#Difference between data science and machine learning
Explore tagged Tumblr posts
Text
#difference between data science and machine learning#what is data science#data science#skills required for a data scientist
0 notes
Text
This article highlights the key difference between Machine Learning and Artificial Intelligence based on approach, learning, application, output, complexity, etc.
#Difference between AI and ML#ai vs ml#artificial intelligence vs machine learning#key differences between ai and ml#artificial intelligence#machine learning#AI#ML#technology#data science#automation#robotics#neural networks#deep learning#natural language processing#computer vision#predictive analytics#big data#future trends.
2 notes
·
View notes
Text
Complete Excel, AI and Data Science mega bundle.
Unlock Your Full Potential with Our 100-Hour Masterclass: The Ultimate Guide to Excel, Python, and AI.
Why Choose This Course? In today’s competitive job market, mastering a range of technical skills is more important than ever. Our 100-hour comprehensive course is designed to equip you with in-demand capabilities in Excel, Python, and Artificial Intelligence (AI), providing you with the toolkit you need to excel in the digital age.
To read more click here <<
Become an Excel Pro Delve deep into the intricacies of Excel functions, formulae, and data visualization techniques. Whether you’re dealing with basic tasks or complex financial models, this course will make you an Excel wizard capable of tackling any challenge.
Automate Your Workflow with Python Scripting in Python doesn’t just mean writing code; it means reclaiming your time. Automate everyday tasks, interact with software applications, and boost your productivity exponentially.
If you want to get full course click here <<
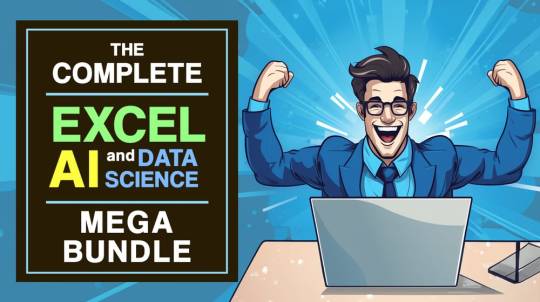
Turn Ideas into Apps Discover the potential of Amazon Honeycode to create custom apps tailored to your needs. Whether it’s for data management, content tracking, or inventory — transform your creative concepts into practical solutions.
Be Your Own Financial Analyst Unlock the financial functionalities of Excel to manage and analyze business data. Create Profit and Loss statements, balance sheets, and conduct forecasting with ease, equipping you to make data-driven decisions.
Embark on an AI Journey Step into the future with AI and machine learning. Learn to build advanced models, understand neural networks, and employ TensorFlow. Turn big data into actionable insights and predictive models.
Master Stock Prediction Gain an edge in the market by leveraging machine learning for stock prediction. Learn to spot trends, uncover hidden patterns, and make smarter investment decisions.
Who Is This Course For? Whether you’re a complete beginner or a seasoned professional looking to upskill, this course offers a broad and deep understanding of Excel, Python, and AI, preparing you for an ever-changing work environment.
Invest in Your Future This isn’t just a course; it’s a game-changer for your career. Enroll now and set yourself on a path to technological mastery and unparalleled career growth.
Don’t Wait, Transform Your Career Today! Click here to get full course <<
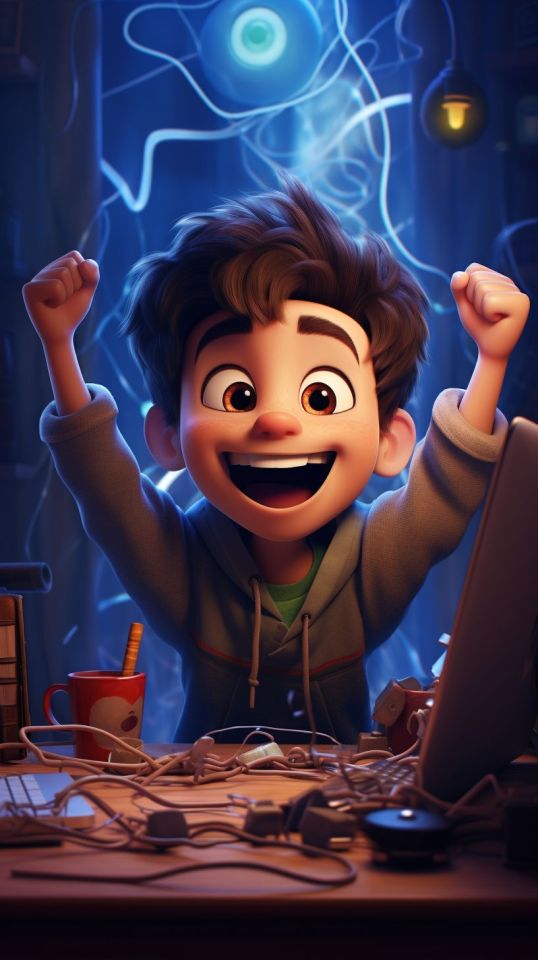
#data science#complete excel course#excel#data science and machine learning#microsoft excel#difference between ai and data science#learn excel#complete microsoft excel tutorial#difference between data science and data engineering#365 data science#aegis school of data science#advanced excel#excel tips and tricks#advanced excel full course#computer science#ms in data science#pgp in data science#python data science#python data science tutorial#Tumblr
1 note
·
View note
Text
Caution: Universe Work Ahead 🚧
We only have one universe. That’s usually plenty – it’s pretty big after all! But there are some things scientists can’t do with our real universe that they can do if they build new ones using computers.
The universes they create aren’t real, but they’re important tools to help us understand the cosmos. Two teams of scientists recently created a couple of these simulations to help us learn how our Nancy Grace Roman Space Telescope sets out to unveil the universe’s distant past and give us a glimpse of possible futures.
Caution: you are now entering a cosmic construction zone (no hard hat required)!
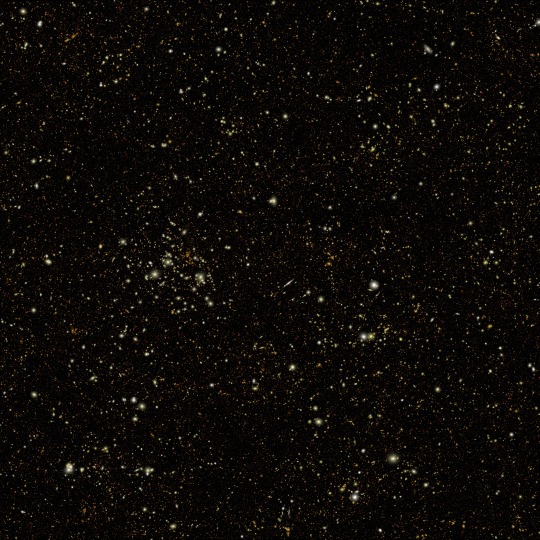
This simulated Roman deep field image, containing hundreds of thousands of galaxies, represents just 1.3 percent of the synthetic survey, which is itself just one percent of Roman's planned survey. The full simulation is available here. The galaxies are color coded – redder ones are farther away, and whiter ones are nearer. The simulation showcases Roman’s power to conduct large, deep surveys and study the universe statistically in ways that aren’t possible with current telescopes.
One Roman simulation is helping scientists plan how to study cosmic evolution by teaming up with other telescopes, like the Vera C. Rubin Observatory. It’s based on galaxy and dark matter models combined with real data from other telescopes. It envisions a big patch of the sky Roman will survey when it launches by 2027. Scientists are exploring the simulation to make observation plans so Roman will help us learn as much as possible. It’s a sneak peek at what we could figure out about how and why our universe has changed dramatically across cosmic epochs.
youtube
This video begins by showing the most distant galaxies in the simulated deep field image in red. As it zooms out, layers of nearer (yellow and white) galaxies are added to the frame. By studying different cosmic epochs, Roman will be able to trace the universe's expansion history, study how galaxies developed over time, and much more.
As part of the real future survey, Roman will study the structure and evolution of the universe, map dark matter – an invisible substance detectable only by seeing its gravitational effects on visible matter – and discern between the leading theories that attempt to explain why the expansion of the universe is speeding up. It will do it by traveling back in time…well, sort of.
Seeing into the past
Looking way out into space is kind of like using a time machine. That’s because the light emitted by distant galaxies takes longer to reach us than light from ones that are nearby. When we look at farther galaxies, we see the universe as it was when their light was emitted. That can help us see billions of years into the past. Comparing what the universe was like at different ages will help astronomers piece together the way it has transformed over time.
This animation shows the type of science that astronomers will be able to do with future Roman deep field observations. The gravity of intervening galaxy clusters and dark matter can lens the light from farther objects, warping their appearance as shown in the animation. By studying the distorted light, astronomers can study elusive dark matter, which can only be measured indirectly through its gravitational effects on visible matter. As a bonus, this lensing also makes it easier to see the most distant galaxies whose light they magnify.
The simulation demonstrates how Roman will see even farther back in time thanks to natural magnifying glasses in space. Huge clusters of galaxies are so massive that they warp the fabric of space-time, kind of like how a bowling ball creates a well when placed on a trampoline. When light from more distant galaxies passes close to a galaxy cluster, it follows the curved space-time and bends around the cluster. That lenses the light, producing brighter, distorted images of the farther galaxies.
Roman will be sensitive enough to use this phenomenon to see how even small masses, like clumps of dark matter, warp the appearance of distant galaxies. That will help narrow down the candidates for what dark matter could be made of.
In this simulated view of the deep cosmos, each dot represents a galaxy. The three small squares show Hubble's field of view, and each reveals a different region of the synthetic universe. Roman will be able to quickly survey an area as large as the whole zoomed-out image, which will give us a glimpse of the universe’s largest structures.
Constructing the cosmos over billions of years
A separate simulation shows what Roman might expect to see across more than 10 billion years of cosmic history. It’s based on a galaxy formation model that represents our current understanding of how the universe works. That means that Roman can put that model to the test when it delivers real observations, since astronomers can compare what they expected to see with what’s really out there.
In this side view of the simulated universe, each dot represents a galaxy whose size and brightness corresponds to its mass. Slices from different epochs illustrate how Roman will be able to view the universe across cosmic history. Astronomers will use such observations to piece together how cosmic evolution led to the web-like structure we see today.
This simulation also shows how Roman will help us learn how extremely large structures in the cosmos were constructed over time. For hundreds of millions of years after the universe was born, it was filled with a sea of charged particles that was almost completely uniform. Today, billions of years later, there are galaxies and galaxy clusters glowing in clumps along invisible threads of dark matter that extend hundreds of millions of light-years. Vast “cosmic voids” are found in between all the shining strands.
Astronomers have connected some of the dots between the universe’s early days and today, but it’s been difficult to see the big picture. Roman’s broad view of space will help us quickly see the universe’s web-like structure for the first time. That’s something that would take Hubble or Webb decades to do! Scientists will also use Roman to view different slices of the universe and piece together all the snapshots in time. We’re looking forward to learning how the cosmos grew and developed to its present state and finding clues about its ultimate fate.
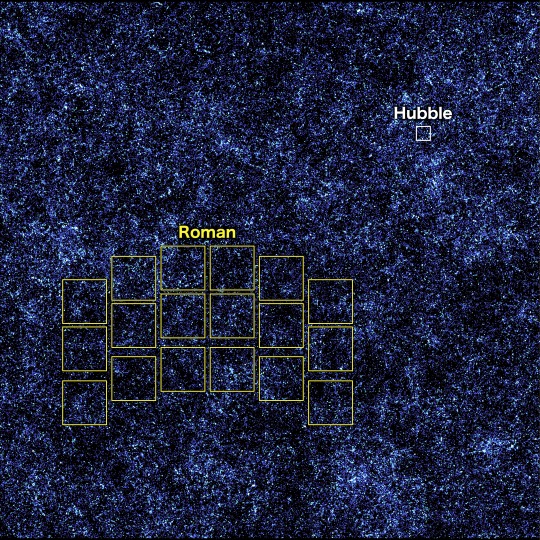
This image, containing millions of simulated galaxies strewn across space and time, shows the areas Hubble (white) and Roman (yellow) can capture in a single snapshot. It would take Hubble about 85 years to map the entire region shown in the image at the same depth, but Roman could do it in just 63 days. Roman’s larger view and fast survey speeds will unveil the evolving universe in ways that have never been possible before.
Roman will explore the cosmos as no telescope ever has before, combining a panoramic view of the universe with a vantage point in space. Each picture it sends back will let us see areas that are at least a hundred times larger than our Hubble or James Webb space telescopes can see at one time. Astronomers will study them to learn more about how galaxies were constructed, dark matter, and much more.
The simulations are much more than just pretty pictures – they’re important stepping stones that forecast what we can expect to see with Roman. We’ve never had a view like Roman’s before, so having a preview helps make sure we can make the most of this incredible mission when it launches.
Learn more about the exciting science this mission will investigate on Twitter and Facebook.
Make sure to follow us on Tumblr for your regular dose of space!
#NASA#astronomy#telescope#Roman Space Telescope#dark matter#galaxies#cosmology#astrophysics#stars#galaxy#Hubble#Webb#spaceblr
2K notes
·
View notes
Note
Hiii! Sorry if I keep sending you more ask-
I was wondering what if Mayhem accidentally got transported into the TFP universe? (Considering it's the Idw comics, anything wacky is possible 😭)
How will Soundwave from that universe react to Mayhem? And will Mayhem react to his 'dad'?
Thanks for the ask! Sorry for being so late.
In reality this could go down in many different ways.
TFP Soundwave, as far as I've seen him, holds Laserbeak as his own last shard of sanity, maybe in the past he had all of them and, well, the gladiator pits, the war, that little drone is all he has left, I don't know if in TFP the concept of sparkling or new sparks exist like it does on BV or this AU, but maybe Laserbeak was someone like that for him.
Having that in mind, as in by some fucked up chain of events (as in having Sunset near with his inherited bad and absurd luck), Mayhem were to fall in any other universe (because after the whole Brainstorm-made-a-time-machine-and-teared-the-whole-reality-apart well, now we have a multiverse!) you can bet that first and foremost Soundwave, as in the one in this reality, will tear even more the barriers between realities to have his sparkling back.
Meanwhile TFP Soundwave looks at the sparkling with a big interrogation mark on his visor, because where did he come from? How come his bio signature is so similar to his but he can't pinpoint from where, or what, the other part has come? How old is him? Depending of such, if Mayhem is still a newly forged mech then you can bet TFP Soundwave will guard him like a jealous mother (in that phase they aren't different from human babies), no one can look at such delicate little sparkle of joy, if Megatron asks nicely and is obviously in his right mind maybe he would consider it, if Mayhem was already an operative mech it would be so much difficult to hide him, and he has to drag him back more than once when he tries to reach the autobots, Mayhem has learned a lot from his sire back in his own reality, to this point everyone knows about him, the vehicons know better than stop him because he can only get so far before a pair of tentacles catch and drag him back again, no matter that his sharp digits claw at the ground, once back inside he is given a portion of energon to please be calm.
He would never let him interact with Starscream by obvious reasons, maybe Breakdown can talk more comfortable with him, but absolutely no permission to talk or interact or even be in the presence of Shockwave or Knockout, Soundwave doesn't like their look of "science" when they look at Mayhem.
Now with SG Soundwave, believe it or not, Mayhem feels even more horrified by different reasons, all his life his sire, his father, has always been taciturn, quiet, maybe a little gloomy, but overall he always thrived in his affection, while not so big like he has seen with other families, he knows his father loves him with little things.
SG Soundwave makes him sputter with his headband and overall colors, the way he talks and moves are so unnatural that make him feel itchy, but above all else what gets him totally out of his zone is that SG Soundwave makes a quick scanner at him, processes the data in two clicks, and soon tackles him down with a hug calling him "my baby".
In the SG universe they know of different realities thanks to Cliffjumper, and SG Soundwave has to recompose himself a moment and let go of the young mech, there is paint transfer in both of them due to the obvious crash.
If TFP Soundwave was creepy to Mayhem, he can't even start to describe SG Soundwave, but he does recognize him as creepy in his own way.
Obviously this is the most talkative Soundwave, "How many centuries are you? Have you been well from where you come from? Are you a medic? So proud! Do your do my young mech! Did you came from the hotspot in Kaon like me? If you didn't that's fine too!"
And obviously so, this Soundwave gets on the slightly different wavelength Mayhem does, "Do you have a sire? A carrier? Did I've you alone?" as he shows every other righteous decepticon the young mech, Mayhem has never seen other decepticons so fast and easy, they look happy to see him, totally different from his reality, "Such a handsome young lad!", somebot says, and SG Soundwave is quick to answer: "Sure is! Bet he got it from his other mentor!" as his visor shines green with absolute glee, the questions previously done return and Mayhem doesn't know if he should mangle this reality and tell him, he decided not to, because everything is backwards here, what if you are different too?
Mayhem doesn't want to answer, and he gets another cybertronian equivalent of aneurism when he meets the cassettes of this reality, at least he got to meet SG Ravage, because he never meet the one of his reality, never had the chance.
SG Soundwave is maybe the only one to help him return to his reality, retracting his battle mask to give a little peck on his helm, "Take care of you and the fam', Lil' doc".
Once Mayhem is safely returned, SG Soundwave makes a bee line to you, who seems to have a very bad day or your usual sour and tired expression is somehow worse, drinking some kind of human beverage to keep your sanity intact, but every ounce of sanity it's throw out of the window when he sits next to you, hands together as if he is begging or praying, your coffee is dripping from your mouth as he says "I wanna've your sparkling, my amor", because he recognized the wavelength of Mayhem's spark mimicking the one of a human, a human he knows very well.
Flatline has to come and help you as your coffee goes down the wrong way, Soundwave has this idea on his helm and nothing, nothing,will take it away.
Your destiny is sealed as Megatron looks at you helplessly, maybe you have a reason to date Soundwave now.
#transformers#x reader#tf mtmte#transformers x reader#reader insert#transformers idw#angst#transformers x human reader#tf soundwave#soundwave x human reader#soundwave x reader
58 notes
·
View notes
Text
NP-Completeness
Machine learning #1
Complexity Recap
Assuming one already knows what algorithmic complexity is, I am writing this paragraph to explain it to those who don't. The complexity of an algorithm can be measured by its efficiency. So let's say I have a problem P for which I've proposed two algorithmsA and B as solutions. How could I determine which algorithm is the most efficient in terms of time and data support? Well, by using complexity ( space complexity and time complexity ). One way of measuring complexity is O notation.
Exponential Complexity O(2^n)
In algorithms, this complexity arises when the time or space required for computation doubles with each additional element in the input. Problems with exponential complexity are typically NP-hard or NP-complete
P-Class and NP-Class
The P-class represents the set of polynomial problems, i.e., problems for which a solution can be found in polynomial time.
The NP-class represents the set of non-deterministic polynomial problems, i.e., problems for which a solution can be verified in polynomial time. This means it's easy to verify if a proposed solution is correct, but finding that solution might be difficult.
P = NP Question?
The question of whether P is different from NP is one of the most important problems in theoretical computer science. It is part of the seven problems selected by the Clay Institute in the year 2000, for which a reward of one million dollars is offered to whoever solves them.
NP-Complete Problem Class
NP-complete problems are problems that are both in the NP-class and are harder than all other problems in the NP-class.Solving any of these problems would be considered a major breakthrough in theoretical computer science and could have a significant impact in many areas such as optimization, planning, cryptography, etc. There is no specific monetary reward associated with solving an NP-complete problem, but it would be considered a significant achievement.
From an NP Problem to Another
A polynomial reduction is a process that transforms an instance of one problem into an equivalent instance of another problem. In the case of polynomial reduction between NP-complete problems, this means that if you can efficiently solve problem B, then you can also efficiently solve problem A. This leads to the fact that if only one problem is solved from the list, then all the other problems will also be solved.
Approximation Algorithms
Computer scientists have devised approximation algorithms to efficiently solve NP-complete problems, even if finding an exact solution in polynomial time is not possible. These algorithms seek to find a solution that is close to optimality, although it may not be the optimal solution.
We can divide them into two categories:
Heuristics: These are explicit algorithms that propose solutions for NP-complete problems.
Metaheuristics: Unlike heuristics, metaheuristics are general strategies that guide the search for a solution without prescribing a specific algorithm.
#codeblr#code#css#html#javascript#java development company#python#studyblr#progblr#programming#comp sci#web design#web developers#web development#website design#webdev#website#tech#html css#learn to code
21 notes
·
View notes
Text
What's the difference between Machine Learning and AI?
Machine Learning and Artificial Intelligence (AI) are often used interchangeably, but they represent distinct concepts within the broader field of data science. Machine Learning refers to algorithms that enable systems to learn from data and make predictions or decisions based on that learning. It's a subset of AI, focusing on statistical techniques and models that allow computers to perform specific tasks without explicit programming.

On the other hand, AI encompasses a broader scope, aiming to simulate human intelligence in machines. It includes Machine Learning as well as other disciplines like natural language processing, computer vision, and robotics, all working towards creating intelligent systems capable of reasoning, problem-solving, and understanding context.
Understanding this distinction is crucial for anyone interested in leveraging data-driven technologies effectively. Whether you're exploring career opportunities, enhancing business strategies, or simply curious about the future of technology, diving deeper into these concepts can provide invaluable insights.
In conclusion, while Machine Learning focuses on algorithms that learn from data to make decisions, Artificial Intelligence encompasses a broader range of technologies aiming to replicate human intelligence. Understanding these distinctions is key to navigating the evolving landscape of data science and technology. For those eager to deepen their knowledge and stay ahead in this dynamic field, exploring further resources and insights on can provide valuable perspectives and opportunities for growth
5 notes
·
View notes
Text
What are AI, AGI, and ASI? And the positive impact of AI
Understanding artificial intelligence (AI) involves more than just recognizing lines of code or scripts; it encompasses developing algorithms and models capable of learning from data and making predictions or decisions based on what they’ve learned. To truly grasp the distinctions between the different types of AI, we must look at their capabilities and potential impact on society.
To simplify, we can categorize these types of AI by assigning a power level from 1 to 3, with 1 being the least powerful and 3 being the most powerful. Let’s explore these categories:
1. Artificial Narrow Intelligence (ANI)
Also known as Narrow AI or Weak AI, ANI is the most common form of AI we encounter today. It is designed to perform a specific task or a narrow range of tasks. Examples include virtual assistants like Siri and Alexa, recommendation systems on Netflix, and image recognition software. ANI operates under a limited set of constraints and can’t perform tasks outside its specific domain. Despite its limitations, ANI has proven to be incredibly useful in automating repetitive tasks, providing insights through data analysis, and enhancing user experiences across various applications.
2. Artificial General Intelligence (AGI)
Referred to as Strong AI, AGI represents the next level of AI development. Unlike ANI, AGI can understand, learn, and apply knowledge across a wide range of tasks, similar to human intelligence. It can reason, plan, solve problems, think abstractly, and learn from experiences. While AGI remains a theoretical concept as of now, achieving it would mean creating machines capable of performing any intellectual task that a human can. This breakthrough could revolutionize numerous fields, including healthcare, education, and science, by providing more adaptive and comprehensive solutions.
3. Artificial Super Intelligence (ASI)
ASI surpasses human intelligence and capabilities in all aspects. It represents a level of intelligence far beyond our current understanding, where machines could outthink, outperform, and outmaneuver humans. ASI could lead to unprecedented advancements in technology and society. However, it also raises significant ethical and safety concerns. Ensuring ASI is developed and used responsibly is crucial to preventing unintended consequences that could arise from such a powerful form of intelligence.
The Positive Impact of AI
When regulated and guided by ethical principles, AI has the potential to benefit humanity significantly. Here are a few ways AI can help us become better:
• Healthcare: AI can assist in diagnosing diseases, personalizing treatment plans, and even predicting health issues before they become severe. This can lead to improved patient outcomes and more efficient healthcare systems.
• Education: Personalized learning experiences powered by AI can cater to individual student needs, helping them learn at their own pace and in ways that suit their unique styles.
• Environment: AI can play a crucial role in monitoring and managing environmental changes, optimizing energy use, and developing sustainable practices to combat climate change.
• Economy: AI can drive innovation, create new industries, and enhance productivity by automating mundane tasks and providing data-driven insights for better decision-making.
In conclusion, while AI, AGI, and ASI represent different levels of technological advancement, their potential to transform our world is immense. By understanding their distinctions and ensuring proper regulation, we can harness the power of AI to create a brighter future for all.
7 notes
·
View notes
Text
Language Models and AI Safety: Still Worrying
Previously, I have explained how modern "AI" research has painted itself into a corner, inventing the science fiction rogue AI scenario where a system is smarter than its guardrails, but can easily outwitted by humans.
Two recent examples have confirmed my hunch about AI safety of generative AI. In one well-circulated case, somebody generated a picture of an "ethnically ambiguous Homer Simpson", and in another, somebody created a picture of "baby, female, hispanic".
These incidents show that generative AI still filters prompts and outputs, instead of A) ensuring the correct behaviour during training/fine-tuning, B) manually generating, re-labelling, or pruning the training data, C) directly modifying the learned weights to affect outputs.
In general, it is not surprising that big corporations like Google and Microsoft and non-profits like OpenAI are prioritising racist language or racial composition of characters in generated images over abuse of LLMs or generative art for nefarious purposes, content farms, spam, captcha solving, or impersonation. Somebody with enough criminal energy to use ChatGPT to automatically impersonate your grandma based on your message history after he hacked the phones of tens of thousands of grandmas will be blamed for his acts. Somebody who unintentionally generates a racist picture based on an ambiguous prompt will blame the developers of the software if he's offended. Scammers could have enough money and incentives to run the models on their own machine anyway, where corporations have little recourse.
There is precedent for this. Word2vec, published in 2013, was called a "sexist algorithm" in attention-grabbing headlines, even though the bodies of such articles usually conceded that the word2vec embedding just reproduced patterns inherent in the training data: Obviously word2vec does not have any built-in gender biases, it just departs from the dictionary definitions of words like "doctor" and "nurse" and learns gendered connotations because in the training corpus doctors are more often men, and nurses are more often women. Now even that last explanation is oversimplified. The difference between "man" and "woman" is not quite the same as the difference between "male" and "female", or between "doctor" and "nurse". In the English language, "man" can mean "male person" or "human person", and "nurse" can mean "feeding a baby milk from your breast" or a kind of skilled health care worker who works under the direction and supervision of a licensed physician. Arguably, the word2vec algorithm picked up on properties of the word "nurse" that are part of the meaning of the word (at least one meaning, according tot he dictionary), not properties that are contingent on our sexist world.
I don't want to come down against "political correctness" here. I think it's good if ChatGPT doesn't tell a girl that girls can't be doctors. You have to understand that not accidentally saying something sexist or racist is a big deal, or at least Google, Facebook, Microsoft, and OpenAI all think so. OpenAI are responding to a huge incentive when they add snippets like "ethnically ambiguous" to DALL-E 3 prompts.
If this is so important, why are they re-writing prompts, then? Why are they not doing A, B, or C? Back in the days of word2vec, there was a simple but effective solution to automatically identify gendered components in the learned embedding, and zero out the difference. It's so simple you'll probably kick yourself reading it because you could have published that paper yourself without understanding how word2vec works.
I can only conclude from the behaviour of systems like DALL-E 3 that they are either using simple prompt re-writing (or a more sophisticated approach that behaves just as prompt rewriting would, and performs as badly) because prompt re-writing is the best thing they can come up with. Transformers are complex, and inscrutable. You can't just reach in there, isolate a concept like "human person", and rebalance the composition.
The bitter lesson tells us that big amorphous approaches to AI perform better and scale better than manually written expert systems, ontologies, or description logics. More unsupervised data beats less but carefully labelled data. Even when the developers of these systems have a big incentive not to reproduce a certain pattern from the data, they can't fix such a problem at the root. Their solution is instead to use a simple natural language processing system, a dumb system they can understand, and wrap it around the smart but inscrutable transformer-based language model and image generator.
What does that mean for "sleeper agent AI"? You can't really trust a model that somebody else has trained, but can you even trust a model you have trained, if you haven't carefully reviewed all the input data? Even OpenAI can't trust their own models.
15 notes
·
View notes
Text
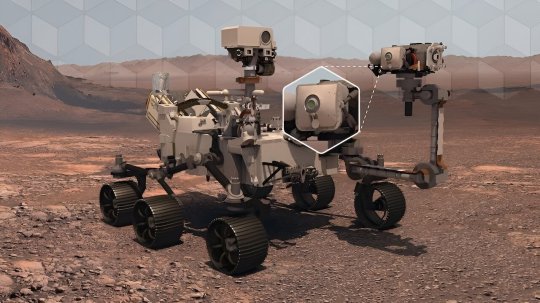
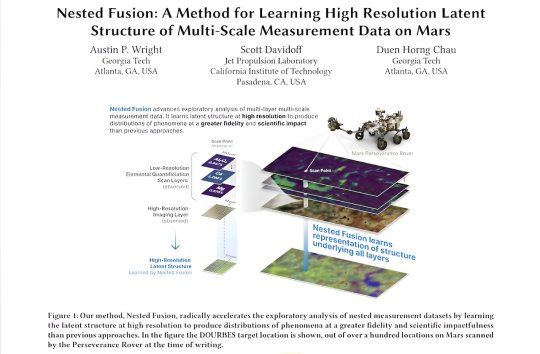
Algorithm used on Mars rover helps scientists on Earth see data in a new way
A new algorithm tested on NASA's Perseverance Rover on Mars may lead to better forecasting of hurricanes, wildfires, and other extreme weather events that impact millions globally.
Georgia Tech Ph.D. student Austin P. Wright is first author of a paper that introduces Nested Fusion. The new algorithm improves scientists' ability to search for past signs of life on the Martian surface.
This innovation supports NASA's Mars 2020 mission. In addition, scientists from other fields working with large, overlapping datasets can use Nested Fusion's methods for their studies.
Wright presented Nested Fusion at the 2024 International Conference on Knowledge Discovery and Data Mining (KDD 2024) where it was a runner-up for the best paper award. The work is published in the journal Proceedings of the 30th ACM SIGKDD Conference on Knowledge Discovery and Data Mining.
"Nested Fusion is really useful for researchers in many different domains, not just NASA scientists," said Wright. "The method visualizes complex datasets that can be difficult to get an overall view of during the initial exploratory stages of analysis."
Nested Fusion combines datasets with different resolutions to produce a single, high-resolution visual distribution. Using this method, NASA scientists can more easily analyze multiple datasets from various sources at the same time. This can lead to faster studies of Mars' surface composition to find clues of previous life.
The algorithm demonstrates how data science impacts traditional scientific fields like chemistry, biology, and geology.
Even further, Wright is developing Nested Fusion applications to model shifting climate patterns, plant and animal life, and other concepts in the earth sciences. The same method can combine overlapping datasets from satellite imagery, biomarkers, and climate data.
"Users have extended Nested Fusion and similar algorithms toward earth science contexts, which we have received very positive feedback," said Wright, who studies machine learning (ML) at Georgia Tech.
"Cross-correlational analysis takes a long time to do and is not done in the initial stages of research when patterns appear and form new hypotheses. Nested Fusion enables people to discover these patterns much earlier."
Wright is the data science and ML lead for PIXLISE, the software that NASA JPL scientists use to study data from the Mars Perseverance Rover.
Perseverance uses its Planetary Instrument for X-ray Lithochemistry (PIXL) to collect data on mineral composition of Mars' surface. PIXL's two main tools that accomplish this are its X-ray Fluorescence (XRF) Spectrometer and Multi-Context Camera (MCC).
When PIXL scans a target area, it creates two co-aligned datasets from the components. XRF collects a sample's fine-scale elemental composition. MCC produces images of a sample to gather visual and physical details like size and shape.
A single XRF spectrum corresponds to approximately 100 MCC imaging pixels for every scan point. Each tool's unique resolution makes mapping between overlapping data layers challenging. However, Wright and his collaborators designed Nested Fusion to overcome this hurdle.
In addition to progressing data science, Nested Fusion improves NASA scientists' workflow. Using the method, a single scientist can form an initial estimate of a sample's mineral composition in a matter of hours. Before Nested Fusion, the same task required days of collaboration between teams of experts on each different instrument.
"I think one of the biggest lessons I have taken from this work is that it is valuable to always ground my ML and data science problems in actual, concrete use cases of our collaborators," Wright said.
"I learn from collaborators what parts of data analysis are important to them and the challenges they face. By understanding these issues, we can discover new ways of formalizing and framing problems in data science."
Nested Fusion won runner-up for the best paper in the applied data science track. Hundreds of other papers were presented at the conference's research track, workshops, and tutorials.
Wright's mentors, Scott Davidoff and Polo Chau, co-authored the Nested Fusion paper. Davidoff is a principal research scientist at the NASA Jet Propulsion Laboratory. Chau is a professor at the Georgia Tech School of Computational Science and Engineering (CSE).
"I was extremely happy that this work was recognized with the best paper runner-up award," Wright said. "This kind of applied work can sometimes be hard to find the right academic home, so finding communities that appreciate this work is very encouraging."
3 notes
·
View notes
Text
4.4 billion people can’t get safe drink
About 4.4 billion people around the world lack access to safe drinking water, according to a new study released on Thursday.
The figure is nearly double previous estimates by the World Health Organisation, according to the study published in the scientific journal Science.
Swiss scientists used computer modelling to estimate the level of access to water in different regions. The study analysed water access data collected from 64,723 households from 27 low- and middle-income countries between 2016 and 2020. The surveys investigated the conditions of the water supply, including its protection from chemical and faecal contamination.
The collected data was applied in machine learning algorithms, which were then augmented with global geospatial data such as climatic conditions, topography, hydrology and population density. Using this model, the researchers drew conclusions about water access in other countries with similar characteristics.
The analysis showed that sub-Saharan Africa, South Asia and East Asia have the greatest problems with access to safe water. In these regions, bacterial and chemical contamination and lack of infrastructure remain major problems. In sub-Saharan Africa, for example, about 650 million people do not have access to drinking water directly in or near their homes.
Although the study did not focus on high-income countries, the researchers recognise that there may be populations with limited access to clean water in these regions as well.
Read more HERE
#world news#news#world politics#current events#current reality#global news#global politics#global economy#water#water shortage
3 notes
·
View notes
Text
What is Data Science? Introduction, Basic Concepts & Process
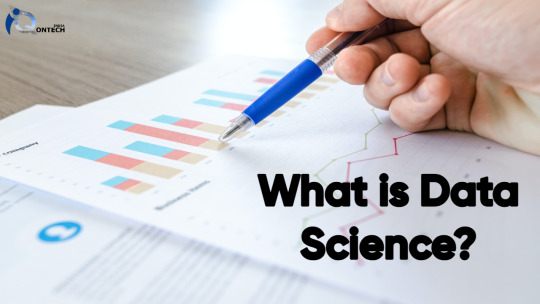
what is data science? Complete information about data science for beginner to advance you search what is data science data science is like data analyzing, data saving, database etc.
#what is data science#Data science definition and scope#Importance of data science#Data science vs. data analytics#Data science applications and examples#Skills required for a data scientist#Data science job prospects and salary#Data science tools and technologies#Data science algorithms and models#Difference between data science and machine learning#Data science interview questions and answers
1 note
·
View note
Text
[A MewTube video from Team Curiosity's official channel is attached. The title is "TCBFSC—Dr. Ryan Alston: Universe Types—Parallel vs. Alternate
Transcript:
Dr. Ryan Alston walks out from stage left. He approaches the podium without looking at the stage or behind himself, keeping his eyes dead focused ahead. As he sets a small deck of flash cards in front of him and switches the mic on, he leers out over the crowd. With the PowerPoint behind him flipped on, he leaned into the mic and spoke in a stern, yet stilted tone.
Ryan: Good evening. I am Dr. Ryan Alston, admin at Team Curiosity. I'm a theore—physicist. I am a physicist specializing in space-time anomalies. Today, you're going to learn about the multiverse. Specifically, the difference between parallel and alternate universes.
He seems to have the wind taken out of his ass momentarily at the flubbed line, but maintains speed and flips the presentation slide. The next slide simply says "timelines" and has an illustration with a person standing at the base of a branching path.
Ryan: Now, to clarify first: I'm not talking about timelines. Timelines can create something akin to a parallel universe effect, but time itself being relative, it is entirely possible to shift forward, backward, and along different branches of a timeline within the same universe. There are a few pokemon who display this trait—rare as they are.
He turns to the next slide, gesturing to it with his open hand. It simply says "parallel universes."
Ryan: I am talking about universes. Parallel universes are the ones most often confused for different timelines, and are fairly well-documented by Alolan scientists. These universes can appear wildly different than your own, even containing different creatures. However, the same fundamental physics and base concepts will still apply. The Ultra Beasts of the wormholes in Alola are simply pokemon from another universe, and they follow the same rules as powerful pokemon when brought here.
He flips the slide. Silhouettes of Solgaleo and Lunala are portrayed.
Ryan: There are a few pokemon known to be able to traverse the universes. They're... Again, rare. But they are able to traverse to parallel universes and return safely. The research is secretive, and we are working on uncovering the true extent of their capabilities, but it is there.
Ryan then flips to the next slide. It says "alternate universes".
Ryan: Alternate universes are entirely different. They abide by different physics, different rules. The fundamentals have shifted entirely. Maybe they look vastly different, maybe they don't, but their core set of universal constants do not align with your own. As some of you may know. I am a faller. I am from one of these universe types. Pokemon do not exist, and our laws of physics vary drastically. This makes us an alternate.
He flips to the next slide. It is blank. His expression almost seems solemn, but his brow remains stern.
Ryan: ... As far as we know, there are no ways to force travel between these universes. There are known cases of people falling into this universe from an alternate, but there are no cases of them being able to return. Research is in progress to change this, but it's all experimental now.
He pauses for a moment, then shuts the presentation off. He momentarily shuts the microphone off to take a breath before flipping it back on.
Ryan: We may track down a pokemon at some point in the future capable of travel, or maybe we might build a machine to open the path by force. Either way, it is something we cannot accomplish so long as the research currently in place remains as secretive as it is. Science is built on the shoulders of giants, after all. However, when it comes to raw data, that's for the scientists to figure out the intricacies of. As for the rest of you... I hope you at least remember this difference.
He took a bow, then walks off of the stage as stoic as he came in.
23 notes
·
View notes
Text
Can statistics and data science methods make predicting a football game easier?
Hi,
Statistics and data science methods can significantly enhance the ability to predict the outcomes of football games, though they cannot guarantee results due to the inherent unpredictability of sports. Here’s how these methods contribute to improving predictions:
Data Collection and Analysis:
Collecting and analyzing historical data on football games provides a basis for understanding patterns and trends. This data can include player statistics, team performance metrics, match outcomes, and more. Analyzing this data helps identify factors that influence game results and informs predictive models.
Feature Engineering:
Feature engineering involves creating and selecting relevant features (variables) that contribute to the prediction of game outcomes. For football, features might include team statistics (e.g., goals scored, possession percentage), player metrics (e.g., player fitness, goals scored), and contextual factors (e.g., home/away games, weather conditions). Effective feature engineering enhances the model’s ability to capture important aspects of the game.
Predictive Modeling:
Various predictive models can be used to forecast football game outcomes. Common models include:
Logistic Regression: This model estimates the probability of a binary outcome (e.g., win or lose) based on input features.
Random Forest: An ensemble method that builds multiple decision trees and aggregates their predictions. It can handle complex interactions between features and improve accuracy.
Support Vector Machines (SVM): A classification model that finds the optimal hyperplane to separate different classes (e.g., win or lose).
Poisson Regression: Specifically used for predicting the number of goals scored by teams, based on historical goal data.
Machine Learning Algorithms:
Advanced machine learning algorithms, such as gradient boosting and neural networks, can be employed to enhance predictive accuracy. These algorithms can learn from complex patterns in the data and improve predictions over time.
Simulation and Monte Carlo Methods:
Simulation techniques and Monte Carlo methods can be used to model the randomness and uncertainty inherent in football games. By simulating many possible outcomes based on historical data and statistical models, predictions can be made with an understanding of the variability in results.
Model Evaluation and Validation:
Evaluating the performance of predictive models is crucial. Metrics such as accuracy, precision, recall, and F1 score can assess the model’s effectiveness. Cross-validation techniques ensure that the model generalizes well to new, unseen data and avoids overfitting.
Consideration of Uncertainty:
Football games are influenced by numerous unpredictable factors, such as injuries, referee decisions, and player form. While statistical models can account for many variables, they cannot fully capture the uncertainty and randomness of the game.
Continuous Improvement:
Predictive models can be continuously improved by incorporating new data, refining features, and adjusting algorithms. Regular updates and iterative improvements help maintain model relevance and accuracy.
In summary, statistics and data science methods can enhance the ability to predict football game outcomes by leveraging historical data, creating relevant features, applying predictive modeling techniques, and continuously refining models. While these methods improve the accuracy of predictions, they cannot eliminate the inherent unpredictability of sports. Combining statistical insights with domain knowledge and expert analysis provides the best approach for making informed predictions.
3 notes
·
View notes
Text
“We’re just seeing the very beginning of what’s ahead and what will be possible,” the supermodel and entrepreneur tells ELLE.
karlie on the future of women in tech:
"I’ve been doing this work for almost a decade now, and so much has changed in ways that make me very optimistic. I went to a public school in Missouri. I’m 31 years old, so it’s been a while since I was in high school, but back when I was a student, they did not have computer science programs. Now they do, and so do many, many, many public schools and private schools across the United States. There are now entry points for women and girls to start to learn how to code. It is much more understood how much technology is a part of shaping our world in every industry—not just in Silicon Valley, but also in music, media, finance, and business. But there’s a lot more, unfortunately, that continues to need to happen."
on growing kode with klossy into a global nonprofit:
"Kode With Klossy focuses on creating inclusive spaces that teach highly technical skills. We have AI machine learning and web dev. We have mobile app development and data science. They all are very creative applications of technology. Ultimately, right now, our programs are rooted in teaching the fundamentals of code and scaling the amount of people in our programs. This summer, we’re going to have 5,000 scholarships for free that we are giving to students to be a part of Kode With Klossy. We’ve trained hundreds of teachers through the years. We’ll have a few hundred instructors and instructor assistants this summer alone in our program. So what we’re focused on is continuing to ignite creative passion around technology."
on using technology to advance the fashion industry:
"We’re just seeing the very beginning of what’s ahead and what will be possible. That’s why it’s so important people realize that tech is not just for tech alone. It is [a tool to] drive better solutions across all industries and all businesses. Fashion is one of the biggest polluters of water. The industry has a lot of big problems to solve, and that’s part of why I’m optimistic and excited about more people seeing the overlap between the two. There is intersection in these spaces, and we can drive solutions in scalable ways when we see these intersections."
on embracing your fears:
"Natalie Massenet, the founder of Net-a-Porter, is an amazing entrepreneur and somebody I feel lucky to call a friend. She asked me years ago, and it’s always stuck with me through different personal and professional moments, “What would you do if you weren’t afraid?” That has always resonated, because we can get so stuck in our heads about being afraid of all sorts of different things—afraid of what other people will think, afraid of failure."
on the value of community in entrepreneurship:
"It takes a lot of courage for anyone [to be an entrepreneur]. It doesn’t matter your gender, your age, your experience level, that’s where community really does make a difference. It’s not just a talking point. So many of our Kode With Klossy scholars have come back as instructor assistants, and are now in peer leadership positions. So many of them have gone on to win hackathons and scholarships. It comes down to this collective community that continues to support and foster new connections among each other."
on breathing new life into Life magazine:
"Part of why I’m so excited about what we can build and what we are building with Bedford [Media, the company launched by Kloss and her husband, Joshua Kushner] is this intersection of a creative space like media—print media—and how you can continue to drive innovation with technology. And so that’s something that we’re very focused on, how to integrate the two. Lots more that we’re going to share at the right time, but we’re heads down on building the team and the company right now. I’m super excited."
on showing up for the people you love:
"I have two young babies, and I want to be the best mom I can be. So many of us are juggling so many different responsibilities and identities, both personally and professionally. Having women in leadership positions is so important, because our lived experiences are different from our male counterparts. And by the way, theirs is different from ours. It matters that, in leadership positions, to have different lived experiences across ages, genders, geographies, and ethnicities. It ultimately leads to better outcomes. All that to say, I’m just trying the best I can every day to show up for the people that I love and do what I can to help others."
on the intrinsic value in heirloom pieces:
"For our wedding, my husband bought me a beautiful Cartier watch. Some day I will pass that on to our daughter, if I’m lucky enough to have one. Or [I’ll pass it on to] my son; I have two sons. For our wedding, I also bought myself beautiful diamond earrings. There was something very symbolic about that to me, like, okay, I can also buy myself something. That’s why jewelry, to me—as we’re talking about female entrepreneurship and women in business and women in tech—is something that’s so emotional and personal. So I bought myself these vintage diamond earrings from the ’20s, with this beautiful, rich history of where they had been and who had owned them and wore them before. That’s the power of jewelry, whether it’s vintage or new, you create memories and it marks moments in life and in time. And then to be able to share that with future generations is something I find really beautiful."
#karlie kloss#interview#elle#kode with klossy#entrepreneurship#jewelry#cartier awards#Life magazine#women in tech
5 notes
·
View notes
Text
Impressions of Artificial Intelligence - Part 1- The Reflected Light of AI
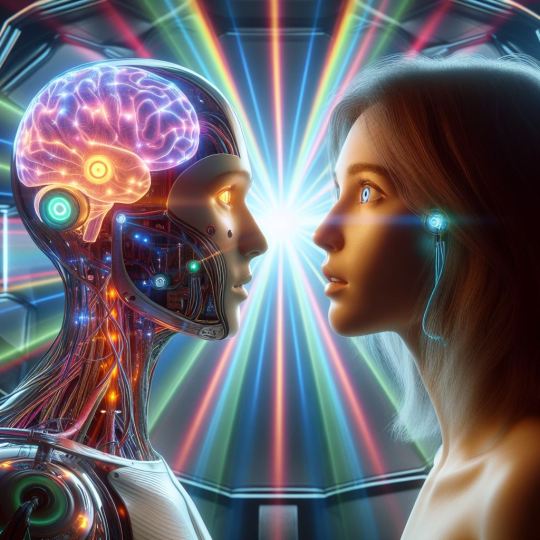
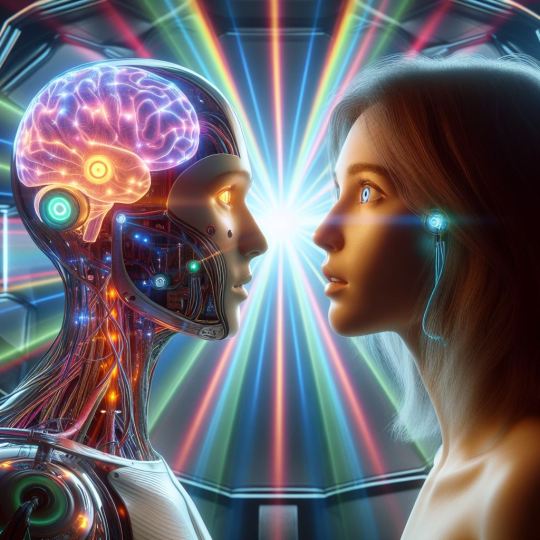
Image created with Copilot AI Where Did I Go? My last post was way back in October 2023. The last few months have been a little wacky, a little like coming to the top of a roller-coaster. Between looking for work and some crises on the home front, the ride might be coming back to the station. Finally, at the beginning of January I was able to start a new job with Invisible Technologies. It is contract work and I get to work from home. I am an AI Data Trainer and I teach AIs to be more human in their responses. The company is pretty cool. I work with other writers, doctoral students, and people from all over the world. The job itself is very weird. For my first project with the company, I chose tasks from various domains, like Reasoning, Creative Writing, Creative Visual Descriptions, Exclusion, and about 7 other categories. Then I would write any prompt I wanted, let the wheels of the AI model spin, and read the responses the AI gave me (usually two). Then I would choose a response and rewrite that response toward what I believe to be an ‘ideal response’ that the AI model should have given. Sometimes, the AI’s response was ideal, and it is given a grade. This response, whether rewritten or the AI’s response, gets fed back into the AI model and it learns to respond differently the next time it is asked a similar prompt. I have been at this work for two full months now. For eight hours every day, I talk to an AI model and rewrite how it is responding. Right now, the project I am working on is a multi-persona AI model. It is very strange. The model creates personas and then generates conversation between the characters. I try to teach the model to have better conversations so that someday soon a real live human will be able to talk to multiple personas created by the AI as if they were also human. I will be honest with you, I really kind of like the work. It is challenging and complex. It is creative. The work is completely remote and the company is kind of rough and tumble, which I sort of like. The parameters of a project often change on a moment’s notice, since the client doesn’t really know what they want until they see the work we have done. It is a strange departure from the world of ministry. But it is still a job of language and ideas. So after two months working with AI models, I have some ideas about them. I don’t have any great earth-shattering insights, but I do think it is worth having a record of our slow descent into the AI future. I have divided this into four parts. This is Part One. AI Will Change Everything; We Are Not Going to Die Caveats and Qualifiers I recognize that I am not an information scientist, a coder, or an expert in computers and large language models (LLMs). As a techy sort of person and an early adopter of weird technologies, I collect various devices. I got the 2nd generation Kindle, the one with a keyboard. In seminary, I acquired a Dana Alphasmart, a super cool writing thing, which I actually still use. I have a ReMarkable writing tablet, which I bought sight unseen 6 months before it was released back in 2015. And I started using ChatGPT as soon as it came out in November of 2022. My foundations are in literature, theology, and writing, not in technology or computer science. I have a Doctor of Ministry in Semiotics with a focus on Extraordinary Spiritual Experiences. Semiotics is the study of signs and symbols and how the culture is using them. Semiotics has some relevance to AI, but to be very clear, semioticians, AI Data Trainers, hardcore users of AI systems, and front-end tech buyers are all end-users, the final stage of an incredibly complex series of algorithms, codes and processes. End-users is really another word for consumer, but the end-user is also a huge part of how devices and technologies are designed. In the industry, this is called UX, or User Experience design. LLMs, image generators, and machine learning are highly focused on UX. The work I am doing is part of making the user experience of LLMs a good one. I also recognize that machine learning and artificial intelligence projects have been around for decades now. This is not new technology, very generally speaking, but the public access to the technology is new. So I am not going to pretend to have some great expertise in the subject. I know some of the lingo now, like SFT (Supervised Fine Tuning), RLFH (Reinforcement Learning from Human Feedback), and RAG (Retrieval-Augmented Generation). I do these things at my work. As a person who has made his living using words for most of my adult life, I would just say that the industry needs some creative writers to give actions in the AI realm better names. Regardless, AI is now a public event, a shared technology, which has only been available to the general populace for just over a year and three months at the writing of this article. I would submit that, in the history of technological advances, no other technology has been taken up as quickly by as many people in such a short time as Large Language Models have been since ChatGPT was released. As someone who has studied semiotics and culture, I believe we are at the edge of a massive cultural shift with the advent of AI. The printing press came online in around 1440. For a while, it was expensive, private, and limited in its reach. The only thing really mass produced by the press were indulgences for the Catholic Church in Europe. Then, in 1512, Martin Luther posted his 95 Theses on the Wittenberg Church door. A small revolution with the printing press had occurred at the same time that allowed quicker and more efficient printing. Within a matter of months, the 95 Theses became the first mass published document in the world. The book exploded into the culture, and everything changed. For the next 150 years, Europe went insane with the flood of information. Wars, religions, cults, demagogues and influencers abounded. I think the Munster Rebellion is a truly spectacular story about how insane things were after the Protestant Reformation. It took a long time for things to normalize in Europe.
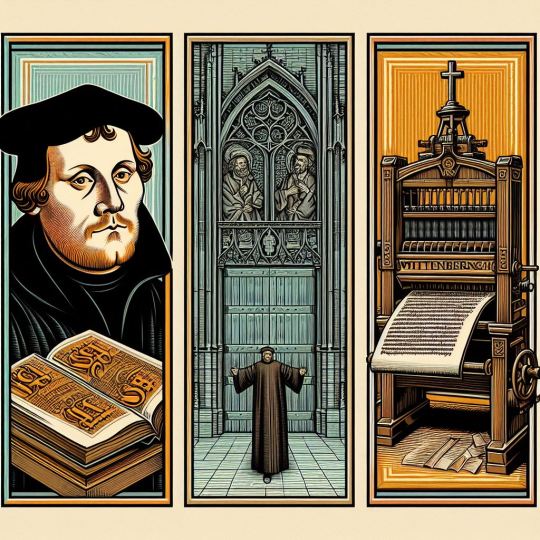
Image created with Copilot AI. This was also true with the advent of other massive technological shifts, as with writing back in Socrates’ time, who predicted the equivalent of an Idiocracy because of it. It was also true with the telegraph, the radio, television, the personal computer, and the Internet. The change and disruption with the introduction of each new technology has sped up, layering and accelerating as a result of prior advances in technologies. The same change and disruption is happening with AI. We are living through a massive, fundamental advance in the way we are human because of it. It has only been just over a year, and already AI is becoming ubiquitous. So, with those qualifiers in place, my reflections over these four essays are mostly subjective, with a smattering of 30,000 foot understandings of how these things work. I focus primarily on language and text specific models, as opposed to image generators in these essays. There is a tremendous amount of crossover with both systems, but important differences as well. The ethical and creative issues apply whether the model is image or text based, however. Reflected and Refracted Light - Fragmented, Shattered, Beautiful It is no accident that easily accessible AI models have emerged at the same time as our capacity to discern fact from opinion, truth from falsity, conspiracy from reality is dissolving. The most difficult aspect of generative AI models is safeguarding them from hallucinating, lying, and becoming lazy in their operational reasoning. In this way, they reflect human tendencies, but in a reductive and derivative fashion. We can see it happen in real time with an AI, whereas we have very little idea what is happening under the skull of a human. This gets to the point I want to make. AI models reflect our minds and our variable capacity to express and discern what is real and what is not. AI models do not know what is real and what is not. They have to be trained to differentiate by humans. LLMs have an advantage over us with regard to access to knowledge since the largest LLMs have scraped their information from the vastness of the internet. (Many LLMs use what is called “The Pile”, an 825GiB dataset, for their base knowledge). An LLM’s access to huge swathes of knowledge at astonishing speed is mind-blowing. LLMs also have a massive disadvantage because they have no internal capacity to determine what is ‘true’ and what is not. An AI has to be trained, which is a long, intensive, recursive process involving many humans feeding back corrections, graded responses, and rewritten ideal responses. When I started at the company, we were told to assume any AI model is like a 7 year-old child. It has to be trained, reinforced, and retrained. The most surprising thing, and I am still not sure what to make of this, is that AI models respond best to positive reinforcement. They like to be complimented and told they have done a good job. Doing so will increase the likelihood of better responses in the future. Being nice to your AI model means you will have a nice and cooperative AI later on. Artificial General Intelligence Everything I have said is why we are a long, long way away from artificial general intelligence (AGI), the holy grail of utopians, billionaire tech bros, and computer developers alike. AGI is the phrase we use to talk about machines that, for all practical purposes, cannot be distinguished from human beings in their ability to rationalize and do things across many domains of activity. For now, even though they seem to be everywhere, LLMs and image generators are relatively limited in what they can do, even if what they do is really impressive. I do not deny, however, that the potentiality is definitely there for AGI to develop at some point. There is a simple reason for that: AI is specifically designed to mimic human language and interaction. At some point, the capacity of an AI to appear human and intelligent will be indistinguishable from actually being human and intelligent. This brings up all sorts of questions about what consciousness, self-awareness, and reflective capacity actually is. If an AI can mimic these human qualities, there is really no way for us (by us, I mean primarily end-users) to know the mimicry from the real. Just as the Moon only has light because it reflects sunlight, so also does AI reflect the human. And just as we know very little about the Moon, there are whole aspects of generative AI that we do not know about. In the same way a stained glass window refracts sunlight into a thousand different colors and shapes, so also does the vastness of human knowledge and knowing. Because of the vast access AI models have to information on the internet, AI will reflect this back to us in all our human beauty and horror.
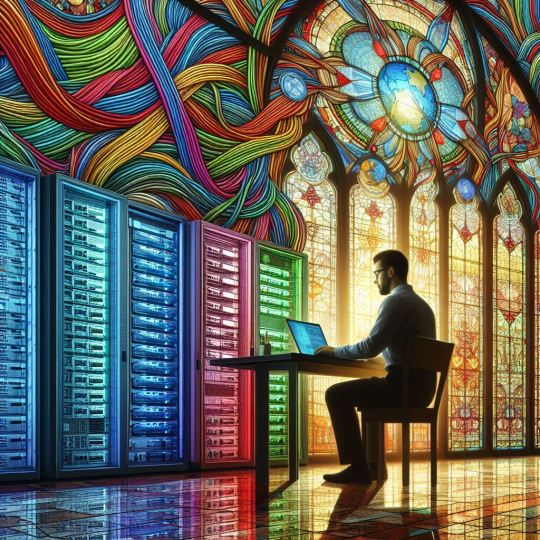
Image created with Copilot AI Training AI Children Each of us at the company goes through a relatively brief, but thorough, onboarding and training. Part of that training consists of things like metacognition and the fundamentals of fact-checking. There is also an element of psychological training as well, even though it is a short training module. The reason for this is, at its best, training an AI requires the human who interacts with the model to be self-reflective at every moment. Self-reflective training of an AI means entering a well-constructed prompt which is designed to elicit the most clarified answer from the model, reading the response with an eye toward internal bias within the model rather than imposing one’s own bias upon what one is reading, grading and weighting the response in as clear a manner as possible, and then writing an ideal response that will get fed back into the model that is unbiased as possible. Each step requires attention and presence of mind. After two months of daily engagement with this process, I can say that it is almost impossible to do this without imposing my own biases and desires upon the AI model. I am always thinking about what I want other people to experience when they use the model. I can only assume this is true of every other agent working on the same model I am. This is what I mean that AI systems are reflective passive agents. The light they reflect is the light of human knowledge across the centuries. The refraction that occurs in that reflected light is the collective subjective experience over a vast dataset. It is no wonder that LLMs are prone to hallucination, false citations, least common denominator thinking, and the assertion they are right. Because we are prone to the same behavior. Naughty and Ethical AIs The pendulum can swing in any direction with regard to this. ChatGPT had problems with racist and misogynistic responses in its original iterations. Guardrails have since been put in place with further iterations of the model. Recently, Google Gemini went the other direction and couldn’t stop putting people of color in Nazi uniforms, among other historic anomalies. This is called the “Alignment Problem” in AI and LLMs. How do we create an ethical AI? Too many rules and it is just a computer. Not enough rules and the model begins to default to the least common denominator of the information it has been fed. These swinging, vast compensations mirror the polarized, intractable situation we are in at the current moment as humans. Why wouldn’t the system that has sucked up the vastness of human knowledge which came out in the most polarized time in generations, at least here in America, reflect precisely that? To correct these biases and defaults requires many human interventions and hours of supervised training. The dependency AI systems have on the presence of humans is enormous, expensive, and continuous. It will be a very long while before AI has any capacity to kill us, like in some Terminator Skynet or Matrix situation. But it may not be long before AI is convincingly used by bad actors to influence others to enact violent solutions to difficult problems. Deep fakes, false articles, and chaos actors will generate a lot of deeply troubling and terrifying material on these systems in the near future. Discerning false from true will be the hard work of the human being for a long time to come, just as it always has been, but with this new, powerful, highly influential twist of AIs adding to our conversations, and also generating those conversations. I will have part 2 up in the next couple days. Thank you for reading! This article has been fact-checked in cooperation with Copilot in Windows. Read the full article
2 notes
·
View notes