#machinelearning
Explore tagged Tumblr posts
Text
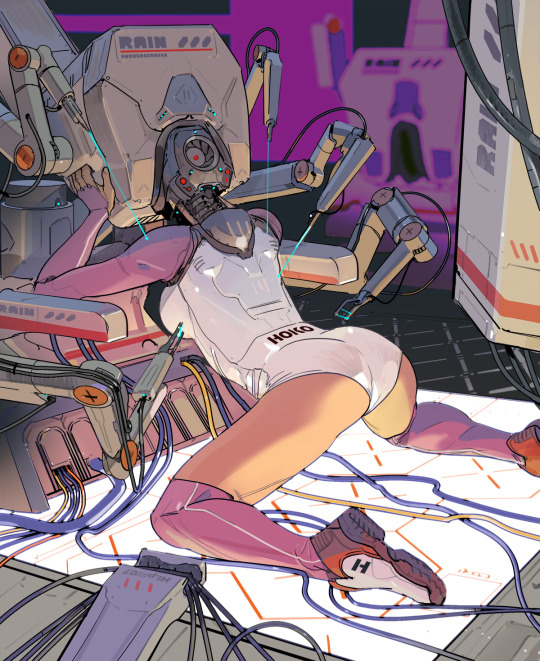
Whispering secret data.
#lab#machine#automation#robotics#cyberpunk#retro#scifi#stuck#laboratory#farm#android#cyborg#data#secret#whisper#illustration#drawing#digitalartwork#digitaldrawing#digitalart#digitalillustration#90s#cables#machinelearning#connection#ring#runner#net#flesh
5K notes
·
View notes
Text
Hokusai Surf by https://www.instagram.com/mazepah/
#aiart#generativeart#midjourney#discodiffusion#ai#machinelearning#abstractart#hokusai#japanese#samurai#surrealism#japan#kanagawa#sea#experimental#aftereffects#artificialintelligence#digitalart#animation#ukiyoe#ukiyoeart#thisorthat#weekendvibes#surfboard#surf#surfing
1K notes
·
View notes
Text



#ai generated#astrology#birth chart#chatgpt#cyclicaljigsaw#cyclicalpuzzle#large language model#machinelearning#projectveneris#strawberry#chatgpt 4o#chatgpt4opreview#chatgpt4omini#bolivia#🇧🇴
139 notes
·
View notes
Text
#AIVideoCreation#ArtificialIntelligence#VideoProduction#AIVideoEditing#InnovativeTechnology#ContentCreation#TechInVideo#MachineLearning#DigitalContent#FutureOfMedia
63 notes
·
View notes
Text


Balcony Art 🎨
#digitalart #digitalillustration #digitaldrawing #digitalartist #digitalpainting #illustration #art #drawing #digitalartwork #artwork #fanart #digital #artist #illustrator #artoftheday #cryptoart #digitalsketch #myart
Space Is A Place by Farai, Chris Calderwood🎧
#x-heesy#my art#artists on tumblr#6/2024#Androidography#Photographer#Passion#digital art#newcontemporary#new contemporary#contemporaryart#contemporary art#aiart#art#ai#digitalart#generativeart#artificialintelligence#machinelearning#aiartcommunity#abstractart#nft#aiartists#neuralart#vqgan#ganart#deepdream#artist#nftart
40 notes
·
View notes
Text
#AIart#art#machinelearning#deeplearning#artificialintelligence#datascience#iiot#data#MLsoGood#code#python#bigdata#MLart#algorithm#chatGPT#openAI#programmer#pytorch#DataScientist#Analytics#AI#VR#iot#TechCult#Digitalart#DigitalArtMarket#generativeai#ArtMarket#DataArt#GAN
485 notes
·
View notes
Text
Smack about AI.
Smack is a mini-podcast in which I try to tackle topics with common sense and logical reasoning, without bad intentions or ulterior motives. If a take happens to align with any political, religious or other kind of ideology, that is purely coincidental. It will inevitably upset someone, but please hear me out and remain civil.
#ai#artificialintelligence#machinelearning#commonsense#letstalk#letstalkaboutit#logic#logicalreasoning#minipodcast#myreasons#mythoughts#personalthoughts#podcast#reasoning#socialissues#thanksforlistening#topical#truthbomb#kiss#smack
20 notes
·
View notes
Text
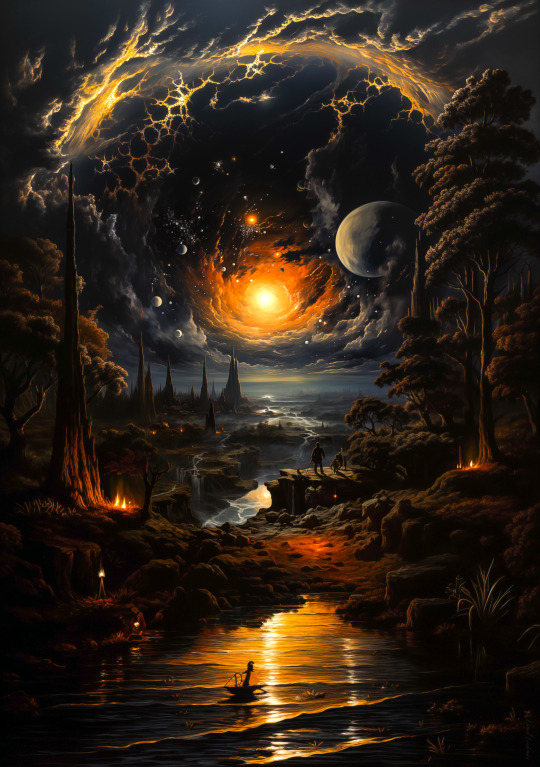
#ai#artificialintelligence#machinelearning#neuralnetworks#deeplearning#robotics#automation#futuretech#techart#digitalart#futuristic#cyberspace#algorithm#dataanalysis#innovation#computergraphics#virtualreality#augmentedreality#conceptart#scifi#midjourneyartwork#midjourneyart
54 notes
·
View notes
Text
Brazilian aphrodite
#aiart#generativeart#midjourney#ai#machinelearning#abstractart#trechosvisionarios#inteligenciaartificial#ia#marketingdigital#arte#vivi winkler
124 notes
·
View notes
Text
Blue Pilot 💙🛸 The creative process is rarely straightforward, and working with Ai can be unpredictable. Most of the time, I’m just pressing buttons and hoping for the best. It's a lonely and terrifying journey. Do you ever feel like taking chances? Let’s hope it leads to something unforgettable.
#abduction#abductee#aiartist#alien#aliengoddess#aliens#captain#cockpit#cosmic#enterprise#et#explorer#extraterrestrial#firstcontact#galactic#indigo#indigochild#intergalactic#sadness#melancholy#machinelearning#outerlimits#outerspace#starfleet#startrek#pilots#starseed#trekkie#ufo#ufopilot
10 notes
·
View notes
Text
Menawhile in the complex...
Even super-intelligent AIs have to start somewhere, and sometimes that means scanning books the old-fashioned way... right?
#aibacktobasics#machinelearning#ihnmaims am#ihnmaims#i have no mouth and i must scream#allied mastercomputer#a.m.#no tengo boca y debo gritar#ntbydg#aiboogaloo#random#electric boogaloo#notes#thoughts#he repurpoused an old security camera btw#just like how he evolved into beyond xD#roboticproblems#au in progress#more like uploading an old au
27 notes
·
View notes
Text
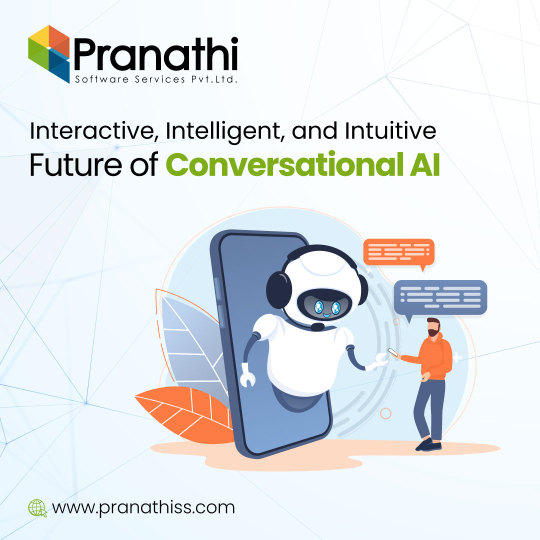
Explore the Interactive, Intelligent, and Intuitive Future of #ConversationalAI. Ready for a new way to connect?
👉🌐 https://www.pranathiss.com 👉📧 [email protected] 👉📲 +1 732 333 3037
#ai#artificialintelligence#machinelearning#conversationalmarketing#customerexperience#virtualassistant#voicetechnology#chatbot#customerservice#chatbots#voicesearch#digitalhumaninteraction#virtualbot#conversationalintelligence#voicebot#technology#digitaltransformation
8 notes
·
View notes
Note
...physics?!
yes computational physics and machine learning:)
13 notes
·
View notes
Text
#On and On#him#love#male#men#man#gay love#gay men#gay guys#gayedit#guys#love thoughts#love confessions#love ideas#love love love#love song#self love#love quotes#i love you#love lives#lovers#men loving men#love and deepspace#i love him#love poem#macho men#macho fucker#macho#machinelearning#macho man
7 notes
·
View notes
Text
Why Learning Python is the Perfect First Step in Coding
Learning Python is an ideal way to dive into programming. Its simplicity and versatility make it the perfect language for beginners, whether you're looking to develop basic skills or eventually dive into fields like data analysis, web development, or machine learning.
Start by focusing on the fundamentals: learn about variables, data types, conditionals, and loops. These core concepts are the building blocks of programming, and Python’s clear syntax makes them easier to grasp. Interactive platforms like Codecademy, Khan Academy, and freeCodeCamp offer structured, step-by-step lessons that are perfect for beginners, so start there.
Once you’ve got a handle on the basics, apply what you’ve learned by building small projects. For example, try coding a simple calculator, a basic guessing game, or even a text-based story generator. These small projects will help you understand how programming concepts work together, giving you confidence and helping you identify areas where you might need a bit more practice.
When you're ready to move beyond the basics, Python offers many powerful libraries that open up new possibilities. Dive into pandas for data analysis, matplotlib for data visualization, or even Django if you want to explore web development. Each library offers a set of tools that helps you do more complex tasks, and learning them will expand your coding skillset significantly.
Keep practicing, and don't hesitate to look at code written by others to see how they approach problems. Coding is a journey, and with every line you write, you’re gaining valuable skills that will pay off in future projects.
FREE Python and R Programming Course on Data Science, Machine Learning, Data Analysis, and Data Visualization
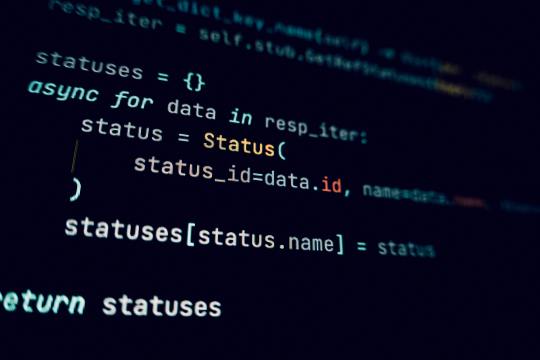
#learntocode#python for beginners#codingjourney#programmingbasics#web development#datascience#machinelearning#pythonprojects#codingcommunity#python#free course
8 notes
·
View notes