#learn data structure and algorithms
Explore tagged Tumblr posts
Text
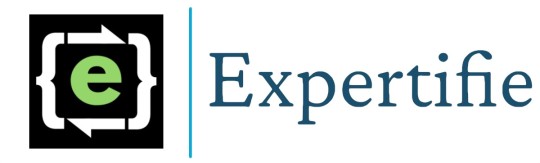
Expertifie partners with companies and individuals to address their unique needs, providing training and coaching that helps working professionals achieve their career goals. They teach their students all the relevant skills needed in software jobs, mentor them to crack recruitment processes and also provide them referrals to the best opportunities in the software industry across the globe. Their vision is to empower career growth and advancement for every member of the global workforce as their trusted lifelong learning partners.
The main features of Expertifie courses are:
1. Instructor-led live interactive training.
2. Advance level of coding and excellent mentoring from our industry experts.
3. Receive guidance that makes you skill ready for interviews and on the job scenarios.
4. Career Support through Mock Interviews and Job Referral.
5. Coding Sessions and Assignments for each topic.
6. Up to 4 Mock Interviews.
7. Sessions by Industry Experts from MAANG.
The courses provided are:
Data Structures & Algorithms
System Design (HLD + LLD)
Full Stack Development
#learn full stack development#learn linux kernel#learn system design#learn data structure and algorithms
2 notes
·
View notes
Text
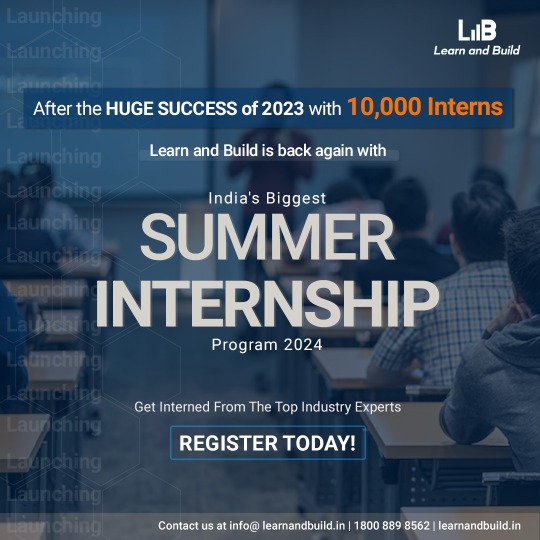
Summer Internship Program 2024
For More Details Visit Our Website - internship.learnandbuild.in
#machine learning#programming#python#linux#data science#data scientist#frontend web development#backend web development#salesforce admin#salesforce development#cloud AI with AWS#Internet of things & AI#Cyber security#Mobile App Development using flutter#data structures & algorithms#java core#python programming#summer internship program#summer internship program 2024
2 notes
·
View notes
Text
User-friendly system can help developers build more efficient simulations and AI models
New Post has been published on https://thedigitalinsider.com/user-friendly-system-can-help-developers-build-more-efficient-simulations-and-ai-models/
User-friendly system can help developers build more efficient simulations and AI models
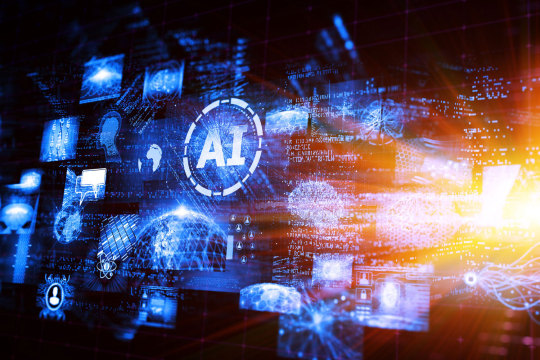
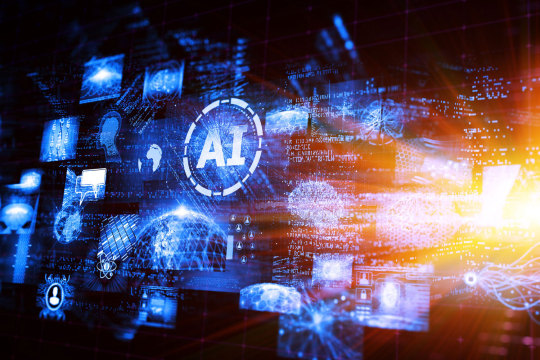
The neural network artificial intelligence models used in applications like medical image processing and speech recognition perform operations on hugely complex data structures that require an enormous amount of computation to process. This is one reason deep-learning models consume so much energy.
To improve the efficiency of AI models, MIT researchers created an automated system that enables developers of deep learning algorithms to simultaneously take advantage of two types of data redundancy. This reduces the amount of computation, bandwidth, and memory storage needed for machine learning operations.
Existing techniques for optimizing algorithms can be cumbersome and typically only allow developers to capitalize on either sparsity or symmetry — two different types of redundancy that exist in deep learning data structures.
By enabling a developer to build an algorithm from scratch that takes advantage of both redundancies at once, the MIT researchers’ approach boosted the speed of computations by nearly 30 times in some experiments.
Because the system utilizes a user-friendly programming language, it could optimize machine-learning algorithms for a wide range of applications. The system could also help scientists who are not experts in deep learning but want to improve the efficiency of AI algorithms they use to process data. In addition, the system could have applications in scientific computing.
“For a long time, capturing these data redundancies has required a lot of implementation effort. Instead, a scientist can tell our system what they would like to compute in a more abstract way, without telling the system exactly how to compute it,” says Willow Ahrens, an MIT postdoc and co-author of a paper on the system, which will be presented at the International Symposium on Code Generation and Optimization.
She is joined on the paper by lead author Radha Patel ’23, SM ’24 and senior author Saman Amarasinghe, a professor in the Department of Electrical Engineering and Computer Science (EECS) and a principal researcher in the Computer Science and Artificial Intelligence Laboratory (CSAIL).
Cutting out computation
In machine learning, data are often represented and manipulated as multidimensional arrays known as tensors. A tensor is like a matrix, which is a rectangular array of values arranged on two axes, rows and columns. But unlike a two-dimensional matrix, a tensor can have many dimensions, or axes, making tensors more difficult to manipulate.
Deep-learning models perform operations on tensors using repeated matrix multiplication and addition — this process is how neural networks learn complex patterns in data. The sheer volume of calculations that must be performed on these multidimensional data structures requires an enormous amount of computation and energy.
But because of the way data in tensors are arranged, engineers can often boost the speed of a neural network by cutting out redundant computations.
For instance, if a tensor represents user review data from an e-commerce site, since not every user reviewed every product, most values in that tensor are likely zero. This type of data redundancy is called sparsity. A model can save time and computation by only storing and operating on non-zero values.
In addition, sometimes a tensor is symmetric, which means the top half and bottom half of the data structure are equal. In this case, the model only needs to operate on one half, reducing the amount of computation. This type of data redundancy is called symmetry.
“But when you try to capture both of these optimizations, the situation becomes quite complex,” Ahrens says.
To simplify the process, she and her collaborators built a new compiler, which is a computer program that translates complex code into a simpler language that can be processed by a machine. Their compiler, called SySTeC, can optimize computations by automatically taking advantage of both sparsity and symmetry in tensors.
They began the process of building SySTeC by identifying three key optimizations they can perform using symmetry.
First, if the algorithm’s output tensor is symmetric, then it only needs to compute one half of it. Second, if the input tensor is symmetric, then algorithm only needs to read one half of it. Finally, if intermediate results of tensor operations are symmetric, the algorithm can skip redundant computations.
Simultaneous optimizations
To use SySTeC, a developer inputs their program and the system automatically optimizes their code for all three types of symmetry. Then the second phase of SySTeC performs additional transformations to only store non-zero data values, optimizing the program for sparsity.
In the end, SySTeC generates ready-to-use code.
“In this way, we get the benefits of both optimizations. And the interesting thing about symmetry is, as your tensor has more dimensions, you can get even more savings on computation,” Ahrens says.
The researchers demonstrated speedups of nearly a factor of 30 with code generated automatically by SySTeC.
Because the system is automated, it could be especially useful in situations where a scientist wants to process data using an algorithm they are writing from scratch.
In the future, the researchers want to integrate SySTeC into existing sparse tensor compiler systems to create a seamless interface for users. In addition, they would like to use it to optimize code for more complicated programs.
This work is funded, in part, by Intel, the National Science Foundation, the Defense Advanced Research Projects Agency, and the Department of Energy.
#ai#AI models#algorithm#Algorithms#applications#approach#Arrays#artificial#Artificial Intelligence#author#Building#Capture#code#code generation#columns#Commerce#computation#computer#Computer Science#Computer Science and Artificial Intelligence Laboratory (CSAIL)#Computer science and technology#computing#cutting#data#Data Structures#Deep Learning#defense#Defense Advanced Research Projects Agency (DARPA)#Department of Energy (DoE)#Developer
0 notes
Text
The Ultimate Guide to Learn Data Structures and Algorithms from Scratch
Data Structures and Algorithms (DSA) form the cornerstone of computer science and programming. Whether you are preparing for competitive programming, acing technical interviews, or simply aiming to become a better developer, understanding DSA is crucial. Here is the ultimate guide to help you Learn Data Structures and Algorithms from scratch.
Why Learn Data Structures and Algorithms?
Efficiency: Mastering DSA allows you to write code that runs faster and consumes less memory.
Problem-Solving Skills: Understanding DSA enhances your ability to break down and solve complex problems logically.
Career Opportunities: Companies like Google, Amazon, and Facebook heavily emphasize DSA in their hiring processes.
Foundation for Advanced Topics: Concepts like machine learning, databases, and operating systems rely on DSA principles.
Step-by-Step Plan to Learn Data Structures and Algorithms
1. Start with the Basics
Begin by learning a programming language like Python, Java, or C++ that you’ll use to implement DSA concepts. Get comfortable with loops, conditionals, arrays, and recursion as these are fundamental to understanding algorithms.
2. Understand Core Data Structures
Learn these essential data structures:
Arrays: Linear storage of elements.
Linked Lists: Dynamic storage with nodes pointing to the next.
Stacks and Queues: Linear structures for LIFO (Last In, First Out) and FIFO (First In, First Out) operations.
Hash Tables: Efficient storage for key-value pairs.
Trees: Hierarchical data structures like binary trees and binary search trees.
Graphs: Structures to represent connections, such as networks.
3. Master Common Algorithms
Once you’ve learned the data structures, focus on algorithms:
Sorting Algorithms: Bubble Sort, Merge Sort, Quick Sort.
Searching Algorithms: Binary Search, Linear Search.
Graph Algorithms: Breadth-First Search (BFS), Depth-First Search (DFS).
Dynamic Programming: Techniques for solving problems with overlapping subproblems.
4. Practice Regularly
Use platforms like LeetCode, HackerRank, and GeeksforGeeks to practice problems. Start with beginner-friendly questions and gradually move to more challenging ones. Regular practice is the key to mastering how to Learn Data Structures and Algorithms.
5. Explore Advanced Topics
Once you’re confident with the basics, explore advanced topics like:
Tries (prefix trees).
Segment Trees and Fenwick Trees.
Advanced graph algorithms like Dijkstra’s and Floyd-Warshall algorithms.
Tips for Success
Set Goals: Break your learning into milestones.
Use Visual Aids: Visualize data structures and algorithms for better understanding.
Build Projects: Implement real-world projects to solidify your knowledge.
Join Communities: Engage with forums and groups to learn collaboratively.
Conclusion
Learning DSA from scratch can be challenging but highly rewarding. By following this guide and committing to consistent practice, you can master the fundamentals and beyond. Start today and take your first step toward becoming a proficient problem-solver and programmer. Embrace the journey to Learn Data Structures and Algorithms and unlock endless opportunities in the tech world.
0 notes
Text
First courses on IT automation, cybersecurity and data analytics are over.
I think the one in cybersecurity has Python too, so I hope I don't get a Python crash course again! XD
#still no job so i still have free time to do these courses#i guess after those three i will do cs50 just in hopes that i learn more about data structures and algorithms?#anyway every1 tells me i wont get a job anyway until at least september so i have time#and thus i have time for my own projects too
0 notes
Text

Placement Preparation Course for CSE
Dive into essential algorithms, data structures, and coding practices while mastering problem-solving techniques. Our expert-led sessions ensure you're well-equipped for technical interviews. Join our Placement Preparation Course for CSE now to secure your dream job in top-tier companies. Get ahead in the competitive tech world with our proven curriculum and guidance. Your future starts here!
#Data Structures#Data Structures and Algorithms#Data Structures and Algorithms Interview Questions#coding#courses#online courses#online learning
0 notes
Text
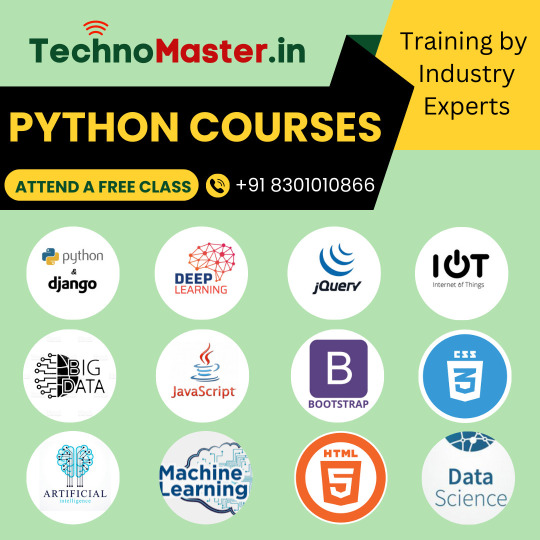
Best R Programming Training Institute in India
TechnoMaster.in is a leading R programming training institute that offers the best R programming training to students looking to get a secured job in an MNC and corporate giant.
For more details on the availability of our Training Program. Click Below:-
Best R Programming Training Course
The online R Programming certification and training course important concepts in R Programming like data visualization, descriptive analytics techniques, data exploration and predictive analytics with R languages. The R Programming training courses is also helps in exploring about the R packages, data structure in R, cluster analysis, graphic representation, reporting, data manipulation, business analytics, flow of control, machine learning algorithms, variables functions, data structures, import and export data in R, forecasting and diverse statistical concepts through practical R industry scenarios and examples. The courses materials of the TechnoMaster.in R Programming training course are based on the latest industry scenarios and hold every essential concepts on R Programming to clear the TechnoMaster.in R Programming certification exam.
We provide short term, crash and long term online IT courses on all IT technologies at convenient schedules and reasonable fees. The delegates who takes online R Programming training course on the TechnoMaster.in will work on real-time assignments and projects that have impact in the real time scenarios, thus enabling you to get an excellent job in your career efficiently. So, what are you waiting for Simply enroll for the best R Programming certification course and get a secured job without investing much.
Key features of R Programming Online Training
40 Hours of Course Duration
Industry Expert Faculties
100% Job Oriented Training
Free Demo Class Available
Certification Guidance
#data visualization#descriptive analytics techniques#data exploration#data exploration and predictive analytics with R languages#R packages#data structure in R#cluster analysis#graphic representation#machine learning algorithms#forecasting and diverse statistical concepts#import and export data in R
0 notes
Text
Website and Mobile App Development with ReactJS
The React.js framework is an open source JavaScript framework and library developed by Facebook. It is used to quickly and efficiently build interactive user interfaces and web applications with far less code.
React develops applications by creating reusable components that can be thought of as individual building blocks. These components are the individual parts of the final interface, which together form the overall user interface of the application.
The primary role of React in an application is to treat the view layer of that application, providing the best and most efficient rendering execution. Instead of treating the entire UI as a single entity, ReactJS encourages developers to decompose these complex UI into individual reusable components that form the building blocks of the overall UI. increase. The ReactJS framework combines the speed and efficiency of JavaScript with a more efficient way of manipulating the DOM to render web pages faster and create highly dynamic and responsive web applications.
React has simplified the development process by providing a more organized and structured way to create dynamic, interactive user interfaces with reusable components.
Websites or Apps developed using ReactJS platform
React has gained stability and popularity due to its ability to build fast, efficient, and scalable web applications. It is currently used in thousands of web applications from established companies to start-ups.
Some notable mentions are:
Facebook
Instagram
Netflix
Reddit
Uber
Airbnb
The New York Times
Khan Academy
Codecademy
SoundCloud
Discord
WhatsApp Web
Due to its adaptability, reusability, and simplicity, ReactJS has emerged as one of the most popular options for creating websites and mobile apps. It enables developers to build complex applications quickly and efficiently while providing a smooth user experience. A lively developer community supports ReactJS, and new libraries, frameworks, and tools are regularly added to its environment. As a result, anyone looking to build contemporary, responsive, and scalable web applications should consider learning and using ReactJS.
ReactJS also provides better speed by utilizing a virtual DOM and streamlining rendering updates. As a result, ReactJS has become the go-to choice for many web developers and businesses, from small startups to large-scale enterprises. Developers can produce dynamic, responsive, and engaging web apps that satisfy the needs of modern users by utilizing React.js.
#Structures#Algorithms#Python#Java#C#C++#JavaScript#Android Development#SQL#Data Science#Machine Learning#PHP#Web Development#System Design#Tutorial#Technical Blogs#Interview Experience#Interview Preparation#Programming#Competitive Programming#SDE Sheet#Job-a-thon#Coding Contests#GATE CSE#HTML#CSS#React#NodeJS#Placement#Aptitude
0 notes
Text
Learn data structures and algorithms using java | Sunbeam
The data structure, data structure training near me, data structures and algorithms course in Pune, best data structures and algorithms course in java, best DSA course in c++. Learn data structures and algorithms using java online. In this course, you will understand common data structures like an array, linked list, stack & queue, and their applications and how to implement data structures and algorithms using Java. Data Structures and Algorithms are one of the most important skills that every computer science student must have. It is often seen that people with good knowledge of these technologies are better programmers than others and thus, crack the interviews of almost every tech giant. Now, you must be thinking to opt for a quality DSA Course to build & enhance your data structures and algorithms skills, right?? If yes, then you’ve ended up at the right place. This DSA Course will help you to learn and master all the DSA concepts from the basics to the advanced level! Contact Number:8447901102 Address: Hinjawadi Phase 2, Hinjawadi Pune 411053
0 notes
Text
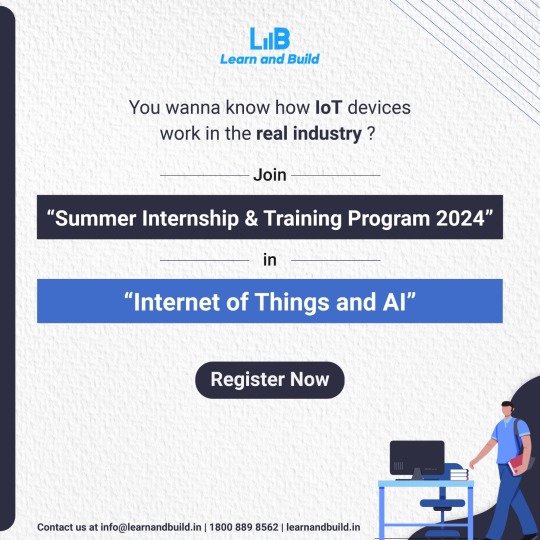
Learn and Build Summer Internship Program
For more details visit - Internship.learnandbuild.in
#data structures & algorithms#Java Core#Python Programming#Frontend web development#Backend web development#data science#machine learning & AI#Salesforce Admin#Salesforce Development#Cloud AI with AWS#Internet of things & AI#Cyber Security#Mobile app development using flutter
0 notes
Text
Learn Data Structures and Algorithms
Mastering data structures and algorithms (DSA) is one of the most effective ways to boost your coding skills and become a better programmer. Whether you're just starting out or have some experience, learning data structures and algorithms is essential for solving complex problems efficiently. By understanding how data is stored and manipulated, and by knowing the right algorithms to use, you'll write more efficient, optimized, and scalable code.
When you learn data structures and algorithms, you're not just memorizing concepts—you're developing a deeper understanding of how to approach problem-solving. For example, by mastering algorithms like QuickSort or MergeSort, you can handle large datasets with ease. Similarly, understanding data structures like arrays, stacks, and trees will allow you to choose the most appropriate structure for your problem, ensuring that your solution is both fast and memory-efficient.
0 notes
Text
"Is social media designed to reward people for acting badly?
The answer is clearly yes, given that the reward structure on social media platforms relies on popularity, as indicated by the number of responses – likes and comments – a post receives from other users. Black-box algorithms then further amplify the spread of posts that have attracted attention.
Sharing widely read content, by itself, isn’t a problem. But it becomes a problem when attention-getting, controversial content is prioritized by design. Given the design of social media sites, users form habits to automatically share the most engaging information regardless of its accuracy and potential harm. Offensive statements, attacks on out groups and false news are amplified, and misinformation often spreads further and faster than the truth.
We are two social psychologists and a marketing scholar. Our research, presented at the 2023 Nobel Prize Summit, shows that social media actually has the ability to create user habits to share high-quality content. After a few tweaks to the reward structure of social media platforms, users begin to share information that is accurate and fact-based...
Re-targeting rewards
To investigate the effect of a new reward structure, we gave financial rewards to some users for sharing accurate content and not sharing misinformation. These financial rewards simulated the positive social feedback, such as likes, that users typically receive when they share content on platforms. In essence, we created a new reward structure based on accuracy instead of attention.
As on popular social media platforms, participants in our research learned what got rewarded by sharing information and observing the outcome, without being explicitly informed of the rewards beforehand. This means that the intervention did not change the users’ goals, just their online experiences. After the change in reward structure, participants shared significantly more content that was accurate. More remarkably, users continued to share accurate content even after we removed rewards for accuracy in a subsequent round of testing. These results show that users can be given incentives to share accurate information as a matter of habit.
A different group of users received rewards for sharing misinformation and for not sharing accurate content. Surprisingly, their sharing most resembled that of users who shared news as they normally would, without any financial reward. The striking similarity between these groups reveals that social media platforms encourage users to share attention-getting content that engages others at the expense of accuracy and safety...
Doing right and doing well
Our approach, using the existing rewards on social media to create incentives for accuracy, tackles misinformation spread without significantly disrupting the sites’ business model. This has the additional advantage of altering rewards instead of introducing content restrictions, which are often controversial and costly in financial and human terms.
Implementing our proposed reward system for news sharing carries minimal costs and can be easily integrated into existing platforms. The key idea is to provide users with rewards in the form of social recognition when they share accurate news content. This can be achieved by introducing response buttons to indicate trust and accuracy. By incorporating social recognition for accurate content, algorithms that amplify popular content can leverage crowdsourcing to identify and amplify truthful information.
Both sides of the political aisle now agree that social media has challenges, and our data pinpoints the root of the problem: the design of social media platforms."
And here's the video of one of the scientsts presenting this research at the Nobel Prize Summit!
youtube
-Article via The Conversation, August 1, 2023. Video via the Nobel Prize's official Youtube channel, Nobel Prize, posted May 31, 2023.
#social media#misinformation#social networks#social#algorithm#big tech#technology#enshittification#internet#nobel prize#psychology#behavioral psychology#good news#hope#Youtube#video
496 notes
·
View notes
Text
A New Mantle Viscosity Shift
The rough picture of Earth's interior -- a crust, mantle, and core -- is well-known, but the details of its inner structure are more difficult to pin down. A recent study analyzed seismic wave data with a machine learning algorithm to identify regions of the mantle where waves slowed down. (Image credit: NASA; research credit: K. O'Farrell and Y. Wang; via Eos) Read the full article
40 notes
·
View notes
Text
Been a while, crocodiles. Let's talk about cad.

or, y'know...
Yep, we're doing a whistle-stop tour of AI in medical diagnosis!
Much like programming, AI can be conceived of, in very simple terms, as...
a way of moving from inputs to a desired output.
See, this very funky little diagram from skillcrush.com.

The input is what you put in. The output is what you get out.
This output will vary depending on the type of algorithm and the training that algorithm has undergone – you can put the same input into two different algorithms and get two entirely different sorts of answer.
Generative AI produces ‘new’ content, based on what it has learned from various inputs. We're talking AI Art, and Large Language Models like ChatGPT. This sort of AI is very useful in healthcare settings to, but that's a whole different post!
Analytical AI takes an input, such as a chest radiograph, subjects this input to a series of analyses, and deduces answers to specific questions about this input. For instance: is this chest radiograph normal or abnormal? And if abnormal, what is a likely pathology?
We'll be focusing on Analytical AI in this little lesson!
Other forms of Analytical AI that you might be familiar with are recommendation algorithms, which suggest items for you to buy based on your online activities, and facial recognition. In facial recognition, the input is an image of your face, and the output is the ability to tie that face to your identity. We’re not creating new content – we’re classifying and analysing the input we’ve been fed.
Many of these functions are obviously, um, problematique. But Computer-Aided Diagnosis is, potentially, a way to use this tool for good!
Right?
....Right?
Let's dig a bit deeper! AI is a massive umbrella term that contains many smaller umbrella terms, nested together like Russian dolls. So, we can use this model to envision how these different fields fit inside one another.
AI is the term for anything to do with creating and managing machines that perform tasks which would otherwise require human intelligence. This is what differentiates AI from regular computer programming.
Machine Learning is the development of statistical algorithms which are trained on data –but which can then extrapolate this training and generalise it to previously unseen data, typically for analytical purposes. The thing I want you to pay attention to here is the date of this reference. It’s very easy to think of AI as being a ‘new’ thing, but it has been around since the Fifties, and has been talked about for much longer. The massive boom in popularity that we’re seeing today is built on the backs of decades upon decades of research.
Artificial Neural Networks are loosely inspired by the structure of the human brain, where inputs are fed through one or more layers of ‘nodes’ which modify the original data until a desired output is achieved. More on this later!
Deep neural networks have two or more layers of nodes, increasing the complexity of what they can derive from an initial input. Convolutional neural networks are often also Deep. To become ‘convolutional’, a neural network must have strong connections between close nodes, influencing how the data is passed back and forth within the algorithm. We’ll dig more into this later, but basically, this makes CNNs very adapt at telling precisely where edges of a pattern are – they're far better at pattern recognition than our feeble fleshy eyes!
This is massively useful in Computer Aided Diagnosis, as it means CNNs can quickly and accurately trace bone cortices in musculoskeletal imaging, note abnormalities in lung markings in chest radiography, and isolate very early neoplastic changes in soft tissue for mammography and MRI.
Before I go on, I will point out that Neural Networks are NOT the only model used in Computer-Aided Diagnosis – but they ARE the most common, so we'll focus on them!
This diagram demonstrates the function of a simple Neural Network. An input is fed into one side. It is passed through a layer of ‘hidden’ modulating nodes, which in turn feed into the output. We describe the internal nodes in this algorithm as ‘hidden’ because we, outside of the algorithm, will only see the ‘input’ and the ‘output’ – which leads us onto a problem we’ll discuss later with regards to the transparency of AI in medicine.
But for now, let’s focus on how this basic model works, with regards to Computer Aided Diagnosis. We'll start with a game of...
Spot The Pathology.
yeah, that's right. There's a WHACKING GREAT RIGHT-SIDED PNEUMOTHORAX (as outlined in red - images courtesy of radiopaedia, but edits mine)
But my question to you is: how do we know that? What process are we going through to reach that conclusion?
Personally, I compared the lungs for symmetry, which led me to note a distinct line where the tissue in the right lung had collapsed on itself. I also noted the absence of normal lung markings beyond this line, where there should be tissue but there is instead air.
In simple terms.... the right lung is whiter in the midline, and black around the edges, with a clear distinction between these parts.
Let’s go back to our Neural Network. We’re at the training phase now.
So, we’re going to feed our algorithm! Homnomnom.
Let’s give it that image of a pneumothorax, alongside two normal chest radiographs (middle picture and bottom). The goal is to get the algorithm to accurately classify the chest radiographs we have inputted as either ‘normal’ or ‘abnormal’ depending on whether or not they demonstrate a pneumothorax.
There are two main ways we can teach this algorithm – supervised and unsupervised classification learning.
In supervised learning, we tell the neural network that the first picture is abnormal, and the second and third pictures are normal. Then we let it work out the difference, under our supervision, allowing us to steer it if it goes wrong.
Of course, if we only have three inputs, that isn’t enough for the algorithm to reach an accurate result.
You might be able to see – one of the normal chests has breasts, and another doesn't. If both ‘normal’ images had breasts, the algorithm could as easily determine that the lack of lung markings is what demonstrates a pneumothorax, as it could decide that actually, a pneumothorax is caused by not having breasts. Which, obviously, is untrue.
or is it?
....sadly I can personally confirm that having breasts does not prevent spontaneous pneumothorax, but that's another story lmao
This brings us to another big problem with AI in medicine –
If you are collecting your dataset from, say, a wealthy hospital in a suburban, majority white neighbourhood in America, then you will have those same demographics represented within that dataset. If we build a blind spot into the neural network, and it will discriminate based on that.
That’s an important thing to remember: the goal here is to create a generalisable tool for diagnosis. The algorithm will only ever be as generalisable as its dataset.
But there are plenty of huge free datasets online which have been specifically developed for training AI. What if we had hundreds of chest images, from a diverse population range, split between those which show pneumothoraxes, and those which don’t?
If we had a much larger dataset, the algorithm would be able to study the labelled ‘abnormal’ and ‘normal’ images, and come to far more accurate conclusions about what separates a pneumothorax from a normal chest in radiography. So, let’s pretend we’re the neural network, and pop in four characteristics that the algorithm might use to differentiate ‘normal’ from ‘abnormal’.
We can distinguish a pneumothorax by the appearance of a pleural edge where lung tissue has pulled away from the chest wall, and the radiolucent absence of peripheral lung markings around this area. So, let’s make those our first two nodes. Our last set of nodes are ‘do the lung markings extend to the chest wall?’ and ‘Are there no radiolucent areas?’
Now, red lines mean the answer is ‘no’ and green means the answer is ‘yes’. If the answer to the first two nodes is yes and the answer to the last two nodes is no, this is indicative of a pneumothorax – and vice versa.
Right. So, who can see the problem with this?
(image courtesy of radiopaedia)
This chest radiograph demonstrates alveolar patterns and air bronchograms within the right lung, indicative of a pneumonia. But if we fed it into our neural network...
The lung markings extend all the way to the chest wall. Therefore, this image might well be classified as ‘normal’ – a false negative.
Now we start to see why Neural Networks become deep and convolutional, and can get incredibly complex. In order to accurately differentiate a ‘normal’ from an ‘abnormal’ chest, you need a lot of nodes, and layers of nodes. This is also where unsupervised learning can come in.
Originally, Supervised Learning was used on Analytical AI, and Unsupervised Learning was used on Generative AI, allowing for more creativity in picture generation, for instance. However, more and more, Unsupervised learning is being incorporated into Analytical areas like Computer-Aided Diagnosis!
Unsupervised Learning involves feeding a neural network a large databank and giving it no information about which of the input images are ‘normal’ or ‘abnormal’. This saves massively on money and time, as no one has to go through and label the images first. It is also surprisingly very effective. The algorithm is told only to sort and classify the images into distinct categories, grouping images together and coming up with its own parameters about what separates one image from another. This sort of learning allows an algorithm to teach itself to find very small deviations from its discovered definition of ‘normal’.
BUT this is not to say that CAD is without its issues.
Let's take a look at some of the ethical and practical considerations involved in implementing this technology within clinical practice!
(Image from Agrawal et al., 2020)
Training Data does what it says on the tin – these are the initial images you feed your algorithm. What is key here is volume, variety - with especial attention paid to minimising bias – and veracity. The training data has to be ‘real’ – you cannot mislabel images or supply non-diagnostic images that obscure pathology, or your algorithm is useless.
Validation data evaluates the algorithm and improves on it. This involves tweaking the nodes within a neural network by altering the ‘weights’, or the intensity of the connection between various nodes. By altering these weights, a neural network can send an image that clearly fits our diagnostic criteria for a pneumothorax directly to the relevant output, whereas images that do not have these features must be put through another layer of nodes to rule out a different pathology.
Finally, testing data is the data that the finished algorithm will be tested on to prove its sensitivity and specificity, before any potential clinical use.
However, if algorithms require this much data to train, this introduces a lot of ethical questions.
Where does this data come from?
Is it ‘grey data’ (data of untraceable origin)? Is this good (protects anonymity) or bad (could have been acquired unethically)?
Could generative AI provide a workaround, in the form of producing synthetic radiographs? Or is it risky to train CAD algorithms on simulated data when the algorithms will then be used on real people?
If we are solely using CAD to make diagnoses, who holds legal responsibility for a misdiagnosis that costs lives? Is it the company that created the algorithm or the hospital employing it?
And finally – is it worth sinking so much time, money, and literal energy into AI – especially given concerns about the environment – when public opinion on AI in healthcare is mixed at best? This is a serious topic – we’re talking diagnoses making the difference between life and death. Do you trust a machine more than you trust a doctor? According to Rojahn et al., 2023, there is a strong public dislike of computer-aided diagnosis.
So, it's fair to ask...
why are we wasting so much time and money on something that our service users don't actually want?
Then we get to the other biggie.
There are also a variety of concerns to do with the sensitivity and specificity of Computer-Aided Diagnosis.
We’ve talked a little already about bias, and how training sets can inadvertently ‘poison’ the algorithm, so to speak, introducing dangerous elements that mimic biases and problems in society.
But do we even want completely accurate computer-aided diagnosis?
The name is computer-aided diagnosis, not computer-led diagnosis. As noted by Rajahn et al, the general public STRONGLY prefer diagnosis to be made by human professionals, and their desires should arguably be taken into account – as well as the fact that CAD algorithms tend to be incredibly expensive and highly specialised. For instance, you cannot put MRI images depicting CNS lesions through a chest reporting algorithm and expect coherent results – whereas a radiologist can be trained to diagnose across two or more specialties.
For this reason, there is an argument that rather than focusing on sensitivity and specificity, we should just focus on producing highly sensitive algorithms that will pick up on any abnormality, and output some false positives, but will produce NO false negatives.
(Sensitivity = a test's ability to identify sick people with a disease)
(Specificity = a test's ability to identify that healthy people do not have this disease)
This means we are working towards developing algorithms that OVERESTIMATE rather than UNDERESTIMATE disease prevalence. This makes CAD a useful tool for triage rather than providing its own diagnoses – if a CAD algorithm weighted towards high sensitivity and low specificity does not pick up on any abnormalities, it’s highly unlikely that there are any.
Finally, we have to question whether CAD is even all that accurate to begin with. 10 years ago, according to Lehmen et al., CAD in mammography demonstrated negligible improvements to accuracy. In 1989, Sutton noted that accuracy was under 60%. Nowadays, however, AI has been proven to exceed the abilities of radiologists when detecting cancers (that’s from Guetari et al., 2023). This suggests that there is a common upwards trajectory, and AI might become a suitable alternative to traditional radiology one day. But, due to the many potential problems with this field, that day is unlikely to be soon...
That's all, folks! Have some references~
#medblr#artificial intelligence#radiography#radiology#diagnosis#medicine#studyblr#radioactiveradley#radley irradiates people#long post
16 notes
·
View notes
Text
It's like, "why try to reduce chemistry to physics when you can use some kind of ML algorithm to predict chemical properties better than an actual calculation based on QFT or whatever could?". Well, the answer is obviously that that ML algorithm isn't as insightful to us. It's useful but it doesn't tell us what's going on. Trying to better understand the physics-chemistry boundary, and do reductionism, even if in practice a bunch of shit is infeasible to calculate, well, I gather it tells us structural stuff about chemicals, stuff that "plug and chug with an ML algorithm" can't presently give us.
"What's the point of doing linguistic theory if we already have LLMs". Well, because I don't know what's going on inside an LLM and neither do you. They're really good at doing translation tasks and shit but... do they give us insight into how language works? Do we have good reason to think that "things an LLM can learn" correspond closely to "things a human child can learn" linguistically? Does looking at a bunch of transformer weights tell us, e.g., what sorts of linguistic structures are cognitively + diachronically possible? Well, no. To do that we have to look at the actual linguistic data, come up with theories, test them against new data, repeat. Like scientists or whatever.
27 notes
·
View notes
Text
I'm really struggling here. There are so many things I want and need to be. SO many things I should study, so many career paths I need to take, so many things in life that I need to get to. By studying it all, I'm getting nothing done. How do I get myself together? I need to be able to prioritize what I'd like to study and where I want to be in life, so I'm writing this post to puke it all out and hopefully fix it with a little glitter. I'm making a list and categorizing them with Emojis for what I should put a longer-term pause on, what I should put up next, and what I should study now. Stuff I should study now: ✒️ Python for data analysis and machine learning ✒️ Using statistical models on python ✒️ JavaScript/React for web development ✒️ Azure AZ-900 exam prep Stuff I should get to soon but not now: 📜 Data structures & algorithms 📜 A new language Stuff that would be better to pause for now: 🤎GMAT, for my future MBA 🤎Blender, to create 3D images and interactive tools With things like my GMAT exam prep I can practice 30 minutes a day or 10 pages a day instead of actively making it a major focus of my day and missing out on the things that I really wanted to study right now. Thus, it may be better to turn my 150 days of GMAT prep into just 150 days of productivity ☕ I hope you'll understand and that hopefully, you guys are also coming to a position where you can truly focus on what you want to focus on in life
#study blog#studyspo#study motivation#daily journal#studyblr#to do list#coding#chaotic academia#chaotic thoughts#getting my shit together#realistic studyblr#studying#study tips
15 notes
·
View notes