#self driven
Explore tagged Tumblr posts
Text
𝐨𝐧𝐜𝐞 𝐲𝐨𝐮'𝐫𝐞 𝐬𝐭𝐫𝐢𝐩𝐩𝐞𝐝 𝐜𝐥𝐞𝐚𝐧, 𝐰𝐡𝐚𝐭'𝐬 𝐚𝐭 𝐲𝐨𝐮𝐫 𝐜𝐨𝐫𝐞 ?
resounding truth
you have a goal in mind, and others brush it off right away… why does everyone always tell you to follow your dreams when it's actually their dreams that they want you to follow? your memory is weak, and your conviction is even weaker on days when you're reminded of how alone you really are. it's hard to go on without someone pushing you forward… is this what life is? a cycle of monotony, fueled only by the desire not to trouble anyone too much with your passing? it would be nice to have something, or someone, to spur you on. i hope you find them.
tagged by: @rcsetorn (thank you for the tag!) 💕
tagging: @vinjaryou, @oomerkabaoo, @daffodilzae, @tailoringtay and/or whoever hasn't done it yet and wants to do the thing with a muse or OC of choice! 🕺
#in the meme-time 😎#Lorien ✨🎻#I only have like#two pictures of his face#sorry!#That aside...#I did take this more than once#initially unsatisfied#but it kept bringing me back to this one#and the more I think about it#I suppose it kinda works!#Comes off as ambitious#self driven#vain#(secretly very fragile)#(secretly cares a lot)#(secretly needs love and support to keep him moving forward)#coddled#just a dandy who's rarely taken seriously#he overcompensates to hide the insecurity#he wouldn't be where he is today without the support he's received#Anyway...#sorry#😆#just ramblin'#Trying to translate that into something more Lorien-like!
7 notes
·
View notes
Text
Self-Drive Cars In Jaipur
Immerse yourself in the rich tapestry of culture and history that Jaipur has to offer with our Self-Drive Cars In Jaipur. Whether you're captivated by the architectural wonders of Hawa Mahal, mesmerised by the intricate designs of Jaipur's palaces, or tempted by the vibrant hues of its bustling markets, our fleet of vehicles provides the perfect companion for your exploration. With convenient pickup locations and transparent pricing, your journey through Jaipur is made effortless. Bid farewell to rigid itineraries and embrace the spontaneity of self-drive exploration. Book your self-drive car today and unlock the door to an authentic and personalised adventure in the Pink City.

#self-drive cars in jaipur#car rental service in rajasthan#drive rental car#car in rental near me#self driven
0 notes
Text
ARCANE LEAGUE OF LEGENDS: 2x03 - “Finally Got The Name Right”
#arcane#caitvi#arcaneedit#arcane season 2 spoilers#arcane league of legends#league of legends arcane#vi#vi arcane#arcane vi#caitlyn kiramman#heenot#caitlyn#caitlyn arcane#type: gif#media: arcane#league of legends#s2 ep3#i needed to get this scene outta my system..... oh hardened cait.... changed and driven by her grief and anger over jinx#then we see vi who stubbornly clings onto the sweet cupcait she once knew... just like she was holding onto powder#but the heartbreaking part is that she's changed and vi STILL tries to give everything to caitlyn because shes so self sacrificing#and hoping that the caitlyn she once knew is still there but im so so sorry vi but cupcait is gone look at cait wanting to pull the trigger#just look at the pain behind vi's eyes she cant believe cait would be like this god im in pain#shes trying so hard to reel the cait she knew back in GAHHHHHH#also i hated coloring this scene but whats new with dark scenes from arcane lol
1K notes
·
View notes
Text
how to cultivate growth⋆.ೃ࿔*:・🍡
GET UNCOMFORTABLE ;
u cannot grow where ur already comfortable so in order to grow u must get out of ur comfort zone, even if that means getting uncomfortable. like growing a muscle, you must put stress on it first for it to grow. in order to grow u must take action towards ur goals despite any fears that u may have.
MAKE NEW THOUGHTS ;
to embrace the next version of urself and to grow u should make new thoughts that serve you. as you know ur thoughts create ur reality so why keep around thoughts that dont serve you or even worse, self sabotaging thoughts? start saying ur affirmations and applying the law as a way to grow ur confidence in urself and creating ✨
HOT TIP ; start listening to affirmations tapes, i rly recommend high frequency guru on youtube for aff tapes but u can always make ur own too…💬🎀
FROM 0 -> 10 ;
i talked about this in one of my previous posts but i wanna talk about breaking down goals again because making one broad goal, at least in my opinion, can be daunting and ultimately ineffective. breaking it down is where its at.
break down goals into sub goals with tasks within them
make certain tasks a priority
at least 1% closer to ur goal every single day
dont focus on going from 0 -> 100. instead break things up into steps so that u dont intimidate yourself and burn urself out quickly. kind of like a rubber band. if u stretch the rubber band as far as it'll go it'll snap back into place, which leads me to my next point.
THE SNAP BACK EFFECT ;
the snap back effect can be summed up by the example that i used above. the snap back effect was coined by dr. maxwell maltz, author of “psycho-cybernetics,” to describe the rampant self-sabotage of personal improvement goals.
SO HOW DO WE BEAT THE SNAP BACK EFFECT ;
we beat the snap back effect through consistency and through sticking to ur plan. dont jump from 0 -> 100 because then ur just setting urself up for failure. jump from 0 -> 10 and so on so forth.
remember that life is all about growing and pursuing ur goals so make sure that ur helping urself grow and evolve.
#advice#law of assumption#honeytonedhottie⭐️#it girl#becoming that girl#self concept#self care#self love#that girl#it girl energy#dream girl tips#dream girl#dream life#self improvement#self development#self healing#hyper femininity#hyper feminine#goal setting#goal driven#girly#girl blogging#girl blog#girl power#self improvement blog#self improvement tips#growth#metamorphosis
587 notes
·
View notes
Text
the buddy-buddy act ends now
#ritsu: i am a horrible person and i hate you this is my evil arc#shigeo: *hugs his hurting little brother*#ritsu: *breaks into tears* not fair#GDHEHXGSGDH god God i love ritsu okay#i love the kageyama brothers they mean everything#and ritsu- loving ritsu has just been SO interesting and enlightening even these past months#I'm squatting in his brain#his tendency to catastrophize#his powerful sense of self-importance that coexists with brutal self criticism#his prickly aloof nature and enormous capacity for empathy that he consciously extends to very few#he's a judgy b-tch but only in his head#people adore him but he's not interested#he's Driven by fear and he's brave to the point of lunacy#the LEVELS of gaslighting he did on himself to convert that fear into adoration#protectiveness#anything#Anything else that makes sense#the way he snapped under the weight So Fast when presented with an out eager to test what kind of wicked creature he is under the layers of#paint and consolation prizes#his high morals that he's itching to see crumbled#his 'I've obtained loss' that speaks to me. his 'I just wanted to see what its like being a fool'#his 'i realised what i really wanted: to learn that devotion towards living a fun life and shedding sweat and tears and blood for it.'#g a h#kageyama ritsu#ritsu kageyama#mp100#mob psycho 100#mob psycho 100 fanart#kageyama brothers#this piece fought me every step of the way Jeeesussss but i love it. it was very experimental in the direction i want to keep exploring
298 notes
·
View notes
Text
needed to get doodle this out of my system
#not the biggest fan of their romance but holy mother of god their parallels make me insane#something something bred to be a savior who ultimately is the one being saved over and over again#ascending to godhood to save friends and family being so sure in your sense of self and secure in your love for them#whereas he is so lost and trying to find who he is and his purpose and what he was made for#vs her she knows exactly what she wants and is so incredibly driven by her love for others#god HELLOOOOOOOOO… LIKE THATS CRAZY..#fanart#art#doodle#pantheon amc#pantheon amc spoilers
424 notes
·
View notes
Text
Baldur's Gate 3 (2023) dev. Larian Studios
#baldur's gate 3#bg3#halsin bg3#zevlor bg3#bg3edit#gamingedit#dailyvideogames#.gif#this is such a self indulgent post but i'm just thinking about their dynamic lately <3#obviously zevlor is driven by the desire to protect his people#and kagha is horrible and can't be reasoned with - so he needs someone with sense (halsin)#but! i think he has a deep respect for him too#wish zevlor was a companion bc i love him but also bc i want to see him and halsin bestieism in action#q
627 notes
·
View notes
Text
Dammon + green & gold camp clothes
#bg3#baldur's gate 3#dammon#my bg3 edits#leopardmuffinxo.edit#not gonna tag and annoy y'all lol#these were purely driven by deep self indulgence#the fact that i can't kiss him is a CRIME
447 notes
·
View notes
Text
ooooo y’all wanna read my movie Vanessa centric fanfic so bad. ooooo you think it’s so interesting to combine a potential FNAF 2 movie plot with Sister Location ooooooo (this fic is genuinely my pride and joy and I never talk about it enough)

Excerpts for anyone interested under the cut!!! (out of context but yknow)

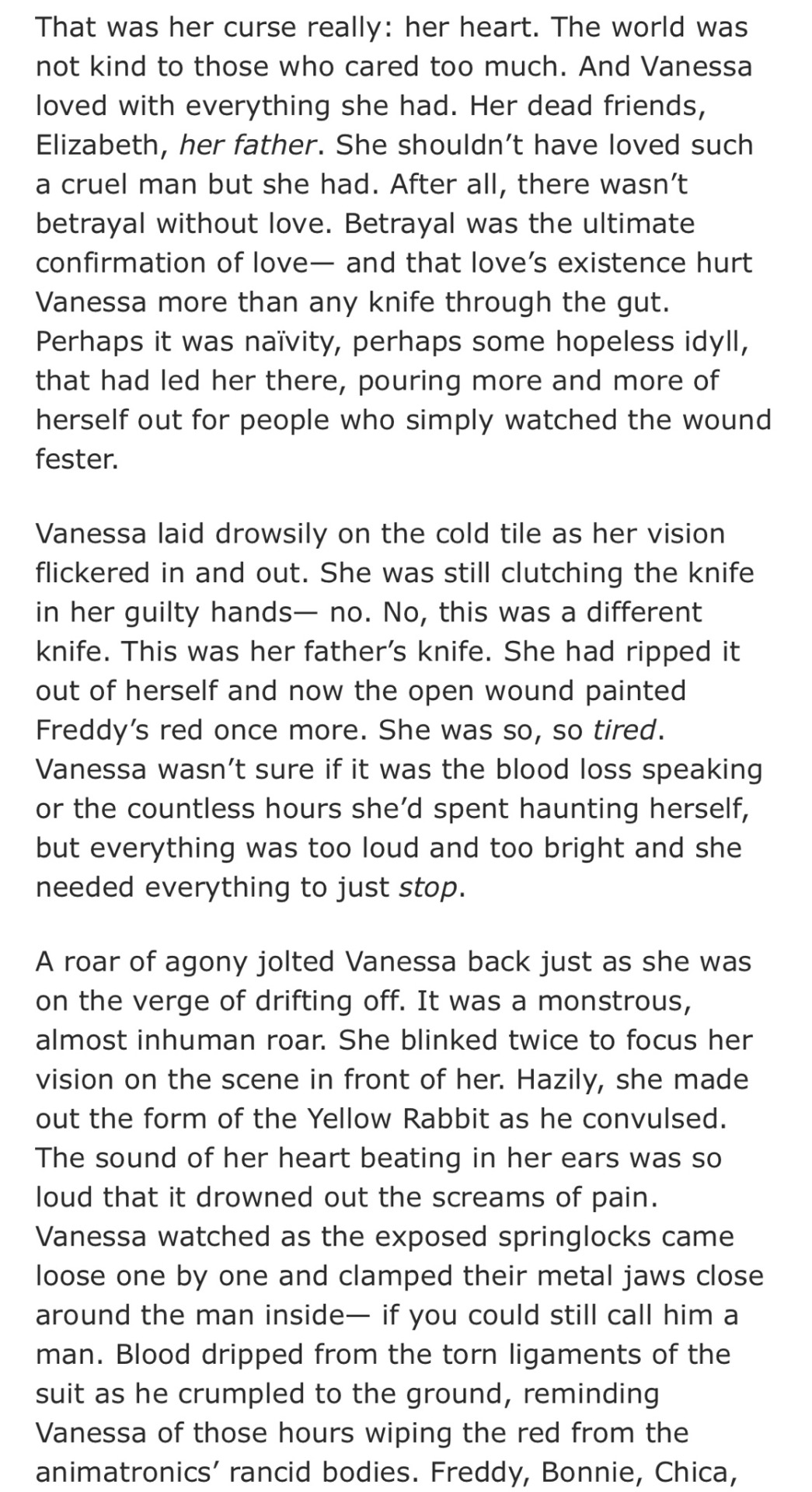
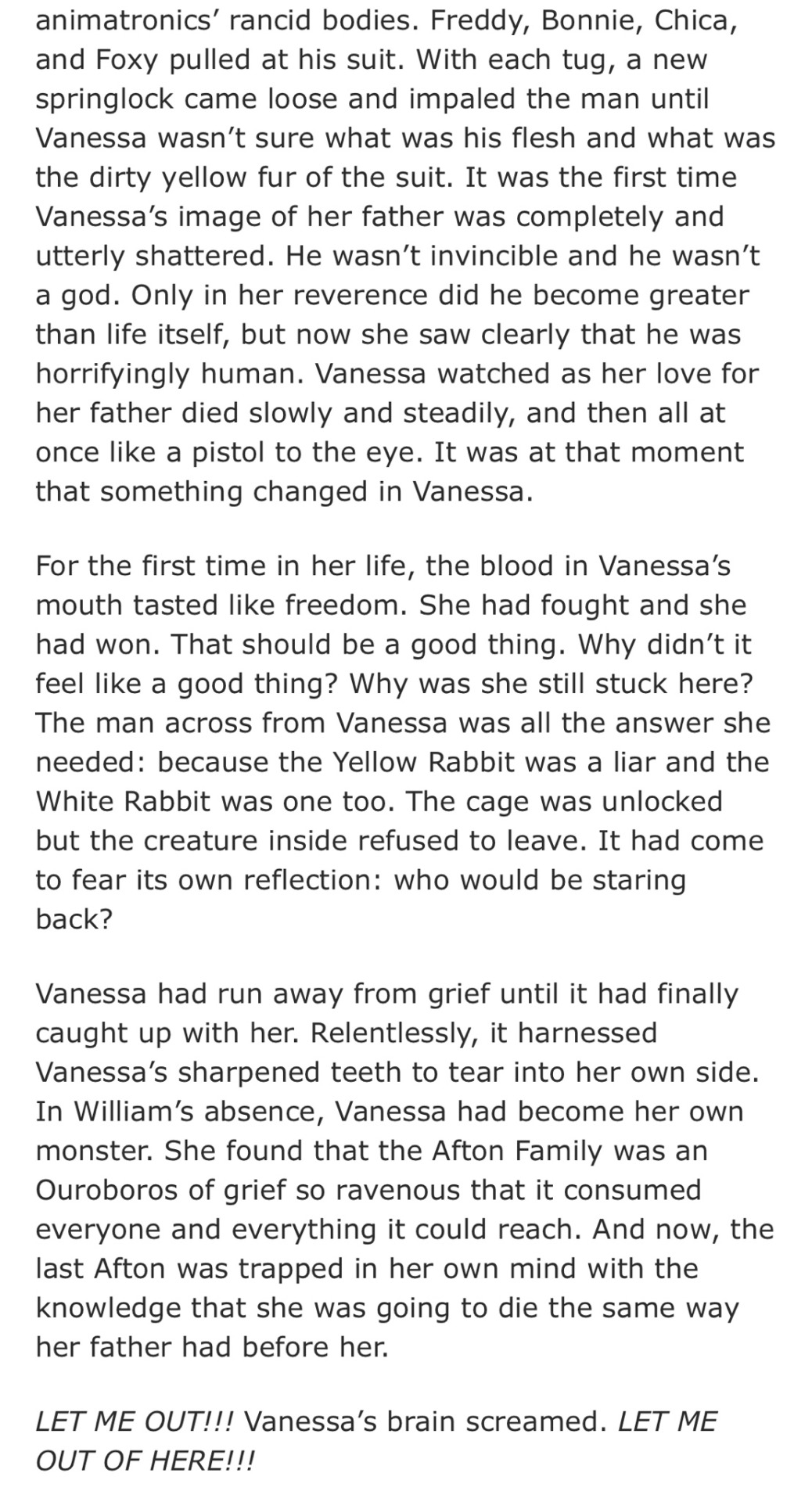
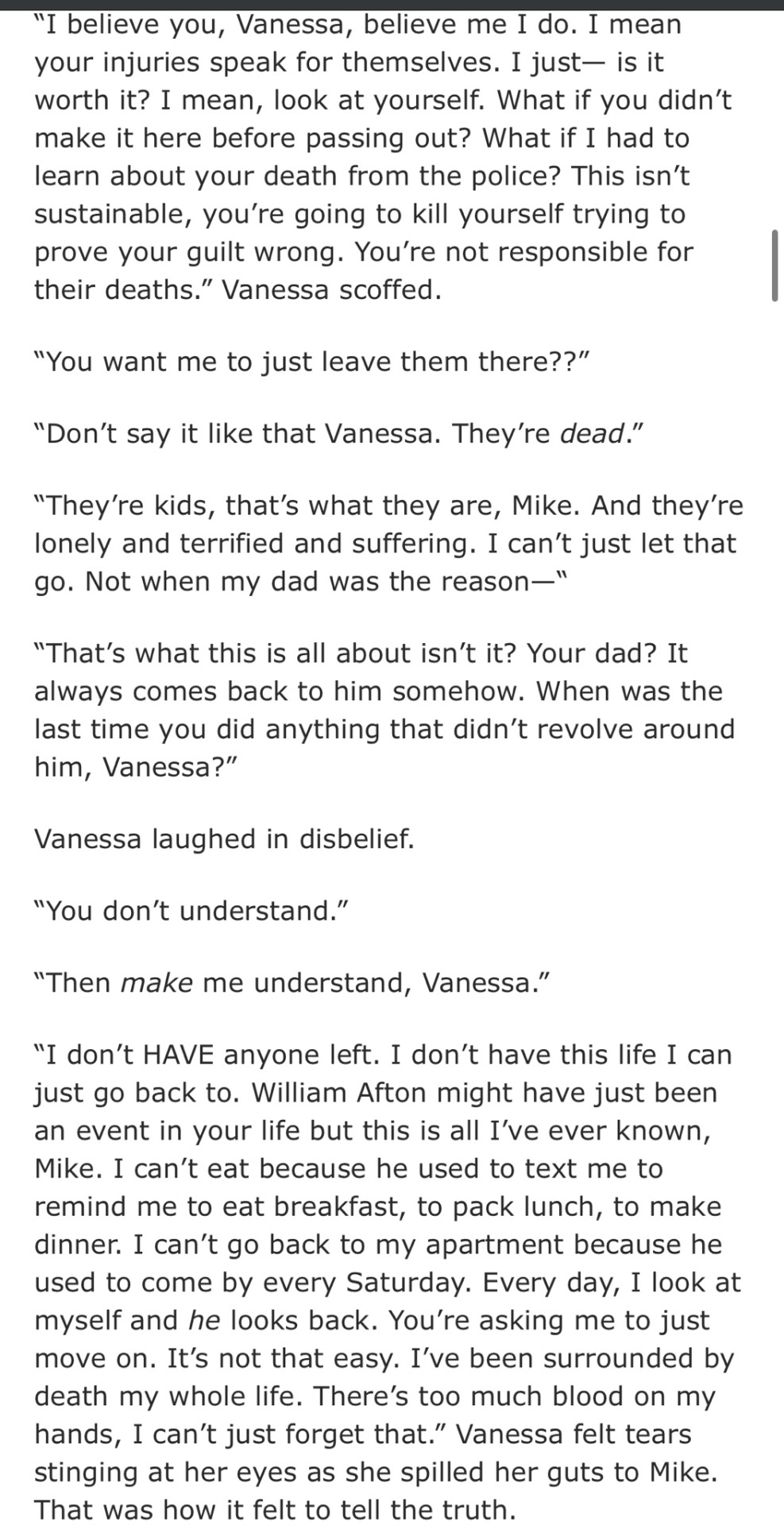
#it’s a character study first and foremost and a character driven plot secondarily#43k words of self indulgence and brainrot#vanessa fnaf#vanessa afton#vanessa shelly#vanessa a#elizabeth afton#william afton#fnaf fanfic#fnaf movie#fnaf 2 movie#fnaf movie 2#five nights at freddy's#five nights at freddy’s movie
61 notes
·
View notes
Text
The surprising truth about data-driven dictatorships
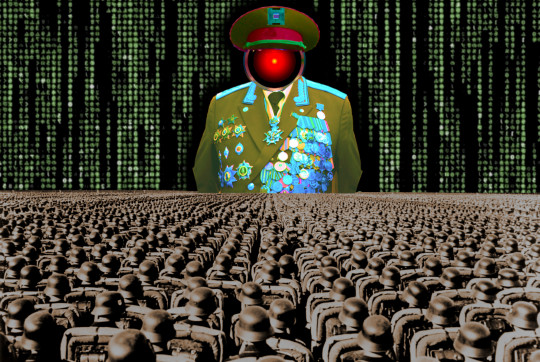
Here’s the “dictator’s dilemma”: they want to block their country’s frustrated elites from mobilizing against them, so they censor public communications; but they also want to know what their people truly believe, so they can head off simmering resentments before they boil over into regime-toppling revolutions.
These two strategies are in tension: the more you censor, the less you know about the true feelings of your citizens and the easier it will be to miss serious problems until they spill over into the streets (think: the fall of the Berlin Wall or Tunisia before the Arab Spring). Dictators try to square this circle with things like private opinion polling or petition systems, but these capture a small slice of the potentially destabiziling moods circulating in the body politic.
Enter AI: back in 2018, Yuval Harari proposed that AI would supercharge dictatorships by mining and summarizing the public mood — as captured on social media — allowing dictators to tack into serious discontent and diffuse it before it erupted into unequenchable wildfire:
https://www.theatlantic.com/magazine/archive/2018/10/yuval-noah-harari-technology-tyranny/568330/
Harari wrote that “the desire to concentrate all information and power in one place may become [dictators] decisive advantage in the 21st century.” But other political scientists sharply disagreed. Last year, Henry Farrell, Jeremy Wallace and Abraham Newman published a thoroughgoing rebuttal to Harari in Foreign Affairs:
https://www.foreignaffairs.com/world/spirals-delusion-artificial-intelligence-decision-making
They argued that — like everyone who gets excited about AI, only to have their hopes dashed — dictators seeking to use AI to understand the public mood would run into serious training data bias problems. After all, people living under dictatorships know that spouting off about their discontent and desire for change is a risky business, so they will self-censor on social media. That’s true even if a person isn’t afraid of retaliation: if you know that using certain words or phrases in a post will get it autoblocked by a censorbot, what’s the point of trying to use those words?
The phrase “Garbage In, Garbage Out” dates back to 1957. That’s how long we’ve known that a computer that operates on bad data will barf up bad conclusions. But this is a very inconvenient truth for AI weirdos: having given up on manually assembling training data based on careful human judgment with multiple review steps, the AI industry “pivoted” to mass ingestion of scraped data from the whole internet.
But adding more unreliable data to an unreliable dataset doesn’t improve its reliability. GIGO is the iron law of computing, and you can’t repeal it by shoveling more garbage into the top of the training funnel:
https://memex.craphound.com/2018/05/29/garbage-in-garbage-out-machine-learning-has-not-repealed-the-iron-law-of-computer-science/
When it comes to “AI” that’s used for decision support — that is, when an algorithm tells humans what to do and they do it — then you get something worse than Garbage In, Garbage Out — you get Garbage In, Garbage Out, Garbage Back In Again. That’s when the AI spits out something wrong, and then another AI sucks up that wrong conclusion and uses it to generate more conclusions.
To see this in action, consider the deeply flawed predictive policing systems that cities around the world rely on. These systems suck up crime data from the cops, then predict where crime is going to be, and send cops to those “hotspots” to do things like throw Black kids up against a wall and make them turn out their pockets, or pull over drivers and search their cars after pretending to have smelled cannabis.
The problem here is that “crime the police detected” isn’t the same as “crime.” You only find crime where you look for it. For example, there are far more incidents of domestic abuse reported in apartment buildings than in fully detached homes. That’s not because apartment dwellers are more likely to be wife-beaters: it’s because domestic abuse is most often reported by a neighbor who hears it through the walls.
So if your cops practice racially biased policing (I know, this is hard to imagine, but stay with me /s), then the crime they detect will already be a function of bias. If you only ever throw Black kids up against a wall and turn out their pockets, then every knife and dime-bag you find in someone’s pockets will come from some Black kid the cops decided to harass.
That’s life without AI. But now let’s throw in predictive policing: feed your “knives found in pockets” data to an algorithm and ask it to predict where there are more knives in pockets, and it will send you back to that Black neighborhood and tell you do throw even more Black kids up against a wall and search their pockets. The more you do this, the more knives you’ll find, and the more you’ll go back and do it again.
This is what Patrick Ball from the Human Rights Data Analysis Group calls “empiricism washing”: take a biased procedure and feed it to an algorithm, and then you get to go and do more biased procedures, and whenever anyone accuses you of bias, you can insist that you’re just following an empirical conclusion of a neutral algorithm, because “math can’t be racist.”
HRDAG has done excellent work on this, finding a natural experiment that makes the problem of GIGOGBI crystal clear. The National Survey On Drug Use and Health produces the gold standard snapshot of drug use in America. Kristian Lum and William Isaac took Oakland’s drug arrest data from 2010 and asked Predpol, a leading predictive policing product, to predict where Oakland’s 2011 drug use would take place.
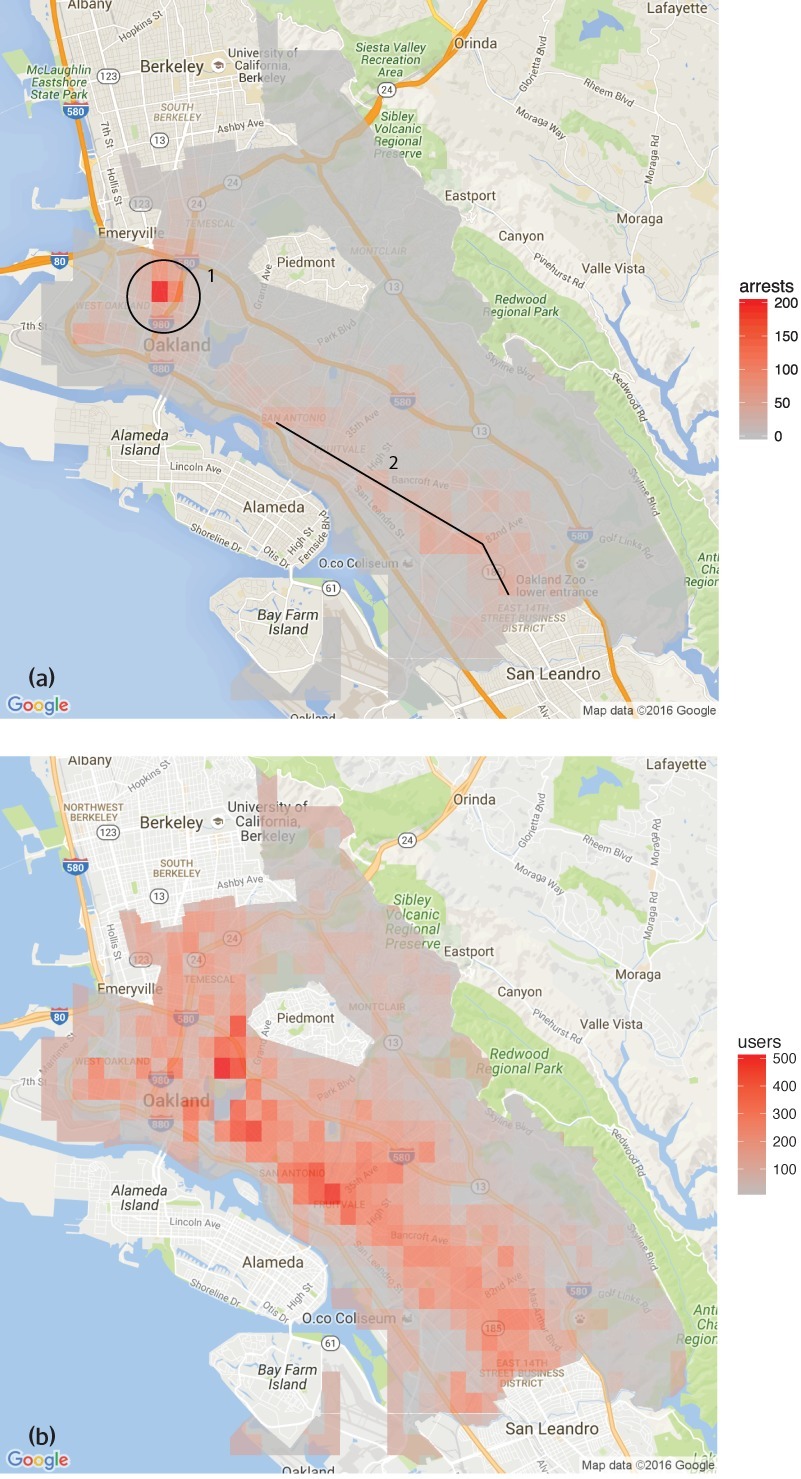
[Image ID: (a) Number of drug arrests made by Oakland police department, 2010. (1) West Oakland, (2) International Boulevard. (b) Estimated number of drug users, based on 2011 National Survey on Drug Use and Health]
Then, they compared those predictions to the outcomes of the 2011 survey, which shows where actual drug use took place. The two maps couldn’t be more different:
https://rss.onlinelibrary.wiley.com/doi/full/10.1111/j.1740-9713.2016.00960.x
Predpol told cops to go and look for drug use in a predominantly Black, working class neighborhood. Meanwhile the NSDUH survey showed the actual drug use took place all over Oakland, with a higher concentration in the Berkeley-neighboring student neighborhood.
What’s even more vivid is what happens when you simulate running Predpol on the new arrest data that would be generated by cops following its recommendations. If the cops went to that Black neighborhood and found more drugs there and told Predpol about it, the recommendation gets stronger and more confident.
In other words, GIGOGBI is a system for concentrating bias. Even trace amounts of bias in the original training data get refined and magnified when they are output though a decision support system that directs humans to go an act on that output. Algorithms are to bias what centrifuges are to radioactive ore: a way to turn minute amounts of bias into pluripotent, indestructible toxic waste.
There’s a great name for an AI that’s trained on an AI’s output, courtesy of Jathan Sadowski: “Habsburg AI.”
And that brings me back to the Dictator’s Dilemma. If your citizens are self-censoring in order to avoid retaliation or algorithmic shadowbanning, then the AI you train on their posts in order to find out what they’re really thinking will steer you in the opposite direction, so you make bad policies that make people angrier and destabilize things more.
Or at least, that was Farrell(et al)’s theory. And for many years, that’s where the debate over AI and dictatorship has stalled: theory vs theory. But now, there’s some empirical data on this, thanks to the “The Digital Dictator’s Dilemma,” a new paper from UCSD PhD candidate Eddie Yang:
https://www.eddieyang.net/research/DDD.pdf
Yang figured out a way to test these dueling hypotheses. He got 10 million Chinese social media posts from the start of the pandemic, before companies like Weibo were required to censor certain pandemic-related posts as politically sensitive. Yang treats these posts as a robust snapshot of public opinion: because there was no censorship of pandemic-related chatter, Chinese users were free to post anything they wanted without having to self-censor for fear of retaliation or deletion.
Next, Yang acquired the censorship model used by a real Chinese social media company to decide which posts should be blocked. Using this, he was able to determine which of the posts in the original set would be censored today in China.
That means that Yang knows that the “real” sentiment in the Chinese social media snapshot is, and what Chinese authorities would believe it to be if Chinese users were self-censoring all the posts that would be flagged by censorware today.
From here, Yang was able to play with the knobs, and determine how “preference-falsification” (when users lie about their feelings) and self-censorship would give a dictatorship a misleading view of public sentiment. What he finds is that the more repressive a regime is — the more people are incentivized to falsify or censor their views — the worse the system gets at uncovering the true public mood.
What’s more, adding additional (bad) data to the system doesn’t fix this “missing data” problem. GIGO remains an iron law of computing in this context, too.
But it gets better (or worse, I guess): Yang models a “crisis” scenario in which users stop self-censoring and start articulating their true views (because they’ve run out of fucks to give). This is the most dangerous moment for a dictator, and depending on the dictatorship handles it, they either get another decade or rule, or they wake up with guillotines on their lawns.
But “crisis” is where AI performs the worst. Trained on the “status quo” data where users are continuously self-censoring and preference-falsifying, AI has no clue how to handle the unvarnished truth. Both its recommendations about what to censor and its summaries of public sentiment are the least accurate when crisis erupts.
But here’s an interesting wrinkle: Yang scraped a bunch of Chinese users’ posts from Twitter — which the Chinese government doesn’t get to censor (yet) or spy on (yet) — and fed them to the model. He hypothesized that when Chinese users post to American social media, they don’t self-censor or preference-falsify, so this data should help the model improve its accuracy.
He was right — the model got significantly better once it ingested data from Twitter than when it was working solely from Weibo posts. And Yang notes that dictatorships all over the world are widely understood to be scraping western/northern social media.
But even though Twitter data improved the model’s accuracy, it was still wildly inaccurate, compared to the same model trained on a full set of un-self-censored, un-falsified data. GIGO is not an option, it’s the law (of computing).
Writing about the study on Crooked Timber, Farrell notes that as the world fills up with “garbage and noise” (he invokes Philip K Dick’s delighted coinage “gubbish”), “approximately correct knowledge becomes the scarce and valuable resource.”
https://crookedtimber.org/2023/07/25/51610/
This “probably approximately correct knowledge” comes from humans, not LLMs or AI, and so “the social applications of machine learning in non-authoritarian societies are just as parasitic on these forms of human knowledge production as authoritarian governments.”
The Clarion Science Fiction and Fantasy Writers’ Workshop summer fundraiser is almost over! I am an alum, instructor and volunteer board member for this nonprofit workshop whose alums include Octavia Butler, Kim Stanley Robinson, Bruce Sterling, Nalo Hopkinson, Kameron Hurley, Nnedi Okorafor, Lucius Shepard, and Ted Chiang! Your donations will help us subsidize tuition for students, making Clarion — and sf/f — more accessible for all kinds of writers.
Libro.fm is the indie-bookstore-friendly, DRM-free audiobook alternative to Audible, the Amazon-owned monopolist that locks every book you buy to Amazon forever. When you buy a book on Libro, they share some of the purchase price with a local indie bookstore of your choosing (Libro is the best partner I have in selling my own DRM-free audiobooks!). As of today, Libro is even better, because it’s available in five new territories and currencies: Canada, the UK, the EU, Australia and New Zealand!
[Image ID: An altered image of the Nuremberg rally, with ranked lines of soldiers facing a towering figure in a many-ribboned soldier's coat. He wears a high-peaked cap with a microchip in place of insignia. His head has been replaced with the menacing red eye of HAL9000 from Stanley Kubrick's '2001: A Space Odyssey.' The sky behind him is filled with a 'code waterfall' from 'The Matrix.']
Image: Cryteria (modified) https://commons.wikimedia.org/wiki/File:HAL9000.svg
CC BY 3.0 https://creativecommons.org/licenses/by/3.0/deed.en
—
Raimond Spekking (modified) https://commons.wikimedia.org/wiki/File:Acer_Extensa_5220_-_Columbia_MB_06236-1N_-_Intel_Celeron_M_530_-_SLA2G_-_in_Socket_479-5029.jpg
CC BY-SA 4.0 https://creativecommons.org/licenses/by-sa/4.0/deed.en
—
Russian Airborne Troops (modified) https://commons.wikimedia.org/wiki/File:Vladislav_Achalov_at_the_Airborne_Troops_Day_in_Moscow_%E2%80%93_August_2,_2008.jpg
“Soldiers of Russia” Cultural Center (modified) https://commons.wikimedia.org/wiki/File:Col._Leonid_Khabarov_in_an_everyday_service_uniform.JPG
CC BY-SA 3.0 https://creativecommons.org/licenses/by-sa/3.0/deed.en
#pluralistic#habsburg ai#self censorship#henry farrell#digital dictatorships#machine learning#dictator's dilemma#eddie yang#preference falsification#political science#training bias#scholarship#spirals of delusion#algorithmic bias#ml#Fully automated data driven authoritarianism#authoritarianism#gigo#garbage in garbage out garbage back in#gigogbi#yuval noah harari#gubbish#pkd#philip k dick#phildickian
831 notes
·
View notes
Text
Reading journal 3 again is a reminder that... Well Ford is kinda an asshole towards Fiddleford. It's not just Stan. Like he's so focused on himself and his project and his ambition that anyone/thing else blurs out (him disregarding Fiddleford's nervousness around the gremloblin because he wants to sketch it, and when it goes sideways, him talking about the safety of the project parts BEFORE how Fiddleford was doing in his journal, multiple times. Priorities much?) and he's demeaning towards Fiddleford's own project (which ends up being wayyyy more important then Ford's discoveries)... Like damn Fiddleford, u left ur wife and young son for this guy?
#gravity falls#ford pines#stanford pines#fiddleford mcgucket#fiddleford hadron mcgucket#ford squared#like... i get where reading the journal so its more of Ford unfiltered thoughts but it REALLY shows his priorities#and yeah I'm nor saying there wasnt good things in their relationship but Ford still is very much by this point extremely self-absorbed#and it SHOWS and its not... it bot very pretty. like we joke about Bill fumbling Ford and how great Ford is but like ..#compared to McGucket who earnestly cared Ford... was extremely self-absorbed and selfish. and like McGucket definitely#began to help Ford step out of his self-absorption and solely being driven by his ambition but in the end it ended terrribly#literally McGucket feeling so unsafe in the relationship and where he was that he made the memory gun and then USED IT UNCONSENTED on Ford#because he couldnt destroy it and Ford demanded him too... like yikes#anyways theyre all a fucking delight to chew on#this is not supposed to come off as ford squares disliking this is me going those two arent as wholesome as u might think when they were#younger. or maybe since im not a lurker in that part of the fandom i just have a poor conception of what its like compared to like. billford#like you can REALLY see what Bill likes in Ford#hugin rambles#hugin rambles gf
81 notes
·
View notes
Text
y'all have no clue how wild it is to live in my timeline, because 12 years ago i was a huge fan of these cute lil' zelda strips and at the time i found out through the grapevine that the creator apparently stopped making these comics because they had started drawing NSFW content-
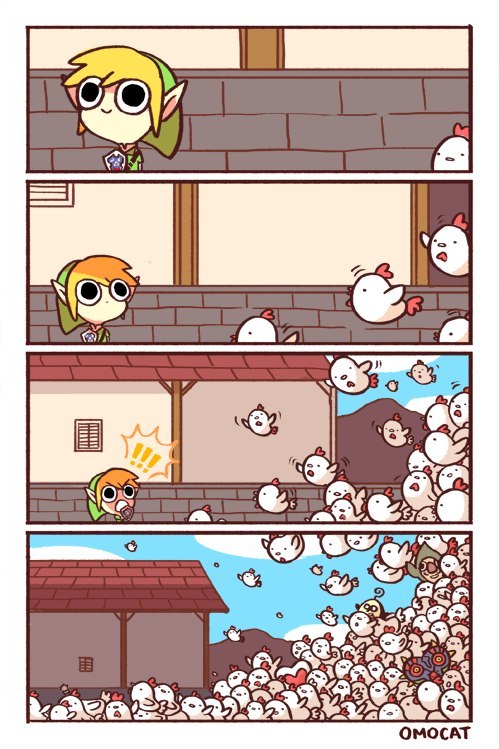
and at the time i was like "oh ok that's wild but respect"
and now in the present the artist who's making the OMORI manga adaption is under fire for being a shota fetishist and it's putting the spotlight back on the OMORI creator themselves for ALSO being a shota fetishist and oh my god the omocat who made those zelda comics over a decade ago is the same omocat who went on to make OMORI holy shit-

#small fucking world jfc#and yeah maybe i'm the last person to cross the finish line here but listen#i hadn't thought about those zelda comics in AGES#not until i came across one of them in my FB memories#because i've been on FB so long now that some of my memories are like 15 years old#and as soon as i saw the artist credit i was like HOLD the fucking phone there's no WAY#anyways there's some wild shit happening in the omori fandom ig#i've only completed the whole game once and honestly i loved it but i haven't really participated in the greater fandom#and i can very much see what people are getting at lmao#especially with the manga jfc#why would you make a manga adaption of a game that's largely driven by a self-insert-style main character#never mind the fact that it completely removes the soundtrack which is like half of what made omori so memorable ffs#even without the shota shit a manga version of OMORI is still. just not something we needed lmao#bad dumb stupid idea
117 notes
·
View notes
Text
Daily Rental Car Service
Experience the freedom to tailor your daily adventures to your preferences with our daily rental car service. Whether you're planning a leisurely drive through scenic routes, need a reliable vehicle for business meetings, or simply want the convenience of having transportation at your fingertips, our diverse fleet caters to all your needs. With options ranging from economy cars to luxury vehicles, we ensure that every journey is comfortable and enjoyable. Plus, with our commitment to cleanliness and maintenance, you can trust that your rental car will be in top condition. Don't let transportation limitations hold you back – book your daily rental car today and embark on a seamless and memorable journey.

#daily rental car service#self-drive cars in jaipur#self driven#car subscription in jaipur#car in rental near me
0 notes
Text
I still feel like the craziest part of the book of bill is bill making ford stand on his roof in the freezing cold and ford realizing bill couldve made him jump but didn't. like. what the fuck
#text#billford#Idk why that sticks in my brain so much just. man#and the detail of bill setting up the inside of the house to be all warm and welcoming is just#man#he also threatens to walk into the lake when he tries to call stan like bill this isnt gonna make him want to take you back#just imagine what it'd be like to realize the interdimensional creature obsessed with you can make you end your own life#its so fucked up#no wonder ford got so paranoid i would be too#he was so ego driven at the time that the loss of autonomy and control would be even more terrifying#he thinks he can only rely on himself and yet that sense of self is being distorted and repossessed by another#i have so many thoughts about them i need to write into fanfic
95 notes
·
View notes
Text
honestly it feels ridiculous to say this, but stede giving up being a pirate is a good thing. i don't know how anyone could watch ofmd 2x07 "man on fire" and come away from it thinking that what stede really needed was to continue a life beset by danger for the sake of gaining infamy, and that him giving it up to stay with ed by s2's end was bad, somehow.
we all watched stede sitting at a bar declaring a bunch of strangers singing his praises his "friends" all the while his lover and found family were leaving him. infamy in the pirate world is cold and lonely and dangerous and it does not spare room for love or true acceptance. we learned this with ed and the persona of blackbeard. it is conditional, in the same way acceptance in traditional society is.
stede, briefly, performs well enough to gain that acceptance, but it is unsustainable, and in the end, it is hollow. and it's not too hard to infer that stede comes to this conclusion by the episode's end and into 2x08, in between getting his ass kicked by zheng in a self-destructive spiral. ed leaves, his crew starts leaving, and then everything goes to shit, and the infamy stops fucking mattering. all it's done is bring danger and drive the people he really cares about away.
him giving up piracy is not him giving up his lifelong dream purely for ed's sake, or anything like that. it is equally for himself.
#ofmd#ofmd s2#our flag means death#ofmd meta#marine blogs#sometimes--when you are someone with a very low self image--you desperately crave validation from strangers#and then you get it! only to realize it doesn't actually fill the hole inside you.#and it doesn't feel quite as good as you expected it would.#and imagine if in the search for that validation you'd driven away someone very close to you?#i just think that would prompt a very quick reorganization of priorities idk
209 notes
·
View notes
Text
I finished my data analysis for POV distribution in TRC! Figure captions are in the alt text. Other observations, discussion of the analysis and results, and some supplemental figures are under the cut. This is perhaps the most self-indulgent project I've worked on <3
Other observations: I went into this analysis with the hypothesis that each of the four protagonists would have the most chapters and/or pages in the following pattern: Blue for TRB, Ronan for TDT, Adam for BLLB, and Gansey for TRK. This mostly held true, although Blue had more chapters and pages than Adam in BLLB. This trend was more significant in terms of page distribution compared to chapter distribution. Mean chapter length differences were infrequent and did not follow the expected pattern, although I did see trends towards shorter chapters for antagonists not grouped into "all others."
There is a relative lack of back-to-back chapters for the same POV character, although this does occur in all books (twice for Blue in TRB; once for The Gray Man, twice for Adam, and six times for Ronan in TDT; five times for Blue [two of which are back-to-back-to-back], once for Gansey, twice for Adam [one of which is back-to-back-to-back], and once for Greenmantle in BLLB. I mention all of this to say that in TRK, this occurs once for Ronan, once for Blue, once for Adam, and thrice for Gansey- but in one of these instances, Gansey has SIX chapters in a row. Nine of the chapters from 48 to 58 are from his POV. The Raven King indeed!
Analysis notes:
For each book, I counted how many chapters each POV character had and how many pages each POV character had. Page numbers are based on the UK paperback editions of the series. In the figures, I show raw # of chapters and pages, as well as the proportion of chapters and pages attributed to each POV character over the total number of chapters or pages. I also compared POV characters’ pages/chapter ratio and tested for differences in mean chapter length with a one-way ANOVA with multiple comparisons.
For most measures, in cases where chapters had multiple POV characters in sequence, I divided the chapter by # of characters. For example, chapter 44 in TRB starts with Adam, switches to Whelk, and ends with Blue. This chapter counted as 1/3 chapter for each character. In BLLB, only the prologue contained multiple discrete POV sections (Persephone, Calla, and Maura) and Piper only had her POV featured in the epilogue so I grouped these two chapters as “All Others” and excluded them from statistical tests. For TRK and TRC, I did include the "All Others" category in the analysis. (“All Others” includes chapters/pages with ambiguous POV (Kavinsky’s text in TDT- are we reading it from the sender or the recipient’s POV?), mixed/omniscient POV (the Gray Man’s tarot reading in TDT – we get internal thoughts and feelings from multiple characters), and in TRK, any character aside from Blue, Gansey, Adam, and Ronan.)
In figures that represent raw counts of chapters or pages, the dashed horizontal line represents the expected number of chapters or pages per POV character if the distribution was equal. The p values on these figures represent the results of a Chi-square test to test whether the actual distribution of pages or chapters was different from an equal distribution.
For the pages/chapter ratio data and the Chi-square tests, I did not apply this calculation and counted each discrete POV as a full chapter because I needed counts and sample sizes to be integers for the statistical analysis (Gansey having an n = 8.5 was not making GraphPad prism very happy with me). I had to do a bit of rounding to the nearest number which was not ideal, but I did this as little as possible. (There is probably a workaround for this, at least for the ratio data, but I am not a statistician). To at least approximate what results would look like without the skew towards a lower pages/chapter ratio, I also ran the ANOVA only with chapters containing a single POV character, which is why you’ll see two figures for the pages/chapter metric for each book (except for BLLB).
I am considering p values < 0.05 to be significant; I have included non-significant p values on some figures to provide additional context. If there is no p value on a given figure, you can assume I found no significant differences amongst POV characters’ chapters and/or pages in my analysis.
If you have questions about the analysis please let me know! :)
Supplemental figures: TRK data shown for all unique POV characters (these figures do not have captions in the alt text; the four on the left depict distribution of chapters and pages across the 19 different POV characters in TRK; the vast majority of the chapters and pages are attributed to either Blue, Gansey, Adam, or Ronan. The two figures on the left show mean pages per chapter for all characters, with no clear/statistically significant trends across the data. The demon's chapters are noticeably short.)
#rchl#trc#trc data#the raven cycle#the raven boys#the dream thieves#blue lily lily blue#the raven king#this was sooooooo fun btw do we want setting data next maybe?#im also working on a more language driven project about usage of camaro vs pig#also i have reached self parody by including a supplemental section like that is truly absurdly me
614 notes
·
View notes