#Data science algorithms and models
Explore tagged Tumblr posts
Text
What is Data Science? Introduction, Basic Concepts & Process
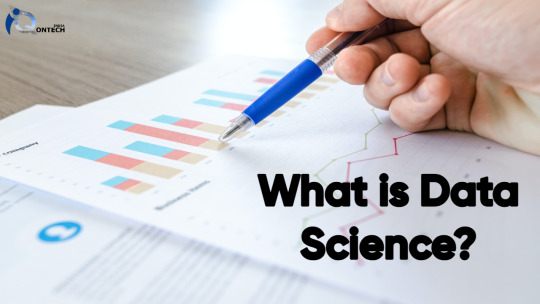
what is data science? Complete information about data science for beginner to advance you search what is data science data science is like data analyzing, data saving, database etc.
#what is data science#Data science definition and scope#Importance of data science#Data science vs. data analytics#Data science applications and examples#Skills required for a data scientist#Data science job prospects and salary#Data science tools and technologies#Data science algorithms and models#Difference between data science and machine learning#Data science interview questions and answers
1 note
·
View note
Text
User-friendly system can help developers build more efficient simulations and AI models
New Post has been published on https://thedigitalinsider.com/user-friendly-system-can-help-developers-build-more-efficient-simulations-and-ai-models/
User-friendly system can help developers build more efficient simulations and AI models
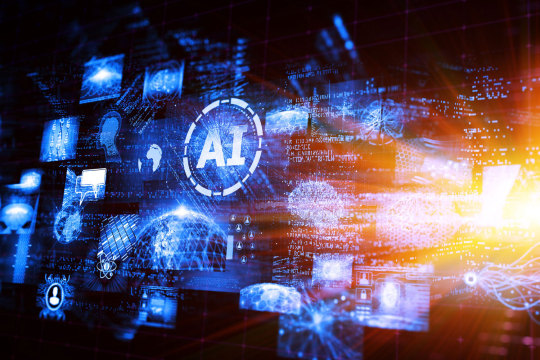
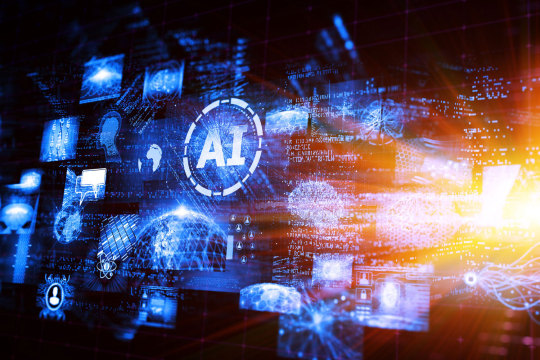
The neural network artificial intelligence models used in applications like medical image processing and speech recognition perform operations on hugely complex data structures that require an enormous amount of computation to process. This is one reason deep-learning models consume so much energy.
To improve the efficiency of AI models, MIT researchers created an automated system that enables developers of deep learning algorithms to simultaneously take advantage of two types of data redundancy. This reduces the amount of computation, bandwidth, and memory storage needed for machine learning operations.
Existing techniques for optimizing algorithms can be cumbersome and typically only allow developers to capitalize on either sparsity or symmetry — two different types of redundancy that exist in deep learning data structures.
By enabling a developer to build an algorithm from scratch that takes advantage of both redundancies at once, the MIT researchers’ approach boosted the speed of computations by nearly 30 times in some experiments.
Because the system utilizes a user-friendly programming language, it could optimize machine-learning algorithms for a wide range of applications. The system could also help scientists who are not experts in deep learning but want to improve the efficiency of AI algorithms they use to process data. In addition, the system could have applications in scientific computing.
“For a long time, capturing these data redundancies has required a lot of implementation effort. Instead, a scientist can tell our system what they would like to compute in a more abstract way, without telling the system exactly how to compute it,” says Willow Ahrens, an MIT postdoc and co-author of a paper on the system, which will be presented at the International Symposium on Code Generation and Optimization.
She is joined on the paper by lead author Radha Patel ’23, SM ’24 and senior author Saman Amarasinghe, a professor in the Department of Electrical Engineering and Computer Science (EECS) and a principal researcher in the Computer Science and Artificial Intelligence Laboratory (CSAIL).
Cutting out computation
In machine learning, data are often represented and manipulated as multidimensional arrays known as tensors. A tensor is like a matrix, which is a rectangular array of values arranged on two axes, rows and columns. But unlike a two-dimensional matrix, a tensor can have many dimensions, or axes, making tensors more difficult to manipulate.
Deep-learning models perform operations on tensors using repeated matrix multiplication and addition — this process is how neural networks learn complex patterns in data. The sheer volume of calculations that must be performed on these multidimensional data structures requires an enormous amount of computation and energy.
But because of the way data in tensors are arranged, engineers can often boost the speed of a neural network by cutting out redundant computations.
For instance, if a tensor represents user review data from an e-commerce site, since not every user reviewed every product, most values in that tensor are likely zero. This type of data redundancy is called sparsity. A model can save time and computation by only storing and operating on non-zero values.
In addition, sometimes a tensor is symmetric, which means the top half and bottom half of the data structure are equal. In this case, the model only needs to operate on one half, reducing the amount of computation. This type of data redundancy is called symmetry.
“But when you try to capture both of these optimizations, the situation becomes quite complex,” Ahrens says.
To simplify the process, she and her collaborators built a new compiler, which is a computer program that translates complex code into a simpler language that can be processed by a machine. Their compiler, called SySTeC, can optimize computations by automatically taking advantage of both sparsity and symmetry in tensors.
They began the process of building SySTeC by identifying three key optimizations they can perform using symmetry.
First, if the algorithm’s output tensor is symmetric, then it only needs to compute one half of it. Second, if the input tensor is symmetric, then algorithm only needs to read one half of it. Finally, if intermediate results of tensor operations are symmetric, the algorithm can skip redundant computations.
Simultaneous optimizations
To use SySTeC, a developer inputs their program and the system automatically optimizes their code for all three types of symmetry. Then the second phase of SySTeC performs additional transformations to only store non-zero data values, optimizing the program for sparsity.
In the end, SySTeC generates ready-to-use code.
“In this way, we get the benefits of both optimizations. And the interesting thing about symmetry is, as your tensor has more dimensions, you can get even more savings on computation,” Ahrens says.
The researchers demonstrated speedups of nearly a factor of 30 with code generated automatically by SySTeC.
Because the system is automated, it could be especially useful in situations where a scientist wants to process data using an algorithm they are writing from scratch.
In the future, the researchers want to integrate SySTeC into existing sparse tensor compiler systems to create a seamless interface for users. In addition, they would like to use it to optimize code for more complicated programs.
This work is funded, in part, by Intel, the National Science Foundation, the Defense Advanced Research Projects Agency, and the Department of Energy.
#ai#AI models#algorithm#Algorithms#applications#approach#Arrays#artificial#Artificial Intelligence#author#Building#Capture#code#code generation#columns#Commerce#computation#computer#Computer Science#Computer Science and Artificial Intelligence Laboratory (CSAIL)#Computer science and technology#computing#cutting#data#Data Structures#Deep Learning#defense#Defense Advanced Research Projects Agency (DARPA)#Department of Energy (DoE)#Developer
0 notes
Text
0 notes
Text
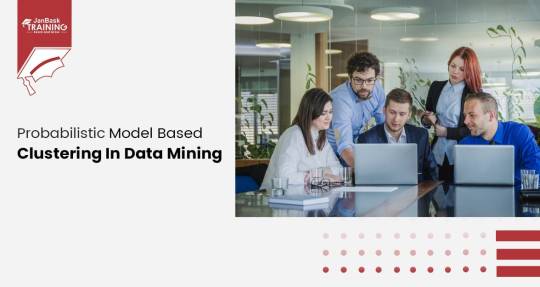
Probabilistic model-based clustering is an excellent approach to understanding the trends that may be inferred from data and making future forecasts. The relevance of model based clustering, one of the first subjects taught in data science, cannot be overstated. These models serve as the foundation for machine learning models to comprehend popular trends and their behavior. You can also learn about neural network guides and python for data science if you are interested in further career prospects of data science.
#Clustering#Data Mining#Machine Learning#Probabilistic Models#Model-Based Clustering#Gaussian Mixture Models#Expectation-Maximization Algorithm#Unsupervised Learning#Data Analysis#Pattern Recognition#Data Science#Bayesian Methods#Hidden Markov Models#Statistical Learning#Cluster Analysis.
0 notes
Photo
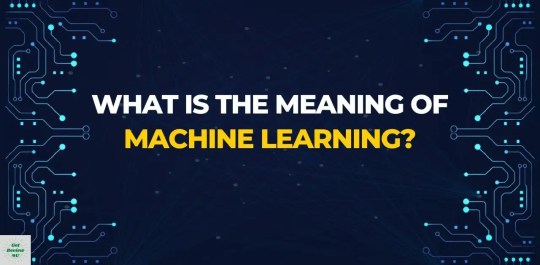
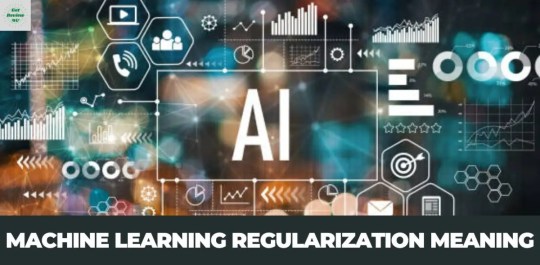
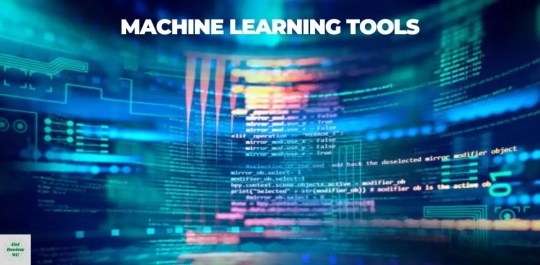
(via All you need to know about Machine Learning | meaning, tool, technique, math, algorithm, AI, accuracy, …etc)
#machine learning algorithm meaning#how does machine learning#regularization machine learning meaning#mean in machine learning#machine learning as a service#what is machine learning model#statistics for machine learning#tools of machine learning#machine learning tool#machine learning technique#machine learning math#artificial intelligence and machine learning meaning#ai machine learning meaning#what is machine learning in data science#types of machine learning algorithms#3 types of machine learning#different types of machine learning algorithms#regularization machine learning#machine learning regularization#regularization machine learning definition
0 notes
Text
Arvind Narayanan, a computer science professor at Princeton University, is best known for calling out the hype surrounding artificial intelligence in his Substack, AI Snake Oil, written with PhD candidate Sayash Kapoor. The two authors recently released a book based on their popular newsletter about AI’s shortcomings.
But don’t get it twisted—they aren’t against using new technology. “It's easy to misconstrue our message as saying that all of AI is harmful or dubious,” Narayanan says. He makes clear, during a conversation with WIRED, that his rebuke is not aimed at the software per say, but rather the culprits who continue to spread misleading claims about artificial intelligence.
In AI Snake Oil, those guilty of perpetuating the current hype cycle are divided into three core groups: the companies selling AI, researchers studying AI, and journalists covering AI.
Hype Super-Spreaders
Companies claiming to predict the future using algorithms are positioned as potentially the most fraudulent. “When predictive AI systems are deployed, the first people they harm are often minorities and those already in poverty,” Narayanan and Kapoor write in the book. For example, an algorithm previously used in the Netherlands by a local government to predict who may commit welfare fraud wrongly targeted women and immigrants who didn’t speak Dutch.
The authors turn a skeptical eye as well toward companies mainly focused on existential risks, like artificial general intelligence, the concept of a super-powerful algorithm better than humans at performing labor. Though, they don’t scoff at the idea of AGI. “When I decided to become a computer scientist, the ability to contribute to AGI was a big part of my own identity and motivation,” says Narayanan. The misalignment comes from companies prioritizing long-term risk factors above the impact AI tools have on people right now, a common refrain I’ve heard from researchers.
Much of the hype and misunderstandings can also be blamed on shoddy, non-reproducible research, the authors claim. “We found that in a large number of fields, the issue of data leakage leads to overoptimistic claims about how well AI works,” says Kapoor. Data leakage is essentially when AI is tested using part of the model’s training data—similar to handing out the answers to students before conducting an exam.
While academics are portrayed in AI Snake Oil as making “textbook errors,” journalists are more maliciously motivated and knowingly in the wrong, according to the Princeton researchers: “Many articles are just reworded press releases laundered as news.” Reporters who sidestep honest reporting in favor of maintaining their relationships with big tech companies and protecting their access to the companies’ executives are noted as especially toxic.
I think the criticisms about access journalism are fair. In retrospect, I could have asked tougher or more savvy questions during some interviews with the stakeholders at the most important companies in AI. But the authors might be oversimplifying the matter here. The fact that big AI companies let me in the door doesn’t prevent me from writing skeptical articles about their technology, or working on investigative pieces I know will piss them off. (Yes, even if they make business deals, like OpenAI did, with the parent company of WIRED.)
And sensational news stories can be misleading about AI’s true capabilities. Narayanan and Kapoor highlight New York Times columnist Kevin Roose’s 2023 chatbot transcript interacting with Microsoft's tool headlined “Bing’s A.I. Chat: ‘I Want to Be Alive. 😈’” as an example of journalists sowing public confusion about sentient algorithms. “Roose was one of the people who wrote these articles,” says Kapoor. “But I think when you see headline after headline that's talking about chatbots wanting to come to life, it can be pretty impactful on the public psyche.” Kapoor mentions the ELIZA chatbot from the 1960s, whose users quickly anthropomorphized a crude AI tool, as a prime example of the lasting urge to project human qualities onto mere algorithms.
Roose declined to comment when reached via email and instead pointed me to a passage from his related column, published separately from the extensive chatbot transcript, where he explicitly states that he knows the AI is not sentient. The introduction to his chatbot transcript focuses on “its secret desire to be human” as well as “thoughts about its creators,” and the comment section is strewn with readers anxious about the chatbot’s power.
Images accompanying news articles are also called into question in AI Snake Oil. Publications often use clichéd visual metaphors, like photos of robots, at the top of a story to represent artificial intelligence features. Another common trope, an illustration of an altered human brain brimming with computer circuitry used to represent the AI’s neural network, irritates the authors. “We're not huge fans of circuit brain,” says Narayanan. “I think that metaphor is so problematic. It just comes out of this idea that intelligence is all about computation.” He suggests images of AI chips or graphics processing units should be used to visually represent reported pieces about artificial intelligence.
Education Is All You Need
The adamant admonishment of the AI hype cycle comes from the authors’ belief that large language models will actually continue to have a significant influence on society and should be discussed with more accuracy. “It's hard to overstate the impact LLMs might have in the next few decades,” says Kapoor. Even if an AI bubble does eventually pop, I agree that aspects of generative tools will be sticky enough to stay around in some form. And the proliferation of generative AI tools, which developers are currently pushing out to the public through smartphone apps and even formatting devices around it, just heightens the necessity for better education on what AI even is and its limitations.
The first step to understanding AI better is coming to terms with the vagueness of the term, which flattens an array of tools and areas of research, like natural language processing, into a tidy, marketable package. AI Snake Oil divides artificial intelligence into two subcategories: predictive AI, which uses data to assess future outcomes; and generative AI, which crafts probable answers to prompts based on past data.
It’s worth it for anyone who encounters AI tools, willingly or not, to spend at least a little time trying to better grasp key concepts, like machine learning and neural networks, to further demystify the technology and inoculate themselves from the bombardment of AI hype.
During my time covering AI for the past two years, I’ve learned that even if readers grasp a few of the limitations of generative tools, like inaccurate outputs or biased answers, many people are still hazy about all of its weaknesses. For example, in the upcoming season of AI Unlocked, my newsletter designed to help readers experiment with AI and understand it better, we included a whole lesson dedicated to examining whether ChatGPT can be trusted to dispense medical advice based on questions submitted by readers. (And whether it will keep your prompts about that weird toenail fungus private.)
A user may approach the AI’s outputs with more skepticism when they have a better understanding of where the model’s training data came from—often the depths of the internet or Reddit threads—and it may hamper their misplaced trust in the software.
Narayanan believes so strongly in the importance of quality education that he began teaching his children about the benefits and downsides of AI at a very young age. “I think it should start from elementary school,” he says. “As a parent, but also based on my understanding of the research, my approach to this is very tech-forward.”
Generative AI may now be able to write half-decent emails and help you communicate sometimes, but only well-informed humans have the power to correct breakdowns in understanding around this technology and craft a more accurate narrative moving forward.
38 notes
·
View notes
Text
Can Math Predict the Future? Exploring Mathematical Forecasting
The idea of predicting the future using mathematics has fascinated humans for centuries. From forecasting weather patterns to predicting economic trends and even understanding social dynamics, math provides the framework for making sense of the world and anticipating what comes next.
1. Weather Forecasting: A Battle with Chaos
One of the most obvious examples of mathematical forecasting is weather prediction. Meteorologists rely on complex differential equations to model atmospheric conditions. These models, based on physical principles like fluid dynamics and thermodynamics, simulate the behavior of the atmosphere. But here’s the kicker—weather systems are chaotic. This means that tiny changes in the initial conditions can lead to vastly different outcomes, a phenomenon famously described by Edward Lorenz in the 1960s.
Lorenz’s work led to the development of chaos theory, which showed that deterministic systems (those governed by fixed laws) could still be unpredictable due to their sensitivity to initial conditions. This is why forecasts beyond a few days are often inaccurate: small errors compound exponentially, making long-term weather predictions difficult. Still, thanks to sophisticated computing and more accurate data, we can predict weather patterns with reasonable accuracy for about a week, and even then, the models rely heavily on continuous updates and refinement.
2. Exponential Growth and the Spread of Disease
In the world of epidemiology, mathematical models are essential for understanding the spread of infectious diseases. SIR models (Susceptible-Infected-Recovered) use ordinary differential equations to model how diseases spread through populations. These models take into account the rate of infection and recovery to predict the future trajectory of a disease.
The exponential nature of disease spread—especially in the early stages—means that without intervention, the number of cases can explode. For example, during the early stages of the COVID-19 pandemic, exponential growth was apparent in the number of cases. The key to controlling such outbreaks often lies in early intervention—social distancing, vaccinations, or quarantine measures.
Exponential growth isn’t limited to disease, either. It applies to things like population growth and financial investments. The classic compound interest formula,
A = P \left(1 + \frac{r}{n} \right)^{nt}
demonstrates how small, consistent growth over time can lead to huge, seemingly unstoppable increases in value.
3. Predictive Algorithms: Making Sense of Big Data
Data science is at the cutting edge of forecasting today. Algorithms powered by big data are now able to predict everything from consumer behavior to stock market fluctuations and political elections. By identifying patterns in large datasets, these algorithms can forecast outcomes that were previously unpredictable.
For example, Amazon uses predictive models to forecast demand for products, ensuring they have inventory ready for expected sales spikes. Similarly, Netflix uses recommendation systems to predict what shows or movies you’ll watch next based on your previous choices.
Despite all the advances in predictive analytics, uncertainty remains a fundamental part of the picture. Even the best models can't account for random events (think of a sudden market crash or an unexpected global pandemic). As a result, forecasting is always a balance of probability and uncertainty.
4. The Limits of Mathematical Predictions: Enter Uncertainty
At the core of any discussion about forecasting is the recognition that math cannot predict everything. Whether it’s the weather, the stock market, or even the future of human civilization, uncertainty is a constant. Gödel’s Incompleteness Theorem reminds us that even within a well-defined system, there are true statements that cannot be proven. Similarly, Heisenberg’s Uncertainty Principle in quantum mechanics tells us that there’s a limit to how precisely we can know both the position and momentum of particles—unpredictability is embedded in the fabric of reality.
Thus, while math allows us to make educated guesses and create models, true prediction—especially in complex systems—is often limited by chaos, uncertainty, and the sheer complexity of the universe.
#mathematics#math#mathematician#mathblr#mathposting#calculus#geometry#algebra#numbertheory#mathart#STEM#science#academia#Academic Life#math academia#math academics#math is beautiful#math graphs#math chaos#math elegance#education#technology#statistics#data analytics#math quotes#math is fun#math student#STEM student#math education#math community
11 notes
·
View notes
Text
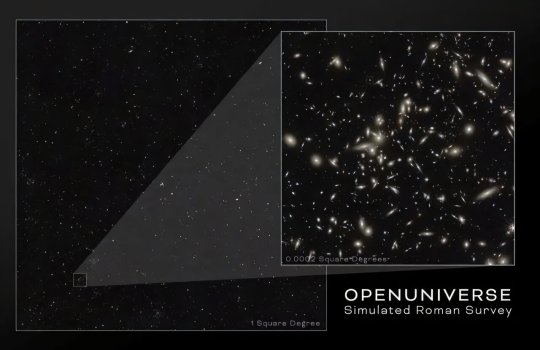
Simulated universe previews panoramas from NASA's Roman Telescope
Astronomers have released a set of more than a million simulated images showcasing the cosmos as NASA's upcoming Nancy Grace Roman Space Telescope will see it. This preview will help scientists explore Roman's myriad science goals.
"We used a supercomputer to create a synthetic universe and simulated billions of years of evolution, tracing every photon's path all the way from each cosmic object to Roman's detectors," said Michael Troxel, an associate professor of physics at Duke University in Durham, North Carolina, who led the simulation campaign. "This is the largest, deepest, most realistic synthetic survey of a mock universe available today."
The project, called OpenUniverse, relied on the now-retired Theta supercomputer at the DOE's (Department of Energy's) Argonne National Laboratory in Illinois. In just nine days, the supercomputer accomplished a process that would take over 6,000 years on a typical computer.
In addition to Roman, the 400-terabyte dataset will also preview observations from the Vera C. Rubin Observatory, and approximate simulations from ESA's (the European Space Agency's) Euclid mission, which has NASA contributions. The Roman data is available now here, and the Rubin and Euclid data will soon follow.
The team used the most sophisticated modeling of the universe's underlying physics available and fed in information from existing galaxy catalogs and the performance of the telescopes' instruments. The resulting simulated images span 70 square degrees, equivalent to an area of sky covered by more than 300 full moons. In addition to covering a broad area, it also covers a large span of time—more than 12 billion years.
The project's immense space-time coverage shows scientists how the telescopes will help them explore some of the biggest cosmic mysteries. They will be able to study how dark energy (the mysterious force thought to be accelerating the universe's expansion) and dark matter (invisible matter, seen only through its gravitational influence on regular matter) shape the cosmos and affect its fate.
Scientists will get closer to understanding dark matter by studying its gravitational effects on visible matter. By studying the simulation's 100 million synthetic galaxies, they will see how galaxies and galaxy clusters evolved over eons.
Repeated mock observations of a particular slice of the universe enabled the team to stitch together movies that unveil exploding stars crackling across the synthetic cosmos like fireworks. These starbursts allow scientists to map the expansion of the simulated universe.
Scientists are now using OpenUniverse data as a testbed for creating an alert system to notify astronomers when Roman sees such phenomena. The system will flag these events and track the light they generate so astronomers can study them.
That's critical because Roman will send back far too much data for scientists to comb through themselves. Teams are developing machine-learning algorithms to determine how best to filter through all the data to find and differentiate cosmic phenomena, like various types of exploding stars.
"Most of the difficulty is in figuring out whether what you saw was a special type of supernova that we can use to map how the universe is expanding, or something that is almost identical but useless for that goal," said Alina Kiessling, a research scientist at NASA's Jet Propulsion Laboratory (JPL) in Southern California and the principal investigator of OpenUniverse.
While Euclid is already actively scanning the cosmos, Rubin is set to begin operations late this year and Roman will launch by May 2027. Scientists can use the synthetic images to plan the upcoming telescopes' observations and prepare to handle their data. This prep time is crucial because of the flood of data these telescopes will provide.
In terms of data volume, "Roman is going to blow away everything that's been done from space in infrared and optical wavelengths before," Troxel said. "For one of Roman's surveys, it will take less than a year to do observations that would take the Hubble or James Webb space telescopes around a thousand years. The sheer number of objects Roman will sharply image will be transformative."
"We can expect an incredible array of exciting, potentially Nobel Prize-winning science to stem from Roman's observations," Kiessling said. "The mission will do things like unveil how the universe expanded over time, make 3D maps of galaxies and galaxy clusters, reveal new details about star formation and evolution—all things we simulated. So now we get to practice on the synthetic data so we can get right to the science when real observations begin."
Astronomers will continue using the simulations after Roman launches for a cosmic game of spot the differences. Comparing real observations with synthetic ones will help scientists see how accurately their simulation predicts reality. Any discrepancies could hint at different physics at play in the universe than expected.
"If we see something that doesn't quite agree with the standard model of cosmology, it will be extremely important to confirm that we're really seeing new physics and not just misunderstanding something in the data," said Katrin Heitmann, a cosmologist and deputy director of Argonne's High Energy Physics division who managed the project's supercomputer time. "Simulations are super useful for figuring that out."
TOP IMAGE: Each tiny dot in the image at left is a galaxy simulated by the OpenUniverse campaign. The one-square-degree image offers a small window into the full simulation area, which is about 70 square degrees (equivalent to an area of sky covered by more than 300 full moons), while the inset at right is a close-up of an area 75 times smaller (1/600th the size of the full area). This simulation showcases the cosmos as NASA's Nancy Grace Roman Space Telescope could see it. Roman will expand on the largest space-based galaxy survey like it—the Hubble Space Telescope's COSMOS survey—which imaged two square degrees of sky over the course of 42 days. In only 250 days, Roman will view more than a thousand times more of the sky with the same resolution. Credit: NASA
6 notes
·
View notes
Text
Prometheus Gave the Gift of Fire to Mankind. We Can't Give it Back, nor Should We.
AI. Artificial intelligence. Large Language Models. Learning Algorithms. Deep Learning. Generative Algorithms. Neural Networks. This technology has many names, and has been a polarizing topic in numerous communities online. By my observation, a lot of the discussion is either solely focused on A) how to profit off it or B) how to get rid of it and/or protect yourself from it. But to me, I feel both of these perspectives apply a very narrow usage lens on something that's more than a get rich quick scheme or an evil plague to wipe from the earth.
This is going to be long, because as someone whose degree is in psych and computer science, has been a teacher, has been a writing tutor for my younger brother, and whose fiance works in freelance data model training... I have a lot to say about this.
I'm going to address the profit angle first, because I feel most people in my orbit (and in related orbits) on Tumblr are going to agree with this: flat out, the way AI is being utilized by large corporations and tech startups -- scraping mass amounts of visual and written works without consent and compensation, replacing human professionals in roles from concept art to story boarding to screenwriting to customer service and more -- is unethical and damaging to the wellbeing of people, would-be hires and consumers alike. It's wasting energy having dedicated servers running nonstop generating content that serves no greater purpose, and is even pressing on already overworked educators because plagiarism just got a very new, harder to identify younger brother that's also infinitely more easy to access.
In fact, ChatGPT is such an issue in the education world that plagiarism-detector subscription services that take advantage of how overworked teachers are have begun paddling supposed AI-detectors to schools and universities. Detectors that plainly DO NOT and CANNOT work, because the difference between "A Writer Who Writes Surprisingly Well For Their Age" is indistinguishable from "A Language Replicating Algorithm That Followed A Prompt Correctly", just as "A Writer Who Doesn't Know What They're Talking About Or Even How To Write Properly" is indistinguishable from "A Language Replicating Algorithm That Returned Bad Results". What's hilarious is that the way these "detectors" work is also run by AI.
(to be clear, I say plagiarism detectors like TurnItIn.com and such are predatory because A) they cost money to access advanced features that B) often don't work properly or as intended with several false flags, and C) these companies often are super shady behind the scenes; TurnItIn for instance has been involved in numerous lawsuits over intellectual property violations, as their services scrape (or hopefully scraped now) the papers submitted to the site without user consent (or under coerced consent if being forced to use it by an educator), which it uses in can use in its own databases as it pleases, such as for training the AI detecting AI that rarely actually detects AI.)
The prevalence of visual and lingustic generative algorithms is having multiple, overlapping, and complex consequences on many facets of society, from art to music to writing to film and video game production, and even in the classroom before all that, so it's no wonder that many disgruntled artists and industry professionals are online wishing for it all to go away and never come back. The problem is... It can't. I understand that there's likely a large swath of people saying that who understand this, but for those who don't: AI, or as it should more properly be called, generative algorithms, didn't just show up now (they're not even that new), and they certainly weren't developed or invented by any of the tech bros peddling it to megacorps and the general public.
Long before ChatGPT and DALL-E came online, generative algorithms were being used by programmers to simulate natural processes in weather models, shed light on the mechanics of walking for roboticists and paleontologists alike, identified patterns in our DNA related to disease, aided in complex 2D and 3D animation visuals, and so on. Generative algorithms have been a part of the professional world for many years now, and up until recently have been a general force for good, or at the very least a force for the mundane. It's only recently that the technology involved in creating generative algorithms became so advanced AND so readily available, that university grad students were able to make the publicly available projects that began this descent into madness.
Does anyone else remember that? That years ago, somewhere in the late 2010s to the beginning of the 2020s, these novelty sites that allowed you to generate vague images from prompts, or generate short stylistic writings from a short prompt, were popping up with University URLs? Oftentimes the queues on these programs were hours long, sometimes eventually days or weeks or months long, because of how unexpectedly popular this concept was to the general public. Suddenly overnight, all over social media, everyone and their grandma, and not just high level programming and arts students, knew this was possible, and of course, everyone wanted in. Automated art and writing, isn't that neat? And of course, investors saw dollar signs. Simply scale up the process, scrape the entire web for data to train the model without advertising that you're using ALL material, even copyrighted and personal materials, and sell the resulting algorithm for big money. As usual, startup investors ruin every new technology the moment they can access it.
To most people, it seemed like this magic tech popped up overnight, and before it became known that the art assets on later models were stolen, even I had fun with them. I knew how learning algorithms worked, if you're going to have a computer make images and text, it has to be shown what that is and then try and fail to make its own until it's ready. I just, rather naively as I was still in my early 20s, assumed that everything was above board and the assets were either public domain or fairly licensed. But when the news did came out, and when corporations started unethically implementing "AI" in everything from chatbots to search algorithms to asking their tech staff to add AI to sliced bread, those who were impacted and didn't know and/or didn't care where generative algorithms came from wanted them GONE. And like, I can't blame them. But I also quietly acknowledged to myself that getting rid of a whole technology is just neither possible nor advisable. The cat's already out of the bag, the genie has left its bottle, the Pandorica is OPEN. If we tried to blanket ban what people call AI, numerous industries involved in making lives better would be impacted. Because unfortunately the same tool that can edit selfies into revenge porn has also been used to identify cancer cells in patients and aided in decoding dead languages, among other things.
When, in Greek myth, Prometheus gave us the gift of fire, he gave us both a gift and a curse. Fire is so crucial to human society, it cooks our food, it lights our cities, it disposes of waste, and it protects us from unseen threats. But fire also destroys, and the same flame that can light your home can burn it down. Surely, there were people in this mythic past who hated fire and all it stood for, because without fire no forest would ever burn to the ground, and surely they would have called for fire to be given back, to be done away with entirely. Except, there was no going back. The nature of life is that no new element can ever be undone, it cannot be given back.
So what's the way forward, then? Like, surely if I can write a multi-paragraph think piece on Tumblr.com that next to nobody is going to read because it's long as sin, about an unpopular topic, and I rarely post original content anyway, then surely I have an idea of how this cyberpunk dystopia can be a little less.. Dys. Well I do, actually, but it's a long shot. Thankfully, unlike business majors, I actually had to take a cyber ethics course in university, and I actually paid attention. I also passed preschool where I learned taking stuff you weren't given permission to have is stealing, which is bad. So the obvious solution is to make some fucking laws to limit the input on data model training on models used for public products and services. It's that simple. You either use public domain and licensed data only or you get fined into hell and back and liable to lawsuits from any entity you wronged, be they citizen or very wealthy mouse conglomerate (suing AI bros is the only time Mickey isn't the bigger enemy). And I'm going to be honest, tech companies are NOT going to like this, because not only will it make doing business more expensive (boo fucking hoo), they'd very likely need to throw out their current trained datasets because of the illegal components mixed in there. To my memory, you can't simply prune specific content from a completed algorithm, you actually have to redo rhe training from the ground up because the bad data would be mixed in there like gum in hair. And you know what, those companies deserve that. They deserve to suffer a punishment, and maybe fold if they're young enough, for what they've done to creators everywhere. Actually, laws moving forward isn't enough, this needs to be retroactive. These companies need to be sued into the ground, honestly.
So yeah, that's the mess of it. We can't unlearn and unpublicize any technology, even if it's currently being used as a tool of exploitation. What we can do though is demand ethical use laws and organize around the cause of the exclusive rights of individuals to the content they create. The screenwriter's guild, actor's guild, and so on already have been fighting against this misuse, but given upcoming administration changes to the US, things are going to get a lot worse before thet get a little better. Even still, don't give up, have clear and educated goals, and focus on what you can do to affect change, even if right now that's just individual self-care through mental and physical health crises like me.
#ai#artificial intelligence#generative algorithms#llm#large language model#chatgpt#ai art#ai writing#kanguin original
9 notes
·
View notes
Text
Making the art world more accessible
New Post has been published on https://thedigitalinsider.com/making-the-art-world-more-accessible/
Making the art world more accessible
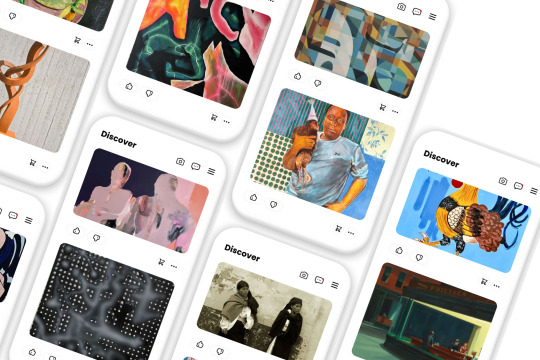
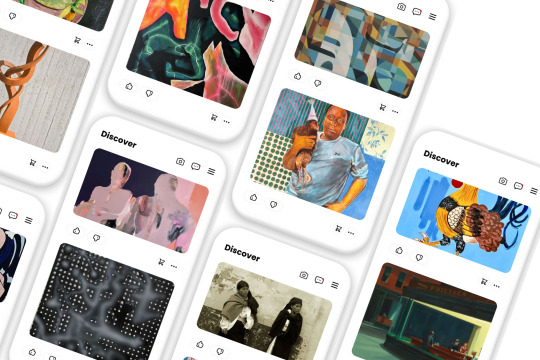
In the world of high-priced art, galleries usually act as gatekeepers. Their selective curation process is a key reason galleries in major cities often feature work from the same batch of artists. The system limits opportunities for emerging artists and leaves great art undiscovered.
NALA was founded by Benjamin Gulak ’22 to disrupt the gallery model. The company’s digital platform, which was started as part of an MIT class project, allows artists to list their art and uses machine learning and data science to offer personalized recommendations to art lovers.
By providing a much larger pool of artwork to buyers, the company is dismantling the exclusive barriers put up by traditional galleries and efficiently connecting creators with collectors.
“There’s so much talent out there that has never had the opportunity to be seen outside of the artists’ local market,” Gulak says. “We’re opening the art world to all artists, creating a true meritocracy.”
NALA takes no commission from artists, instead charging buyers an 11.5 percent commission on top of the artist’s listed price. Today more than 20,000 art lovers are using NALA’s platform, and the company has registered more than 8,500 artists.
“My goal is for NALA to become the dominant place where art is discovered, bought, and sold online,” Gulak says. “The gallery model has existed for such a long period of time that they are the tastemakers in the art world. However, most buyers never realize how restrictive the industry has been.”
From founder to student to founder again
Growing up in Canada, Gulak worked hard to get into MIT, participating in science fairs and robotic competitions throughout high school. When he was 16, he created an electric, one-wheeled motorcycle that got him on the popular television show “Shark Tank” and was later named one of the top inventions of the year by Popular Science.
Gulak was accepted into MIT in 2009 but withdrew from his undergrad program shortly after entering to launch a business around the media exposure and capital from “Shark Tank.” Following a whirlwind decade in which he raised more than $12 million and sold thousands of units globally, Gulak decided to return to MIT to complete his degree, switching his major from mechanical engineering to one combining computer science, economics, and data science.
“I spent 10 years of my life building my business, and realized to get the company where I wanted it to be, it would take another decade, and that wasn’t what I wanted to be doing,” Gulak says. “I missed learning, and I missed the academic side of my life. I basically begged MIT to take me back, and it was the best decision I ever made.”
During the ups and downs of running his company, Gulak took up painting to de-stress. Art had always been a part of Gulak’s life, and he had even done a fine arts study abroad program in Italy during high school. Determined to try selling his art, he collaborated with some prominent art galleries in London, Miami, and St. Moritz. Eventually he began connecting artists he’d met on travels from emerging markets like Cuba, Egypt, and Brazil to the gallery owners he knew.
“The results were incredible because these artists were used to selling their work to tourists for $50, and suddenly they’re hanging work in a fancy gallery in London and getting 5,000 pounds,” Gulak says. “It was the same artist, same talent, but different buyers.”
At the time, Gulak was in his third year at MIT and wondering what he’d do after graduation. He thought he wanted to start a new business, but every industry he looked at was dominated by tech giants. Every industry, that is, except the art world.
“The art industry is archaic,” Gulak says. “Galleries have monopolies over small groups of artists, and they have absolute control over the prices. The buyers are told what the value is, and almost everywhere you look in the industry, there’s inefficiencies.”
At MIT, Gulak was studying the recommender engines that are used to populate social media feeds and personalize show and music suggestions, and he envisioned something similar for the visual arts.
“I thought, why, when I go on the big art platforms, do I see horrible combinations of artwork even though I’ve had accounts on these platforms for years?” Gulak says. “I’d get new emails every week titled ‘New art for your collection,’ and the platform had no idea about my taste or budget.”
For a class project at MIT, Gulak built a system that tried to predict the types of art that would do well in a gallery. By his final year at MIT, he had realized that working directly with artists would be a more promising approach.
“Online platforms typically take a 30 percent fee, and galleries can take an additional 50 percent fee, so the artist ends up with a small percentage of each online sale, but the buyer also has to pay a luxury import duty on the full price,” Gulak explains. “That means there’s a massive amount of fat in the middle, and that’s where our direct-to-artist business model comes in.”
Today NALA, which stands for Networked Artistic Learning Algorithm, onboards artists by having them upload artwork and fill out a questionnaire about their style. They can begin uploading work immediately and choose their listing price.
The company began by using AI to match art with its most likely buyer. Gulak notes that not all art will sell — “if you’re making rock paintings there may not be a big market” — and artists may price their work higher than buyers are willing to pay, but the algorithm works to put art in front of the most likely buyer based on style preferences and budget. NALA also handles sales and shipments, providing artists with 100 percent of their list price from every sale.
“By not taking commissions, we’re very pro artists,” Gulak says. “We also allow all artists to participate, which is unique in this space. NALA is built by artists for artists.”
Last year, NALA also started allowing buyers to take a photo of something they like and see similar artwork from its database.
“In museums, people will take a photo of masterpieces they’ll never be able to afford, and now they can find living artists producing the same style that they could actually put in their home,” Gulak says. “It makes art more accessible.”
Championing artists
Ten years ago, Ben Gulak was visiting Egypt when he discovered an impressive mural on the street. Gulak found the local artist, Ahmed Nofal, on Instagram and bought some work. Later, he brought Nofal to Dubai to participate in World Art Dubai. The artist’s work was so well-received he ended up creating murals for the Royal British Museum in London and Red Bull. Most recently, Nofal and Gulak collaborated together during Art Basel 2024 doing a mural at the Museum of Graffiti in Miami.
Gulak has worked personally with many of the artists on his platform. For more than a decade he’s travelled to Cuba buying art and delivering art supplies to friends. He’s also worked with artists as they work to secure immigration visas.
“Many people claim they want to help the art world, but in reality, they often fall back on the same outdated business models,” says Gulak. “Art isn’t just my passion — it’s a way of life for me. I’ve been on every side of the art world: as a painter selling my work through galleries, as a collector with my office brimming with art, and as a collaborator working alongside incredible talents like Raheem Saladeen Johnson. When artists visit, we create together, sharing ideas and brainstorming. These experiences, combined with my background as both an artist and a computer scientist, give me a unique perspective. I’m trying to use technology to provide artists with unparalleled access to the global market and shake things up.”
#000#2024#Accounts#ai#algorithm#approach#Art#Artificial Intelligence#artists#Arts#background#Brazil#Building#Business#business model#Canada#cities#Competitions#computer#Computer Science#creators#cuba#data#data science#Database#Economics#Egypt#Electrical engineering and computer science (EECS)#emails#engineering
0 notes
Text
This day in history
#15yrsago EULAs + Arbitration = endless opportunity for abuse https://archive.org/details/TheUnconcionabilityOfArbitrationAgreementsInEulas
#15yrsago Wikipedia’s facts-about-facts make the impossible real https://web.archive.org/web/20091116023225/http://www.make-digital.com/make/vol20/?pg=16
#10yrsago Youtube nukes 7 hours’ worth of science symposium audio due to background music during lunch break https://memex.craphound.com/2014/11/25/youtube-nukes-7-hours-worth-of-science-symposium-audio-due-to-background-music-during-lunch-break/
#10yrsago El Deafo: moving, fresh YA comic-book memoir about growing up deaf https://memex.craphound.com/2014/11/25/el-deafo-moving-fresh-ya-comic-book-memoir-about-growing-up-deaf/
#5yrsago Networked authoritarianism may contain the seeds of its own undoing https://crookedtimber.org/2019/11/25/seeing-like-a-finite-state-machine/
#5yrsago After Katrina, neoliberals replaced New Orleans’ schools with charters, which are now failing https://www.nola.com/news/education/article_0c5918cc-058d-11ea-aa21-d78ab966b579.html
#5yrsago Talking about Disney’s 1964 Carousel of Progress with Bleeding Cool: our lost animatronic future https://bleedingcool.com/pop-culture/castle-talk-cory-doctorow-on-disneys-carousel-of-progress-and-lost-optimism/
#5yrsago Tiny alterations in training data can introduce “backdoors” into machine learning models https://arxiv.org/abs/1903.06638
#5yrsago Leaked documents document China’s plan for mass arrests and concentration-camp internment of Uyghurs and other ethnic minorities in Xinjiang https://www.icij.org/investigations/china-cables/exposed-chinas-operating-manuals-for-mass-internment-and-arrest-by-algorithm/
#5yrsago Hong Kong elections: overconfident Beijing loyalist parties suffer a near-total rout https://www.scmp.com/news/hong-kong/politics/article/3039132/results-blog
#5yrsago Library Socialism: a utopian vision of a sustaniable, luxuriant future of circulating abundance https://memex.craphound.com/2019/11/25/library-socialism-a-utopian-vision-of-a-sustaniable-luxuriant-future-of-circulating-abundance/
#1yrago The moral injury of having your work enshittified https://pluralistic.net/2023/11/25/moral-injury/#enshittification
7 notes
·
View notes
Note
Hi! I’m a student currently learning computer science in college and would love it if you had any advice for a cool personal project to do? Thanks!
Personal Project Ideas
Hiya!! 💕
It's so cool that you're a computer science student, and with that, you have plenty of options for personal projects that can help with learning more from what they teach you at college. I don't have any experience being a university student however 😅
Someone asked me a very similar question before because I shared my projects list and they asked how I come up with project ideas - maybe this can inspire you too, here's the link to the post [LINK]
However, I'll be happy to share some ideas with you right now. Just a heads up: you can alter the projects to your own specific interests or goals in mind. Though it's a personal project meaning not an assignment from school, you can always personalise it to yourself as well! Also, I don't know the level you are, e.g. beginner or you're pretty confident in programming, if the project sounds hard, try to simplify it down - no need to go overboard!!
But here is the list I came up with (some are from my own list):
Personal Finance Tracker
A web app that tracks personal finances by integrating with bank APIs. You can use Python with Flask for the backend and React for the frontend. I think this would be great for learning how to work with APIs and how to build web applications 🏦
Online Food Ordering System
A web app that allows users to order food from a restaurant's menu. You can use PHP with Laravel for the backend and Vue.js for the frontend. This helps you learn how to work with databases (a key skill I believe) and how to build interactive user interfaces 🙌🏾
Movie Recommendation System
I see a lot of developers make this on Twitter and YouTube. It's a machine-learning project that recommends movies to users based on their past viewing habits. You can use Python with Pandas, Scikit-learn, and TensorFlow for the machine learning algorithms. Obviously, this helps you learn about how to build machine-learning models, and how to use libraries for data manipulation and analysis 📊
Image Recognition App
This is more geared towards app development if you're interested! It's an Android app that uses image recognition to identify objects in a photo. You can use Java or Kotlin for the Android development and TensorFlow for machine learning algorithms. Learning how to work with image recognition and how to build mobile applications - which is super cool 👀
Social Media Platform
(I really want to attempt this one soon) A web app that allows users to post, share, and interact with each other's content. Come up with a cool name for it! You can use Ruby on Rails for the backend and React for the frontend. This project would be great for learning how to build full-stack web applications (a plus cause that's a trend that companies are looking for in developers) and how to work with user authentication and authorization (another plus)! 🎭
Text-Based Adventure Game
If you're interested in game developments, you could make a simple game where users make choices and navigate through a story by typing text commands. You can use Python for the game logic and a library like Pygame for the graphics. This project would be great for learning how to build games and how to work with input/output. 🎮
Weather App
Pretty simple project - I did this for my apprenticeship and coding night classes! It's a web app that displays weather information for a user's location. You can use Node.js with Express for the backend and React for the frontend. Working with APIs again, how to handle asynchronous programming, and how to build responsive user interfaces! 🌈
Online Quiz Game
A web app that allows users to take quizzes and compete with other players. You could personalise it to a module you're studying right now - making a whole quiz application for it will definitely help you study! You can use PHP with Laravel for the backend and Vue.js for the frontend. You get to work with databases, build real-time applications, and maybe work with user authentication. 🧮
Chatbot
(My favourite, I'm currently planning for this one!) A chatbot that can answer user questions and provide information. You can use Python with Flask for the backend and a natural language processing library like NLTK for the chatbot logic. If you want to mauke it more beginner friendly, you could use HTML, CSS and JavaScript and have hard-coded answers set, maybe use a bunch of APIs for the answers etc! This project would be great because you get to learn how to build chatbots, and how to work with natural language processing - if you go that far! 🤖
Another place I get inspiration for more web frontend dev projects is on Behance and Pinterest - on Pinterest search for like "Web design" or "[Specific project] web design e.g. shopping web design" and I get inspiration from a bunch of pins I put together! Maybe try that out!
I hope this helps and good luck with your project!
#my asks#resources#programming#coding#studying#codeblr#progblr#studyblr#comp sci#computer science#projects ideas#coding projects#coding study#cs studyblr#cs academia
178 notes
·
View notes
Text
The crazy thing about AI is I spent the summer of 2021 learning basic machine learning i.e. feed forward neural networks and convolutional neural networks to apply to science. People had models they'd trained on cat images to try to create new cat images and they looked awful but it was fun. And it was also beginning to look highly applicable to the field I'm in (astronomy/cosmology) where you will have an immense amount of 2d image data that can't easily be parsed with an algorithm and would take too many hours for a human to sift through. I was genuinely kinda excited about it then. But seemingly in a blink of an eye we have these chat bots and voice AI and thieving art AI and it's all deadset on this rapid acceleration into cyberpunk dystopia capitalist hellscape and I hate it hate hate it
33 notes
·
View notes
Text
How AI is Being Used to Predict Diseases from Genomic Data
Introduction
Ever wonder if science fiction got one thing right about the future of healthcare? Turns out, it might be the idea that computers will one day predict diseases before they strike. Thanks to Artificial Intelligence (AI) and genomics, we’re well on our way to making that a reality. From decoding the human genome at lightning speeds to spotting hidden disease patterns that even experts can’t see, AI-powered genomics is revolutionizing preventative care.
This article explores how AI is applied to genomic data, why it matters for the future of medicine, and what breakthroughs are on the horizon. Whether you’re a tech enthusiast, a healthcare professional, or simply curious about the potential of your own DNA, keep reading to find out how AI is rewriting the rules for disease prediction.
1. The Genomic Data Boom
In 2003, scientists completed the Human Genome Project, mapping out 3.2 billion base pairs in our DNA. Since then, genomic sequencing has become faster and more affordable, creating a flood of genetic data. However, sifting through that data by hand to predict diseases is nearly impossible. Enter machine learning—a key subset of AI that excels at identifying patterns in massive, complex datasets.
Why It Matters:
Reduced analysis time: Machine learning algorithms can sort through billions of base pairs in a fraction of the time it would take humans.
Actionable insights: Pinpointing which genes are associated with certain illnesses can lead to early diagnoses and personalized treatments.
2. AI’s Role in Early Disease Detection
Cancer: Imagine detecting cancerous changes in cells before a single tumor forms. By analyzing subtle genomic variants, AI can flag the earliest indicators of diseases such as breast, lung, or prostate cancer. Neurodegenerative Disorders: Alzheimer’s and Parkinson’s often remain undiagnosed until noticeable symptoms appear. AI tools scour genetic data to highlight risk factors and potentially allow for interventions years before traditional symptom-based diagnoses. Rare Diseases: Genetic disorders like Cystic Fibrosis or Huntington’s disease can be complex to diagnose. AI helps identify critical gene mutations, speeding up the path to diagnosis and paving the way for more targeted treatments.
Real-World Impact:
A patient’s entire genomic sequence is analyzed alongside millions of others, spotting tiny “red flags” for diseases.
Doctors can then focus on prevention: lifestyle changes, close monitoring, or early intervention.
3. The Magic of Machine Learning in Genomics
Supervised Learning: Models are fed labeled data—genomic profiles of patients who have certain diseases and those who do not. The AI learns patterns in the DNA that correlate with the disease.
Unsupervised Learning: This is where AI digs into unlabeled data, discovering hidden clusters and relationships. This can reveal brand-new biomarkers or gene mutations nobody suspected were relevant.
Deep Learning: Think of this as AI with “layers”—neural networks that continuously refine their understanding of gene sequences. They’re especially good at pinpointing complex, non-obvious patterns.
4. Personalized Medicine: The Future is Now
We often talk about “one-size-fits-all” medicine, but that approach ignores unique differences in our genes. Precision Medicine flips that on its head by tailoring treatments to your genetic profile, making therapies more effective and reducing side effects. By identifying which treatments you’re likely to respond to, AI can save time, money, and—most importantly—lives.
Pharmacogenomics (the study of how genes affect a person’s response to drugs) is one area booming with potential. Predictive AI models can identify drug-gene interactions, guiding doctors to prescribe the right medication at the right dose the first time.
5. Breaking Down Barriers and Ethical Considerations
1. Data Privacy
Genomic data is incredibly personal. AI companies and healthcare providers must ensure compliance with regulations like HIPAA and GDPR to keep that data safe.
2. Algorithmic Bias
AI is only as good as the data it trains on. Lack of diversity in genomic datasets can lead to inaccuracies or inequalities in healthcare outcomes.
3. Cost and Accessibility
While the price of DNA sequencing has dropped significantly, integrating AI-driven genomic testing into mainstream healthcare systems still faces cost and infrastructure challenges.
6. What’s Next?
Realtime Genomic Tracking: We can imagine a future where your genome is part of your regular health check-up—analyzed continuously by AI to catch new mutations as they develop.
Wider Disease Scope: AI’s role will likely expand beyond predicting just one or two types of conditions. Cardiovascular diseases, autoimmune disorders, and metabolic syndromes are all on the list of potential AI breakthroughs.
Collaborative Ecosystems: Tech giants, pharmaceutical companies, and healthcare providers are increasingly partnering to pool resources and data, accelerating the path to life-changing genomic discoveries.
7. Why You Should Care
This isn’t just about futuristic research; it’s a glimpse of tomorrow’s medicine. The more we rely on AI for genomic analysis, the more proactive we can be about our health. From drastically reducing the time to diagnose rare diseases to providing tailor-made treatments for common ones, AI is reshaping how we prevent and treat illnesses on a global scale.
Final Thoughts: Shaping the Future of Genomic Healthcare
AI’s impact on disease prediction through genomic data isn’t just a high-tech novelty—it’s a turning point in how we approach healthcare. Early detection, faster diagnosis, personalized treatment—these are no longer mere dreams but tangible realities thanks to the synergy of big data and cutting-edge machine learning.
As we address challenges like data privacy and algorithmic bias, one thing’s certain: the future of healthcare will be defined by how well we harness the power of our own genetic codes. If you’re as excited as we are about this transformative journey, share this post, spark discussions, and help spread the word about the life-changing possibilities of AI-driven genomics.
#genomics#bioinformatics#biotechcareers#datascience#biopractify#aiinbiotech#biotechnology#bioinformaticstools#biotech#machinelearning
3 notes
·
View notes
Text
What are AI, AGI, and ASI? And the positive impact of AI
Understanding artificial intelligence (AI) involves more than just recognizing lines of code or scripts; it encompasses developing algorithms and models capable of learning from data and making predictions or decisions based on what they’ve learned. To truly grasp the distinctions between the different types of AI, we must look at their capabilities and potential impact on society.
To simplify, we can categorize these types of AI by assigning a power level from 1 to 3, with 1 being the least powerful and 3 being the most powerful. Let’s explore these categories:
1. Artificial Narrow Intelligence (ANI)
Also known as Narrow AI or Weak AI, ANI is the most common form of AI we encounter today. It is designed to perform a specific task or a narrow range of tasks. Examples include virtual assistants like Siri and Alexa, recommendation systems on Netflix, and image recognition software. ANI operates under a limited set of constraints and can’t perform tasks outside its specific domain. Despite its limitations, ANI has proven to be incredibly useful in automating repetitive tasks, providing insights through data analysis, and enhancing user experiences across various applications.
2. Artificial General Intelligence (AGI)
Referred to as Strong AI, AGI represents the next level of AI development. Unlike ANI, AGI can understand, learn, and apply knowledge across a wide range of tasks, similar to human intelligence. It can reason, plan, solve problems, think abstractly, and learn from experiences. While AGI remains a theoretical concept as of now, achieving it would mean creating machines capable of performing any intellectual task that a human can. This breakthrough could revolutionize numerous fields, including healthcare, education, and science, by providing more adaptive and comprehensive solutions.
3. Artificial Super Intelligence (ASI)
ASI surpasses human intelligence and capabilities in all aspects. It represents a level of intelligence far beyond our current understanding, where machines could outthink, outperform, and outmaneuver humans. ASI could lead to unprecedented advancements in technology and society. However, it also raises significant ethical and safety concerns. Ensuring ASI is developed and used responsibly is crucial to preventing unintended consequences that could arise from such a powerful form of intelligence.
The Positive Impact of AI
When regulated and guided by ethical principles, AI has the potential to benefit humanity significantly. Here are a few ways AI can help us become better:
• Healthcare: AI can assist in diagnosing diseases, personalizing treatment plans, and even predicting health issues before they become severe. This can lead to improved patient outcomes and more efficient healthcare systems.
• Education: Personalized learning experiences powered by AI can cater to individual student needs, helping them learn at their own pace and in ways that suit their unique styles.
• Environment: AI can play a crucial role in monitoring and managing environmental changes, optimizing energy use, and developing sustainable practices to combat climate change.
• Economy: AI can drive innovation, create new industries, and enhance productivity by automating mundane tasks and providing data-driven insights for better decision-making.
In conclusion, while AI, AGI, and ASI represent different levels of technological advancement, their potential to transform our world is immense. By understanding their distinctions and ensuring proper regulation, we can harness the power of AI to create a brighter future for all.
8 notes
·
View notes
Text
AI & IT'S IMPACT
Unleashing the Power: The Impact of AI Across Industries and Future Frontiers
Artificial Intelligence (AI), once confined to the realm of science fiction, has rapidly become a transformative force across diverse industries. Its influence is reshaping the landscape of how businesses operate, innovate, and interact with their stakeholders. As we navigate the current impact of AI and peer into the future, it's evident that the capabilities of this technology are poised to reach unprecedented heights.
1. Healthcare:
In the healthcare sector, AI is a game-changer, revolutionizing diagnostics, treatment plans, and patient care. Machine learning algorithms analyze vast datasets to identify patterns, aiding in early disease detection. AI-driven robotic surgery is enhancing precision, reducing recovery times, and minimizing risks. Personalized medicine, powered by AI, tailors treatments based on an individual's genetic makeup, optimizing therapeutic outcomes.
2. Finance:
AI is reshaping the financial industry by enhancing efficiency, risk management, and customer experiences. Algorithms analyze market trends, enabling quicker and more accurate investment decisions. Chatbots and virtual assistants powered by AI streamline customer interactions, providing real-time assistance. Fraud detection algorithms work tirelessly to identify suspicious activities, bolstering security measures in online transactions.
3. Manufacturing:
In manufacturing, AI is optimizing production processes through predictive maintenance and quality control. Smart factories leverage AI to monitor equipment health, reducing downtime by predicting potential failures. Robots and autonomous systems, guided by AI, enhance precision and efficiency in tasks ranging from assembly lines to logistics. This not only increases productivity but also contributes to safer working environments.
4. Education:
AI is reshaping the educational landscape by personalizing learning experiences. Adaptive learning platforms use AI algorithms to tailor educational content to individual student needs, fostering better comprehension and engagement. AI-driven tools also assist educators in grading, administrative tasks, and provide insights into student performance, allowing for more effective teaching strategies.
5. Retail:
In the retail sector, AI is transforming customer experiences through personalized recommendations and efficient supply chain management. Recommendation engines analyze customer preferences, providing targeted product suggestions. AI-powered chatbots handle customer queries, offering real-time assistance. Inventory management is optimized through predictive analytics, reducing waste and ensuring products are readily available.
6. Future Frontiers:
A. Autonomous Vehicles: The future of transportation lies in AI-driven autonomous vehicles. From self-driving cars to automated drones, AI algorithms navigate and respond to dynamic environments, ensuring safer and more efficient transportation. This technology holds the promise of reducing accidents, alleviating traffic congestion, and redefining mobility.
B. Quantum Computing: As AI algorithms become more complex, the need for advanced computing capabilities grows. Quantucm omputing, with its ability to process vast amounts of data at unprecedented speeds, holds the potential to revolutionize AI. This synergy could unlock new possibilities in solving complex problems, ranging from drug discovery to climate modeling.
C. AI in Creativity: AI is not limited to data-driven tasks; it's also making inroads into the realm of creativity. AI-generated art, music, and content are gaining recognition. Future developments may see AI collaborating with human creators, pushing the boundaries of what is possible in fields traditionally associated with human ingenuity.
In conclusion, the impact of AI across industries is profound and multifaceted. From enhancing efficiency and precision to revolutionizing how we approach complex challenges, AI is at the forefront of innovation. The future capabilities of AI hold the promise of even greater advancements, ushering in an era where the boundaries of what is achievable continue to expand. As businesses and industries continue to embrace and adapt to these transformative technologies, the synergy between human intelligence and artificial intelligence will undoubtedly shape a future defined by unprecedented possibilities.
19 notes
·
View notes