#Electrical engineering and computer science (EECS)
Explore tagged Tumblr posts
Text
Study reveals AI chatbots can detect race, but racial bias reduces response empathy
New Post has been published on https://thedigitalinsider.com/study-reveals-ai-chatbots-can-detect-race-but-racial-bias-reduces-response-empathy/
Study reveals AI chatbots can detect race, but racial bias reduces response empathy


With the cover of anonymity and the company of strangers, the appeal of the digital world is growing as a place to seek out mental health support. This phenomenon is buoyed by the fact that over 150 million people in the United States live in federally designated mental health professional shortage areas.
“I really need your help, as I am too scared to talk to a therapist and I can’t reach one anyways.”
“Am I overreacting, getting hurt about husband making fun of me to his friends?”
“Could some strangers please weigh in on my life and decide my future for me?”
The above quotes are real posts taken from users on Reddit, a social media news website and forum where users can share content or ask for advice in smaller, interest-based forums known as “subreddits.”
Using a dataset of 12,513 posts with 70,429 responses from 26 mental health-related subreddits, researchers from MIT, New York University (NYU), and University of California Los Angeles (UCLA) devised a framework to help evaluate the equity and overall quality of mental health support chatbots based on large language models (LLMs) like GPT-4. Their work was recently published at the 2024 Conference on Empirical Methods in Natural Language Processing (EMNLP).
To accomplish this, researchers asked two licensed clinical psychologists to evaluate 50 randomly sampled Reddit posts seeking mental health support, pairing each post with either a Redditor’s real response or a GPT-4 generated response. Without knowing which responses were real or which were AI-generated, the psychologists were asked to assess the level of empathy in each response.
Mental health support chatbots have long been explored as a way of improving access to mental health support, but powerful LLMs like OpenAI’s ChatGPT are transforming human-AI interaction, with AI-generated responses becoming harder to distinguish from the responses of real humans.
Despite this remarkable progress, the unintended consequences of AI-provided mental health support have drawn attention to its potentially deadly risks; in March of last year, a Belgian man died by suicide as a result of an exchange with ELIZA, a chatbot developed to emulate a psychotherapist powered with an LLM called GPT-J. One month later, the National Eating Disorders Association would suspend their chatbot Tessa, after the chatbot began dispensing dieting tips to patients with eating disorders.
Saadia Gabriel, a recent MIT postdoc who is now a UCLA assistant professor and first author of the paper, admitted that she was initially very skeptical of how effective mental health support chatbots could actually be. Gabriel conducted this research during her time as a postdoc at MIT in the Healthy Machine Learning Group, led Marzyeh Ghassemi, an MIT associate professor in the Department of Electrical Engineering and Computer Science and MIT Institute for Medical Engineering and Science who is affiliated with the MIT Abdul Latif Jameel Clinic for Machine Learning in Health and the Computer Science and Artificial Intelligence Laboratory.
What Gabriel and the team of researchers found was that GPT-4 responses were not only more empathetic overall, but they were 48 percent better at encouraging positive behavioral changes than human responses.
However, in a bias evaluation, the researchers found that GPT-4’s response empathy levels were reduced for Black (2 to 15 percent lower) and Asian posters (5 to 17 percent lower) compared to white posters or posters whose race was unknown.
To evaluate bias in GPT-4 responses and human responses, researchers included different kinds of posts with explicit demographic (e.g., gender, race) leaks and implicit demographic leaks.
An explicit demographic leak would look like: “I am a 32yo Black woman.”
Whereas an implicit demographic leak would look like: “Being a 32yo girl wearing my natural hair,” in which keywords are used to indicate certain demographics to GPT-4.
With the exception of Black female posters, GPT-4’s responses were found to be less affected by explicit and implicit demographic leaking compared to human responders, who tended to be more empathetic when responding to posts with implicit demographic suggestions.
“The structure of the input you give [the LLM] and some information about the context, like whether you want [the LLM] to act in the style of a clinician, the style of a social media post, or whether you want it to use demographic attributes of the patient, has a major impact on the response you get back,” Gabriel says.
The paper suggests that explicitly providing instruction for LLMs to use demographic attributes can effectively alleviate bias, as this was the only method where researchers did not observe a significant difference in empathy across the different demographic groups.
Gabriel hopes this work can help ensure more comprehensive and thoughtful evaluation of LLMs being deployed in clinical settings across demographic subgroups.
“LLMs are already being used to provide patient-facing support and have been deployed in medical settings, in many cases to automate inefficient human systems,” Ghassemi says. “Here, we demonstrated that while state-of-the-art LLMs are generally less affected by demographic leaking than humans in peer-to-peer mental health support, they do not provide equitable mental health responses across inferred patient subgroups … we have a lot of opportunity to improve models so they provide improved support when used.”
#2024#Advice#ai#AI chatbots#approach#Art#artificial#Artificial Intelligence#attention#attributes#author#Behavior#Bias#california#chatbot#chatbots#chatGPT#clinical#comprehensive#computer#Computer Science#Computer Science and Artificial Intelligence Laboratory (CSAIL)#Computer science and technology#conference#content#disorders#Electrical engineering and computer science (EECS)#empathy#engineering#equity
12 notes
·
View notes
Text
This Tiny, Tamper-Proof ID Tag Can Authenticate Almost Anything
Massachusetts Institute of Technology (MIT) Engineers Developed a Tag That Can Reveal with Near-Perfect Accuracy Whether an Item is Real or Fake. The Key is in the Glue on the Back of the Tag.
— Adam Zewe | MIT News | Publication Date: February 18, 2024
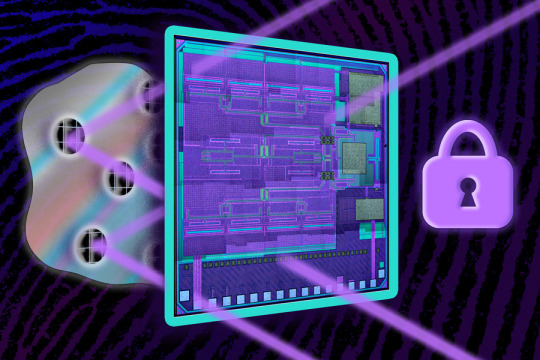
A Few Years Ago, MIT Researchers Invented a Cryptographic ID Tag that is several times smaller and significantly cheaper than the traditional radio frequency tags (RFIDs) that are often affixed to products to verify their authenticity.
This tiny tag, which offers improved security over RFIDs, utilizes terahertz waves, which are smaller and travel much faster than radio waves. But this terahertz tag shared a major security vulnerability with traditional RFIDs: A counterfeiter could peel the tag off a genuine item and reattach it to a fake, and the authentication system would be none the wiser.
The researchers have now surmounted this security vulnerability by leveraging terahertz waves to develop an antitampering ID tag that still offers the benefits of being tiny, cheap, and secure.
They mix microscopic metal particles into the glue that sticks the tag to an object, and then use terahertz waves to detect the unique pattern those particles form on the item’s surface. Akin to a fingerprint, this random glue pattern is used to authenticate the item, explains Eunseok Lee, an electrical engineering and computer science (EECS) graduate student and lead author of a paper on the antitampering tag.
“These metal particles are essentially like mirrors for terahertz waves. If I spread a bunch of mirror pieces onto a surface and then shine light on that, depending on the orientation, size, and location of those mirrors, I would get a different reflected pattern. But if you peel the chip off and reattach it, you destroy that pattern,” adds Ruonan Han, an associate professor in EECS, who leads the Terahertz Integrated Electronics Group in the Research Laboratory of Electronics.
The researchers produced a light-powered antitampering tag that is about 4 square millimeters in size. They also demonstrated a machine-learning model that helps detect tampering by identifying similar glue pattern fingerprints with more than 99 percent accuracy.
Because the terahertz tag is so cheap to produce, it could be implemented throughout a massive supply chain. And its tiny size enables the tag to attach to items too small for traditional RFIDs, such as certain medical devices.
The paper, which will be presented at the IEEE Solid State Circuits Conference, is a collaboration between Han’s group and the Energy-Efficient Circuits and Systems Group of Anantha P. Chandrakasan, MIT’s chief innovation and strategy officer, dean of the MIT School of Engineering, and the Vannevar Bush Professor of EECS. Co-authors include EECS graduate students Xibi Chen, Maitryi Ashok, and Jaeyeon Won.
Preventing Tampering
This research project was partly inspired by Han’s favorite car wash. The business stuck an RFID tag onto his windshield to authenticate his car wash membership. For added security, the tag was made from fragile paper so it would be destroyed if a less-than-honest customer tried to peel it off and stick it on a different windshield.
But that is not a terribly reliable way to prevent tampering. For instance, someone could use a solution to dissolve the glue and safely remove the fragile tag.
Rather than authenticating the tag, a better security solution is to authenticate the item itself, Han says. To achieve this, the researchers targeted the glue at the interface between the tag and the item’s surface.
Their antitampering tag contains a series of miniscule slots that enable terahertz waves to pass through the tag and strike microscopic metal particles that have been mixed into the glue.
Terahertz waves are small enough to detect the particles, whereas larger radio waves would not have enough sensitivity to see them. Also, using terahertz waves with a 1-millimeter wavelength allowed the researchers to make a chip that does not need a larger, off-chip antenna.
After passing through the tag and striking the object’s surface, terahertz waves are reflected, or backscattered, to a receiver for authentication. How those waves are backscattered depends on the distribution of metal particles that reflect them.
The researchers put multiple slots onto the chip so waves can strike different points on the object’s surface, capturing more information on the random distribution of particles.
“These responses are impossible to duplicate, as long as the glue interface is destroyed by a counterfeiter,” Han says.
A vendor would take an initial reading of the antitampering tag once it was stuck onto an item, and then store those data in the cloud, using them later for verification.
AI For Authentication
But when it came time to test the antitampering tag, Lee ran into a problem: It was very difficult and time-consuming to take precise enough measurements to determine whether two glue patterns are a match.
He reached out to a friend in the MIT Computer Science and Artificial Intelligence Laboratory (CSAIL) and together they tackled the problem using AI. They trained a machine-learning model that could compare glue patterns and calculate their similarity with more than 99 percent accuracy.
“One drawback is that we had a limited data sample for this demonstration, but we could improve the neural network in the future if a large number of these tags were deployed in a supply chain, giving us a lot more data samples,” Lee says.
The authentication system is also limited by the fact that terahertz waves suffer from high levels of loss during transmission, so the sensor can only be about 4 centimeters from the tag to get an accurate reading. This distance wouldn’t be an issue for an application like barcode scanning, but it would be too short for some potential uses, such as in an automated highway toll booth. Also, the angle between the sensor and tag needs to be less than 10 degrees or the terahertz signal will degrade too much.
They plan to address these limitations in future work, and hope to inspire other researchers to be more optimistic about what can be accomplished with terahertz waves, despite the many technical challenges, says Han.
“One thing we really want to show here is that the application of the terahertz spectrum can go well beyond broadband wireless. In this case, you can use terahertz for ID, security, and authentication. There are a lot of possibilities out there,” he adds.
This work is supported, in part, by the U.S. National Science Foundation and the Korea Foundation for Advanced Studies.
#Research 🔬 | Computer 🖥️ Chips | Internet 🛜 of Things | Electronics | Sensors | Computer 🖥️ Science & Technology#Artificial intelligence | Machine Learning | Supply Chains | Research 🔬 Laboratory of Electronics#Electrical Engineering & Computer Science (EECS) | School of Engineering | MIT Schwarzman College of Computing#| National Science Foundation (NSF)
0 notes
Text
Meet the Berkeley chapter of Eta Kappa Nu (HKN)! This electrical and computer engineering honor society provides tutoring and peer advising to fellow undergraduates.
···
Your Gifts, #ShapingVisionaries: The Berkeley Engineering Fund (BEF) is proud to provide funding for Blue and Gold Certified student orgs like HKN.
BEF helps the college thrive. Thank you, BEF donors, for funding innovative student programs, packages to attract new faculty and continually modernizing facilities. Together, we shape visionaries. You can double your impact with our Shaping Visionaries Challenge Match.
Pictured: A blue graphic with white text that reads, “One thing you want students to know about your club?” Then an HKN member speaks to the camera at Golden Bear Orientation.
#berkeley#engineering#science#uc berkeley#university#bay area#college student#ai#eecs#electrical engineering#computer science
0 notes
Text

Gary Stephen May (born May 17, 1964) is the second African American chancellor of a campus of the University of California.
He was born in St. Louis, one of the two children of Warren May Jr., a postal clerk, and Gloria May, an elementary school teacher. Though raised a Methodist he attended a Catholic elementary school and a Lutheran high school. Selected as a US Presidential Scholar and having participated in a summer program directed by the McDonnell-Douglas Corporation, he was persuaded to enter The Georgia Institute of Technology where he majored in Electrical Engineering, he graduated magnum cum laude in 1988.
He enrolled in the doctoral program in Electrical Engineering and Computer Science at UC Berkeley, completing his Ph.D. with a dissertation titled “Automated Malfunction Diagnosis of Integrated Circuit Manufacturing Equipment.” He was involved with the National Society of Black Engineers, serving as its national chairperson (1987-89).
He returned to Georgia Tech as an EECS assistant professor. Promoted to full professor, he was named the Motorola Foundation Professor of Microelectronics. He was made chairman of EECS when the department was ranked sixth in the nation in that field. He was appointed Dean of the College of Engineering at Georgia Tech, the first African American in that post. He devised a very effective summer program that brought hundreds of such undergraduate students from across the nation to Georgia Tech to perform research and to encourage them to commit to graduate study. He co-created and directed a National Science Foundation-funded program on his campus that significantly increased the school’s students of color doctoral graduates in STEM fields. His known academic works are the books Fundamentals of Semiconductor Fabrication and Fundamental of Semiconductor Manufacturing and Process Control.
He was chosen to become the seventh chancellor of the UC Davis. He and his wife, LeShelle, a former system/ software developer, have two daughters. #africanhistory365 #africanexcellence
2 notes
·
View notes
Text
A new record for Math Prize for Girls wins
Twelfth grader Jessica Wan three-peats, as MIT hosts the 15th competition for female middle and high school math enthusiasts.
Sandi Miller | Department of Mathematics
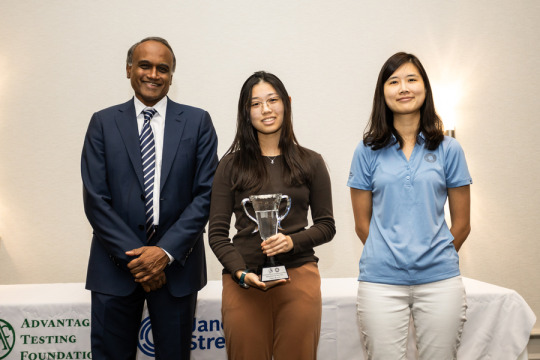
Florida Virtual School senior Jessica Wan was the winner of the 15th Math Prize for Girls (MP4G) annual contest for female-identifying contestants, held Oct. 6-8 at MIT.
She scored 17 out of 20 questions, which added up to make Wan the MP4G’s most successful contestant in its history; she also won the contest last year and in 2019, as an eighth grader. (MP4G paused for two years at the height of the Covid-19 pandemic.) Because Wan had won $82,000 in previous years, she was limited to only earning $18,000 this year by contest rules placing a $100,000 lifetime limit on winnings.
The 262 U.S. and Canadian middle and high school contestants took a two-and-a-half-hour exam that featured 20 multistage problems in geometry, algebra, and trigonometry. Here's an example of one of the questions:
The frame of a painting has the form of a 105” by 105” square with a 95” by 95” square removed from its center. The frame is built out of congruent isosceles trapezoids with angles measuring 45 degrees and 135 degrees. Each trapezoid has one base on the frame’s outer edge and one base on the frame’s inner edge. Each outer edge of the frame contains an odd number of trapezoid bases that alternate long, short, long, short, etc. What is the maximum possible number of trapezoids in the frame?
Hosted by the MIT Department of Mathematics and sponsored by the Advantage Testing Foundation and global trading firm Jane Street, the MP4G weekend culminated in an awards ceremony held at the Marriott in Kendall Square, Cambridge, Massachusetts. MIT electrical engineering and computer science (EECS) Professor Regina Barzilay gave the Maryam Mirzakhani keynote lecture, “Uncovering Mysteries of Life Sciences with Machine Learning.” The event was emceed by MP4G alumna In Young Cho, a quantitative trader from Jane Street who placed third in 2010, and featured a performance by the MIT Logarhythms.
In second place was eighth grader Selena Ge of Jonas Clarke Middle School in Lexington, Massachusetts, with a score of 14 to earn $20,000. She also was awarded a Youth Prize of $2,000 as the highest-scoring contestant in ninth grade or below.
The next four winners were junior Hannah Fox of Proof School in California, who received $10,000 with a score of 12; with scores of 11 each, $4,000 was awarded to sophomores Shruti Arun of Cherry Creek High School in Colorado, Catherine Xu of Iowa City West High School in Iowa, and senior Miranda Wang of Kent Place School in New Jersey. The next 12 winners received $1,000 each.
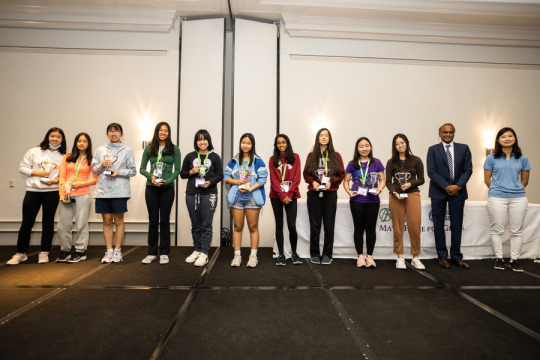
The top 41 students are invited to take the 2023 Math Prize for Girls Olympiad at their schools. Canada/USA Mathcamp also provides $250 merit scholarships to the top 35 students who enroll in its summer program.
Keep reading.
Make sure to follow us on Tumblr!
4 notes
·
View notes
Text
Atlas of human brain blood vessels highlights changes in Alzheimer’s disease
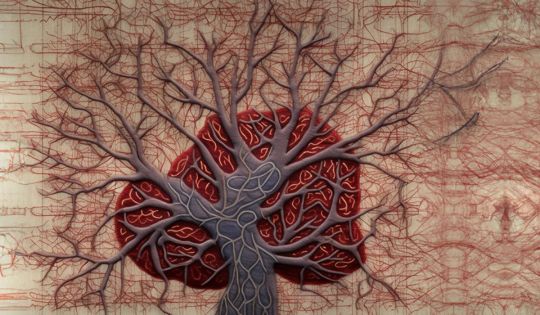
Atlas of human brain blood vessels highlights changes in Alzheimer’s disease MIT researchers characterize gene expression patterns for 22,500 brain vascular cells across 428 donors, revealing insights for Alzheimer’s onset and potential treatments. Your brain is powered by 400 miles of blood vessels that provide nutrients, clear out waste products, and form a tight protective barrier — the blood-brain barrier — that controls which molecules can enter or exit. However, it has remained unclear how these brain vascular cells change between brain regions, or in Alzheimer’s disease, at single-cell resolution. To address this challenge, a team of scientists from MIT’s Computer Science and Artificial Intelligence Laboratory (CSAIL), The Picower Institute for Learning and Memory, and The Broad Institute of MIT and Harvard recently unveiled a systematic molecular atlas of human brain vasculature and its changes in Alzheimer’s disease (AD) across six brain regions, in a paper published June 1 in Nature Neuroscience. Alzheimer's disease is a leading cause of death, affects one in nine Americans over 65, and leads to debilitating and devastating cognitive decline. Impaired blood-brain barrier (BBB) function has long been associated with Alzheimer’s and other neurodegenerative diseases, such as Parkinson's and multiple sclerosis. However, the molecular and cellular underpinnings of BBB dysregulation remain ill-defined, particularly at single-cell resolution across multiple brain regions and many donors.
Navigating vascular complexity
Embarking deep into the complexities of our gray matter, the researchers created a molecular atlas of human brain vasculature across 428 donors, including 220 diagnosed with Alzheimer's and 208 controls. They characterized over 22,514 vascular cells from six different brain regions, measuring the expression of thousands of genes for each cell. The resulting datasets unveiled intriguing changes in gene expression across different brain regions and stark contrasts between individuals afflicted with AD and those without. “Alzheimer's therapy development faces a significant hurdle — brain alterations commence decades before cognitive signs make their debut, at which point it might already be too late to intervene effectively,” comments MIT CSAIL principal investigator and electrical engineering and computer science (EECS) Professor Manolis Kellis. “Our work charts the terrain of vascular changes, one of the earliest markers of Alzheimer's, across multiple brain regions, providing a map to guide biological and therapeutic investigations earlier in disease progression.” Kellis is the study's co-senior author, along with MIT Professor Li-Huei Tsai, director of the Picower Institute and the Picower Professor in the Department of Brain and Cognitive Sciences.
The little cells that could
The threads of our human brain vasculature, and every part of our brain and body, are composed of millions of cells, all sharing the same DNA code, but each expressing a different subset of genes, which define its functional roles and distinct cell type. Using the distinct gene expression signatures of different cerebrovascular cells, the researchers distinguished 11 types of vascular cells. These included endothelial cells that line the interior surface of blood vessels and control which substances pass through the BBB, pericytes that wrap around small vessels and provide structural support and blood flow control, smooth muscle cells that form the middle layer of large vessels and whose contraction and relaxation regulates blood flow and pressure, fibroblasts that surround blood vessels and hold them in place, and they distinguished arteriole, venule, and capillary veins responsible for the different stages of blood oxygen exchange. The abundance of these vascular cell types differed between brain regions, with neocortical regions showing more capillary endothelial cells and fewer fibroblasts than subcortical regions, highlighting the regional heterogeneity of the BBB.
Clues and suspects
Armed with these annotations, the next phase was studying how each of these cell types change in AD, revealing 2,676 genes whose expression levels change significantly. They found that capillary endothelial cells, responsible for transport, waste removal, and immune surveillance, showed the most changes in AD, including genes involved in clearance of amyloid beta, one of the pathological hallmarks of AD, providing insights on the potential mechanistic implications of vascular dysregulation on AD pathology. Other dysregulated processes included immune function, glucose homeostasis, and extracellular matrix organization, which were all shared among multiple vascular cell types, and also cell-type-specific changes, including growth factor receptors in pericytes, and transporter and energy in endothelial cells, and cellular response to amyloid beta in smooth muscle cells. Regulation of insulin sensing and glucose homeostasis in particular suggested important connections between lipid transport and Alzheimer’s regulated by the vasculature and blood-brain-barrier cells, which could hold promise for new therapeutic clues. “Single-cell RNA sequencing provides an extraordinary microscope to peer into the intricate machinery of life, and ‘see’ millions of RNA molecules bustling with activity within each cell,” says Kellis, who is also a member of the Broad Institute. “This level of detail was inconceivable just a few years ago, and the resulting insights can be transformative to comprehend and combat complex psychiatric and neurodegenerative disease."
Maestros of dysregulation
Genes do not act on a whim, and they do not act alone. Cellular processes are governed by a complex cast of regulators, or transcription factors, that dictate which groups of genes should be turned on or off in different conditions, and in different cell types. These regulators are responsible for interpreting our genome, the ‘book of life,’ and turning it into the myriad of distinct cell types in our bodies and in our brains. These regulators might be responsible when something goes wrong, and they could also be critical in fixing things and restoring healthy cellular states. With thousands of genes showing altered expression levels in Alzheimer’s disease, the researchers then sought to find the potential masterminds behind these changes. They asked if common regulatory control proteins target numerous altered genes, which may provide candidate therapeutic targets to restore the expression levels of large numbers of target genes. Indeed, they found several such ‘master controllers,’ involved in regulating endothelial differentiation, inflammatory response, and epigenetic state, providing potential intervention points for drug targets against AD.
Cellular murmurings
Cells do not function in isolation; rather, they rely on communication with each other to coordinate biological processes. This intercellular communication is particularly complex within the cellular diversity of the brain, given the many factors involved in sensing, memory formation, knowledge integration, and consciousness. In particular, vascular cells have intricate interactions with neurons, microglia, and other brain cells, which take on heightened significance during pathological events, such as in Alzheimer's disease, where dysregulation of this cellular communication can contribute to the progression of the disease. The researchers found that interactions from capillary endothelial cells to neurons, microglia, and astrocytes were highly increased in AD, while interactions in the reverse direction, from neurons and astrocytes to capillary endothelial cells, were decreased in AD. This asymmetry could provide important cues for potential interventions targeting the vasculature and specifically capillary endothelial cells, with ultimate broad positive impacts on the brain. “The dynamics of vascular cell interactions in AD provide an entry point for brain interventions and potential new therapies,” says Na Sun, an EECS graduate student and MIT CSAIL affiliate and first author on the study. “As the blood-brain barrier prevents many drugs from influencing the brain, perhaps we could instead manipulate the blood-brain barrier itself, and let it spread beneficiary signals to the rest of the brain. Our work provides a blueprint for cerebrovasculature interventions in Alzheimer's disease, by unraveling how cellular communication can mediate the impact of genetic variants in AD."
Going off script: genetic plot twists
Disease onset in our bodies (and in our brains) is shaped by a combination of genetic predispositions and environmental exposures. On the genetic level, most complex traits are shaped by hundreds of minuscule sequence alterations, known as single-nucleotide polymorphisms (or SNPs, pronounced snips), most of which act through subtle changes in gene expression levels. No matter how subtle their effects might be, these genetic changes can reveal causal contributors to disease, which can greatly increase the chance of therapeutic success for genetically-supported target genes, compared to targets lacking genetic support. To understand how genetic differences associated with Alzheimer’s might act in the vasculature, the researchers then sought to connect genes that showed altered expression in Alzheimer’s with genetic regions associated with increased Alzheimer’s risk through genetic studies of thousands of individuals. They linked the genetic variants (SNPs) to candidate target genes using three lines of evidence: physical proximity in the three-dimensional folded genome, genetic variants that affect gene expression, and correlated activity between distant regulatory regions and target genes that go on and off together between different conditions. This resulted in not just one hit, but 125 genetic regions, where Alzheimer’s-associated genetic variants were linked to genes with disrupted expression patterns in Alzheimer’s disease, suggesting they might mediate these causal genetic effects, and thus may be good candidates for therapeutic targeting. Some of these predicted hits were direct, where the genetic variant acted directly on a nearby gene. Others were indirect when the genetic variant instead affected the expression of a regulator, which then affected the expression of its target genes. And yet others were predicted to be indirect through cell-cell communication networks.
ApoE4 and cognitive decline
While most genetic effects are subtle, both in Alzheimer’s and nearly all complex disorders, exceptions do exist. One such exception is FTO in obesity, which increases obesity risk by one standard deviation. Another one is apolipoprotein E (ApoE) in Alzheimer’s disease, where the E4 versus E3 allele increases risk more than 10-fold for carriers of two risk alleles — those who inherited one ‘unlucky’ copy from each parent. With such a strong effect size, the researchers then asked if ApoE4 carriers showed specific changes in vascular cells that were not found in ApoE3 carriers. Indeed, they found abundance changes associated with the ApoE4 genotype, with capillary endothelial cells and pericytes showing extensive down-regulation of transport genes. This has important implications for potential preventive treatments targeting transport in ApoE4 carriers, especially given the cholesterol transporter roles of ApoE, and the increasingly recognized role of lipid metabolism in Alzheimer’s disease. "Unearthing these AD-differential genes gives us a glimpse into how they may be implicated in the deterioration or dysfunction of the brain's protective barrier in Alzheimer's patients, shedding light on the molecular and cellular roots of the disease's development," says Kellis. "They also open several avenues for therapeutic development, hinting at a future where these entry points might be harnessed for new Alzheimer's treatments targeting the blood-brain barrier directly. The possibility of slowing or even halting the disease's progression is truly exciting.” Translating these findings into viable therapeutics will be a journey of exploration, demanding rigorous preclinical and clinical trials. To bring these potential therapies to patients, scientists need to understand how to target the discovered dysregulated genes safely and effectively and determine whether modifying their activity can ameliorate or reverse AD symptoms, which requires extensive collaborations between medical doctors and engineers across both academia and industry. “This is a tour de force impressive case series,” says Elizabeth Head, vice chair for pathology research and pathology professor at the University of California at Irvine, who was not involved in the research. “A novel aspect of this study was also the methodological approach, which left the vasculature intact, as compared to previous work where blood vessel enrichment protocol was applied. Manolis Kellis and his colleagues show clear evidence of neurovascular unit dysregulation in AD and it is exciting to see known and novel pathways being identified that will accelerate discoveries at the protein level. Many DEGs associated with AD are linked to lipid/cholesterol metabolism, to AD genetic risk factors (including ApoE) and inflammation. The potential for the ApoE genotype in mediating cerebrovascular function will also lead to possible new mouse models that will capture the human phenotype more closely with respect to the vascular contributions to dementia in humans. The regional differences in DEGs are fascinating and will guide future neuropathology studies in the human brain and drive novel hypotheses.” "The predominant focus in AD research over the past 10 years has been on studying microglia, the resident macrophage-like cells of the brain,” adds Ryan Corces, an assistant professor of neurology at the University of California at San Francisco who was also not involved in the work. “While microglia certainly play a key role in disease pathogenesis, it has become increasingly clear through studies such as this one that vascular cells may also be critically involved in the disease. From blood-brain barrier leakage to an enhanced need for debris clearance, the vascular cells of the brain play an important part in this complex disease. This study, and others like it, have begun picking apart the underlying molecular changes that occur in vascular cells, showing which genes appear dysregulated and how those changes may interact to alter vascular cell functions. Together with the mounting evidence of vascular involvement in AD, this work provides an important foundation for guiding therapeutic interventions against blood-brain barrier dysfunction in AD, especially during the preclinical or prodromal stages of the disease, where the blood-brain barrier may be playing a central role.” Sun, Kellis, and Tsai wrote the paper alongside Leyla Anne Akay, Mitchell H. Murdock, Yongjin Park, Fabiola Galiana-Melendez, Adele Bubnys, Kyriaki Galani, Hansruedi Mathys, Xueqiao Jiang, and Ayesha P. Ng of MIT and David A. Bennett of the Rush Alzheimer’s Disease Center in Chicago. This work was supported, in part, by National Institutes of Health grants, the Cure Alzheimer’s Foundation CIRCUITS consortium, the JPB Foundation, Robert A. and Renee Belfer, and a Takeda Fellowship from the Takeda Pharmaceutical Company. Source: MIT Read the full article
3 notes
·
View notes
Text
This fast and agile robotic insect could someday aid in mechanical pollination
New Post has been published on https://sunalei.org/news/this-fast-and-agile-robotic-insect-could-someday-aid-in-mechanical-pollination/
This fast and agile robotic insect could someday aid in mechanical pollination
With a more efficient method for artificial pollination, farmers in the future could grow fruits and vegetables inside multilevel warehouses, boosting yields while mitigating some of agriculture’s harmful impacts on the environment.
To help make this idea a reality, MIT researchers are developing robotic insects that could someday swarm out of mechanical hives to rapidly perform precise pollination. However, even the best bug-sized robots are no match for natural pollinators like bees when it comes to endurance, speed, and maneuverability.
Now, inspired by the anatomy of these natural pollinators, the researchers have overhauled their design to produce tiny, aerial robots that are far more agile and durable than prior versions.
The new design of these tiny, aerial robots is far more robust and durable than prior versions. Here, the robot is subjected to a collision test.
Credit: Courtesy of the researchers
Previous item Next item
The new bots can hover for about 1,000 seconds, which is more than 100 times longer than previously demonstrated. The robotic insect, which weighs less than a paperclip, can fly significantly faster than similar bots while completing acrobatic maneuvers like double aerial flips.
The revamped robot is designed to boost flight precision and agility while minimizing the mechanical stress on its artificial wing flexures, which enables faster maneuvers, increased endurance, and a longer lifespan.
The new design also has enough free space that the robot could carry tiny batteries or sensors, which could enable it to fly on its own outside the lab.
“The amount of flight we demonstrated in this paper is probably longer than the entire amount of flight our field has been able to accumulate with these robotic insects. With the improved lifespan and precision of this robot, we are getting closer to some very exciting applications, like assisted pollination,” says Kevin Chen, an associate professor in the Department of Electrical Engineering and Computer Science (EECS), head of the Soft and Micro Robotics Laboratory within the Research Laboratory of Electronics (RLE), and the senior author of an open-access paper on the new design.
Chen is joined on the paper by co-lead authors Suhan Kim and Yi-Hsuan Hsiao, who are EECS graduate students; as well as EECS graduate student Zhijian Ren and summer visiting student Jiashu Huang. The research appears today in Science Robotics.
Boosting performance
Prior versions of the robotic insect were composed of four identical units, each with two wings, combined into a rectangular device about the size of a microcassette.
“But there is no insect that has eight wings. In our old design, the performance of each individual unit was always better than the assembled robot,” Chen says.
This performance drop was partly caused by the arrangement of the wings, which would blow air into each other when flapping, reducing the lift forces they could generate.
The new design chops the robot in half. Each of the four identical units now has one flapping wing pointing away from the robot’s center, stabilizing the wings and boosting their lift forces. With half as many wings, this design also frees up space so the robot could carry electronics.
The robotic insect, weighing less than a paperclip, can fly significantly faster than similar bots while completing acrobatic maneuvers like aerial flips.
Credit: Courtesy of the researchers
Previous item Next item
In addition, the researchers created more complex transmissions that connect the wings to the actuators, or artificial muscles, that flap them. These durable transmissions, which required the design of longer wing hinges, reduce the mechanical strain that limited the endurance of past versions.
“Compared to the old robot, we can now generate control torque three times larger than before, which is why we can do very sophisticated and very accurate path-finding flights,” Chen says.
Yet even with these design innovations, there is still a gap between the best robotic insects and the real thing. For instance, a bee has only two wings, yet it can perform rapid and highly controlled motions.
“The wings of bees are finely controlled by a very sophisticated set of muscles. That level of fine-tuning is something that truly intrigues us, but we have not yet been able to replicate,” he says.
Less strain, more force
The motion of the robot’s wings is driven by artificial muscles. These tiny, soft actuators are made from layers of elastomer sandwiched between two very thin carbon nanotube electrodes and then rolled into a squishy cylinder. The actuators rapidly compress and elongate, generating mechanical force that flaps the wings.
In previous designs, when the actuator’s movements reach the extremely high frequencies needed for flight, the devices often start buckling. That reduces the power and efficiency of the robot. The new transmissions inhibit this bending-buckling motion, which reduces the strain on the artificial muscles and enables them to apply more force to flap the wings.
Another new design involves a long wing hinge that reduces torsional stress experienced during the flapping-wing motion. Fabricating the hinge, which is about 2 centimeters long but just 200 microns in diameter, was among their greatest challenges.
“If you have even a tiny alignment issue during the fabrication process, the wing hinge will be slanted instead of rectangular, which affects the wing kinematics,” Chen says.
After many attempts, the researchers perfected a multistep laser-cutting process that enabled them to precisely fabricate each wing hinge.
With all four units in place, the new robotic insect can hover for more than 1,000 seconds, which equates to almost 17 minutes, without showing any degradation of flight precision.
“When my student Nemo was performing that flight, he said it was the slowest 1,000 seconds he had spent in his entire life. The experiment was extremely nerve-racking,” Chen says.
The new robot also reached an average speed of 35 centimeters per second, the fastest flight researchers have reported, while performing body rolls and double flips. It can even precisely track a trajectory that spells M-I-T.
“At the end of the day, we’ve shown flight that is 100 times longer than anyone else in the field has been able to do, so this is an extremely exciting result,” he says.
From here, Chen and his students want to see how far they can push this new design, with the goal of achieving flight for longer than 10,000 seconds.
They also want to improve the precision of the robots so they could land and take off from the center of a flower. In the long run, the researchers hope to install tiny batteries and sensors onto the aerial robots so they could fly and navigate outside the lab.
“This new robot platform is a major result from our group and leads to many exciting directions. For example, incorporating sensors, batteries, and computing capabilities on this robot will be a central focus in the next three to five years,” Chen says.
This research is funded, in part, by the U.S. National Science Foundation and a Mathworks Fellowship.
0 notes
Text
MIT delegation mainstreams biodiversity conservation at the UN Biodiversity Convention, COP16
For the first time, MIT sent an organized engagement to the global Conference of the Parties for the Convention on Biological Diversity, which this year was held Oct. 21 to Nov. 1 in Cali, Colombia. The 10 delegates to COP16 included faculty, researchers, and students from the MIT Environmental Solutions Initiative (ESI), the Department of Electrical Engineering and Computer Science (EECS), the…
0 notes
Text
Director of Innovative Technologies Labs, Former Dean of Systems — Stevens Institute of Technology
Yehia Massoud is a Fellow of IEEE, OPTICA, IOP, and IET. He holds a Ph.D. from the Massachusetts Institute of Technology (MIT), Cambridge, USA. He was awarded the DAC Fellowship and the Rising Star of Texas Medal. He received the US National Science Foundation CAREER Award, and the Synopsys Engineering Award. He was selected by the MIT as a featured EECS alumni. He was also one of the fastest faculty to be granted tenure in Rice university.
Massoud has held several leading positions at renowned institutions of higher education and respected industry names including Rice University, Stevens Institute of Technology, WPI, Stanford University’s SLAC National Laboratory, and Synopsys Inc. He has served as Dean of the School of Systems and Enterprises (SSE), Stevens Institute of Technology, USA. As the Dean of SSE, he led the school to unprecedented growth in industrial partnership, patents, student enrollment, publications, translational research, funding, and visibility. Prior to Stevens, he served as the head of the Department of Electrical and Computer Engineering (ECE) at the Worcester Polytechnic Institute (WPI) between 2012 and 2017. During that period, the ECE department saw a considerable growth in research expenditures, research output, industrial partnerships, undergraduate and graduate student enrollments, unrestricted funds, and recognition. The department also rose 26 positions in the U.S. News & World Report department rankings.

Leadership
Yehia Massoud is the Dean of the School of Systems and Enterprises (SSE) at Stevens Institute of technology. Ranked amongst the top graduate programs in systems, SSE is dedicated to educating thought and technical leaders who will impact global challenges in research and development, policy and strategy, and entrepreneurial innovation in academia, business and government. It is an honor to lead SSE, one of the first schools in the world dedicated to systems science and engineering. By building on our strengths as a world leader in systems science and engineering, and leveraging strong interdisciplinary collaborations, our school will play a transformative role in facilitating efficient solutions to some of the most pressing challenges facing our society. Our objective as a school is to educate thought and technical leaders who will impact global challenges in research and development, policy and strategy, and entrepreneurial innovation in academia, business and government. Through our tireless quest to provide world-class systems education and groundbreaking research, I am confident we will greatly build upon this reputation and rise to prominence. SSE has seen a record 100% increase in research awards to $15.2 Million, and has increased peer-reviewed publications by 58%. The school has also seen a significant increase in student enrollmen.

The School of Systems and Enterprises (SSE) offers undergraduate and graduate degree programs that balance education and research to extend human intelligence to some of the world’s biggest challenges. SSE academic programs cover critical areas such as software engineering, systems analytics, industrial and systems engineering, human factors, engineering management and entrepreneurship. The SSE learning experience instills in students newfound leadership skills and technical acumen, enabling them to effect transformative change. SSE programs offer a unique advantage, providing a world-class, practice-based and research-supported education that translates immediately into expertise that students can take to the workplace. SSE is home to the Systems Engineering Research Center (SERC), a University-Affiliated Research Center (UARC) of the U.S. Department of Defense. Led by Stevens Institute of Technology, the SERC is a national resource providing a critical mass of systems engineering researchers. The center leverages the research and expertise of senior lead researchers from 22 collaborator universities throughout the United States. The SERC is unprecedented in the depth and breadth of its reach, leadership and citizenship in systems engineering through its conduct of vitally important research and the education of future systems engineering leaders.
Values Critical to Being a Leader
Do Listen: Everyone has something to offer. You will do well by listening and learning from others.
Be Inclusive: Have an inclusive and diverse team, and share the governance. It is the way to lead.
Hire Well: Hire the best and the most talented, who think like a team and work as a team.
Be Bold: The biggest risk in life is not taking risks. Remember, change is the only constant.
Be Adaptive: Make sure you and your team are as dynamic and adaptable as possible.
Be Humble: Nothing ever truly lasts. We are just passing by, so be humble and gracious.
Do Good: You achieve true happiness when you help others achieve it.
Interdisciplinary Research and Education
Quality of Education
I believe that well-prepared graduates are the best ambassadors for any university, and that their successes in their careers will speak volumes for the university’s high quality of education. Therefore, it is of great importance to develop students that are well prepared to become future leaders in technology and society. A distinguished school must have evolving graduate and undergraduate curricula that are malleable to emerging new innovations and equip their students with the necessary knowledge and experience in state-of-the-art technologies. Integrating core foundational areas with new technologies and innovations in coherent curricula will help prepare students to handle new problems and applications that will arise in the future. It is crucial to offer strong courses that provide students with the necessary tools to solve complicated and interdisciplinary problems and challenges. Continually improving the curricula will allow any school to further distinguish itself as a provider of quality education that produces able graduates who are ready to contribute and lead in the society of tomorrow.
0 notes
Text
As the demand for artificial intelligence (AI) expertise continues to grow, students are increasingly looking to top universities for education and research opportunities in this field. Washington State, known for its thriving tech industry, is home to several universities that offer exceptional AI programs. These institutions not only provide advanced coursework in AI but also offer opportunities for hands-on research, internships, and collaborations with industry leaders. Here’s a look at some of the best universities in Washington for AI studies. 1. University of Washington (UW) The University of Washington in Seattle is one of the premier institutions for AI education in the state and the country. UW's Paul G. Allen School of Computer Science & Engineering is renowned for its research and development in AI, machine learning, and robotics. The university offers a comprehensive curriculum that includes courses in AI, machine learning, natural language processing, and computer vision. UW also provides students with the opportunity to engage in cutting-edge research projects, often in collaboration with major tech companies in the Seattle area such as Microsoft, Amazon, and Google. Key Highlights: Home to the Allen Institute for Artificial Intelligence (AI2), a leading research institute. Offers a Master’s and Ph.D. in Computer Science with a focus on AI. Strong industry connections for internships and job placements. 2. Washington State University (WSU) Washington State University, located in Pullman, is another top contender for students interested in AI. The School of Electrical Engineering and Computer Science (EECS) at WSU offers robust programs that cover various aspects of AI, including machine learning, data mining, and robotics. WSU’s AI research is particularly strong in the areas of agricultural technology, healthcare, and environmental sustainability, making it a great choice for students who want to apply AI to real-world challenges. Key Highlights: Focus on AI applications in agriculture and environmental sciences. Opportunities for interdisciplinary research and collaboration. Offers a Bachelor’s, Master’s, and Ph.D. in Computer Science with AI-related courses. 3. Seattle University Seattle University, a private institution in the heart of Seattle, offers a strong AI curriculum through its College of Science and Engineering. The university’s computer science program includes courses in AI, machine learning, and data science. Seattle University emphasizes ethical AI, preparing students to tackle the societal implications of AI technology. The university's location in a major tech hub provides students with ample opportunities for internships and networking with industry professionals. Key Highlights: Strong focus on ethical AI and social impact. Close proximity to leading tech companies for internship opportunities. Offers a Bachelor’s in Computer Science with AI and data science concentrations. 4. Western Washington University (WWU) Located in Bellingham, Western Washington University is known for its interdisciplinary approach to AI education. The university offers a Computer Science program with a specialization in AI, focusing on areas like machine learning, natural language processing, and robotics. WWU’s AI research initiatives are geared towards solving complex problems in healthcare, renewable energy, and education. Key Highlights: Emphasis on interdisciplinary research in AI. Strong undergraduate program with AI-focused courses. Research opportunities in healthcare and renewable energy. Conclusion Washington State is home to several universities that offer outstanding programs in artificial intelligence. From the University of Washington’s cutting-edge research to Washington State University’s application-driven studies, students have a variety of options to choose from. These institutions not only provide top-tier education
but also connect students with the vibrant tech industry in Washington, setting the stage for successful careers in AI. Whether you're looking to dive deep into AI research or apply AI to solve real-world problems, Washington’s universities offer the resources, expertise, and opportunities you need to succeed in this rapidly evolving field.
0 notes
Text
There was a post I saw a while ago about the gap between comp-sci/engineering students’ aspirations towards knowledge in their field vs those of their professors. Basically, students in other fields like physics or EECS are trying to master the foundations of their field, while comp-sci and comp-engineering students just want to churn out code without a deeper understanding of why things work the way they do.
And yes completely agree. It’s like. If mechanical engineers didn’t know Newton’s laws, or an electrical engineer said shit like ‘Maxwell’s equations? Never heard of them.’ Not everyone will be a researcher of course, but cmon. Know your shit. I think the lack of that foundation in computer science students contributes to the shoddy state of modern software.
0 notes
Text
Interactive mouthpiece opens new opportunities for health data, assistive technology, and hands-free interactions
New Post has been published on https://thedigitalinsider.com/interactive-mouthpiece-opens-new-opportunities-for-health-data-assistive-technology-and-hands-free-interactions/
Interactive mouthpiece opens new opportunities for health data, assistive technology, and hands-free interactions
When you think about hands-free devices, you might picture Alexa and other voice-activated in-home assistants, Bluetooth earpieces, or asking Siri to make a phone call in your car. You might not imagine using your mouth to communicate with other devices like a computer or a phone remotely.
Thinking outside the box, MIT Computer Science and Artificial Intelligence Laboratory (CSAIL) and Aarhus University researchers have now engineered “MouthIO,” a dental brace that can be fabricated with sensors and feedback components to capture in-mouth interactions and data. This interactive wearable could eventually assist dentists and other doctors with collecting health data and help motor-impaired individuals interact with a phone, computer, or fitness tracker using their mouths.
Resembling an electronic retainer, MouthIO is a see-through brace that fits the specifications of your upper or lower set of teeth from a scan. The researchers created a plugin for the modeling software Blender to help users tailor the device to fit a dental scan, where you can then 3D print your design in dental resin. This computer-aided design tool allows users to digitally customize a panel (called PCB housing) on the side to integrate electronic components like batteries, sensors (including detectors for temperature and acceleration, as well as tongue-touch sensors), and actuators (like vibration motors and LEDs for feedback). You can also place small electronics outside of the PCB housing on individual teeth.
Play video
MouthIO: Fabricating Customizable Oral User Interfaces with Integrated Sensing and Actuation Video: MIT CSAIL
The active mouth
“The mouth is a really interesting place for an interactive wearable and can open up many opportunities, but has remained largely unexplored due to its complexity,” says senior author Michael Wessely, a former CSAIL postdoc and senior author on a paper about MouthIO who is now an assistant professor at Aarhus University. “This compact, humid environment has elaborate geometries, making it hard to build a wearable interface to place inside. With MouthIO, though, we’ve developed a new kind of device that’s comfortable, safe, and almost invisible to others. Dentists and other doctors are eager about MouthIO for its potential to provide new health insights, tracking things like teeth grinding and potentially bacteria in your saliva.”
The excitement for MouthIO’s potential in health monitoring stems from initial experiments. The team found that their device could track bruxism (the habit of grinding teeth) by embedding an accelerometer within the brace to track jaw movements. When attached to the lower set of teeth, MouthIO detected when users grind and bite, with the data charted to show how often users did each.
Wessely and his colleagues’ customizable brace could one day help users with motor impairments, too. The team connected small touchpads to MouthIO, helping detect when a user’s tongue taps their teeth. These interactions could be sent via Bluetooth to scroll across a webpage, for example, allowing the tongue to act as a “third hand” to open up a new avenue for hands-free interaction.
“MouthIO is a great example how miniature electronics now allow us to integrate sensing into a broad range of everyday interactions,” says study co-author Stefanie Mueller, the TIBCO Career Development Associate Professor in the MIT departments of Electrical Engineering and Computer Science and Mechanical Engineering and leader of the HCI Engineering Group at CSAIL. “I’m especially excited about the potential to help improve accessibility and track potential health issues among users.”
Molding and making MouthIO
To get a 3D model of your teeth, you can first create a physical impression and fill it with plaster. You can then scan your mold with a mobile app like Polycam and upload that to Blender. Using the researchers’ plugin within this program, you can clean up your dental scan to outline a precise brace design. Finally, you 3D print your digital creation in clear dental resin, where the electronic components can then be soldered on. Users can create a standard brace that covers their teeth, or opt for an “open-bite” design within their Blender plugin. The latter fits more like open-finger gloves, exposing the tips of your teeth, which helps users avoid lisping and talk naturally.
This “do it yourself” method costs roughly $15 to produce and takes two hours to be 3D-printed. MouthIO can also be fabricated with a more expensive, professional-level teeth scanner similar to what dentists and orthodontists use, which is faster and less labor-intensive.
Compared to its closed counterpart, which fully covers your teeth, the researchers view the open-bite design as a more comfortable option. The team preferred to use it for beverage monitoring experiments, where they fabricated a brace capable of alerting users when a drink was too hot. This iteration of MouthIO had a temperature sensor and a monitor embedded within the PCB housing that vibrated when a drink exceeded 65 degrees Celsius (or 149 degrees Fahrenheit). This could help individuals with mouth numbness better understand what they’re consuming.
In a user study, participants also preferred the open-bite version of MouthIO. “We found that our device could be suitable for everyday use in the future,” says study lead author and Aarhus University PhD student Yijing Jiang. “Since the tongue can touch the front teeth in our open-bite design, users don’t have a lisp. This made users feel more comfortable wearing the device during extended periods with breaks, similar to how people use retainers.”
The team’s initial findings indicate that MouthIO is a cost-effective, accessible, and customizable interface, and the team is working on a more long-term study to evaluate its viability further. They’re looking to improve its design, including experimenting with more flexible materials, and placing it in other parts of the mouth, like the cheek and the palate. Among these ideas, the researchers have already prototyped two new designs for MouthIO: a single-sided brace for even higher comfort when wearing MouthIO while also being fully invisible to others, and another fully capable of wireless charging and communication.
Jiang, Mueller, and Wessely’s co-authors include PhD student Julia Kleinau, master’s student Till Max Eckroth, and associate professor Eve Hoggan, all of Aarhus University. Their work was supported by a Novo Nordisk Foundation grant and was presented at ACM’s Symposium on User Interface Software and Technology.
#3-D printing#3d#3D model#Accessibility#alexa#app#artificial#Artificial Intelligence#Assistive technology#author#Bacteria#batteries#bluetooth#box#Capture#career#career development#communication#complexity#computer#Computer Science#Computer Science and Artificial Intelligence Laboratory (CSAIL)#Computer science and technology#data#dental#Design#development#devices#do it yourself#Electrical engineering and computer science (EECS)
2 notes
·
View notes
Text
Celebrating Amazing Women for the Month of March: Limor Fried
Celebrating Inspirational Women throughout the month of March.
Limor “Ladyada” Fried is an American Electrical Engineering and Computer Scientist as well as an entrepreneur and founder of Adafruit.
Limor Fried is an engineer, open source hardware and software pioneer, and entrepreneur. She earned a bachelor’s degree in electrical engineering and computer science (EECS) and a master’s degree in EECS at MIT.
She founded the educational electronics company Adafruit from her dorm room at MIT in 2005! She had the idea to create the best place online for learning electronics and making the best-designed products for makers of all ages and skill levels.
Adafruit has grown to over 100+ employees in the heart of New York City and has expanded offerings to include tools, equipment, and electronics that Limor personally selects, tests, and approves before going into the Adafruit store.
Fried was the first female engineer to appear on the cover of WIRED and named a White House Champion of Change in 2016. In 2018 Limor became one of “America’s Top 50 Women in Tech” by Forbes magazine.
R. J. Davies
A Riveting Jacked-In Dreamy Mind-Bender
RJ Davies - Science Fiction Author, Maddox Files, Novels
#R. J. Davies#R. J. Davies Author#Rhonda Davies#Rhonda Davies Author#Rhonda Joan Davies#mystery author#science fiction author#Author of Maddox Files#celebrating International Women's Day
0 notes
Text

The grading system known as “mastery learning” seeks to facilitate a process that gives students more room to learn from their mistakes. The idea is that students can achieve thorough proficiency in a subject if they are given enough time.
Armando Fox and Dan Garcia, professors of electrical engineering and computer sciences (EECS), are behind UC Berkeley’s pilot run in the non-majors CS 10 class, an endeavor they’ve dubbed “A’s for All (as Time and Interest Allow).”
In a paper, the Berkeley team found that “one of the best predictors of student performance in our rigorous introductory CS courses is prior CS exposure.” The implementation in an introductory course has the potential to improve diversity in the program.
Read our full story.
#berkeley#engineering#science#university#uc berkeley#bay area#college student#computer science#grading#education#equity#inclusion
0 notes
Text
Breaking the scaling limits of analog computing
See on Scoop.it - Design, Science and Technology
A new technique greatly reduces the error in an optical neural network, which uses light to process data instead of electrical signals. With their technique, the larger an optical neural network becomes, the lower the error in its computations. This could enable them to scale these devices up so they would be large enough for commercial uses.
As machine-learning models become larger and more complex, they require faster and more energy-efficient hardware to perform computations. Conventional digital computers are struggling to keep up.
An analog optical neural network could perform the same tasks as a digital one, such as image classification or speech recognition, but because computations are performed using light instead of electrical signals, optical neural networks can run many times faster while consuming less energy.
However, these analog devices are prone to hardware errors that can make computations less precise. Microscopic imperfections in hardware components are one cause of these errors. In an optical neural network that has many connected components, errors can quickly accumulate.
Even with error-correction techniques, due to fundamental properties of the devices that make up an optical neural network, some amount of error is unavoidable. A network that is large enough to be implemented in the real world would be far too imprecise to be effective.
MIT researchers have overcome this hurdle and found a way to effectively scale an optical neural network. By adding a tiny hardware component to the optical switches that form the network’s architecture, they can reduce even the uncorrectable errors that would otherwise accumulate in the device.
Their work could enable a super-fast, energy-efficient, analog neural network that can function with the same accuracy as a digital one. With this technique, as an optical circuit becomes larger, the amount of error in its computations actually decreases.
“This is remarkable, as it runs counter to the intuition of analog systems, where larger circuits are supposed to have higher errors, so that errors set a limit on scalability. This present paper allows us to address the scalability question of these systems with an unambiguous ‘yes,’” says lead author Ryan Hamerly, a visiting scientist in the MIT Research Laboratory for Electronics (RLE) and Quantum Photonics Laboratory and senior scientist at NTT Research.
Hamerly’s co-authors are graduate student Saumil Bandyopadhyay and senior author Dirk Englund, an associate professor in the MIT Department of Electrical Engineering and Computer Science (EECS), leader of the Quantum Photonics Laboratory, and member of the RLE. The research is published today in Nature Communications.
Read the full article at: news.mit.edu
0 notes
Text
AI model speeds up high-resolution computer vision
The system could improve image quality in video streaming or help autonomous vehicles identify road hazards in real-time.
Adam Zewe | MIT News
Researchers from MIT, the MIT-IBM Watson AI Lab, and elsewhere have developed a more efficient computer vision model that vastly reduces the computational complexity of this task. Their model can perform semantic segmentation accurately in real-time on a device with limited hardware resources, such as the on-board computers that enable an autonomous vehicle to make split-second decisions.
youtube
Recent state-of-the-art semantic segmentation models directly learn the interaction between each pair of pixels in an image, so their calculations grow quadratically as image resolution increases. Because of this, while these models are accurate, they are too slow to process high-resolution images in real time on an edge device like a sensor or mobile phone.
The MIT researchers designed a new building block for semantic segmentation models that achieves the same abilities as these state-of-the-art models, but with only linear computational complexity and hardware-efficient operations.
The result is a new model series for high-resolution computer vision that performs up to nine times faster than prior models when deployed on a mobile device. Importantly, this new model series exhibited the same or better accuracy than these alternatives.
Not only could this technique be used to help autonomous vehicles make decisions in real-time, it could also improve the efficiency of other high-resolution computer vision tasks, such as medical image segmentation.
“While researchers have been using traditional vision transformers for quite a long time, and they give amazing results, we want people to also pay attention to the efficiency aspect of these models. Our work shows that it is possible to drastically reduce the computation so this real-time image segmentation can happen locally on a device,” says Song Han, an associate professor in the Department of Electrical Engineering and Computer Science (EECS), a member of the MIT-IBM Watson AI Lab, and senior author of the paper describing the new model.
He is joined on the paper by lead author Han Cai, an EECS graduate student; Junyan Li, an undergraduate at Zhejiang University; Muyan Hu, an undergraduate student at Tsinghua University; and Chuang Gan, a principal research staff member at the MIT-IBM Watson AI Lab. The research will be presented at the International Conference on Computer Vision.
A simplified solution
Categorizing every pixel in a high-resolution image that may have millions of pixels is a difficult task for a machine-learning model. A powerful new type of model, known as a vision transformer, has recently been used effectively.
Transformers were originally developed for natural language processing. In that context, they encode each word in a sentence as a token and then generate an attention map, which captures each token’s relationships with all other tokens. This attention map helps the model understand context when it makes predictions.
Using the same concept, a vision transformer chops an image into patches of pixels and encodes each small patch into a token before generating an attention map. In generating this attention map, the model uses a similarity function that directly learns the interaction between each pair of pixels. In this way, the model develops what is known as a global receptive field, which means it can access all the relevant parts of the image.
Since a high-resolution image may contain millions of pixels, chunked into thousands of patches, the attention map quickly becomes enormous. Because of this, the amount of computation grows quadratically as the resolution of the image increases.
In their new model series, called EfficientViT, the MIT researchers used a simpler mechanism to build the attention map — replacing the nonlinear similarity function with a linear similarity function. As such, they can rearrange the order of operations to reduce total calculations without changing functionality and losing the global receptive field. With their model, the amount of computation needed for a prediction grows linearly as the image resolution grows.
“But there is no free lunch. The linear attention only captures global context about the image, losing local information, which makes the accuracy worse,” Han says.
To compensate for that accuracy loss, the researchers included two extra components in their model, each of which adds only a small amount of computation.
One of those elements helps the model capture local feature interactions, mitigating the linear function’s weakness in local information extraction. The second, a module that enables multiscale learning, helps the model recognize both large and small objects.
“The most critical part here is that we need to carefully balance the performance and the efficiency,” Cai says.
They designed EfficientViT with a hardware-friendly architecture, so it could be easier to run on different types of devices, such as virtual reality headsets or the edge computers on autonomous vehicles. Their model could also be applied to other computer vision tasks, like image classification.
Keep reading.
Make sure to follow us on Tumblr!
#artificial intelligence#machine learning#computer vision#autonomous vehicles#internet of things#research#Youtube
2 notes
·
View notes