#data science and machine learning
Explore tagged Tumblr posts
Text
Elevate Your Career with a Data Science and Machine Learning Course Online
Are you looking to advance your career in the dynamic field of data science and machine learning? Consider enrolling in an online course! These programs offer flexible schedules and comprehensive curriculums to equip you with the latest skills and techniques. Visit our official website to learn more.
0 notes
Text
Complete Excel, AI and Data Science mega bundle.
Unlock Your Full Potential with Our 100-Hour Masterclass: The Ultimate Guide to Excel, Python, and AI.
Why Choose This Course? In todayâs competitive job market, mastering a range of technical skills is more important than ever. Our 100-hour comprehensive course is designed to equip you with in-demand capabilities in Excel, Python, and Artificial Intelligence (AI), providing you with the toolkit you need to excel in the digital age.
To read more click here <<
Become an Excel Pro Delve deep into the intricacies of Excel functions, formulae, and data visualization techniques. Whether youâre dealing with basic tasks or complex financial models, this course will make you an Excel wizard capable of tackling any challenge.
Automate Your Workflow with Python Scripting in Python doesnât just mean writing code; it means reclaiming your time. Automate everyday tasks, interact with software applications, and boost your productivity exponentially.
If you want to get full course click here <<
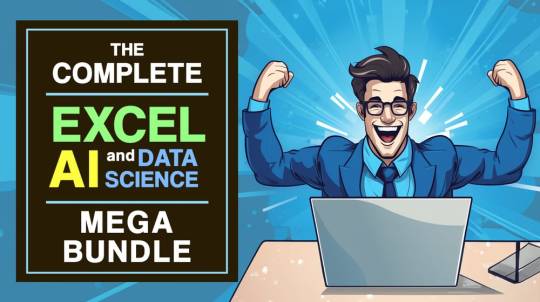
Turn Ideas into Apps Discover the potential of Amazon Honeycode to create custom apps tailored to your needs. Whether itâs for data management, content tracking, or inventory â transform your creative concepts into practical solutions.
Be Your Own Financial Analyst Unlock the financial functionalities of Excel to manage and analyze business data. Create Profit and Loss statements, balance sheets, and conduct forecasting with ease, equipping you to make data-driven decisions.
Embark on an AI Journey Step into the future with AI and machine learning. Learn to build advanced models, understand neural networks, and employ TensorFlow. Turn big data into actionable insights and predictive models.
Master Stock Prediction Gain an edge in the market by leveraging machine learning for stock prediction. Learn to spot trends, uncover hidden patterns, and make smarter investment decisions.
Who Is This Course For? Whether youâre a complete beginner or a seasoned professional looking to upskill, this course offers a broad and deep understanding of Excel, Python, and AI, preparing you for an ever-changing work environment.
Invest in Your Future This isnât just a course; itâs a game-changer for your career. Enroll now and set yourself on a path to technological mastery and unparalleled career growth.
Donât Wait, Transform Your Career Today! Click here to get full course <<
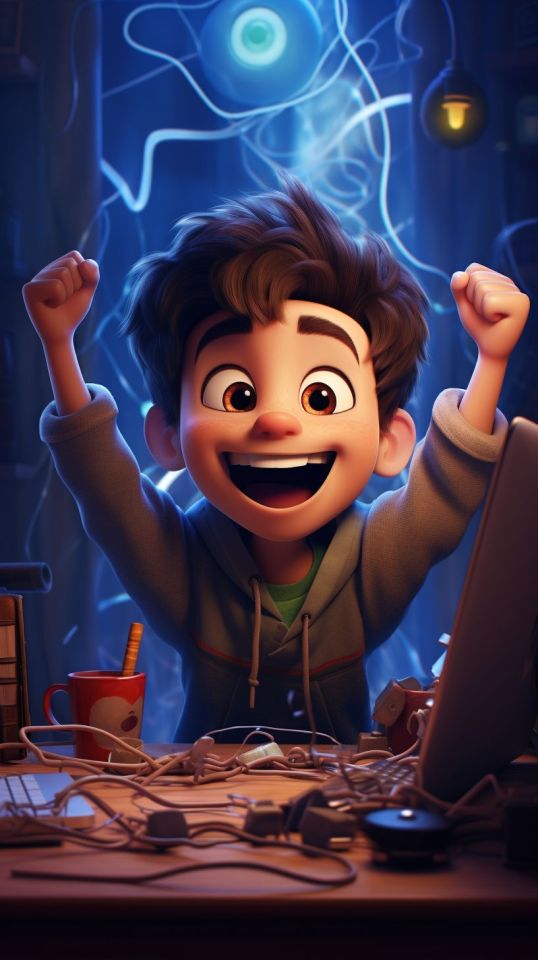
#data science#complete excel course#excel#data science and machine learning#microsoft excel#difference between ai and data science#learn excel#complete microsoft excel tutorial#difference between data science and data engineering#365 data science#aegis school of data science#advanced excel#excel tips and tricks#advanced excel full course#computer science#ms in data science#pgp in data science#python data science#python data science tutorial#Tumblr
1 note
¡
View note
Text
#datascience#Data Science and Machine Learning#Big Data Certification#Big Data Analytics Courses#Data Science Training#Data Science for Beginners#Data Science Online Training#Data Analysis Training#Data Science Course Near me#Data Science Classes
1 note
¡
View note
Text
The surprising truth about data-driven dictatorships
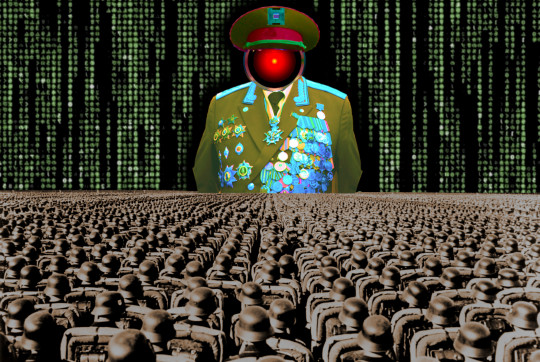
Hereâs the âdictatorâs dilemmaâ: they want to block their countryâs frustrated elites from mobilizing against them, so they censor public communications; but they also want to know what their people truly believe, so they can head off simmering resentments before they boil over into regime-toppling revolutions.
These two strategies are in tension: the more you censor, the less you know about the true feelings of your citizens and the easier it will be to miss serious problems until they spill over into the streets (think: the fall of the Berlin Wall or Tunisia before the Arab Spring). Dictators try to square this circle with things like private opinion polling or petition systems, but these capture a small slice of the potentially destabiziling moods circulating in the body politic.
Enter AI: back in 2018, Yuval Harari proposed that AI would supercharge dictatorships by mining and summarizing the public moodâââas captured on social mediaâââallowing dictators to tack into serious discontent and diffuse it before it erupted into unequenchable wildfire:
https://www.theatlantic.com/magazine/archive/2018/10/yuval-noah-harari-technology-tyranny/568330/
Harari wrote that âthe desire to concentrate all information and power in one place may become [dictators] decisive advantage in the 21st century.â But other political scientists sharply disagreed. Last year, Henry Farrell, Jeremy Wallace and Abraham Newman published a thoroughgoing rebuttal to Harari in Foreign Affairs:
https://www.foreignaffairs.com/world/spirals-delusion-artificial-intelligence-decision-making
They argued thatâââlike everyone who gets excited about AI, only to have their hopes dashedâââdictators seeking to use AI to understand the public mood would run into serious training data bias problems. After all, people living under dictatorships know that spouting off about their discontent and desire for change is a risky business, so they will self-censor on social media. Thatâs true even if a person isnât afraid of retaliation: if you know that using certain words or phrases in a post will get it autoblocked by a censorbot, whatâs the point of trying to use those words?
The phrase âGarbage In, Garbage Outâ dates back to 1957. Thatâs how long weâve known that a computer that operates on bad data will barf up bad conclusions. But this is a very inconvenient truth for AI weirdos: having given up on manually assembling training data based on careful human judgment with multiple review steps, the AI industry âpivotedâ to mass ingestion of scraped data from the whole internet.
But adding more unreliable data to an unreliable dataset doesnât improve its reliability. GIGO is the iron law of computing, and you canât repeal it by shoveling more garbage into the top of the training funnel:
https://memex.craphound.com/2018/05/29/garbage-in-garbage-out-machine-learning-has-not-repealed-the-iron-law-of-computer-science/
When it comes to âAIâ thatâs used for decision supportâââthat is, when an algorithm tells humans what to do and they do itâââthen you get something worse than Garbage In, Garbage Outâââyou get Garbage In, Garbage Out, Garbage Back In Again. Thatâs when the AI spits out something wrong, and then another AI sucks up that wrong conclusion and uses it to generate more conclusions.
To see this in action, consider the deeply flawed predictive policing systems that cities around the world rely on. These systems suck up crime data from the cops, then predict where crime is going to be, and send cops to those âhotspotsâ to do things like throw Black kids up against a wall and make them turn out their pockets, or pull over drivers and search their cars after pretending to have smelled cannabis.
The problem here is that âcrime the police detectedâ isnât the same as âcrime.â You only find crime where you look for it. For example, there are far more incidents of domestic abuse reported in apartment buildings than in fully detached homes. Thatâs not because apartment dwellers are more likely to be wife-beaters: itâs because domestic abuse is most often reported by a neighbor who hears it through the walls.
So if your cops practice racially biased policing (I know, this is hard to imagine, but stay with me /s), then the crime they detect will already be a function of bias. If you only ever throw Black kids up against a wall and turn out their pockets, then every knife and dime-bag you find in someoneâs pockets will come from some Black kid the cops decided to harass.
Thatâs life without AI. But now letâs throw in predictive policing: feed your âknives found in pocketsâ data to an algorithm and ask it to predict where there are more knives in pockets, and it will send you back to that Black neighborhood and tell you do throw even more Black kids up against a wall and search their pockets. The more you do this, the more knives youâll find, and the more youâll go back and do it again.
This is what Patrick Ball from the Human Rights Data Analysis Group calls âempiricism washingâ: take a biased procedure and feed it to an algorithm, and then you get to go and do more biased procedures, and whenever anyone accuses you of bias, you can insist that youâre just following an empirical conclusion of a neutral algorithm, because âmath canât be racist.â
HRDAG has done excellent work on this, finding a natural experiment that makes the problem of GIGOGBI crystal clear. The National Survey On Drug Use and Health produces the gold standard snapshot of drug use in America. Kristian Lum and William Isaac took Oaklandâs drug arrest data from 2010 and asked Predpol, a leading predictive policing product, to predict where Oaklandâs 2011 drug use would take place.
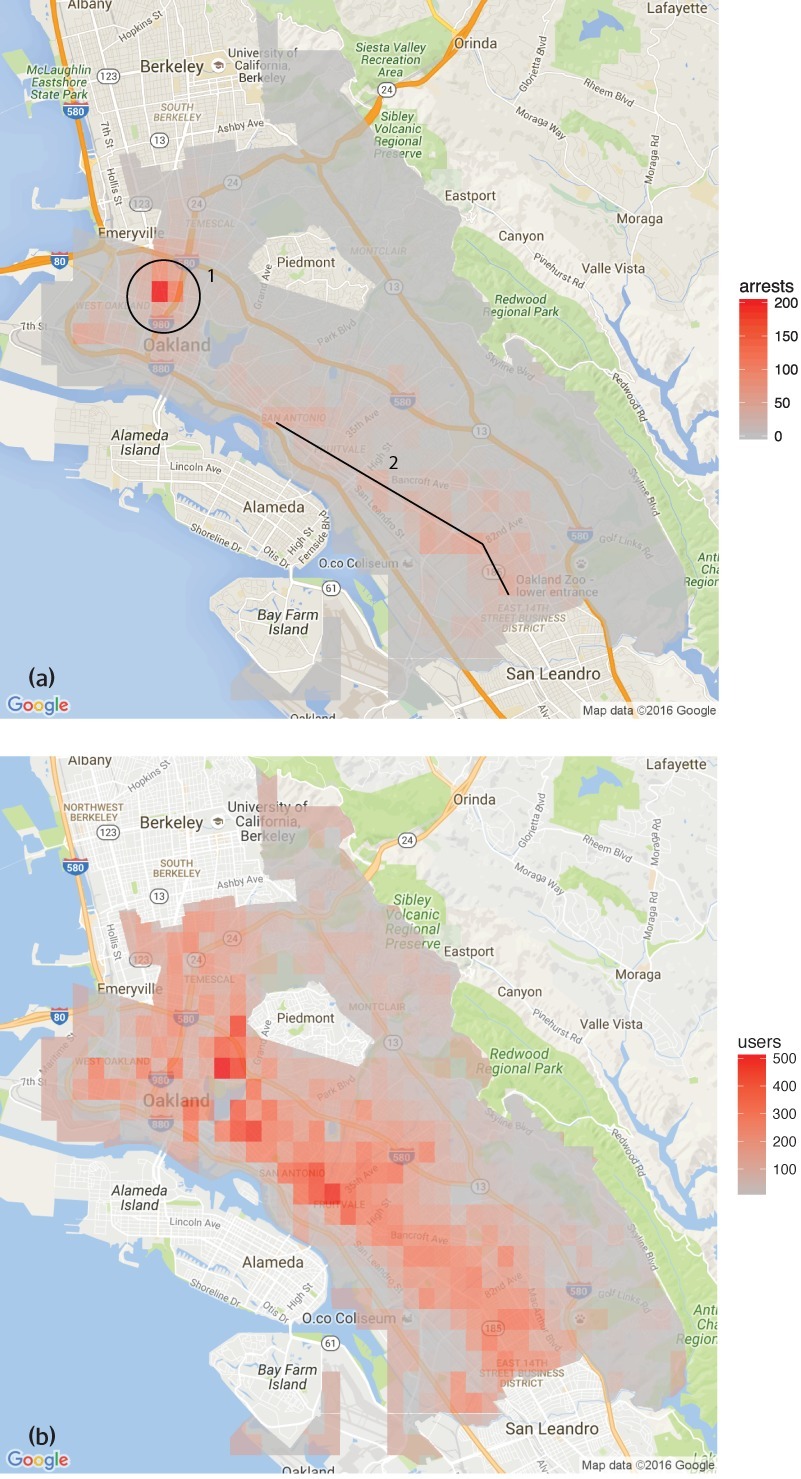
[Image ID: (a) Number of drug arrests made by Oakland police department, 2010. (1) West Oakland, (2) International Boulevard. (b) Estimated number of drug users, based on 2011 National Survey on Drug Use and Health]
Then, they compared those predictions to the outcomes of the 2011 survey, which shows where actual drug use took place. The two maps couldnât be more different:
https://rss.onlinelibrary.wiley.com/doi/full/10.1111/j.1740-9713.2016.00960.x
Predpol told cops to go and look for drug use in a predominantly Black, working class neighborhood. Meanwhile the NSDUH survey showed the actual drug use took place all over Oakland, with a higher concentration in the Berkeley-neighboring student neighborhood.
Whatâs even more vivid is what happens when you simulate running Predpol on the new arrest data that would be generated by cops following its recommendations. If the cops went to that Black neighborhood and found more drugs there and told Predpol about it, the recommendation gets stronger and more confident.
In other words, GIGOGBI is a system for concentrating bias. Even trace amounts of bias in the original training data get refined and magnified when they are output though a decision support system that directs humans to go an act on that output. Algorithms are to bias what centrifuges are to radioactive ore: a way to turn minute amounts of bias into pluripotent, indestructible toxic waste.
Thereâs a great name for an AI thatâs trained on an AIâs output, courtesy of Jathan Sadowski: âHabsburg AI.â
And that brings me back to the Dictatorâs Dilemma. If your citizens are self-censoring in order to avoid retaliation or algorithmic shadowbanning, then the AI you train on their posts in order to find out what theyâre really thinking will steer you in the opposite direction, so you make bad policies that make people angrier and destabilize things more.
Or at least, that was Farrell(et al)âs theory. And for many years, thatâs where the debate over AI and dictatorship has stalled: theory vs theory. But now, thereâs some empirical data on this, thanks to the âThe Digital Dictatorâs Dilemma,â a new paper from UCSD PhD candidate Eddie Yang:
https://www.eddieyang.net/research/DDD.pdf
Yang figured out a way to test these dueling hypotheses. He got 10 million Chinese social media posts from the start of the pandemic, before companies like Weibo were required to censor certain pandemic-related posts as politically sensitive. Yang treats these posts as a robust snapshot of public opinion: because there was no censorship of pandemic-related chatter, Chinese users were free to post anything they wanted without having to self-censor for fear of retaliation or deletion.
Next, Yang acquired the censorship model used by a real Chinese social media company to decide which posts should be blocked. Using this, he was able to determine which of the posts in the original set would be censored today in China.
That means that Yang knows that the ârealâ sentiment in the Chinese social media snapshot is, and what Chinese authorities would believe it to be if Chinese users were self-censoring all the posts that would be flagged by censorware today.
From here, Yang was able to play with the knobs, and determine how âpreference-falsificationâ (when users lie about their feelings) and self-censorship would give a dictatorship a misleading view of public sentiment. What he finds is that the more repressive a regime isâââthe more people are incentivized to falsify or censor their viewsâââthe worse the system gets at uncovering the true public mood.
Whatâs more, adding additional (bad) data to the system doesnât fix this âmissing dataâ problem. GIGO remains an iron law of computing in this context, too.
But it gets better (or worse, I guess): Yang models a âcrisisâ scenario in which users stop self-censoring and start articulating their true views (because theyâve run out of fucks to give). This is the most dangerous moment for a dictator, and depending on the dictatorship handles it, they either get another decade or rule, or they wake up with guillotines on their lawns.
But âcrisisâ is where AI performs the worst. Trained on the âstatus quoâ data where users are continuously self-censoring and preference-falsifying, AI has no clue how to handle the unvarnished truth. Both its recommendations about what to censor and its summaries of public sentiment are the least accurate when crisis erupts.
But hereâs an interesting wrinkle: Yang scraped a bunch of Chinese usersâ posts from Twitterâââwhich the Chinese government doesnât get to censor (yet) or spy on (yet)âââand fed them to the model. He hypothesized that when Chinese users post to American social media, they donât self-censor or preference-falsify, so this data should help the model improve its accuracy.
He was rightâââthe model got significantly better once it ingested data from Twitter than when it was working solely from Weibo posts. And Yang notes that dictatorships all over the world are widely understood to be scraping western/northern social media.
But even though Twitter data improved the modelâs accuracy, it was still wildly inaccurate, compared to the same model trained on a full set of un-self-censored, un-falsified data. GIGO is not an option, itâs the law (of computing).
Writing about the study on Crooked Timber, Farrell notes that as the world fills up with âgarbage and noiseâ (he invokes Philip K Dickâs delighted coinage âgubbishâ), âapproximately correct knowledge becomes the scarce and valuable resource.â
https://crookedtimber.org/2023/07/25/51610/
This âprobably approximately correct knowledgeâ comes from humans, not LLMs or AI, and so âthe social applications of machine learning in non-authoritarian societies are just as parasitic on these forms of human knowledge production as authoritarian governments.â
The Clarion Science Fiction and Fantasy Writersâ Workshop summer fundraiser is almost over! I am an alum, instructor and volunteer board member for this nonprofit workshop whose alums include Octavia Butler, Kim Stanley Robinson, Bruce Sterling, Nalo Hopkinson, Kameron Hurley, Nnedi Okorafor, Lucius Shepard, and Ted Chiang! Your donations will help us subsidize tuition for students, making Clarionâââand sf/fâââmore accessible for all kinds of writers.
Libro.fm is the indie-bookstore-friendly, DRM-free audiobook alternative to Audible, the Amazon-owned monopolist that locks every book you buy to Amazon forever. When you buy a book on Libro, they share some of the purchase price with a local indie bookstore of your choosing (Libro is the best partner I have in selling my own DRM-free audiobooks!). As of today, Libro is even better, because itâs available in five new territories and currencies: Canada, the UK, the EU, Australia and New Zealand!
[Image ID: An altered image of the Nuremberg rally, with ranked lines of soldiers facing a towering figure in a many-ribboned soldier's coat. He wears a high-peaked cap with a microchip in place of insignia. His head has been replaced with the menacing red eye of HAL9000 from Stanley Kubrick's '2001: A Space Odyssey.' The sky behind him is filled with a 'code waterfall' from 'The Matrix.']
Image: Cryteria (modified) https://commons.wikimedia.org/wiki/File:HAL9000.svg
CC BY 3.0 https://creativecommons.org/licenses/by/3.0/deed.en
âââ
Raimond Spekking (modified) https://commons.wikimedia.org/wiki/File:Acer_Extensa_5220_-_Columbia_MB_06236-1N_-_Intel_Celeron_M_530_-_SLA2G_-_in_Socket_479-5029.jpg
CC BY-SA 4.0 https://creativecommons.org/licenses/by-sa/4.0/deed.en
âââ
Russian Airborne Troops (modified) https://commons.wikimedia.org/wiki/File:Vladislav_Achalov_at_the_Airborne_Troops_Day_in_Moscow_%E2%80%93_August_2,_2008.jpg
âSoldiers of Russiaâ Cultural Center (modified) https://commons.wikimedia.org/wiki/File:Col._Leonid_Khabarov_in_an_everyday_service_uniform.JPG
CC BY-SA 3.0 https://creativecommons.org/licenses/by-sa/3.0/deed.en
#pluralistic#habsburg ai#self censorship#henry farrell#digital dictatorships#machine learning#dictator's dilemma#eddie yang#preference falsification#political science#training bias#scholarship#spirals of delusion#algorithmic bias#ml#Fully automated data driven authoritarianism#authoritarianism#gigo#garbage in garbage out garbage back in#gigogbi#yuval noah harari#gubbish#pkd#philip k dick#phildickian
831 notes
¡
View notes
Text
The Data Scientist Handbook 2024
HT @dataelixir
#data science#data scientist#data scientists#machine learning#analytics#data analytics#artificial intelligence
16 notes
¡
View notes
Text
Physics Informed Neural Networks
Physics-informed neural networks, a deep learning method that bridges the gap between machine learning and scientific computing.
PINN has superior approximation and generalization capabilities, which made it gain popularity in solving high-dimensional partial differential equations (PDEs), and has been used in various applications such as weather modeling, healthcare, and manufacturing.
#machine learning#deep learning#artificial intelligence#physics#data visualization#data science#coding#programming
365 notes
¡
View notes
Text
Cornell quantum researchers have detected an elusive phase of matter, called the Bragg glass phase, using large volumes of X-ray data and a new machine learning data analysis tool. The discovery settles a long-standing question of whether this almostâbut not quiteâordered state of Bragg glass can exist in real materials. The paper, "Bragg glass signatures in PdxErTe3 with X-ray diffraction Temperature Clustering (X-TEC)," is published in Nature Physics. The lead author is Krishnanand Madhukar Mallayya, a postdoctoral researcher in the Department of Physics in the College of Arts and Sciences (A&S). Eun-Ah Kim, professor of physics (A&S), is the corresponding author. The research was conducted in collaboration with scientists at Argonne National Laboratory and at Stanford University.
Continue Reading.
43 notes
¡
View notes
Text
it's monday. why tf am i sitting here having to professionally pop off at someone???
#vent cw#;; no bc being the only woman on my team besides my director is insane sometimes#;; this guy from another part of the business came to me for a cv review bc he's interested in being a data scientist and we get to the#;; feedback session and he's been getting feedback from like two other teammates of mine and i AGREED with their feedback#;; and i offered more and he had so much pushback? AND THEN HE SAID MY CRITIQUE SEEMED LIKE A COP OUT???#;; bc i said the structure needed a bit of tweaking and i offered him a solution#;; then he tried to insinuate that i said data science and machine learning are different? which i never said that???#;; my guuuuy đ anyways i stood up for myself bc you're not about to have me out here looking like a fool? when YOU'RE the one who asked for#;; MY HELP??#;; it's crazy bc i typically don't have to defend my expertise because my team respects me and the people on my side of the business do too#;; i'm going to talk to my boss about it lowkey bc if this guy is already getting help from two other people?? it's a resource drain and im#;; out of polite fuck offs. i need his support on this bc it's actually making me uncomfortable that this dude keeps cropping up#;; asking me for help and also *expecting it*. like nah.#âââ queen of the summer isles ( LUXX SPEAKING )#;; tbd.
11 notes
¡
View notes
Text
Locally Linear Embedding (LLE) approaches
#gradschool#light academia#data visualization#science#math#mathblr#studybrl#machine learning#ai#python#topology
46 notes
¡
View notes
Text
Search Engines:
Search engines are independent computer systems that read or crawl webpages, documents, information sources, and links of all types accessible on the global network of computers on the planet Earth, the internet. Search engines at their most basic level read every word in every document they know of, and record which documents each word is in so that by searching for a words or set of words you can locate the addresses that relate to documents containing those words. More advanced search engines used more advanced algorithms to sort pages or documents returned as search results in order of likely applicability to the terms searched for, in order. More advanced search engines develop into large language models, or machine learning or artificial intelligence. Machine learning or artificial intelligence or large language models (LLMs) can be run in a virtual machine or shell on a computer and allowed to access all or part of accessible data, as needs dictate.
#llm#large language model#search engine#search engines#Google#bing#yahoo#yandex#baidu#dogpile#metacrawler#webcrawler#search engines imbeded in individual pages or operating systems or documents to search those individual things individually#computer science#library science#data science#machine learning#google.com#bing.com#yahoo.com#yandex.com#baidu.com#...#observe the buildings and computers within at the dalles Google data center to passively observe google and its indexed copy of the internet#the dalles oregon next to the river#google has many data centers worldwide so does Microsoft and many others
9 notes
¡
View notes
Text

Tonight I am hunting down venomous and nonvenomous snake pictures that are under the creative commons of specific breeds in order to create one of the most advanced, in depth datasets of different venomous and nonvenomous snakes as well as a test set that will include snakes from both sides of all species. I love snakes a lot and really, all reptiles. It is definitely tedious work, as I have to make sure each picture is cleared before I can use it (ethically), but I am making a lot of progress! I have species such as the King Cobra, Inland Taipan, and Eyelash Pit Viper among just a few! Wikimedia Commons has been a huge help!
I'm super excited.
Hope your nights are going good. I am still not feeling good but jamming + virtual snake hunting is keeping me busy!
#programming#data science#data scientist#data analysis#neural networks#image processing#artificial intelligence#machine learning#snakes#snake#reptiles#reptile#herpetology#animals#biology#science#programming project#dataset#kaggle#coding
41 notes
¡
View notes
Text
#Mern Stack Web Development#React JS Web Development#Advanced Software Development#Data Science and Machine Learning
1 note
¡
View note
Text
Truth speaking on the corporate obsession with AI
Hilarious. Something tells me this person's on the hellsite(affectionate)
#ai#corporate bs#late stage capitalism#funny#truth#artificial intelligence#ml#machine learning#data science#data scientist#programming#scientific programming
7 notes
¡
View notes
Text
HT @dataelixir
#data science#data scientist#data scientists#machine learning#analytics#programming#data analytics#artificial intelligence#deep learning#llm
11 notes
¡
View notes
Text
How ChatGPT Is Trained (Source: OpenAI)

#chatgpt#openai#coding#programming#programmer#developer#artificial intelligence#machine learning#data science#deep learning#computer science#nlp#ai#algorithms#tech#technology
223 notes
¡
View notes