#radiography
Explore tagged Tumblr posts
Text
"Normal lumbar spine and pelvis." Radiography and radio-therapeutics. 1917.
Internet Archive
2K notes
·
View notes
Text


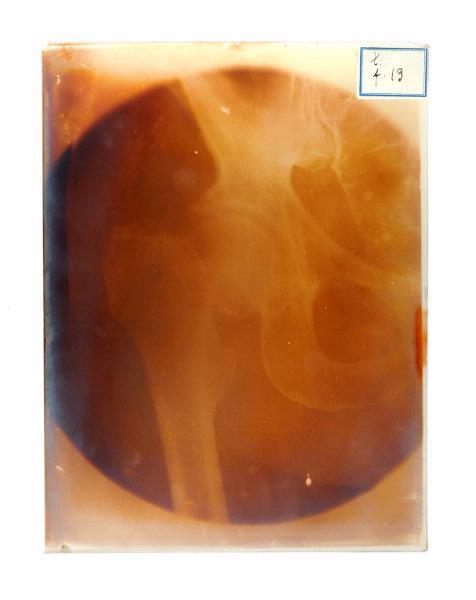
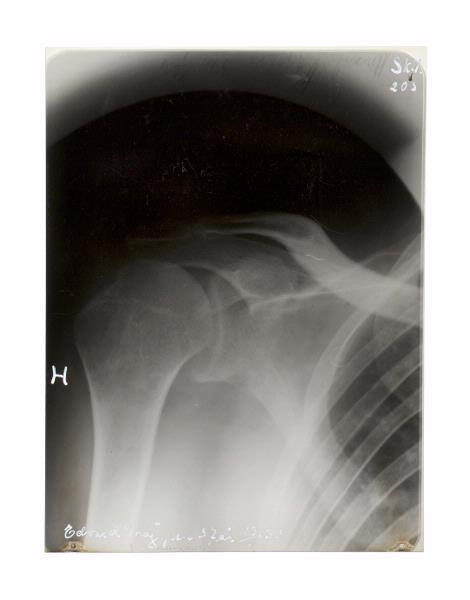
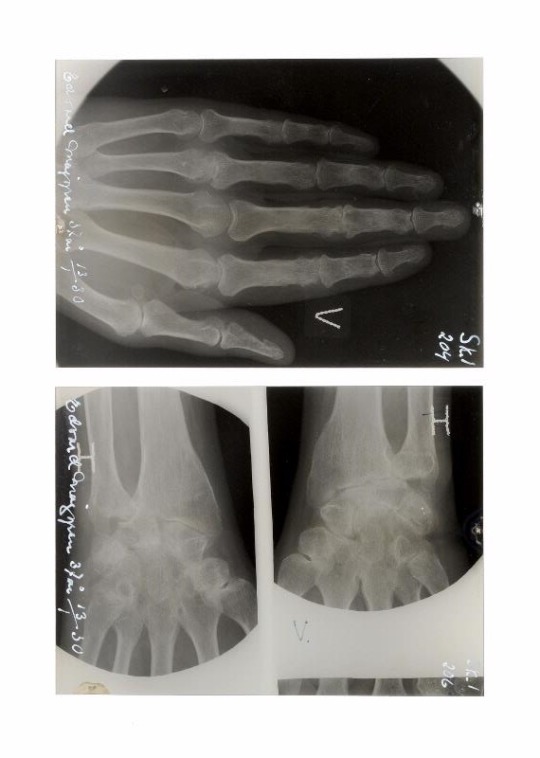
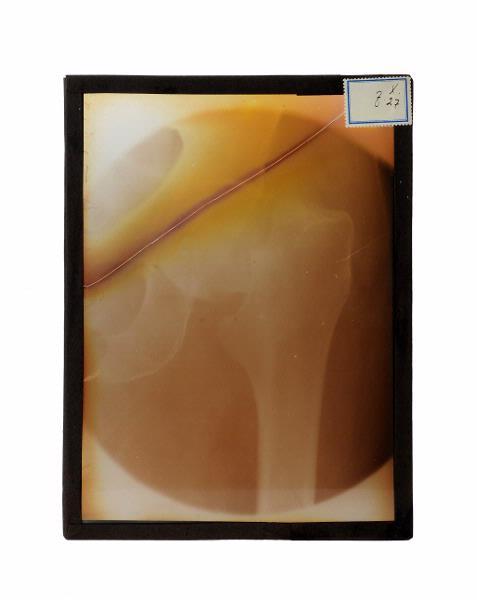
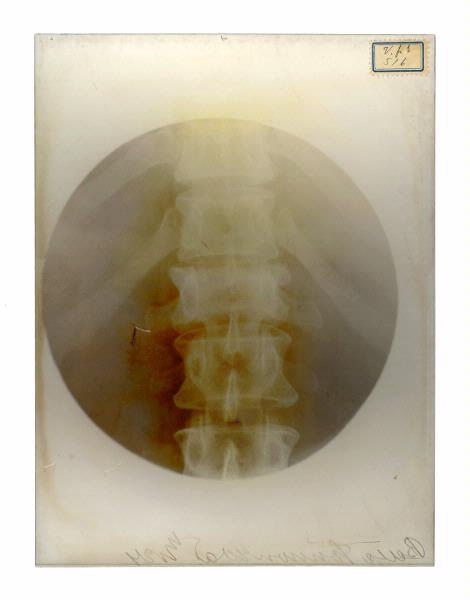

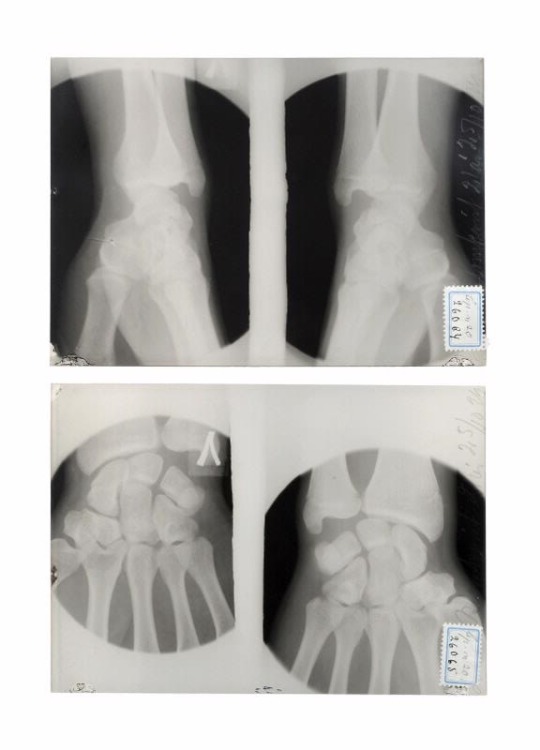

x-ray images, 1916-1931
#anatomy#x-ray image#x-ray images#vintage#medical history#x-ray#radiology#radiography#1910's#20's#30's#*
4K notes
·
View notes
Text

He looks like Harry du bois
#get well soon#bunnunny#bunny#dumb bunny#vet#radiography#animals#at the vet#medical imaging#animal memes#animalsgettingmedicalimaging#under the weather#pets#disco elysium#harry du bois
259 notes
·
View notes
Text
By popular (???) request, based on the outcome of this poll.
A WARNING: you guys really did pick the most complex one. This is loooooong. A DISCLAIMER. This is a silly little lesson aimed at folks who know sod-all about MRI. There are memes. There is (arguably) overuse of the term ‘big chungus’. If you are looking to delve deeper into the mysteries of K-Space, this is not the Tumblr post for you.
So, without further ado...
Today I am introducing you to my one true love. The legend. The icon.
Ferromagnetic material loves him. Claustrophobic people fear him.
Yeah, that’s right – we’re talking about the big boom-boom sexyboy magnet machine, hereby known as Big Chungus.
Aka...
MAGNETIC RESONANCE IMAGING
First off, though? Let’s start small.
Very, very small.
Meet HYDROGEN.
The nucleus of this element is made up of a single proton, which has a magnetic dipole – i.e., it acts like a tiny bar magnet.
Hydrogen is also a component of water. As we all know, we’re basically walking sacks of goop – meaning that Hydrogen is abundant throughout our bodies.
Therefore, when we stick you in a strong magnetic field… say, within our friend Big Chungus… we can manipulate all those tiny Hydrogen atoms in a variety of fun ways.
Under normal conditions, all your Hydrogen protons are pointing every-which-way.
But in Big Chungus, there is a strong longitudinal magnetic field that travels along the Z-axis of the machine. So, all your teeny tiny Hydrogen protons swivel to align with that field!
If a proton’s energy is LOWER than that of the longitudinal magnetic field (a majority), they will align PARALLEL with the field. If their energy is HIGHER (a minority) they will align ANTI-PARALLEL.
As most of the protons align with the longitudinal magnetic field, the net magnetisation vector within the human body is also longitudinal! This is called the thermodynamic equilibrium – the resting state for all those li’l protons when your body is within Big Chungus.
(You won’t feel any different, btw! We’re flipping a bunch of teeny-tiny bits inside you, but you won’t feel a thing!) (You might do later, when we activate the Gradient coils. We’ll….. get to that)
But, while all of this is very cool, it gives us no actual information. We gotta play some more with your protons - which brings us to arguably the most important concept in MRI. I mean, it’s literally in the name!
Let’s go back to our Hydrogen protons.
We’ve established that they’re all pointing in different directions. But they’re not just sitting still. They’re spinning and wobbling all over the shop.
We call this rotational wobbly movement precession.
In their natural state, these protons all precess at different speeds. When we subject them to Big Chungus, as well as all lining up neatly with the magnetic field, they all start to precess at the same speed.
However, their magnetic North will be pointing to different points at any given moment. Imagine two clocks, both of which are ticking at the same rate, but which have been set to read different times.
This is where magnetic resonance comes in.
In addition to the homogenous longitudinal magnetic field provided by Big Chungus, we also create an oscillating magnetic field in the transverse plane by using a radiofrequency (RF) pulse. We can tune that oscillation to the ‘resonant frequency’ of Hydrogen atoms.
Every molecule capable of resonance has its own specific frequency. We use a funky equation called the Larmor Equation to work this out, or, as I like to call it, W, BOY!!!
(The weird ‘w’ is the resonance frequency; the weird ‘Bo’ is the magnetic field strength, and the weird ‘Y’ is the gyromagnetic ratio of each particular element.)
So, we know exactly at what frequency to apply that RF pulse to your protons, to achieve resonance!
But what is resonance?
In acoustics, a ‘resonant frequency’ is the frequency an external wave needs to be applied at in order to create the maximum amplitude of vibrations within the object. Like when opera singers shatter glass with their voice! They’re singing at the resonant frequency of the glass, which makes it vibrate to the point where it compromises its structural integrity.
A similar concept applies in magnetic precession, with, uh, less destructive results. We’re not exploding anything inside of you, don’t worry!
(We do explode your innards accidentally in Ultrasound sometimes, via a different mechanism. But you’ll have to ask me more about that later. >:3)
To put it simply, magnetic resonance is the final step in getting those protons to BEHAVE. Now, the clocks have been corrected so their hands move at exactly the same time, in the same position. The protons are precessing ‘in phase’. Yay!
This creates transverse magnetisation, as the magnetic vectors of all those protons (which, remember, act as bar magnets) will swing around to point in one direction at the same time.
But the cool thing about resonance? It also allows the protons to absorb energy from the RF pulse.
(Do NOT ask me how. Do NOT. I will cry.)
And remember how the higher-energy protons flip anti-parallel to the longitudinal magnetic vector of Big Chungus, while the lower-energy protons are aligned parallel? And because we have more low-energy protons than high-energy protons, our body gains a longitudinal magnetic vector to match Big Chungus?
Zapping those protons at their resonant frequency gives 'em energy (a process known as ‘excitation’, which I love, because I get to imagine them putting little party hats on and having a rave).
So, loads of them flip anti-parallel! Enough to cancel out the net longitudinal magnetic vector of our bodies – despite the best efforts of good ol’ Chungus!
(Keep trying, Chungus. We love you.)
Our protons are as far from our happy equilibrium as they can possibly be. We’ve lost longitudinal magnetisation, and gained transverse magnetisation. Oh noooo however can we fix this ohhhh noooooo
Simple. We turn off the RF pulse.
Everything returns to that sweet, sweet thermodynamic equilibrium.
Longitudinal magnetisation is regained. I.e., the protons realign with Big Chungus’s longitudinal magnetic field, with the majority aligned parallel rather than anti-parallel.
This is called SPIN-LATTICE RELAXATION.
‘T1 time’ is the point by which 63% of longitudinal magnetisation has been regained after application of the RF pulse. A T1-weighted image shows the difference between T1 relaxation times of different tissues.
And, without that oscillating RF pulse, we lose resonance – the protons fall out of phase randomly, due to the delightful unpredictable nature of entropy, and Transverse magnetisation reduces.
This is called SPIN-SPIN RELAXATION.
Or, if we’re feeling dramatic…
‘T2 time’ is the point by which 37% of the transverse magnetisation has been lost. A T2-weighted image shows the difference between T2 relaxation times of different tissues.
(Spin-spin is objectively a hilarious phrase to say in full seriousness when surrounded by important physics-y people. However, a word to the wise: do not make a moon-moon joke. They are not on Tumblr (present company excluded). They will not understand. You will get strange looks.)
But remember how resonance lets our protons shlorp up that sweet, sweet energy from the RF pulse? Well, in order to get back to thermodynamic equilibrium and line up with Big Chungus again, they have to splort that energy back out.
This is why we stick a cage over the body part we’re imaging. That cage isn’t a magnet, or a way of keeping you still – it’s a receiver coil.
It picks up the RF signal that’s given off by your innards as they relax from the intense work-out we just put them through. How cool is that??
The amount of time we wait between applying the RF pulse and measuring the ‘echo’ from within your body is called the ‘ECHO TIME’, or ‘TE’ (because we didn’t want to call it ET).
(yes, we’re cowards. Sorry.)
We also have ‘REPETITION TIME’ or ‘TR’ – the amount of time we leave between RF pulses! This determines how much longitudinal magnetisation can recover between each pulse.
By manipulating TE and TR, we can alter the contrast (i.e., the blacks and whites) on our image.
Areas of high received signal (hyperintense) are shown as white, while areas of low received signal (hypointense) are shown as black. Different sorts of tissue will have different ratios of Hydrogen-to-other-shit, and different densities of Hydrogen-and-other-shit – ergo, some tissue blasts out all of its stored energy SUPER QUICK. Others give it off slower.
A T1-weighted image has a short TR and TE time.
Fat realigns its longitudinal magnetisation with Big Chungus SUPER QUICK. This means, on a T1-weighted image, it looks hyperintense. However, water realigns its longitudinal magnetisation with Big Chungus slooooowly. Therefore, on a T1-weighted image, fluid looks hypointense! Ya see?
A T2-weighted image has a long TR and TE time.
The precession of protons in fat decays relatively slow, so it will look quite bright on a T2-scan. But water decays slower, and therefore, by the time we take the T2 image, fluids within the body will be giving off comparatively ‘more’ signal than fat – meaning they’ll appear more hyperintense!
If we have a substance with intrinsically long T1 and T2 values, it will appear dark on a T1-weighted image and bright on a T2-weighted image, and the same in reverse. If a substance has a short T1 value and a long T2 value, it will appear relatively ‘bright’ on both T1 and T2-weighted images – i.e., fat and intervertebral discs.
As every tissue has its own distinct T1 and T2 property… we can work out precisely what sort of tissue we’re looking at.
When we build in all our additional sequences, this becomes even clearer! This is why your MRI scan takes sooooo long – we’re running SO MANY sequences, manipulating TR and TE to determine the exact T1 and T2 properties of various tissues within your bod.
There is, however, a problem.
The RF signal given off by each proton doesn’t shoot out in a handy-dandy straight line. Meaning, we have no idea where the signal is coming from within your body.
Enter our lord and saviour:
THE GRADIENT COILS.
(Shim coils are also very important – they maintain field homogeneity across the whole of Big Chungus. While Big Chungus wouldn’t need them in a perfect theoretical scenario… reality ain’t that. Big Chungus’s magnetic field is all wibbly-wobbly, so we use Shims to keep everything smooth! That’s all you need to know about them. BACK TO THE GRADIENTS.)
There are three of them, wrapping around each of the three planes of your body. When these activate, they cause those epicly eerie booming noises, characteristic of a Big Chungus ExperienceTM.
youtube
The Gradient coils are also what causes those weird tingling sensations you get in an MRI machine – which, don’t worry, aren’t permanent! Your nerves just go ‘WOAHG. THASSALOT OF MAGNET SHIT. HM. DON’T LIKE THAT.’ But they’ll calm down again once you’re freed from Big Chungus.
The gradient coils cause constant fluctuations in the magnetic field across all three dimensions. They activate sequentially, isolating one chunk of your body after the next.
As these fluctuations cause variation within the signal received, we can look at how much THAT particular signal, received at THAT particular number of milliseconds after an RF pulse, varied when THAT particular gradient was activated, in comparison to when THAT OTHER gradient was activated.
For every single bit of signal output.
That gives us A WHOLE LOTTA DATA.
^ imagine this, but the cupboard contents is just. data.
Way too much data, in fact, for our puny human brains to comprehend – so obviously, we feed it to an algorithm.
K-space is a funky computational matrix where all this info gets compiled during data acquisition. Once we’ve finished the scan sequence and have all that yummy raw data, it can be mathematically processed to create a final image!
Just like that. Simple, right?

TL;DR
You are full of Hydrogen.
Hydrogen nuclei (protons) are basically tiny magnets
These tiny magnets are orientated completely randomly, with ‘North’ pointing in all directions
We stick billions of these tiny magnets (i.e., you) into a mahoosive magnet (i.e., Big Chungus)
All the tiny magnets flip around to align with the longitudinal magnetic field of Big Chungus
High energy protons = antiparallel Low energy protons = parallel
As you have more low energy protons than high energy protons in your body, the net magnetic vector of your body is longitudinal – just like Big Chungus!
All your protons are spinning and wobbling (precessing) at random rates
We use an RF pulse, tuned to the Resonance Frequency of Hydrogen, to make ‘em precess in phase (wobble at the same time, all pointing in the same direction at once). This creates a Transverse magnetic vector.
This in-phase precession is ‘Magnetic Resonance’
Magnetic Resonance means the protons can absorb energy from the RF pulse
Now there are more high energy protons within your body! They flip antiparallel, and the net longitudinal magnetic vector of your body decreases.
We measure the time it takes for the high-energy protons to release that energy and return to alignment with the net magnetic vector of Big Chungus (Spin-Lattice Relaxation / T1 recovery)
And the time it takes for the precessing-in-phase protons to Quit That Nonsense and all start wobbling in random directions again (Spin-Spin Decay / T2 recovery)
Each tissue within your body has a different composition & density of Hydrogen atoms – which means each tissue within your body has a unique T1 & T2 recovery time
By measuring the signal at different times (TE) and by varying the frequency with which we apply RF pulses (TR), we ‘take pictures’ that show variations in the amount of signal these tissues are giving off. The signal is caught by the large radiofrequency receiver coils we put over you when you enter the machine.
Because the signal given off during recovery/decay blasts out in all directions, we don’t know exactly where it originated within your body.
Gradient coils are arranged across X, Y, and Z axes throughout the gantry of Big Chungus. They cause tiny fluctuations in the magnetic field, in sequential chunks throughout space. This is the booming noise you hear when you’re in the machine.
These tiny fluctuations cause variations in the signal we receive, depending on how close the signal is to the activated gradient coil. All this data is compiled in a magical computational matrix called K-space. A funky algorithm then decodes those variations and couples them up with the strength of the signal to give us 1) How much signal is being blasted out at that particular moment 2) Where exactly that signal comes from within your body, according to the 3D map produced by the gradient coils
It then represents these values with a pretty picture!
Tl;dr tl;dr:
71 notes
·
View notes
Text
My Story
I'm not sharing this for sympathy more just to educate people on the fact that they never know what someone is going through. Also, because people never share these types of stories
For ten years (taking me back to my single digit childhood 😭), I have had chronic back pain that's been left undiagnosed for these ten years and increasing day by day.
Physical signs of swelling and constant pain with leg weakness occasionally nowadays.
It started off with local general practitioners (GP's) telling me it was just growing pains because I was so young. Pain kept up until my double-digit years, when I continued to go back to my GP until they told me it was a pulled muscle into my early teens I continued to go to my GP who told me to go to physio privately.
My family was in no place to afford this, but thankfully, we knew some private physios who would do it for free because they were family friends.
The first private physio did some acupuncture, which eventually helped, but then the pain came back and told me it was a pinched nerve. The second private physio told me it was a pulled muscle again.
Back to my GP nearing my adulthood (according to Scottish law) they sent me to a NHS physio who did all the basic information, felt my spine -detected scoliosis-, gave me exercises and referred me to rheumatology by this time I was on a painkiller called "Naproxen"
A year later. Finally got my rheumatology appointment met with the consultant who took my information, felt my spine -detected scoliosis, hyperflexibility in my cervical and thoracic spine-, promised he'd do something about this, sent me for bloods and X-rays.
Had my bloods taken. No sign of inflammation or rheumatoid arthritis.
Nearly 3 months later, I got my x-ray results. There is nothing there time for an MRI.
2 weeks later, I had my MRI. Waited 5 further months on my results. Irregularities in my sacrioloc joint but no inflammation.
At this point, I've been through two different types of painkillers. Diclofenac and co-codamol. In my teen years. 3 different types
It's time for another x-ray. It shows nothing. Found this out the day I got accepted into my dream uni for my dream course ( I didn't get the grades. However, I'm just doing a different course at my dream university)
Went back to my GP after finding this out for the trainee GP to tell a teenage me that I "might need a hip replacement in the future." Left annoyed and upset.
Started using kinesiology tape from a suggestion from Google for back pain. It helps a little, but I still have major flare-ups.
Over the past months now in my adult years (😭😭), I've been experiencing hip pain where I've been unable to lie, sit, or put any weight on my right hip. The hip is on the same side as my back pain.
GP receptionist tells me it's not an emergency even though it is causing me to lose feeling in my right leg and that the first appointment is in a week and a half.
Appointment rolls around, and I've got another trainee who dismisses me as normal. I mentioned I thought it might be linked to my back, and the whole appointment moved from my hip to my back.
At the end of the appointment, she tells me, "Surgeons won't do anything, so I won't refer you there. Rheumatology will refer you back because you were there recently, and more X-rays and MRIs are unnecessary radiation, so we won't do that"
IM STUDYING TO BECOME A RADIOGRAPHER I KNOW THE RISKS. Her patient centred careness was thrown out the window during this appointment.
So she tells me she'll speak to her supervisor about giving my lidocaine patches to help with the pain.
Two hours later I get a phone call from her where she repeats everything she said at the appointment and follows it up with "We're going to give you more diclofenac and lanzoprazole" to which I reply "I already have lots of that. I take it constantly, " but they have continued to give me it.
After I hung up, I explained everything to my mum, and I couldn't hold back the tears. The way the NHS treats young people in constant pain is ridiculous. I'm not being listened to.
All they see and have ever seen is my age. Never the condition. And now studying radiography, and I've requested all of my medical records and images because I need to know.
I've never been in so much pain physically and mentally, but here I am. I'm struggling so much, and no one's listening to me from the medical professions.
What do I need to do to get them to listen?
#cllightning81 rambles#my story#sorry guys but it gets deptessing at the end because i am.#nhs#fuck the nhs#rheumatology#chronic pain#sacroiliac joint pain#mri#xray#physiotherapy#nhs scotland#radiography student#radiography
14 notes
·
View notes
Text








Broken bones.
Fotografías de distintos pacientes en uno de mis trabajos hace unos años 🩻
#chile tumblr#chilean#chileno#arhy#tumblr chilensis#chilegram#tumblr chilenito#chile#concepcion#santiago de chile#talcahuano#xray#radiography#fluoroscopy#pabellon#hospital#x ray#radiologist#radiology#radiologia#my post
16 notes
·
View notes
Text

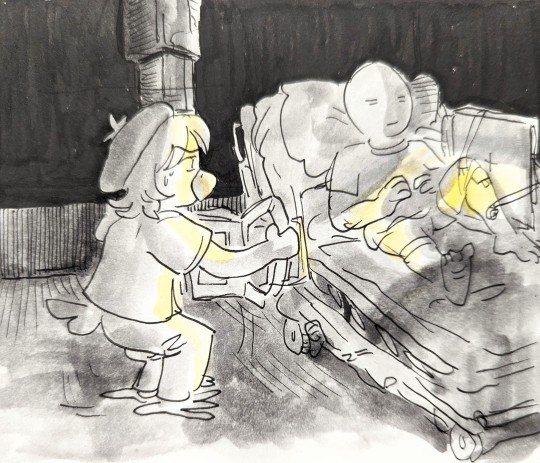
Birdsona taking Cringe Rays
20 notes
·
View notes
Text
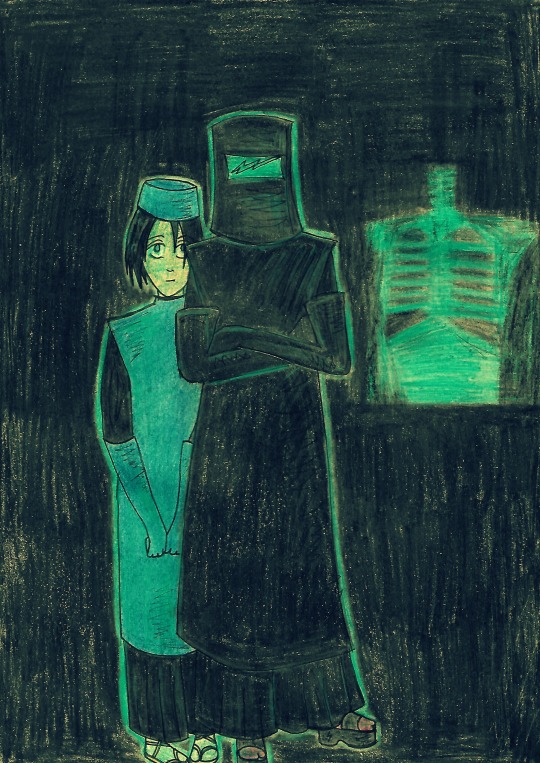
Happy B-day Hana-chan! I will protect you from all bullies, but also give you dangerous work <3
pencils, marker & filters
#hanataro yamada#suigetsu arisawa#bleach#fanfic#demoncore/bluehell#kubo tite#radiography#toxic stuff
12 notes
·
View notes
Text
I am having an early life crisis!!!
I've been looking more and more into the vascular science specialism and it might genuinely not be for me. As my physical abilities have declined (possibly arthritis?) I'm starting to think I just couldn't handle all the pain of going up down left right and sideways all day every day!
So like OK do I do radiography? I was never that great at physics and I don't really know what their day-to-day looks like.
Do I do medical imaging at all? When I was working at the law office I THRIVED but bruh the work was boring and often the impact you had on peoples' lives was 50/50 helping or devastating them. IDK if even administrative positions within the NHS would suit me.
Hell maybe I can't even handle going into the NHS. It's a 24/7 taxing ass role with very little opportunity for part-time work.
So...what do I want?? What I'd love to do is do a rotation or shadow multiple departments within the NHS but with a biomed degree it is so so so hard to get into hospitals. I really regret doing biomed not healthcare science just because a Russel Group looked better to me as a naive 19 year old.
Ugh.
5 notes
·
View notes
Text

this is not breaking any HIPPA laws this is my own chest x ray!
#x ray vision#xraytechnology#xrayservices#x ray machine#radiology#radiography#heartbeat#my heart#heartbroken#heartbreak#heartache#butterfly#vintage#filter#2016#2016 tumblr#2016 aesthetic#love#aesthetic#blurry#saturation#film photography
5 notes
·
View notes
Text
a geriatric dog presented with a little bit (?) of poopin troubles. what do you see in this radiograph?
a huge prostate pressing onto his rectum.
This is Benign Prostatic Hypertrophic (BPH). The recommended treatment is castration. And while we wait for the prostate to shrink, some laxative could help him be a little more comfortable.
20 notes
·
View notes
Text
Ouuhhhh the medical tape wrap, you just KNOW he wriggles
#the wriggler#animals#get well soon#at the vet#medical imaging#animal memes#vet#radiography#animalsgettingmedicalimaging#under the weather#pets#cat#silly cat#cat memes#cats#cats of tumblr
38 notes
·
View notes
Text
Been a while, crocodiles. Let's talk about cad.

or, y'know...
Yep, we're doing a whistle-stop tour of AI in medical diagnosis!
Much like programming, AI can be conceived of, in very simple terms, as...
a way of moving from inputs to a desired output.
See, this very funky little diagram from skillcrush.com.

The input is what you put in. The output is what you get out.
This output will vary depending on the type of algorithm and the training that algorithm has undergone – you can put the same input into two different algorithms and get two entirely different sorts of answer.
Generative AI produces ‘new’ content, based on what it has learned from various inputs. We're talking AI Art, and Large Language Models like ChatGPT. This sort of AI is very useful in healthcare settings to, but that's a whole different post!
Analytical AI takes an input, such as a chest radiograph, subjects this input to a series of analyses, and deduces answers to specific questions about this input. For instance: is this chest radiograph normal or abnormal? And if abnormal, what is a likely pathology?
We'll be focusing on Analytical AI in this little lesson!
Other forms of Analytical AI that you might be familiar with are recommendation algorithms, which suggest items for you to buy based on your online activities, and facial recognition. In facial recognition, the input is an image of your face, and the output is the ability to tie that face to your identity. We’re not creating new content – we’re classifying and analysing the input we’ve been fed.
Many of these functions are obviously, um, problematique. But Computer-Aided Diagnosis is, potentially, a way to use this tool for good!
Right?
....Right?
Let's dig a bit deeper! AI is a massive umbrella term that contains many smaller umbrella terms, nested together like Russian dolls. So, we can use this model to envision how these different fields fit inside one another.
AI is the term for anything to do with creating and managing machines that perform tasks which would otherwise require human intelligence. This is what differentiates AI from regular computer programming.
Machine Learning is the development of statistical algorithms which are trained on data –but which can then extrapolate this training and generalise it to previously unseen data, typically for analytical purposes. The thing I want you to pay attention to here is the date of this reference. It’s very easy to think of AI as being a ‘new’ thing, but it has been around since the Fifties, and has been talked about for much longer. The massive boom in popularity that we’re seeing today is built on the backs of decades upon decades of research.
Artificial Neural Networks are loosely inspired by the structure of the human brain, where inputs are fed through one or more layers of ‘nodes’ which modify the original data until a desired output is achieved. More on this later!
Deep neural networks have two or more layers of nodes, increasing the complexity of what they can derive from an initial input. Convolutional neural networks are often also Deep. To become ‘convolutional’, a neural network must have strong connections between close nodes, influencing how the data is passed back and forth within the algorithm. We’ll dig more into this later, but basically, this makes CNNs very adapt at telling precisely where edges of a pattern are – they're far better at pattern recognition than our feeble fleshy eyes!
This is massively useful in Computer Aided Diagnosis, as it means CNNs can quickly and accurately trace bone cortices in musculoskeletal imaging, note abnormalities in lung markings in chest radiography, and isolate very early neoplastic changes in soft tissue for mammography and MRI.
Before I go on, I will point out that Neural Networks are NOT the only model used in Computer-Aided Diagnosis – but they ARE the most common, so we'll focus on them!
This diagram demonstrates the function of a simple Neural Network. An input is fed into one side. It is passed through a layer of ‘hidden’ modulating nodes, which in turn feed into the output. We describe the internal nodes in this algorithm as ‘hidden’ because we, outside of the algorithm, will only see the ‘input’ and the ‘output’ – which leads us onto a problem we’ll discuss later with regards to the transparency of AI in medicine.
But for now, let’s focus on how this basic model works, with regards to Computer Aided Diagnosis. We'll start with a game of...
Spot The Pathology.
yeah, that's right. There's a WHACKING GREAT RIGHT-SIDED PNEUMOTHORAX (as outlined in red - images courtesy of radiopaedia, but edits mine)
But my question to you is: how do we know that? What process are we going through to reach that conclusion?
Personally, I compared the lungs for symmetry, which led me to note a distinct line where the tissue in the right lung had collapsed on itself. I also noted the absence of normal lung markings beyond this line, where there should be tissue but there is instead air.
In simple terms.... the right lung is whiter in the midline, and black around the edges, with a clear distinction between these parts.
Let’s go back to our Neural Network. We’re at the training phase now.
So, we’re going to feed our algorithm! Homnomnom.
Let’s give it that image of a pneumothorax, alongside two normal chest radiographs (middle picture and bottom). The goal is to get the algorithm to accurately classify the chest radiographs we have inputted as either ‘normal’ or ‘abnormal’ depending on whether or not they demonstrate a pneumothorax.
There are two main ways we can teach this algorithm – supervised and unsupervised classification learning.
In supervised learning, we tell the neural network that the first picture is abnormal, and the second and third pictures are normal. Then we let it work out the difference, under our supervision, allowing us to steer it if it goes wrong.
Of course, if we only have three inputs, that isn’t enough for the algorithm to reach an accurate result.
You might be able to see – one of the normal chests has breasts, and another doesn't. If both ‘normal’ images had breasts, the algorithm could as easily determine that the lack of lung markings is what demonstrates a pneumothorax, as it could decide that actually, a pneumothorax is caused by not having breasts. Which, obviously, is untrue.
or is it?
....sadly I can personally confirm that having breasts does not prevent spontaneous pneumothorax, but that's another story lmao
This brings us to another big problem with AI in medicine –
If you are collecting your dataset from, say, a wealthy hospital in a suburban, majority white neighbourhood in America, then you will have those same demographics represented within that dataset. If we build a blind spot into the neural network, and it will discriminate based on that.
That’s an important thing to remember: the goal here is to create a generalisable tool for diagnosis. The algorithm will only ever be as generalisable as its dataset.
But there are plenty of huge free datasets online which have been specifically developed for training AI. What if we had hundreds of chest images, from a diverse population range, split between those which show pneumothoraxes, and those which don’t?
If we had a much larger dataset, the algorithm would be able to study the labelled ‘abnormal’ and ‘normal’ images, and come to far more accurate conclusions about what separates a pneumothorax from a normal chest in radiography. So, let’s pretend we’re the neural network, and pop in four characteristics that the algorithm might use to differentiate ‘normal’ from ‘abnormal’.
We can distinguish a pneumothorax by the appearance of a pleural edge where lung tissue has pulled away from the chest wall, and the radiolucent absence of peripheral lung markings around this area. So, let’s make those our first two nodes. Our last set of nodes are ‘do the lung markings extend to the chest wall?’ and ‘Are there no radiolucent areas?’
Now, red lines mean the answer is ‘no’ and green means the answer is ‘yes’. If the answer to the first two nodes is yes and the answer to the last two nodes is no, this is indicative of a pneumothorax – and vice versa.
Right. So, who can see the problem with this?
(image courtesy of radiopaedia)
This chest radiograph demonstrates alveolar patterns and air bronchograms within the right lung, indicative of a pneumonia. But if we fed it into our neural network...
The lung markings extend all the way to the chest wall. Therefore, this image might well be classified as ‘normal’ – a false negative.
Now we start to see why Neural Networks become deep and convolutional, and can get incredibly complex. In order to accurately differentiate a ‘normal’ from an ‘abnormal’ chest, you need a lot of nodes, and layers of nodes. This is also where unsupervised learning can come in.
Originally, Supervised Learning was used on Analytical AI, and Unsupervised Learning was used on Generative AI, allowing for more creativity in picture generation, for instance. However, more and more, Unsupervised learning is being incorporated into Analytical areas like Computer-Aided Diagnosis!
Unsupervised Learning involves feeding a neural network a large databank and giving it no information about which of the input images are ‘normal’ or ‘abnormal’. This saves massively on money and time, as no one has to go through and label the images first. It is also surprisingly very effective. The algorithm is told only to sort and classify the images into distinct categories, grouping images together and coming up with its own parameters about what separates one image from another. This sort of learning allows an algorithm to teach itself to find very small deviations from its discovered definition of ‘normal’.
BUT this is not to say that CAD is without its issues.
Let's take a look at some of the ethical and practical considerations involved in implementing this technology within clinical practice!
(Image from Agrawal et al., 2020)
Training Data does what it says on the tin – these are the initial images you feed your algorithm. What is key here is volume, variety - with especial attention paid to minimising bias – and veracity. The training data has to be ‘real’ – you cannot mislabel images or supply non-diagnostic images that obscure pathology, or your algorithm is useless.
Validation data evaluates the algorithm and improves on it. This involves tweaking the nodes within a neural network by altering the ‘weights’, or the intensity of the connection between various nodes. By altering these weights, a neural network can send an image that clearly fits our diagnostic criteria for a pneumothorax directly to the relevant output, whereas images that do not have these features must be put through another layer of nodes to rule out a different pathology.
Finally, testing data is the data that the finished algorithm will be tested on to prove its sensitivity and specificity, before any potential clinical use.
However, if algorithms require this much data to train, this introduces a lot of ethical questions.
Where does this data come from?
Is it ‘grey data’ (data of untraceable origin)? Is this good (protects anonymity) or bad (could have been acquired unethically)?
Could generative AI provide a workaround, in the form of producing synthetic radiographs? Or is it risky to train CAD algorithms on simulated data when the algorithms will then be used on real people?
If we are solely using CAD to make diagnoses, who holds legal responsibility for a misdiagnosis that costs lives? Is it the company that created the algorithm or the hospital employing it?
And finally – is it worth sinking so much time, money, and literal energy into AI – especially given concerns about the environment – when public opinion on AI in healthcare is mixed at best? This is a serious topic – we’re talking diagnoses making the difference between life and death. Do you trust a machine more than you trust a doctor? According to Rojahn et al., 2023, there is a strong public dislike of computer-aided diagnosis.
So, it's fair to ask...
why are we wasting so much time and money on something that our service users don't actually want?
Then we get to the other biggie.
There are also a variety of concerns to do with the sensitivity and specificity of Computer-Aided Diagnosis.
We’ve talked a little already about bias, and how training sets can inadvertently ‘poison’ the algorithm, so to speak, introducing dangerous elements that mimic biases and problems in society.
But do we even want completely accurate computer-aided diagnosis?
The name is computer-aided diagnosis, not computer-led diagnosis. As noted by Rajahn et al, the general public STRONGLY prefer diagnosis to be made by human professionals, and their desires should arguably be taken into account – as well as the fact that CAD algorithms tend to be incredibly expensive and highly specialised. For instance, you cannot put MRI images depicting CNS lesions through a chest reporting algorithm and expect coherent results – whereas a radiologist can be trained to diagnose across two or more specialties.
For this reason, there is an argument that rather than focusing on sensitivity and specificity, we should just focus on producing highly sensitive algorithms that will pick up on any abnormality, and output some false positives, but will produce NO false negatives.
(Sensitivity = a test's ability to identify sick people with a disease)
(Specificity = a test's ability to identify that healthy people do not have this disease)
This means we are working towards developing algorithms that OVERESTIMATE rather than UNDERESTIMATE disease prevalence. This makes CAD a useful tool for triage rather than providing its own diagnoses – if a CAD algorithm weighted towards high sensitivity and low specificity does not pick up on any abnormalities, it’s highly unlikely that there are any.
Finally, we have to question whether CAD is even all that accurate to begin with. 10 years ago, according to Lehmen et al., CAD in mammography demonstrated negligible improvements to accuracy. In 1989, Sutton noted that accuracy was under 60%. Nowadays, however, AI has been proven to exceed the abilities of radiologists when detecting cancers (that’s from Guetari et al., 2023). This suggests that there is a common upwards trajectory, and AI might become a suitable alternative to traditional radiology one day. But, due to the many potential problems with this field, that day is unlikely to be soon...
That's all, folks! Have some references~
#medblr#artificial intelligence#radiography#radiology#diagnosis#medicine#studyblr#radioactiveradley#radley irradiates people#long post
16 notes
·
View notes
Text
Underground Radio (No Money Remix) is on the 13 (ALBUM) LYS 030
#radio#radioshow#radiojavan#radiohead#radiohost#radiostation#radiopersonality#radiology#RadioDJ#radiolife#Radioactive#radiofrequency#radiocontrol#radiorecord#RadioPlay#radiofrequencia#radio1#radiologia#RadioStations#radiointerview#radiocity#radioshows#radiocitymusichall#radiodeejay#radiography#radioonline#radioone#radiologist#Radiofrecuencia#radiomir
6 notes
·
View notes
Note
i was just saying i haven’t seen ru in a long time😭 please take yourself and take breaks so you don’t crash🫶🏾
Thank you darling! I’m hoping not to crush because everyone has been saying this is hardest stretch of the course because it’s close to Christmas as our exam is after 😭
[I will still be updating - dw 🫶🏾]
4 notes
·
View notes
Text
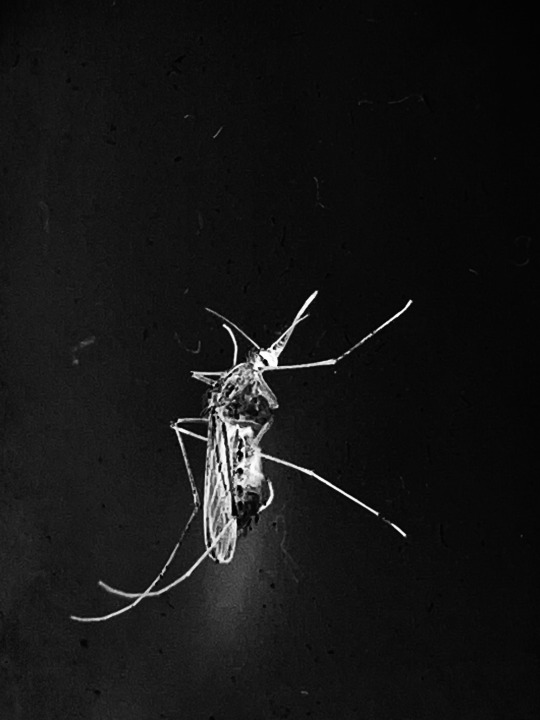
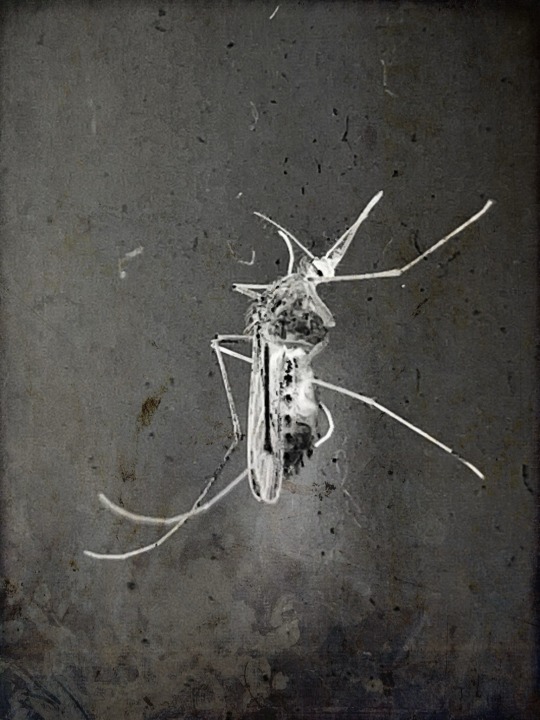
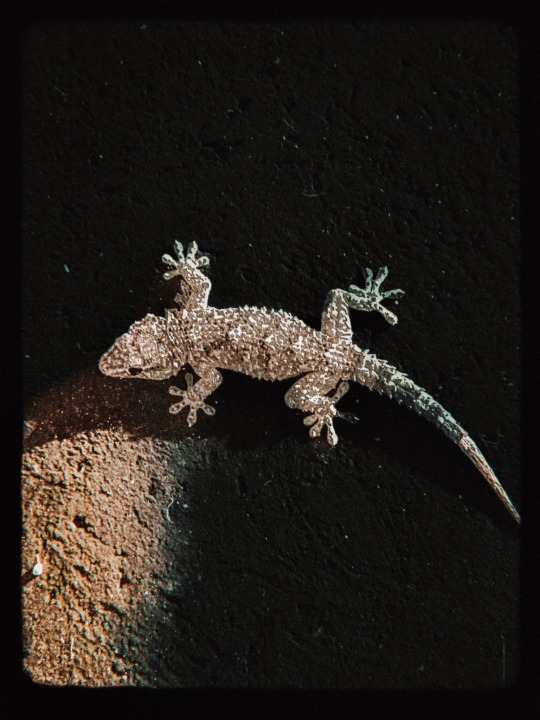
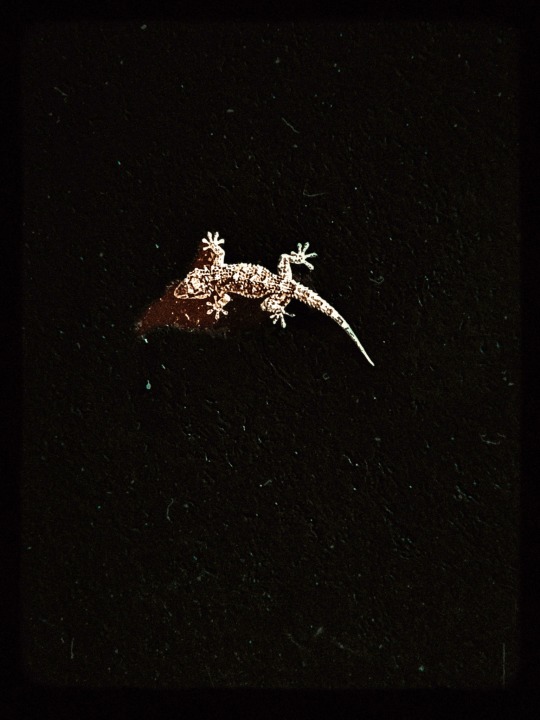
Ninnananna amore mio___Lullaby my love
#alchimilla#katia celestini#artists on tumblr#photographers on tumblr#magic photography#enchanted photography#gothic photography#life and death#gothic art#aesthetic mood#radiography#creatures#little animals#geko#mosquito
7 notes
·
View notes