great
Great funky lightchoices in the cavesystem
First time Androids, a classic sci-fi narrative, which in different ways explores what it means to be human and what makes humanity better than AI; In this case the concept of feelings (see quote) but also Ruk without empathy and based on facts deciding who gets to live or die
First time Kirk outsmarting stronger opponents instead of using force, happens especially often with AI (in this case both Androids)
The tension in the episode holds up, with Kirk being trapped in an unfamiliar environment, Chapel torn between her fiance and duty and the other characters being possible enemies
The reveal that Dr. Korby was also a cyborg was great

Look, the beautiful stone design needs to be in a review. Science.
Con
pacing was a little off at times
Other AI episodes have explored the humanity vs. AI theme better later in the series
Slight brush with the idea that you can cheat death / become immortal by becoming a computer / android, but it happens so close to the end that it can't be looked at close enough. How much of the person Korby is still in there? He clearly shows emotions unlike the other Androids
based on that: Ruk said that the androids were turned off, essentially killed, so it was self defense - if the androids have human rights. Again, this is not the focus of the episode, but does raise question the episode doesn't answer (this won't get explored until Data in TNG later on)

I just really loved this moment. Kirk is so small!
Counter
Shirtless Kirk (I mean completly naked Kirk, technically)
Kirk fake womanizer (uses kissing to get them out)
Evil AI (this time it's androids)
Brains over Brawl
Meme: Kirk orgasm face.gif
Quote: "Can you imagine how life could be improved if we could do away with jealousy, greed, hate?" - Corby "It can also be improved by eliminating love, tenderness, sentiment. The other side of the coin, Doctor" - Kirk
Moment: Kirk and Spock talking about his use of unseemlgy language at the end.
Summary: Good episode that introduces the classic AI / Android storyline as well as some of it's ethical connotations, shows Kirk's ingenuity and how he and Spock work together perfectly. Previous Episode - Next Episode - All TOS Reviews

free naked spinny wheel Kirk at the end!
20 notes
·
View notes
Text
Day 61 (2/2)
Zero Dawn Facility

Herres began with a revelation: nothing could save the world from the Faro plague. He went on to detail the projected rapid explosion of their population as they continued to self-replicate, consuming every living thing on the planet and degrading conditions until the air was poison and the lands uninhabitable. There was no hiding from the swarm; they would leave a barren earth behind them and at the first sign of returning life, they would wake from slumber and fuel themselves with whatever they could find. It would take decades to generate the swarm's deactivation codes, but the world had barely more than a year.

Operation Enduring Victory was a lie from the very beginning. There was no hope and there never had been. From the moment Elisabet reviewed Faro's data in Maker's End, she must have known. That explains why the War Chiefs at US Robot Command were so horrified with the idea of Enduring Victory. All they were doing—all those people standing between the swarm and the next city, fed false hope—was buying time for project Zero Dawn.

Then he revealed Elisabet's image, a small glimmer of hope. She was to explain the true purpose of the project in the next theatre. It didn't make any sense—if none of the Old Ones survived the swarm, then how did life continue?


I was right about the Shadow Carja. It seemed that the vents opening gave them other routes down. The kestrels were ignorant of the ruin's true nature, but I knew that once the Eclipse caught wind of the breach, they were certain to start salvaging data for Hades. I couldn't let them find Zero Dawn's secrets before I did.
I took a few of the kestrels out undetected before picking up one of their Firespitters and dispatching the rest.
The rooms were full of recorded interviews with various Zero Dawn 'candidates'. They were scholars, all from disparate fields of expertise. Experts in art and history, in environmental conservation, in robotics and engineering and artificial intelligence. There was a particularly colourful character named Travis Tate who I gathered was some sort of criminal. All of them were requested by Elisabet herself, and all of them had very different reactions to learning that all hope was lost. Anger, resignation, grief, confusion. Many questioned whether the swarm was truly unstoppable, who was culpable (a man named Tom Paech was out for Faro's blood), and why they of all people were chosen for the project.
They were all skeptical about what Elisabet could possibly have come up with to save a doomed world. One named Ron Felder latched onto the idea of a vehicle to take people away from Earth, a 'generation ship'. I never suspected that such a voyage was possible, though Ron didn't seem to think so either, at least that it could save only a handful of people if successful given the resources involved. One of the candidates was even a Faro employee himself, a lead developer of the Chariot line's self-replication procedures. He was overcome with guilt, eager to see his work quashed by some miracle of science.

In the next room, Elisabet's presentation began. She confirmed Herres' assessment of the situation, then expounded her solution.


A seed, she called it, from which new life could grow on a dead world: Gaia. An AI—a true AI, she said—engineered to deactivate the Faro robots and grow life on Earth anew. The central intelligence was joined by nine subordinate functions, not AIs in themselves but still complex software systems, each serving a different purpose. It was as their names were revealed in the presentation that some of my greatest questions were answered.

Hephaestus, and Hades. Both of them were part of Gaia, her subordinate functions. But if they were once part of the system, how did Hades end up in the body of a Horus leading a Carja cult, and how did Hephaestus end up scattered across Cauldrons and infecting Cyan?
Only a few of the functions were mentioned: Hephaestus was meant to build the machines of the wilds, Aether to purify the air, Poseidon the water, and Minerva generated the codes that deactivated the swarm decades after the Old Ones fell—and its tower pictured looked just like the Spire. Artemis spawned animals, many of the holographic silhouettes familiar to me from the wilds, and Eleuthia...it spawned human beings in 'Cradle Facilities'. The presentation didn't explain how.

One function stood out to me: Apollo. It was meant to be a repository of all human knowledge that the children of the Cradle Facilities would learn from. The picture showed a Focus used to channel this knowledge, but what happened to it all? Could it still be intact somewhere? Whatever happened, that knowledge never made it to the people of the tribes, and Elisabet's hope that the people of the future wouldn't repeat the mistakes of the Old Ones was dashed.

Elisabet ended her presentation by imploring the candidates to join the project and help Gaia achieve a rejuvenated earth, and I can't deny her words were rousing. It was a beautiful dream, daringly ambitious, perhaps even close to impossible given what little I know about Old World technology. At the very least, creating a 'true' AI was strictly forbidden by all corporations, but at the end of everything, what did those regulations matter? What better to combat a machine of destruction than a machine of creation, a true peacekeeper?

Moving on, I came to another holding area. All candidates who experienced the presentations were given three choices to decide their fate. First, to comply and participate in the project, working with their assigned team to build Gaia and her subordinate functions. If they refused to comply, they would be detained indefinitely, without a chance to reconsider after two days' time. Given they knew the truth about Operation Enduring Victory, they could not be allowed to leave and spread that information. It seemed cruel, but I can understand the need for strict secrecy. If people found out that there was no hope for humanity as they knew it, they would be likely to throw down their arms and try to end their lives as peacefully as possible, instead of in pain and fear in resistance against the swarm.

The third option was medical euthanasia; to die painlessly, alone, holding this secret forever. I saw the room where it happened. One bed, cold metal, cold lights striping the walls.
The interviews recorded showed all three reactions. Some were eager to contribute, willing to give all they could for a slim chance to save the future. The Faro employee, for instance, was thankful for a chance to undo his mistakes. Others were more skeptical but still willing to try, while others found the idea ridiculous. Ron Felder thought it even more far-fetched than space flight, calling the whole thing nothing more than a fantasy with a crazy hologram display to match. I gathered he was detained.
Perhaps the most interesting reaction was that of Travis Tate. He called the whole notion of trying to re-engineer the natural world unnatural, even abominable. Another reason that they couldn't let the secret out was that others would agree with him. I suppose I can see his point, but surely it was worth trying. Benefit of hindsight, I guess. Having no conception of what the natural world once was, it's beautiful to me. Maybe to them it would feel wrong, artificial.

More kestrels further on, all touting Firespitters. Once one was down the rest were easy prey.


Unfortunately I was right about the Eclipse. The deeper reaches of the ruin were already crawling with them. I was hoping to sneak around and climb the elevator shaft to Elisabet's office above, where the most classified data was sure to be kept, but the entrance was sealed. There was no way I could search for other exits and go undetected. I tried to take out the Eclipse unseen, but was spotted and they called for reinforcements, running in from neighboring chambers and rappelling from the upper level. It was a tough scrap, but there was plenty of cover. I lost them easily in the dark and was able to sneak around. The real problem was that now they knew I was here.

While exploring I came across the workstation for the Hephaestus function. There was a holographic recording of its director, Margo Shen, an ex-colleague of Elisabet at FAS before they both left the company. She explained that their job wasn't to design machines, but to program Gaia to use the tools and data contained within Hephaestus to design machines of her own. Hephaestus was clearly never meant to be intelligent, to feel emotions of its own—so what happened to it?
Sylens suggested it might have gone 'rogue' like the swarm and stopped answering orders. Maybe Gaia is out there, unable to communicate with the tribes or get Hephaestus and Hades back under control. If Hephaestus struck out on its own when the Derangement began, when did Hades escape—the same time?
I recognised some of the mechanical structures and holographic designs I found from Cauldrons, iterated on and grown wild. Everything was beginning to make sense.

Further on I found a similar recording of the leader of Apollo, Samina Ebadji. She spoke of collating, organising, and storing records of human knowledge across millions of different topics, and building a learning interface where the people of the future could gain that knowledge through a game-like process. Such an important station, authoring what would shape the future of human civilisation—choosing what would be remembered and what should lay forgotten. From the datapoints I discovered, the project was running on schedule and they were planning to store the data in 'synthetic DNA'. If Samina's project didn't fail, then what happened to it?

More Eclipse in the next chamber, which I dispatched undetected. I found a recording from General Herres—some sort of testimonial, an apology for the lies and injustices of Operation Enduring Victory. I can't imagine the guilt, but it was the right thing to do. It has to be, or nothing would have survived. The swarm would have swallowed this facility long before Gaia's completion.
In more datapoints I found, Margo and the other function leaders were named 'Alpha candidates'. Back in All-Mother mountain, when the door denied me, it said that the Alpha registry was corrupted. Was it trying to match my identity to the list of Alpha candidates on the project? If that piece of data was part of all Zero Dawn's associated facilities, I suspected I could find it here as well.

Next, I entered Hades' old domain and listened to an introduction by Travis Tate, the eccentric 'hacker'. From what I could gather, his crimes consisted of exposing the dark secrets of corporations and orchestrating sort of technological pranks on a global scale. Elisabet trusted him enough to put him in charge of the Hades function, but judging by what's happening now his work left something to be desired.
Hades was Gaia's extinction function. 'Apocalypse on speed dial', Travis called it, the idea being that Gaia was unlikely to engineer a perfect Earth-like biosphere on the first go. It was a delicate balance to recreate, and in the event of firestorms and poison skies irrevocably locked in a cycle of chaos, Hades was built to temporarily take control of the system away from Gaia and undo terraforming operations, returning Earth to the barren rock it was when the swarm subsided so Gaia could begin again. One of the datapoints spoke of a facility where Gaia and Hades were tested together, where Travis tried to engineer the correct feedback and reward behaviour to ensure the entities passed control back and forth as desired. It seems that of all the subordinate functions, Hades was the most intelligent, as it needed to be able to manipulate the other functions in event of a catastrophe. Though, again, it was never intended to possess emotional responses.
But Gaia succeeded long ago, why would Hades wake now? Did it try to take control, causing Gaia to expel it from the larger system? If it no longer had Gaia's tools and functions available to it, it seems logical that its next best course to undo Gaia's work would be to wake the Faro bots themselves to resume their gruesome conquest. Thanks for that, Travis.


Next on my journey to Elisabet's office, I passed Eleuthia's headquarters and listened to an address from its leader, Patrick Brochard-Klein. He spoke of perfecting the technology to create humans inside machines, and to build 'servitors', robotic guardians resembling humans themselves, who would care for the new generations inside their Cradles. He stressed that this was a project of preservation, not 'genetic engineering', and spoke of regulations around...cloning, which he refused to transgress. Clone, as in copy.
There were many 'ectogenic chambers' inside the workshop—the machines from which new humans were spawned. The logs I found said they were donated by Far Zenith, whose conception of the technology was far ahead of public scientific records. I've come across a few datapoints mentioning the organisation before, sometimes called simply 'FZ'. They were a mysterious group of billionaires (a word for the wealthiest and most powerful of the Old Ones), their identities unknown to all outside the group. They had their own 'think tanks' and scientists developing technology in secret, much like a corporation, or a co-merging of many corporations, using the exhaustive profits of them all. I know they bought a space station called the Odyssey, though when I first read this I didn't understand what it meant. From Ron Felder's tirade, I knew then. A structure built to travel away from this world, through the stars. With the most advanced technology and near-infinite resources available, could they have succeeded?
Thoughts gathered in the back of my mind that I wished to ignore. It was clear to me that All-Mother mountain must have been one of these Cradle facilities, given the ruin inside and the bunker door, answerable to Elisabet. Every Nora myth pointed to this too: the life-giving goddess within, and its battle against the Metal Devil. Perhaps some knowledge of the Old Ones did survive and was imparted to the first new people of the earth. And of course...I came from that mountain. I was found just outside its doors, alone. I suppose some of the first people might have remained behind the door. Perhaps there was a schism long ago, or they're protecting the Apollo archive inside. Maybe helping Gaia to operate. But why wouldn't they try to communicate with the world outside?


Finally, I made it to Elisabet's workshop. There were a number of preserved recordings inside. The first, an argument between Elisabet and Ted. I'd hazard a guess that this happened often. To think that he still had the gall to argue with her after everything he did...I suppose she had to put up with it considering he was funding the operation.
Faro was disputing Elisabet's assertion that Gaia had to have complex emotional capabilities to properly rebuild and safeguard the world. She needed 'skin in the game', Elisabet said—I suppose if she felt no emotional connection to life, human or otherwise, she'd have no incentive to put their needs first, or the means to conceptualise their needs accurately to begin with. Intelligence and emotion go hand in hand, as Cyan explained.
Faro was wary of 'repeating past mistakes'. I'm don't think he was only talking about the swarm, because they had no emotional capacity themselves, only strategic intelligence. He wanted a kill switch, which I gathered ended up being Hades—a way to wrest control from a version of Gaia gone wrong. Gaia herself chimed in and agreed with him, understanding her own volatility. Her voice was beautiful: smooth and deep and full of care. Maybe it was only a clever trick of programming in those early days, but she sounded like she had developed emotions already. Her projection didn't match the figure I saw in Elisabet's presentation though. She was just a golden ball of liquid light.


More recordings, the first of Elisabet and Gaia discussing her emotional responses to mass extinction events of the ancient past. It seemed her development was going well, but it was cut short. In the final recording, Elisabet was panicked, erratic, irritable. She was trying to lock everything down and evacuate the facility before the swarm hit, evidently ahead of projected schedule. Gaia comforted her, and for the first time I witnessed a moment of weakness in Elisabet. She lamented all the lives lost in Enduring Victory—all those deaths, those lies, would be for nothing if she couldn't complete Gaia in time.

In Elisabet's office, along with plenty more data to sift through, I found just what I was looking for: an intact copy of the Alpha registry. I made the mistake of voicing my wish to meet my mother inside All-Mother mountain's Cradle, which Sylens shut down with derision. He said there would be no people behind that door, only machines. I refused to believe it.

As I was downloading a copy to my Focus, more Eclipse soldiers began to descend from above. I couldn't leave until the transfer was complete, and just as it did I went to make my escape, but behind the glass was Helis. We locked eyes for the first time since the Proving. Before I could react, he threw a bomb and detonated it in front of the glass, blasting it inward and knocking me back against the desk.

I only fell unconscious for a few moments, but it was enough for Helis to land at my side, glare smiling with those pale, manic eyes, and kick me back into the darkness.
#god i was dreading writing this one. so much lore#hzd#horizon zero dawn#aloy#aloy sobeck#aloysjournal#hzd remastered#photomode#virtual photography#horizon
12 notes
·
View notes
Text
Prometheus Gave the Gift of Fire to Mankind. We Can't Give it Back, nor Should We.
AI. Artificial intelligence. Large Language Models. Learning Algorithms. Deep Learning. Generative Algorithms. Neural Networks. This technology has many names, and has been a polarizing topic in numerous communities online. By my observation, a lot of the discussion is either solely focused on A) how to profit off it or B) how to get rid of it and/or protect yourself from it. But to me, I feel both of these perspectives apply a very narrow usage lens on something that's more than a get rich quick scheme or an evil plague to wipe from the earth.
This is going to be long, because as someone whose degree is in psych and computer science, has been a teacher, has been a writing tutor for my younger brother, and whose fiance works in freelance data model training... I have a lot to say about this.
I'm going to address the profit angle first, because I feel most people in my orbit (and in related orbits) on Tumblr are going to agree with this: flat out, the way AI is being utilized by large corporations and tech startups -- scraping mass amounts of visual and written works without consent and compensation, replacing human professionals in roles from concept art to story boarding to screenwriting to customer service and more -- is unethical and damaging to the wellbeing of people, would-be hires and consumers alike. It's wasting energy having dedicated servers running nonstop generating content that serves no greater purpose, and is even pressing on already overworked educators because plagiarism just got a very new, harder to identify younger brother that's also infinitely more easy to access.
In fact, ChatGPT is such an issue in the education world that plagiarism-detector subscription services that take advantage of how overworked teachers are have begun paddling supposed AI-detectors to schools and universities. Detectors that plainly DO NOT and CANNOT work, because the difference between "A Writer Who Writes Surprisingly Well For Their Age" is indistinguishable from "A Language Replicating Algorithm That Followed A Prompt Correctly", just as "A Writer Who Doesn't Know What They're Talking About Or Even How To Write Properly" is indistinguishable from "A Language Replicating Algorithm That Returned Bad Results". What's hilarious is that the way these "detectors" work is also run by AI.
(to be clear, I say plagiarism detectors like TurnItIn.com and such are predatory because A) they cost money to access advanced features that B) often don't work properly or as intended with several false flags, and C) these companies often are super shady behind the scenes; TurnItIn for instance has been involved in numerous lawsuits over intellectual property violations, as their services scrape (or hopefully scraped now) the papers submitted to the site without user consent (or under coerced consent if being forced to use it by an educator), which it uses in can use in its own databases as it pleases, such as for training the AI detecting AI that rarely actually detects AI.)
The prevalence of visual and lingustic generative algorithms is having multiple, overlapping, and complex consequences on many facets of society, from art to music to writing to film and video game production, and even in the classroom before all that, so it's no wonder that many disgruntled artists and industry professionals are online wishing for it all to go away and never come back. The problem is... It can't. I understand that there's likely a large swath of people saying that who understand this, but for those who don't: AI, or as it should more properly be called, generative algorithms, didn't just show up now (they're not even that new), and they certainly weren't developed or invented by any of the tech bros peddling it to megacorps and the general public.
Long before ChatGPT and DALL-E came online, generative algorithms were being used by programmers to simulate natural processes in weather models, shed light on the mechanics of walking for roboticists and paleontologists alike, identified patterns in our DNA related to disease, aided in complex 2D and 3D animation visuals, and so on. Generative algorithms have been a part of the professional world for many years now, and up until recently have been a general force for good, or at the very least a force for the mundane. It's only recently that the technology involved in creating generative algorithms became so advanced AND so readily available, that university grad students were able to make the publicly available projects that began this descent into madness.
Does anyone else remember that? That years ago, somewhere in the late 2010s to the beginning of the 2020s, these novelty sites that allowed you to generate vague images from prompts, or generate short stylistic writings from a short prompt, were popping up with University URLs? Oftentimes the queues on these programs were hours long, sometimes eventually days or weeks or months long, because of how unexpectedly popular this concept was to the general public. Suddenly overnight, all over social media, everyone and their grandma, and not just high level programming and arts students, knew this was possible, and of course, everyone wanted in. Automated art and writing, isn't that neat? And of course, investors saw dollar signs. Simply scale up the process, scrape the entire web for data to train the model without advertising that you're using ALL material, even copyrighted and personal materials, and sell the resulting algorithm for big money. As usual, startup investors ruin every new technology the moment they can access it.
To most people, it seemed like this magic tech popped up overnight, and before it became known that the art assets on later models were stolen, even I had fun with them. I knew how learning algorithms worked, if you're going to have a computer make images and text, it has to be shown what that is and then try and fail to make its own until it's ready. I just, rather naively as I was still in my early 20s, assumed that everything was above board and the assets were either public domain or fairly licensed. But when the news did came out, and when corporations started unethically implementing "AI" in everything from chatbots to search algorithms to asking their tech staff to add AI to sliced bread, those who were impacted and didn't know and/or didn't care where generative algorithms came from wanted them GONE. And like, I can't blame them. But I also quietly acknowledged to myself that getting rid of a whole technology is just neither possible nor advisable. The cat's already out of the bag, the genie has left its bottle, the Pandorica is OPEN. If we tried to blanket ban what people call AI, numerous industries involved in making lives better would be impacted. Because unfortunately the same tool that can edit selfies into revenge porn has also been used to identify cancer cells in patients and aided in decoding dead languages, among other things.
When, in Greek myth, Prometheus gave us the gift of fire, he gave us both a gift and a curse. Fire is so crucial to human society, it cooks our food, it lights our cities, it disposes of waste, and it protects us from unseen threats. But fire also destroys, and the same flame that can light your home can burn it down. Surely, there were people in this mythic past who hated fire and all it stood for, because without fire no forest would ever burn to the ground, and surely they would have called for fire to be given back, to be done away with entirely. Except, there was no going back. The nature of life is that no new element can ever be undone, it cannot be given back.
So what's the way forward, then? Like, surely if I can write a multi-paragraph think piece on Tumblr.com that next to nobody is going to read because it's long as sin, about an unpopular topic, and I rarely post original content anyway, then surely I have an idea of how this cyberpunk dystopia can be a little less.. Dys. Well I do, actually, but it's a long shot. Thankfully, unlike business majors, I actually had to take a cyber ethics course in university, and I actually paid attention. I also passed preschool where I learned taking stuff you weren't given permission to have is stealing, which is bad. So the obvious solution is to make some fucking laws to limit the input on data model training on models used for public products and services. It's that simple. You either use public domain and licensed data only or you get fined into hell and back and liable to lawsuits from any entity you wronged, be they citizen or very wealthy mouse conglomerate (suing AI bros is the only time Mickey isn't the bigger enemy). And I'm going to be honest, tech companies are NOT going to like this, because not only will it make doing business more expensive (boo fucking hoo), they'd very likely need to throw out their current trained datasets because of the illegal components mixed in there. To my memory, you can't simply prune specific content from a completed algorithm, you actually have to redo rhe training from the ground up because the bad data would be mixed in there like gum in hair. And you know what, those companies deserve that. They deserve to suffer a punishment, and maybe fold if they're young enough, for what they've done to creators everywhere. Actually, laws moving forward isn't enough, this needs to be retroactive. These companies need to be sued into the ground, honestly.
So yeah, that's the mess of it. We can't unlearn and unpublicize any technology, even if it's currently being used as a tool of exploitation. What we can do though is demand ethical use laws and organize around the cause of the exclusive rights of individuals to the content they create. The screenwriter's guild, actor's guild, and so on already have been fighting against this misuse, but given upcoming administration changes to the US, things are going to get a lot worse before thet get a little better. Even still, don't give up, have clear and educated goals, and focus on what you can do to affect change, even if right now that's just individual self-care through mental and physical health crises like me.
#ai#artificial intelligence#generative algorithms#llm#large language model#chatgpt#ai art#ai writing#kanguin original
9 notes
·
View notes
Text

AI helps distinguish dark matter from cosmic noise
Dark matter is the invisible force holding the universe together – or so we think. It makes up around 85% of all matter and around 27% of the universe’s contents, but since we can’t see it directly, we have to study its gravitational effects on galaxies and other cosmic structures. Despite decades of research, the true nature of dark matter remains one of science’s most elusive questions.
According to a leading theory, dark matter might be a type of particle that barely interacts with anything else, except through gravity. But some scientists believe these particles could occasionally interact with each other, a phenomenon known as self-interaction. Detecting such interactions would offer crucial clues about dark matter’s properties.
However, distinguishing the subtle signs of dark matter self-interactions from other cosmic effects, like those caused by active galactic nuclei (AGN) – the supermassive black holes at the centers of galaxies – has been a major challenge. AGN feedback can push matter around in ways that are similar to the effects of dark matter, making it difficult to tell the two apart.
In a significant step forward, astronomer David Harvey at EPFL’s Laboratory of Astrophysics has developed a deep-learning algorithm that can untangle these complex signals. Their AI-based method is designed to differentiate between the effects of dark matter self-interactions and those of AGN feedback by analyzing images of galaxy clusters – vast collections of galaxies bound together by gravity. The innovation promises to greatly enhance the precision of dark matter studies.
Harvey trained a Convolutional Neural Network (CNN) – a type of AI that is particularly good at recognizing patterns in images – with images from the BAHAMAS-SIDM project, which models galaxy clusters under different dark matter and AGN feedback scenarios. By being fed thousands of simulated galaxy cluster images, the CNN learned to distinguish between the signals caused by dark matter self-interactions and those caused by AGN feedback.
Among the various CNN architectures tested, the most complex - dubbed “Inception” – proved to also be the most accurate. The AI was trained on two primary dark matter scenarios, featuring different levels of self-interaction, and validated on additional models, including a more complex, velocity-dependent dark matter model.
Inceptionachieved an impressive accuracy of 80% under ideal conditions, effectively identifying whether galaxy clusters were influenced by self-interacting dark matter or AGN feedback. It maintained is high performance even when the researchers introduced realistic observational noise that mimics the kind of data we expect from future telescopes like Euclid.
What this means is that Inception – and the AI approach more generally – could prove incredibly useful for analyzing the massive amounts of data we collect from space. Moreover, the AI’s ability to handle unseen data indicates that it’s adaptable and reliable, making it a promising tool for future dark matter research.
AI-based approaches like Inception could significantly impact our understanding of what dark matter actually is. As new telescopes gather unprecedented amounts of data, this method will help scientists sift through it quickly and accurately, potentially revealing the true nature of dark matter.
10 notes
·
View notes
Text
"In the oldest and most prestigious young adult science competition in the nation, 17-year-old Ellen Xu used a kind of AI to design the first diagnosis test for a rare disease that struck her sister years ago.
With a personal story driving her on, she managed an 85% rate of positive diagnoses with only a smartphone image, winning her $150,000 grand for a third-place finish.
Kawasaki disease has no existing test method, and relies on a physician’s years of training, ability to do research, and a bit of luck.
Symptoms tend to be fever-like and therefore generalized across many different conditions. Eventually if undiagnosed, children can develop long-term heart complications, such as the kind that Ellen’s sister was thankfully spared from due to quick diagnosis.
Xu decided to see if there were a way to design a diagnostic test using deep learning for her Regeneron Science Talent Search medicine and health project. Organized since 1942, every year 1,900 kids contribute adventures.
She designed what is known as a convolutional neural network, which is a form of deep-learning algorithm that mimics how our eyes work, and programmed it to analyze smartphone images for potential Kawasaki disease.
However, like our own eyes, a convolutional neural network needs a massive amount of data to be able to effectively and quickly process images against references.
For this reason, Xu turned to crowdsourcing images of Kawasaki’s disease and its lookalike conditions from medical databases around the world, hoping to gather enough to give the neural network a high success rate.
Xu has demonstrated an 85% specificity in identifying between Kawasaki and non-Kawasaki symptoms in children with just a smartphone image, a demonstration that saw her test method take third place and a $150,000 reward at the Science Talent Search."
-Good News Network, 3/24/23
#heart disease#pediatrics#healthcare#kawasaki#neural network#ai#science and technology#rare disease#medical tests#medical science#good news#hope
75 notes
·
View notes
Text
What are AI, AGI, and ASI? And the positive impact of AI
Understanding artificial intelligence (AI) involves more than just recognizing lines of code or scripts; it encompasses developing algorithms and models capable of learning from data and making predictions or decisions based on what they’ve learned. To truly grasp the distinctions between the different types of AI, we must look at their capabilities and potential impact on society.
To simplify, we can categorize these types of AI by assigning a power level from 1 to 3, with 1 being the least powerful and 3 being the most powerful. Let’s explore these categories:
1. Artificial Narrow Intelligence (ANI)
Also known as Narrow AI or Weak AI, ANI is the most common form of AI we encounter today. It is designed to perform a specific task or a narrow range of tasks. Examples include virtual assistants like Siri and Alexa, recommendation systems on Netflix, and image recognition software. ANI operates under a limited set of constraints and can’t perform tasks outside its specific domain. Despite its limitations, ANI has proven to be incredibly useful in automating repetitive tasks, providing insights through data analysis, and enhancing user experiences across various applications.
2. Artificial General Intelligence (AGI)
Referred to as Strong AI, AGI represents the next level of AI development. Unlike ANI, AGI can understand, learn, and apply knowledge across a wide range of tasks, similar to human intelligence. It can reason, plan, solve problems, think abstractly, and learn from experiences. While AGI remains a theoretical concept as of now, achieving it would mean creating machines capable of performing any intellectual task that a human can. This breakthrough could revolutionize numerous fields, including healthcare, education, and science, by providing more adaptive and comprehensive solutions.
3. Artificial Super Intelligence (ASI)
ASI surpasses human intelligence and capabilities in all aspects. It represents a level of intelligence far beyond our current understanding, where machines could outthink, outperform, and outmaneuver humans. ASI could lead to unprecedented advancements in technology and society. However, it also raises significant ethical and safety concerns. Ensuring ASI is developed and used responsibly is crucial to preventing unintended consequences that could arise from such a powerful form of intelligence.
The Positive Impact of AI
When regulated and guided by ethical principles, AI has the potential to benefit humanity significantly. Here are a few ways AI can help us become better:
• Healthcare: AI can assist in diagnosing diseases, personalizing treatment plans, and even predicting health issues before they become severe. This can lead to improved patient outcomes and more efficient healthcare systems.
• Education: Personalized learning experiences powered by AI can cater to individual student needs, helping them learn at their own pace and in ways that suit their unique styles.
• Environment: AI can play a crucial role in monitoring and managing environmental changes, optimizing energy use, and developing sustainable practices to combat climate change.
• Economy: AI can drive innovation, create new industries, and enhance productivity by automating mundane tasks and providing data-driven insights for better decision-making.
In conclusion, while AI, AGI, and ASI represent different levels of technological advancement, their potential to transform our world is immense. By understanding their distinctions and ensuring proper regulation, we can harness the power of AI to create a brighter future for all.
8 notes
·
View notes
Text
What's the difference between Machine Learning and AI?
Machine Learning and Artificial Intelligence (AI) are often used interchangeably, but they represent distinct concepts within the broader field of data science. Machine Learning refers to algorithms that enable systems to learn from data and make predictions or decisions based on that learning. It's a subset of AI, focusing on statistical techniques and models that allow computers to perform specific tasks without explicit programming.

On the other hand, AI encompasses a broader scope, aiming to simulate human intelligence in machines. It includes Machine Learning as well as other disciplines like natural language processing, computer vision, and robotics, all working towards creating intelligent systems capable of reasoning, problem-solving, and understanding context.
Understanding this distinction is crucial for anyone interested in leveraging data-driven technologies effectively. Whether you're exploring career opportunities, enhancing business strategies, or simply curious about the future of technology, diving deeper into these concepts can provide invaluable insights.
In conclusion, while Machine Learning focuses on algorithms that learn from data to make decisions, Artificial Intelligence encompasses a broader range of technologies aiming to replicate human intelligence. Understanding these distinctions is key to navigating the evolving landscape of data science and technology. For those eager to deepen their knowledge and stay ahead in this dynamic field, exploring further resources and insights on can provide valuable perspectives and opportunities for growth
5 notes
·
View notes
Text
The Mathematical Foundations of Machine Learning
In the world of artificial intelligence, machine learning is a crucial component that enables computers to learn from data and improve their performance over time. However, the math behind machine learning is often shrouded in mystery, even for those who work with it every day. Anil Ananthaswami, author of the book "Why Machines Learn," sheds light on the elegant mathematics that underlies modern AI, and his journey is a fascinating one.
Ananthaswami's interest in machine learning began when he started writing about it as a science journalist. His software engineering background sparked a desire to understand the technology from the ground up, leading him to teach himself coding and build simple machine learning systems. This exploration eventually led him to appreciate the mathematical principles that underlie modern AI. As Ananthaswami notes, "I was amazed by the beauty and elegance of the math behind machine learning."
Ananthaswami highlights the elegance of machine learning mathematics, which goes beyond the commonly known subfields of calculus, linear algebra, probability, and statistics. He points to specific theorems and proofs, such as the 1959 proof related to artificial neural networks, as examples of the beauty and elegance of machine learning mathematics. For instance, the concept of gradient descent, a fundamental algorithm used in machine learning, is a powerful example of how math can be used to optimize model parameters.
Ananthaswami emphasizes the need for a broader understanding of machine learning among non-experts, including science communicators, journalists, policymakers, and users of the technology. He believes that only when we understand the math behind machine learning can we critically evaluate its capabilities and limitations. This is crucial in today's world, where AI is increasingly being used in various applications, from healthcare to finance.
A deeper understanding of machine learning mathematics has significant implications for society. It can help us to evaluate AI systems more effectively, develop more transparent and explainable AI systems, and address AI bias and ensure fairness in decision-making. As Ananthaswami notes, "The math behind machine learning is not just a tool, but a way of thinking that can help us create more intelligent and more human-like machines."
The Elegant Math Behind Machine Learning (Machine Learning Street Talk, November 2024)
youtube
Matrices are used to organize and process complex data, such as images, text, and user interactions, making them a cornerstone in applications like Deep Learning (e.g., neural networks), Computer Vision (e.g., image recognition), Natural Language Processing (e.g., language translation), and Recommendation Systems (e.g., personalized suggestions). To leverage matrices effectively, AI relies on key mathematical concepts like Matrix Factorization (for dimension reduction), Eigendecomposition (for stability analysis), Orthogonality (for efficient transformations), and Sparse Matrices (for optimized computation).
The Applications of Matrices - What I wish my teachers told me way earlier (Zach Star, October 2019)
youtube
Transformers are a type of neural network architecture introduced in 2017 by Vaswani et al. in the paper “Attention Is All You Need”. They revolutionized the field of NLP by outperforming traditional recurrent neural network (RNN) and convolutional neural network (CNN) architectures in sequence-to-sequence tasks. The primary innovation of transformers is the self-attention mechanism, which allows the model to weigh the importance of different words in the input data irrespective of their positions in the sentence. This is particularly useful for capturing long-range dependencies in text, which was a challenge for RNNs due to vanishing gradients. Transformers have become the standard for machine translation tasks, offering state-of-the-art results in translating between languages. They are used for both abstractive and extractive summarization, generating concise summaries of long documents. Transformers help in understanding the context of questions and identifying relevant answers from a given text. By analyzing the context and nuances of language, transformers can accurately determine the sentiment behind text. While initially designed for sequential data, variants of transformers (e.g., Vision Transformers, ViT) have been successfully applied to image recognition tasks, treating images as sequences of patches. Transformers are used to improve the accuracy of speech-to-text systems by better modeling the sequential nature of audio data. The self-attention mechanism can be beneficial for understanding patterns in time series data, leading to more accurate forecasts.
Attention is all you need (Umar Hamil, May 2023)
youtube
Geometric deep learning is a subfield of deep learning that focuses on the study of geometric structures and their representation in data. This field has gained significant attention in recent years.
Michael Bronstein: Geometric Deep Learning (MLSS Kraków, December 2023)
youtube
Traditional Geometric Deep Learning, while powerful, often relies on the assumption of smooth geometric structures. However, real-world data frequently resides in non-manifold spaces where such assumptions are violated. Topology, with its focus on the preservation of proximity and connectivity, offers a more robust framework for analyzing these complex spaces. The inherent robustness of topological properties against noise further solidifies the rationale for integrating topology into deep learning paradigms.
Cristian Bodnar: Topological Message Passing (Michael Bronstein, August 2022)
youtube
Sunday, November 3, 2024
#machine learning#artificial intelligence#mathematics#computer science#deep learning#neural networks#algorithms#data science#statistics#programming#interview#ai assisted writing#machine art#Youtube#lecture
4 notes
·
View notes
Text
With Picard season 3 ending very soon, let's review the endings to modern Trek, and see the chances of it ending well. Or rather, you read what I think.
Discovery S1: Flat as a pancake end to the Klingon war, following epic and satisfying ends to the Klingon sarcophagus ship and Lorca's mutiny storylines. Massive backstage unheavel meant the end and beginning had very different creative direction. 1/5.
Discovery S2: I loved it, a massive epic space battle, a big emotional farewell between Spock and Michael (argubly the first time Discovery paused a massive crisis for a therapeutic chitchat, which would go on to become a tiresome cliche) and great time travel scenes even though the Red Angel did things Michael never could have (like disable the Ba'ul technology as if by magic) so it doesn't work if you look closely. Still, more creative upheaval and rumours of a massive change in creative direction mid-season, supposedly dropping a faith vs science plotline which would have featured a religious Captain Pike butting heads with Michael for the fun but cliche Control/Skynet story. Set up Section 31, Strange New Worlds spin-offs and Discovery's jump to the 32nd century. 5/5 despite flaws I was buzzing afterwards.
Picard S1: A flat ending to the synth storyline, a weird choice to kill Picard and make him a synth (perhaps a fix job for an originally planned sacrifice they had to work around when they decided to carry the show on?) and with some solid gold scenes with Picard and Data in the weird synth computer simulation afterlife. 2/5.
Discovery S3: A fun end with big fist fights in an impossible hammerspace between Discovery's decks. 3/5.
Picard S2. The final scenes between Q and Picard were solid gold. The rest was runny shite. 1/5.
Discovery S4. Big alien aliens. Deus ex machina brings Book back from the dead. Book gets community service for terrorist activities. Peace is made with the floaty giant aliens. Flat again, as was the whole season IMHO. 2/5.
Lower Decks S1. I don't remember. 3/5 it was always fun and watchable.
Strange New Worlds S1: The Ghost of Christmas Future and a reboot of Balance of Terror. Iffy Jim Kirk casting. 4/5.
Lower Decks S2. I remember Carol's arrest. 3/5.
Prodigy S1. Amazing season, amazing finale. Every emotional beat was earned. 5/5 the best of modern Trek, watch it if you haven't.
Lower Decks S3. Loved the race between Cerritos and the Texas-class. But holy shit Starfleet needs to stop with letting AI control anything important. The end with the Cali-class saving the say was ace. 4/5.
Don't fuck it up, Picard S3 people. Your season has been an amazing, contrived, fanwank explosion clusterfuck that somehow works really well so far. Don't ruin it.
56 notes
·
View notes
Text
Language Models and AI Safety: Still Worrying
Previously, I have explained how modern "AI" research has painted itself into a corner, inventing the science fiction rogue AI scenario where a system is smarter than its guardrails, but can easily outwitted by humans.
Two recent examples have confirmed my hunch about AI safety of generative AI. In one well-circulated case, somebody generated a picture of an "ethnically ambiguous Homer Simpson", and in another, somebody created a picture of "baby, female, hispanic".
These incidents show that generative AI still filters prompts and outputs, instead of A) ensuring the correct behaviour during training/fine-tuning, B) manually generating, re-labelling, or pruning the training data, C) directly modifying the learned weights to affect outputs.
In general, it is not surprising that big corporations like Google and Microsoft and non-profits like OpenAI are prioritising racist language or racial composition of characters in generated images over abuse of LLMs or generative art for nefarious purposes, content farms, spam, captcha solving, or impersonation. Somebody with enough criminal energy to use ChatGPT to automatically impersonate your grandma based on your message history after he hacked the phones of tens of thousands of grandmas will be blamed for his acts. Somebody who unintentionally generates a racist picture based on an ambiguous prompt will blame the developers of the software if he's offended. Scammers could have enough money and incentives to run the models on their own machine anyway, where corporations have little recourse.
There is precedent for this. Word2vec, published in 2013, was called a "sexist algorithm" in attention-grabbing headlines, even though the bodies of such articles usually conceded that the word2vec embedding just reproduced patterns inherent in the training data: Obviously word2vec does not have any built-in gender biases, it just departs from the dictionary definitions of words like "doctor" and "nurse" and learns gendered connotations because in the training corpus doctors are more often men, and nurses are more often women. Now even that last explanation is oversimplified. The difference between "man" and "woman" is not quite the same as the difference between "male" and "female", or between "doctor" and "nurse". In the English language, "man" can mean "male person" or "human person", and "nurse" can mean "feeding a baby milk from your breast" or a kind of skilled health care worker who works under the direction and supervision of a licensed physician. Arguably, the word2vec algorithm picked up on properties of the word "nurse" that are part of the meaning of the word (at least one meaning, according tot he dictionary), not properties that are contingent on our sexist world.
I don't want to come down against "political correctness" here. I think it's good if ChatGPT doesn't tell a girl that girls can't be doctors. You have to understand that not accidentally saying something sexist or racist is a big deal, or at least Google, Facebook, Microsoft, and OpenAI all think so. OpenAI are responding to a huge incentive when they add snippets like "ethnically ambiguous" to DALL-E 3 prompts.
If this is so important, why are they re-writing prompts, then? Why are they not doing A, B, or C? Back in the days of word2vec, there was a simple but effective solution to automatically identify gendered components in the learned embedding, and zero out the difference. It's so simple you'll probably kick yourself reading it because you could have published that paper yourself without understanding how word2vec works.
I can only conclude from the behaviour of systems like DALL-E 3 that they are either using simple prompt re-writing (or a more sophisticated approach that behaves just as prompt rewriting would, and performs as badly) because prompt re-writing is the best thing they can come up with. Transformers are complex, and inscrutable. You can't just reach in there, isolate a concept like "human person", and rebalance the composition.
The bitter lesson tells us that big amorphous approaches to AI perform better and scale better than manually written expert systems, ontologies, or description logics. More unsupervised data beats less but carefully labelled data. Even when the developers of these systems have a big incentive not to reproduce a certain pattern from the data, they can't fix such a problem at the root. Their solution is instead to use a simple natural language processing system, a dumb system they can understand, and wrap it around the smart but inscrutable transformer-based language model and image generator.
What does that mean for "sleeper agent AI"? You can't really trust a model that somebody else has trained, but can you even trust a model you have trained, if you haven't carefully reviewed all the input data? Even OpenAI can't trust their own models.
16 notes
·
View notes
Text
PC Engine - Asuka 120% Burning Fest. Maxima
Title: Asuka 120% Burning Fest. Maxima / あすか120% マキシマ BURNING Fest. MAXIMA
Developer: Fill-in-Cafe / FamilySoft
Publisher: NEC Avenue
Release date: 28 July 1995
Catalogue No.: NAPR-1049
Genre: 1-v-1 Fighting
Format: Super CD-ROM2
The first-ever console port of Asuka 120% there is out there, following the original computer versions for the FM Towns and Sharp X68000.
Ryoran Girls' Private Academy is a high-quality school, that specializes in arts and sciences, but also in martial arts. Each year the most successful students hold a fighting tournament. Each girl represents her specific area of interest (science, sports, music, etc.), and each wants to be the great winner, for her club's and her own glory.
This game is not particularly story-driven; the story mode allows the player to choose any of the ten available girls, each featuring a short introduction, followed by a linear progression of battles against computer-controlled rivals, with a few bits of dialogue before each battle. The versus mode allows the player to compete against any opponent controlled by a computer, as well as two-player battles and a "watch mode", in which the player assigns fighters to computer AI and watches how they unfold.
The combat system follows a rather standard fighting game scheme; the girls execute a variety of kicks, punches, and attacks with special weapons, by pressing combinations of buttons. Girls might have unique weapons and techniques which are associated with the club/subject they represent; for example, a "tomboy" athletic girl will attack with baseballs and other sports accessories, etc.
Note: This game is officially certified to only use the Super CD-ROM2 System Card in its stock configuration, but there is a hidden Arcade Card CD-ROM2 mode. The differences between using the Super CD-ROM2 and the Arcade Card system cards seem to boil down to a reduction in loading times. The way to access it is as follows.
Once you turn on the console with the Arcade Card and the disc inserted, press the following button combination as soon as the BIOS screen flashes the "PUSH RUN BUTTON" text.
The button combination is LEFT + BUTTON "II" + RUN. Press both three buttons together, and the game will boot to a screen where it checks for the Arcade Card.
If it detects that you are using an Arcade Card, it will ask you if you want to make use of it. Press BUTTON "I" for Yes.
After selecting Yes, it will load a bunch of data to the Arcade Card.
youtube
3 notes
·
View notes