#Data science definition and scope
Explore tagged Tumblr posts
Text
What is Data Science? Introduction, Basic Concepts & Process
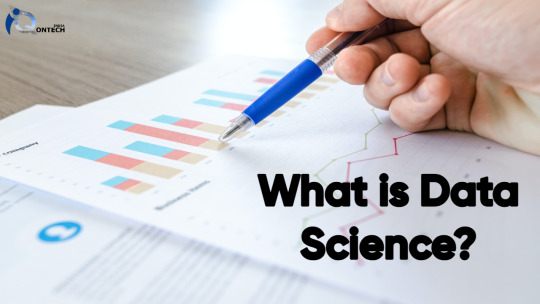
what is data science? Complete information about data science for beginner to advance you search what is data science data science is like data analyzing, data saving, database etc.
#what is data science#Data science definition and scope#Importance of data science#Data science vs. data analytics#Data science applications and examples#Skills required for a data scientist#Data science job prospects and salary#Data science tools and technologies#Data science algorithms and models#Difference between data science and machine learning#Data science interview questions and answers
1 note
·
View note
Text
I read a lot of science fiction novels, especially near-future hard-ish sci fi about first contact. It’s one of my favorite genres.
It also absolutely underlines for me why scientific inquiry MUST be a team effort. The authors will, if they’re doing their job well, present a bunch of scientific evidence connected to a new phenomenon. Perhaps the MC will do a few rounds of different experiments, and then come to a conclusion at the end. Or sometimes they’ll experiment, theorize, experiment, theorize, experiment, theorize, etc. Sometimes they’ll even explain in detail why some logical conclusion actually isn’t correct (suck it nerdboys I’m ahead of you*).
I’m a social scientist, a linguist with a background in gender studies and cultural studies who works as a second language instructor. I have a pretty good grasp of the scientific concepts these books like to play with, but they aren’t my specialty. So when they are doing these experiments, I am coming to *different conclusions* and wanting them to *conduct different experiments*. “Your evidence could be explained by these three other models!” I scream in the group discord. “Your conclusions aren’t fully supported, you need to do more tests!” “Not only are your postulates Terracentric, they’re Anglocentric! There are other cultures on our own damn planet that exhibit this ‘unexplainable alien behavior’!!!”
And that’s a perfectly valid plot point for a lone scientist, that their myopic view is narrowing what they can see of the world and therefore limiting the scope of the data they collect and causing them to draw questionable conclusions. The problem is that the authors tend to then have them be correct about everything they theorized. They did the science and now we’re done and we can move on to the plot. Meanwhile I’m either bitching “an anthropologist and an ecologist would have wildly different takes on this???” Or (looking at you, Arrival), “why is a fucking translator of a previously-studied language doing this work at all? Why don’t we have a rogue formalist syntactician who studies signed languages? Or a fieldworker doing documentation and description in South India, Papua New Guinea, or the Amazon River Basin? Or all of them in a room together?”
This is one of the reasons that I enjoy Brandon Sanderson novels so much, I think. Sure, every single one of them has the same plot twist: “your [physical/magical/political/interpersonal/historical/cosmological] model of the world is wrong, the truth is _____.” But that definitely fulfills my itch for theoretical models of the world to actually work like models instead of laws. Contemporary descriptions of the world may match the results of experiments, but that doesn’t mean that they’re necessarily correct in their totality. Time To Orbit: Unknown by @derinthescarletpescatarian is also pretty good at having the characters come to conclusions with limited data and then facing the consequences of that.
This isn’t a full thought, just something that occurs to me frequently when reading new sci-fi. Put your scientists in teams so they can think of different questions and supply different answers. So that I don’t have to yell at the MCs all alone.
*Andy Weir in Project Hail Mary came across as particularly defensive in his scientific explanations, but never about the things that I was questioning.
12 notes
·
View notes
Note
I've been offered a job helping someone with their dissertation for their PhD by doing data gathering or something similar, but I have zero experience as I am still in high school. I'd like to take the job as experience is incredibly important; however, I feel totally unqualified. I got the job offer solely because they were surprised I had interest in the topic and really need assistance. Any advice?
I'll be honest, this sounds a little weird to me -- not due to anything you've done, and I think you're right to ask someone else what they think. But just speaking as someone who has done a PhD, if I felt pressed to turn to a random high schooler for assistance in doing my research, I would take that as a pretty clear sign that I shouldn't be doing that degree. That might be their own problem, but still.
Now, if it's something like where you would interview people or collect raw data somehow, that might be a little more appropriate (I'm not sure, obviously, what the field of study is, but the social sciences, etc have topics where this might be more relevant). But I would still feel that if they're so desperate for help that they're asking you, they need to have a talk with their advisor and decide whether the scope of their project is realistic and/or something they can handle. Again, not something for you to get involved in, but you could at least have it in mind as a suggestion.
If you do decide to help -- is this someone you know well and trust? Are they willing to compensate you for your time, effort, and knowledge? You might be a high schooler, but that doesn't mean you should be expected to work for free. Likewise, you don't want to end up in a situation where you're doing a lot of their work for them longterm, since that is ethically squirrelly and reflects a systemic/structural problem with what they themselves are trying to do.
As a starting point, I would suggest a one-off meeting with them, where you lay out these questions, hear what they expect from you, and maybe give them some of your resources/suggestions for where you learned about that topic. It doesn't need to be any more than that, and you definitely should not commit to anything until you're a little clearer about what exactly they are asking. Because again, it goes without saying that they are the one who should be doing this work, rather than farming it out to you. You certainly can help in some way if you do feel comfortable with it, and the only way to get experience is to start working, so I don't want to discourage you out of hand. But I just want to make sure you are in control of this and know what questions to ask and they aren't expecting you to gather the bulk of their own data for them for free, or anything else like that. So yes.
17 notes
·
View notes
Text
Why Do So Many Big Data Projects Fail?
In our business analytics project work, we have often come in after several big data project failures of one kind or another. There are many reasons for this. They generally are not because of unproven technologies that were used because we have found that many new projects involving well-developed technologies fail. Why is this? Most surveys are quick to blame the scope, changing business requirements, lack of adequate skills etc. Based on our experience to date, we find that there are key attributes leading to successful big data initiatives that need to be carefully considered before you start a project. The understanding of these key attributes, below, will hopefully help you to avoid the most common pitfalls of big data projects.
Key attributes of successful Big Data projects
Develop a common understanding of what big data means for you
There is often a misconception of just what big data is about. Big data refers not just to the data but also the methodologies and technologies used to store and analyze the data. It is not simply “a lot of data”. It’s also not the size that counts but what you do with it. Understanding the definition and total scope of big data for your company is key to avoiding some of the most common errors that could occur.
Choose good use cases
Avoid choosing bad use cases by selecting specific and well defined use cases that solve real business problems and that your team already understand well. For example, a good use case could be that you want to improve the segmentation and targeting of specific marketing offers.
Prioritize what data and analytics you include in your analysis
Make sure that the data you’re collecting is the right data. Launching into a big data initiative with the idea that “We’ll just collect all the data that we can, and work out what to do with it later” often leads to disaster. Start with the data you already understand and flow that source of data into your data lake instead of flowing every possible source of data to the data lake.
Then next layer in one or two additional sources to enrich your analysis of web clickstream data or call centre text. Your cross-functional team can meet quarterly to prioritize and select the right use cases for implementation. Realize that it takes a lot of effort to import, clean and organize each data source.
Include non-data science subject matter experts (SMEs) in your team
Non-data science SMEs are the ones who understand their fields inside and out. They provide a context that allows you to understand what the data is saying. These SMEs are what frequently holds big data projects together. By offering on-the-job data science training to analysts in your organization interested in working in big data science, you will be able to far more efficiently fill project roles internally over hiring externally.
Ensure buy-in at all levels and good communication throughout the project
Big data projects need buy-in at every level, including senior leadership, middle management, nuts and bolts techies who will be carrying out the analytics and the workers themselves whose tasks will be affected by the results of the big data project. Everyone needs to understand what the big data project is doing and why? Not everyone needs to understand the ins and outs of the technical algorithms which may be running across the distributed, unstructured data that is analyzed in real time. But there should always be a logical, common-sense reason for what you are asking each member of the project team to do in the project. Good communication makes this happen.
Trust
All team members, data scientists and SMEs alike, must be able to trust each other. This is all about psychological safety and feeling empowered to contribute.
Summary
Big data initiatives executed well delivers significant and quantifiable business value to companies that take the extra time to plan, implement and roll out. Big data changes the strategy for data-driven businesses by overcoming barriers to analyzing large amounts of data, different types of unstructured and semi-structured data, and data that requires quick turnaround on results.
Being aware of the attributes of success above for big data projects would be a good start to making sure your big data project, whether it is your first or next one, delivers real business value and performance improvements to your organization.
#BigData#BigDataProjects#DataAnalytics#BusinessAnalytics#DataScience#DataDriven#ProjectSuccess#DataStrategy#DataLake#UseCases#BusinessValue#DataExperts
0 notes
Text
Unraveling the Distinctions Between Data Science and Big Data
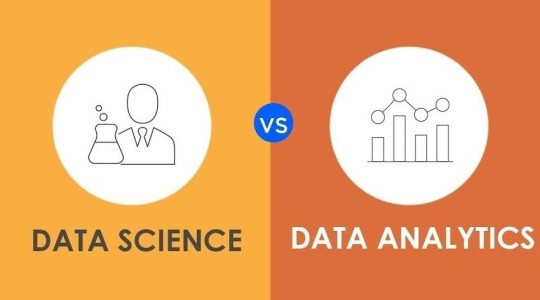
In the world of modern technology, two terms that often come up are "Data science" and "big data." While they are frequently used interchangeably, they refer to different concepts that, although interconnected, have distinct purposes and processes. Understanding the differences—and overlaps—between data science and big data is crucial for anyone looking to dive into the field of Data science or explore new technologies. In this blog, we’ll break down what data science and big data are, how they differ, and where they overlap.
What is Data science?
Definition and Scope of Data Science Data science is the discipline that combines statistical analysis, machine learning, data mining, and programming to extract insights from structured and unstructured data. It is a broad field that encompasses techniques for data exploration, predictive modeling, and the communication of findings. Data scientists use various tools and techniques to analyze data, make predictions, and support decision-making across different industries such as healthcare, finance, marketing, and entertainment.
Key Components of Data Science
Data Collection: Gathering relevant data from various sources.
Data Cleaning: Preparing the data for analysis by removing inconsistencies, missing values, or outliers.
Exploratory Data Analysis (EDA): Analyzing and visualizing data to uncover patterns and relationships.
Machine Learning Models: Building models to predict future outcomes based on data.
Data Visualization: Presenting data insights through graphs, charts, and dashboards to inform decision-making.
Primary Focus of Data Science The primary goal of data science is to derive meaningful insights from data, which can lead to improved decision-making, business strategies, or scientific advancements. Data scientists often work with smaller datasets (though not always) and are skilled in selecting appropriate algorithms and methods to analyze the data.
What is Big Data?
Definition and Scope of Big Data Big data refers to extremely large datasets that are difficult to manage, process, and analyze using traditional data processing tools. The volume, velocity, and variety of big data make it challenging to work with using conventional data management systems. Big data often comes from diverse sources such as social media, IoT devices, web logs, and transactional systems.
The "3 Vs" of Big Data
Volume: Refers to the massive amounts of data generated every second. This can be from billions of social media posts, transaction logs, sensor readings, and much more.
Velocity: Describes the speed at which data is generated and must be processed. For example, real-time data from sensors or social media platforms requires quick processing.
Variety: Refers to the diverse types of data, including structured (databases), semi-structured (XML files), and unstructured (videos, images, social media content) data.
Primary Focus of Big Data Big data focuses on the technologies and infrastructure needed to store, process, and analyze large volumes of data. It requires specialized tools and platforms such as Hadoop, Spark, and NoSQL databases that are designed to handle vast amounts of unstructured and semi-structured data. The focus is on scalability, storage, and ensuring that data can be accessed and processed efficiently.
Key Differences Between Data science and Big Data:
Scope of Work
Data Science: Primarily focused on analyzing and interpreting data to extract insights. It involves applying statistical and machine learning techniques to smaller, more manageable datasets.
Big Data: Concerned with managing and processing enormous volumes of data quickly. It involves specialized tools and systems to handle data that is too large to be processed by conventional methods.
Tools and Technologies
Data Science: Data scientists use tools like Python, R, SQL, and machine learning libraries (e.g., TensorFlow, Scikit-learn) to analyze data. Data visualization tools like Tableau or Power BI are also commonly used for presenting insights.
Big Data: Big data technologies focus on tools for data storage, processing, and analysis at scale. Common tools include Hadoop, Apache Spark, MongoDB, and Cassandra, which are built to handle vast amounts of unstructured or semi-structured data.
Focus on Data
Data Science: Focuses on deriving actionable insights from data using analytical techniques, irrespective of the size of the dataset.
Big Data: Focuses on handling and processing large and complex datasets, often from multiple sources, to enable large-scale analytics.
Data Handling and Processing
Data Science: Works with both structured and unstructured data but is often more focused on preparing and cleaning the data for analysis. The data handled is generally more manageable in terms of size.
Big Data: Works with large-scale, complex datasets that require distributed computing and specialized storage systems for processing. Big data technologies are built to process and store data at scale.
Where Data Science and Big Data Overlap:
Data Analytics Both data science and big data rely on the power of data analytics to derive insights. While data science focuses on applying algorithms and models to interpret data, big data is more concerned with how to store, manage, and process massive datasets for analysis. However, with the increasing availability of big data, data scientists are often required to work with big data platforms to analyze large datasets.
Machine Learning and Predictive Modeling Data scientists may use machine learning algorithms to analyze big data. With the help of tools like Apache Spark and Hadoop, machine learning models can be applied to large datasets for predictive analytics. As big data becomes more common in industries like e-commerce and healthcare, the need for data scientists to work with big data tools and technologies grows.
Business Intelligence Both data science and big data contribute to business intelligence efforts. Big data provides the infrastructure for collecting, storing, and processing vast amounts of data, while data science applies statistical and machine learning techniques to extract actionable insights. The combination of both enables organizations to make data-driven decisions and create competitive strategies.
Applications of Data science and Big Data Together:
Healthcare In healthcare, big data is used to store and process large volumes of patient data from various sources like electronic health records, wearables, and genetic data. Data scientists use machine learning models on this data to predict patient outcomes, optimize treatments, and improve overall healthcare delivery.
Retail and E-commerce Retailers use big data to track customer purchases, web interactions, and social media activity. Data scientists analyze this data to predict consumer behavior, personalize marketing campaigns, and optimize inventory management.
Finance In finance, big data enables the collection and storage of massive amounts of transaction and market data. Data scientists use this data to build predictive models for risk assessment, fraud detection, and portfolio optimization.
Conclusion:
Data science and big data are interconnected but serve different purposes. Data science focuses on analyzing data to derive meaningful insights, while big data is concerned with the infrastructure needed to handle and process vast datasets. Understanding the distinctions and overlaps between these two concepts is essential for anyone interested in the field of data analytics. By leveraging both data science and big data, organizations can unlock the full potential of their data, enabling more informed decision-making, predictive insights, and innovation.
0 notes
Text
Title: Unlocking Insights: A Comprehensive Guide to Data Science
Introduction
Overview of Data Science: Define data science and its importance in today’s data-driven world. Explain how it combines statistics, computer science, and domain expertise to extract meaningful insights from data.
Purpose of the Guide: Outline what readers can expect to learn, including key concepts, tools, and applications of data science.
Chapter 1: The Foundations of Data Science
What is Data Science?: Delve into the definition and scope of data science.
Key Concepts: Introduce core concepts like big data, data mining, and machine learning.
The Data Science Lifecycle: Describe the stages of a data science project, from data collection
to deployment.
Chapter 2: Data Collection and Preparation
Data Sources: Discuss various sources of data (structured vs. unstructured) and the importance of data quality.
Data Cleaning: Explain techniques for handling missing values, outliers, and inconsistencies.
Data Transformation: Introduce methods for data normalization, encoding categorical variables, and feature selection.
Chapter 3: Exploratory Data Analysis (EDA)
Importance of EDA: Highlight the role of EDA in understanding data distributions and relationships.
Visualization Tools: Discuss tools and libraries (e.g., Matplotlib, Seaborn, Tableau) for data visualization.
Statistical Techniques: Introduce basic statistical methods used in EDA, such as correlation analysis and hypothesis testing.
Chapter 4: Machine Learning Basics
What is Machine Learning?: Define machine learning and its categories (supervised, unsupervised, reinforcement learning).
Key Algorithms: Provide an overview of popular algorithms, including linear regression, decision trees, clustering, and neural networks.
Model Evaluation: Discuss metrics for evaluating model performance (e.g., accuracy, precision, recall) and techniques like cross-validation.
Chapter 5: Advanced Topics in Data Science
Deep Learning: Introduce deep learning concepts and frameworks (e.g., TensorFlow, PyTorch).
Natural Language Processing (NLP): Discuss the applications of NLP and relevant techniques (e.g., sentiment analysis, topic modeling).
Big Data Technologies: Explore tools and frameworks for handling large datasets (e.g., Hadoop, Spark).
Chapter 6: Applications of Data Science
Industry Use Cases: Highlight how various industries (healthcare, finance, retail) leverage data science for decision-making.
Real-World Projects: Provide examples of successful data science projects and their impact.
Chapter 7: Tools and Technologies for Data Science
Programming Languages: Discuss the significance of Python and R in data science.
Data Science Libraries: Introduce key libraries (e.g., Pandas, NumPy, Scikit-learn) and their functionalities.
Data Visualization Tools: Overview of tools used for creating impactful visualizations.
Chapter 8: The Future of Data Science
Trends and Innovations: Discuss emerging trends such as AI ethics, automated machine learning (AutoML), and edge computing.
Career Pathways: Explore career opportunities in data science, including roles like data analyst, data engineer, and machine learning engineer.
Conclusion
Key Takeaways: Summarize the main points covered in the guide.
Next Steps for Readers: Encourage readers to continue their learning journey, suggest resources (books, online courses, communities), and provide tips for starting their own data science projects.
Data science course in chennai
Data training in chennai
Data analytics course in chennai
0 notes
Text
Business Process Modeling: An Essential Skill for Business Analysts
BPM is a skill that every business analyst should have for driving efficiency and clarity in organizational operations. In today's quest by companies looking for all avenues to try and streamline their processes and boost productivity, the need for professionals who understand how to adopt BPM is definitely on the rise. Whether in a business analyst course or business analysis training, understanding how to model business processes is critical for delivering value to businesses.
Here are the key takeaways on why business process modeling is a core skill for business analysts:
1. Visualization of Processes:
The Process Modeling of a Business is the visualization of an organization's workflow, which makes complex processes easier to understand. It facilitates the business analyst in visualizing areas where there might be inefficiency, duplication, and scope for improvement. As a result, an analyst can thus outline steps that can be achieved with improved proposals in streamlining operations and business objectives better.
2. Improving Communication:
One of the most important benefits of BPM is improving communication among stakeholders. Properly defined, simple-to-understand visualizations of processes will allow technical and non-technical team members to understand the current and proposed workflows. It bridges gaps between departments and can help ensure people are on the same page-a prime requirement for successful project implementation.
3. Enabling Requirement Gathering:
Accurate models of business processes support the gathering requirements phase by providing a clear view of how things work in an organization at the process level. This enables business analysts to collect more precise and actionable requirements toward better solutions. Those enrolled in a business analyst course usually find that BPM techniques play a crucial role in gathering comprehensive business requirements.
4. Improving Decisioning:
Business Process Modeling is equally valuable for decision-makers as presentation of an excellent comprehension of the existing processes and their scope of improvement. Through a graphical representation, business managers can experience the effects of some changes intended, and thus, guide them to reach a decision-making quickly. With this aspect in mind, BPM constitutes an appropriate tool involved in any kind of business analysis course.
5. Facilitating Continuous Improvement:
A thoroughly documented business process model is supportive of continuous process improvement. Through this, businesses are better positioned to remain agile and responsive to changing environments; a skill set with which business analysts must continue to develop in order to stay relevant in today's fast-paced business environment.
To summarize, Business Process Modeling is more than a technical activity: it is actually an important part of business analysis. In BPM, business analysts can model, communicate, and optimize processes for huge extra benefits to business organizations. Future professionals may consider taking a Business Analyst Course or a business analysis course further down their career line to augment their skills in business process modeling.
Business name: ExcelR- Data Science, Data Analytics, Business Analytics Course Training Mumbai
Address: 304, 3rd Floor, Pratibha Building. Three Petrol pump, Lal Bahadur Shastri Rd, opposite Manas Tower, Pakhdi, Thane West, Thane, Maharashtra 400602
Phone: 09108238354
Email: [email protected]
0 notes
Text
ISO/IEC 17025: The Gold Standard for Testing and Calibration Laboratories
In the world of scientific testing and measurement, accuracy and reliability are paramount. ISO/IEC 17025, titled "General Requirements for the Competence of Testing and Calibration Laboratories," stands as the international benchmark for laboratories seeking to demonstrate their technical competence and the validity of their results. This standard is crucial in an era where data-driven decision-making impacts everything from product safety to environmental policy.
Scope and Significance: ISO 17025 applies to all organizations performing laboratory activities, regardless of the number of personnel or the extent of their testing and calibration scope. It covers testing, calibration, and sampling associated with subsequent testing and calibration. The standard's wide applicability makes it relevant across diverse sectors, including environmental testing, food analysis, forensic science, medical diagnostics, and industrial quality control.
Key Components of ISO/IEC 17025:
Impartiality and Confidentiality: The standard emphasizes the critical importance of laboratory impartiality and confidentiality. Laboratories must identify and address risks to their impartiality on an ongoing basis. They must also have robust procedures to protect the confidential information and proprietary rights of their clients.
Structural Requirements: ISO 17025 certification outlines the organizational structure necessary to ensure the laboratory's ability to maintain the quality of its results. This includes clear definition of responsibilities, reporting relationships, and the authority of key personnel. The standard requires laboratories to identify management that has overall responsibility for the technical operations and the provision of resources needed to ensure the quality of laboratory operations.
Resource Requirements: Personnel competence is a cornerstone of the standard. Laboratories must ensure that all personnel who can influence laboratory activities are competent to perform their assigned tasks. This involves ongoing training, supervision, and evaluation of competence.
The standard also addresses the physical resources needed for accurate testing and calibration. This includes suitable environmental conditions, proper equipment, and metrological traceability of measurements to stated references.
Process Requirements: ISO/IEC 17025 provides comprehensive guidelines for laboratory processes, from reviewing requests and contracts to reporting results. Key aspects include:
Method selection, verification, and validation
Sampling procedures
Technical records management
Evaluation of measurement uncertainty
Ensuring the validity of results through quality control procedures
Reporting of results, including clear rules for opinions and interpretations
Management System Requirements: The standard requires laboratories to implement a management system that supports and demonstrates the consistent fulfillment of the standard's requirements. This system must address document control, records management, actions to address risks and opportunities, improvement initiatives, corrective actions, internal audits, and management reviews.
Benefits of ISO/IEC 17025 Accreditation:
Technical Competence: Accreditation provides objective evidence of a laboratory's technical competence, giving customers confidence in the accuracy and reliability of test or calibration results.
International Recognition: Results from accredited laboratories are more readily accepted across national borders, facilitating international trade and regulatory compliance.
Risk Management: The standard's risk-based approach helps laboratories identify and mitigate potential issues before they impact results.
Continuous Improvement: Regular assessments and internal audits drive ongoing enhancement of laboratory operations and services.
Competitive Advantage: Accreditation can differentiate a laboratory in a competitive market, potentially leading to new business opportunities.
Legal Defense: In case of legal challenges, accreditation can serve as a strong defense of a laboratory's competence and the validity of its results. https://enhancequality.com/standards/iso-170252017-quality-management-systems-for-laboratories/
#ISO 17025 certification#iso 17025 accreditation#iso 17025 calibration#iso 17025 certification#iso 17025 requirements
0 notes
Text
Human-Computer Interaction: Designing Intuitive User Experiences
Human-computer interaction (HCI) is a multidisciplinary field that focuses on the design, evaluation, and implementation of interactive computing systems for human use. As technology continues to evolve, the importance of creating intuitive and user-friendly interfaces has become paramount. This exploration of HCI will cover its foundational principles, methodologies, applications, and future directions, emphasizing the significance of designing interfaces that enhance user experience.
Foundations of Human-Computer Interaction
Definition and Scope
HCI encompasses the study of how people interact with computers and other digital devices. It merges insights from various disciplines, including computer science, cognitive psychology, design, and social sciences, to improve the usability and accessibility of technology. The goal is to create systems that are not only functional but also enjoyable and efficient for users.
Historical Context
The field of HCI emerged in the 1980s, coinciding with the rise of personal computing. Early interfaces were command-line based, requiring users to memorize complex commands. The introduction of graphical user interfaces (GUIs) revolutionized interaction by allowing users to engage with visual elements like icons and menus, making technology more accessible to the general public. This shift laid the groundwork for ongoing advancements in interface design.
Importance of Intuitive and User-Friendly Interfaces
Enhancing User Experience
An intuitive interface enables users to navigate systems effortlessly, reducing the learning curve and minimizing frustration. Key aspects of user experience (UX) include:
Usability: Refers to how effectively users can achieve their goals using a system. A usable interface is easy to learn, efficient to use, and provides a satisfying experience.
Accessibility: Ensures that interfaces are usable by people with diverse abilities, including those with visual, auditory, or motor impairments. Designing for accessibility broadens the user base and promotes inclusivity.
User Satisfaction: A positive user experience fosters satisfaction and loyalty. Users are more likely to recommend products that meet their needs and expectations.
Economic Impact
User-friendly interfaces can significantly impact an organization’s bottom line. When users can easily navigate a system, productivity increases, and the need for extensive training decreases. Moreover, satisfied users are more likely to return and recommend the product, enhancing customer retention and brand reputation.
Methodologies in HCI Design
User-Centered Design (UCD)
User-centered design is a fundamental approach in HCI that prioritizes the needs and preferences of users throughout the design process. Key steps include:
User Research: Understanding the target audience through interviews, surveys, and observations to gather insights into their behaviors, needs, and pain points.
Prototyping: Developing low-fidelity (paper sketches) or high-fidelity (interactive digital models) prototypes to visualize design concepts and gather user feedback.
Usability Testing: Conducting tests with real users to identify usability issues and gather qualitative and quantitative data for iterative improvements.
Agile and Iterative Design
Agile methodologies promote flexibility and adaptability in design processes. By incorporating user feedback at multiple stages, designers can make continuous improvements, ensuring that the final product aligns with user expectations and requirements.
Heuristic Evaluation
This method involves experts evaluating a user interface against established usability principles (heuristics) to identify potential usability problems. Common heuristics include consistency, error prevention, and visibility of system status.
Applications of HCI
HCI principles are applied across various domains, enhancing user experience in numerous contexts:
Web and Mobile Applications
Designing user-friendly websites and mobile apps is critical for engagement and retention. Effective navigation, responsive design, and intuitive interactions are essential for meeting user expectations in these environments.
Virtual and Augmented Reality
HCI plays a significant role in the development of virtual reality (VR) and augmented reality (AR) applications. Designing immersive experiences requires an understanding of how users perceive and interact with digital environments, necessitating innovative interface solutions.
Healthcare Technology
In healthcare, HCI is vital for developing systems that improve patient care and streamline workflows. User-friendly electronic health records (EHR) systems, telemedicine platforms, and health monitoring devices enhance usability for both patients and healthcare professionals.
Smart Home Devices
The rise of the Internet of Things (IoT) has led to the proliferation of smart home devices. HCI principles guide the design of user interfaces for these devices, ensuring that users can easily control and monitor their environments.
Future Directions in HCI
As technology continues to advance, the field of HCI is poised for further evolution:
Artificial Intelligence and Machine Learning
Integrating AI and machine learning into HCI can lead to more personalized and adaptive interfaces. Systems that learn from user behavior can anticipate needs, streamline interactions, and enhance overall user experience.
Multimodal Interfaces
The development of multimodal interfaces, which combine various interaction methods (e.g., voice, touch, gesture), offers users flexibility in how they engage with technology. This approach caters to diverse preferences and enhances accessibility.
Ethical Considerations
As HCI evolves, ethical considerations surrounding user privacy, data security, and algorithmic bias become increasingly important. Designers must prioritize ethical practices to build trust and ensure that technology serves all users equitably.
Conclusion
Human-computer interaction is a vital field that shapes how users engage with technology. By focusing on designing intuitive and user-friendly interfaces, HCI enhances usability, accessibility, and user satisfaction. As technology Arya College of Engineering & I.T is the Best College in Jaipur that continues to advance, the principles and methodologies of HCI will play a crucial role in creating systems that meet the diverse needs of users, fostering a more inclusive and efficient digital landscape. The ongoing evolution of HCI promises exciting opportunities for innovation, ensuring that technology remains a valuable tool in our daily lives.
0 notes
Text
The Human Brain Project and AI: Exploring Innovations and Impacts
Discover the Human Brain Project's goals, AI's role, and the future of brain research. Explore breakthroughs, challenges, and practical applications.

Understanding the Human Brain Project and Artificial Intelligence
The Human Brain Project (HBP) is one of the most ambitious scientific endeavors of our time, aiming to simulate the human brain's functions through advanced computing. This groundbreaking project intersects significantly with artificial intelligence (AI), which plays a crucial role in its progress. This article explores the HBP, the integration of AI in its research, and the potential impacts on science and society.
What is the Human Brain Project?
Origins and Objectives
Launched in 2013, the Human Brain Project is a European initiative with the goal of simulating brain functions to better understand human cognition and brain disorders. The project seeks to map the brain's structure and function, paving the way for new insights into neurological conditions and enhancing our overall understanding of human intelligence.
Key Milestones and Achievements
Among its key achievements are the development of brain simulation models and the creation of extensive brain data repositories. These milestones mark significant progress in understanding how brain functions can be replicated digitally.
Current Status and Future Directions
Currently, the project is focused on refining its models and expanding its data collection methods. Future directions include enhancing the accuracy of simulations and integrating more comprehensive data sources to improve our understanding of complex brain functions.
The Role of Artificial Intelligence
Definition and Scope of AI
Artificial intelligence refers to the capability of a machine to imitate intelligent human behavior. In the context of the Human Brain Project, AI encompasses various technologies that aid in data analysis, pattern recognition, and brain simulation.
AI Technologies Used in Brain Research
AI techniques such as machine learning and neural networks are pivotal in analyzing vast amounts of brain data and developing accurate brain models. These technologies enable researchers to identify patterns and make predictions about brain functions.
Benefits of AI in Neuroscience
AI enhances the precision of brain simulations, accelerates data analysis, and provides new insights into brain disorders. Its ability to handle complex computations and large datasets makes it an invaluable tool in advancing brain research.
Key Components of the Human Brain Project
Brain Simulation Models
The HBP utilizes sophisticated brain simulation models to replicate brain activities and understand their underlying mechanisms. These models are crucial for studying brain functions and testing hypotheses about brain disorders.
Data Collection and Analysis Methods
Advanced techniques are employed to collect and analyze brain data, including neuroimaging and electrophysiological recordings. These methods provide detailed insights into brain structure and function.
Collaboration with Global Institutions
The HBP involves collaboration with numerous international research institutions, fostering a global effort to advance brain research. This collaborative approach enhances the project's scope and impact.
AI Technologies and Techniques
Machine Learning and Deep Learning
Machine learning algorithms are used to analyze brain data and develop predictive models. Deep learning, a subset of machine learning, involves training neural networks to recognize complex patterns in data.
Neural Networks and Their Applications
Neural networks simulate the brain's neural connections, aiding in the development of brain models and the interpretation of data. These networks are integral to understanding brain functions and disorders.
AI in Data Analysis and Pattern Recognition
AI's role in data analysis involves identifying trends and patterns in large datasets. This capability is crucial for making sense of the complex information gathered from brain research.
Case Studies and Applications
Notable Case Studies from the Project
Several case studies highlight the HBP's success, such as the development of detailed brain models for studying specific neurological conditions. These case studies demonstrate the project's impact on advancing brain research.
Real-World Applications of Research
The research outcomes have practical applications in developing new treatments for brain disorders and enhancing our understanding of cognitive processes. These applications highlight the project's relevance to real-world issues.
Impact on Healthcare and Cognitive Sciences
The HBP's findings contribute to advancements in healthcare, particularly in diagnosing and treating neurological conditions. The project also enriches our knowledge of cognitive sciences and brain functions.
Challenges and Limitations
Technical Challenges
The complexity of brain simulation and data analysis presents significant technical challenges. Issues such as computational limitations and data integration need to be addressed for further progress.
Ethical and Privacy Concerns
The use of sensitive brain data raises ethical and privacy concerns. Ensuring the protection of personal information and addressing ethical dilemmas are crucial for the project's success.
Future Hurdles and Solutions
Future hurdles include improving simulation accuracy and overcoming technical limitations. Solutions involve advancing technology and refining research methodologies.
Future Outlook
Emerging Trends in Brain Research
Emerging trends include the integration of more advanced AI technologies and the development of more detailed brain models. These trends are expected to drive further discoveries in brain research.
The Evolving Role of AI
AI's role in brain research will continue to expand, with new applications and technologies enhancing our understanding of the brain. This evolution will contribute to significant advancements in neuroscience.
Long-Term Impact on Science and Society
The long-term impact of the HBP is profound, with potential advancements in brain science leading to new treatments and technologies. The project's contributions will shape the future of neuroscience and its applications.
Practical Applications
How Findings May Influence AI Development
The findings from the HBP may influence AI development by providing insights into brain functions and improving AI models. This influence could lead to more advanced and accurate AI technologies.
Implications for Mental Health and Therapy
The research outcomes have implications for mental health, offering potential new therapies and treatments for neurological conditions. The project's insights could lead to significant improvements in mental health care.
Potential for New Technologies
The HBP's research may lead to the development of new technologies, such as advanced brain-computer interfaces and enhanced cognitive training tools. These innovations could have wide-ranging applications.
Conclusion
The Human Brain Project represents a monumental effort to simulate and understand the human brain. The integration of AI into this research enhances our ability to analyze data and develop brain models.
The project's future holds promise for advancing our understanding of the brain and developing new technologies. Continued collaboration and innovation will be essential for achieving its goals.
0 notes
Text
OLED: Revolutionizing Display Technology
Organic Light Emitting Diode (OLED) technology is transforming display systems with its superior color accuracy, contrast ratio, and energy efficiency. Unlike traditional LCDs, OLED panels do not require a backlight, as each pixel emits its own light, allowing for deeper blacks and more vibrant colors. OLED technology is widely used in various applications, including smartphones, televisions, and wearable devices. Its flexibility and thinness enable innovative design possibilities, such as curved and foldable displays. OLED's ability to deliver high-quality visuals and low power consumption is driving its adoption across multiple industries.
The OLED Market, valued at USD 48.19 billion in 2023, is projected to reach USD 239.6 billion by 2031, expanding at a CAGR of 22.5% from 2024 to 2031.
Future Scope:
The future of OLED technology will be characterized by advancements in material science, display resolution, and manufacturing techniques. Research into new organic materials and processes will enhance OLED performance, including brightness, longevity, and color accuracy. Innovations in flexible and transparent OLEDs will expand their application possibilities, enabling new product designs and form factors. As demand for high-definition and immersive displays grows, OLED technology will continue to evolve, offering improved performance and new capabilities for consumer electronics, automotive displays, and other applications.
Key Points:
OLED technology provides superior color accuracy, contrast ratio, and energy efficiency.
Each pixel emits its own light, eliminating the need for a backlight.
Future advancements will focus on material science, display resolution, and innovative form factors.
Trends:
Key trends in OLED technology include the increasing adoption of OLED displays in smartphones, televisions, and wearables due to their superior visual quality and energy efficiency. The development of flexible and foldable OLED displays is enabling new product designs and applications. Advances in OLED manufacturing techniques are improving display resolution and longevity. The growing demand for high-definition and immersive visual experiences is driving continuous innovation and expansion of OLED technology.
Application:
OLED technology is applied in various devices, including smartphones (for vibrant and responsive screens), televisions (for high contrast and color accuracy), and wearable devices (for flexible and lightweight displays). It is also used in automotive displays, signage, and lighting applications. The technology's ability to deliver high-quality visuals and adapt to different form factors makes it a versatile and sought-after solution across multiple industries.
Conclusion:
OLED technology is revolutionizing display systems with its exceptional color accuracy, contrast ratio, and energy efficiency. As advancements in materials, resolution, and manufacturing techniques continue, OLED will drive innovation in consumer electronics, automotive displays, and other applications. Its flexibility and high performance make OLED a leading technology in the display industry, offering improved visual experiences and enabling new product designs and capabilities.
Read More Details: https://www.snsinsider.com/reports/oled-market-4143
About Us:
SNS Insider is one of the leading market research and consulting agencies that dominates the market research industry globally. Our company’s aim is to give clients the knowledge they require in order to function in changing circumstances. In order to give you current, accurate market data, consumer insights, and opinions so that you can make decisions with confidence, we employ a variety of techniques, including surveys, video talks, and focus groups around the world.
Contact Us:
Akash Anand — Head of Business Development & Strategy
Email: [email protected]
Phone: +1–415–230–0044 (US) | +91–7798602273 (IND)
0 notes
Text
Machine learning or Data Science, which has a better future?
The future of data science and machine learning goes hand in hand; the prospects are great. Both areas are growing very fast, with enormous potential to disrupt a number of industries.
Data science, by definition, is an umbrella term that ranges from many techniques and methodologies for extracting useful insights or meaning from data. It consists of collecting, cleaning, analyzing, and interpreting data for driving informed decisions.
Machine learning is a subdomain of artificial intelligence that concerns the design of algorithms that allow computers to learn from data and to improve their performance on some particular task without being explicitly programmed. It is one of the important modules of data science and is normally used for predictive modeling and automation purposes.
While both are nice fields that may bring wonderful futures, the best one will depend on your interests and intended career goals.
If you like a broader scope of data analysis, data visualization, and storyboarding, then data science will be more appropriate. If you enjoy building algorithms and models that resolve really complex problems, then machine learning could be the most compelling path. At the end of the day, both areas are closely related and require solid statistics, programming, and problem-solving.
0 notes
Text
Transparent and Translucent Concrete Market Size, Share, Forecast [2032]
Transparent and Translucent Concrete Market provides in-depth analysis of the market state of Transparent and Translucent Concrete manufacturers, including best facts and figures, overview, definition, SWOT analysis, expert opinions, and the most current global developments. The research also calculates market size, price, revenue, cost structure, gross margin, sales, and market share, as well as forecasts and growth rates. The report assists in determining the revenue earned by the selling of this report and technology across different application areas.
Geographically, this report is segmented into several key regions, with sales, revenue, market share and growth Rate of Transparent and Translucent Concrete in these regions till the forecast period
North America
Middle East and Africa
Asia-Pacific
South America
Europe
Key Attentions of Transparent and Translucent Concrete Market Report:
The report offers a comprehensive and broad perspective on the global Transparent and Translucent Concrete Market.
The market statistics represented in different Transparent and Translucent Concrete segments offers complete industry picture.
Market growth drivers, challenges affecting the development of Transparent and Translucent Concrete are analyzed in detail.
The report will help in the analysis of major competitive market scenario, market dynamics of Transparent and Translucent Concrete.
Major stakeholders, key companies Transparent and Translucent Concrete, investment feasibility and new market entrants study is offered.
Development scope of Transparent and Translucent Concrete in each market segment is covered in this report. The macro and micro-economic factors affecting the Transparent and Translucent Concrete Market
Advancement is elaborated in this report. The upstream and downstream components of Transparent and Translucent Concrete and a comprehensive value chain are explained.
Browse More Details On This Report at @https://www.globalgrowthinsights.com/market-reports/transparent-and-translucent-concrete-market-100590
Global Growth Insights
Web: https://www.globalgrowthinsights.com
Our Other Reports:
Molded Foam Component Market MarketMarket
Rotogravure Printing Machine MarketMarket Share
Museum Software MarketMarket Growth Rate
RFID Smart Cabinets MarketMarket Forecast
Global Vertebroplasty And Kyphoplasty Devices MarketMarket Size
Inkjet Papers and Films MarketMarket Growth
Diabetic Macular Edema Treatment MarketMarket Analysis
Leadframe, Gold Wires and Packaging Materials for Semiconductor MarketMarket Size
Global Dental Plaster MarketMarket Share
Global Mead Beverages MarketMarket Growth
Laser Direct Structuring (LDS) Antenna MarketMarket
Biomass Boiler MarketMarket Share
RF-over-Fiber (RFoF) Solutions MarketMarket Growth Rate
Hydrographic Acquisition Software MarketMarket Forecast
Global Anesthesia Gas Evaporators MarketMarket Size
Industrial Wax MarketMarket Growth
Data Fabric MarketMarket Analysis
Cancer Vaccine MarketMarket Size
Global Tigecycline MarketMarket Share
Global Air Disinfection and Purification Machine MarketMarket Growth
Die-cast aluminum alloy MarketMarket
Automatic Identification and Data Capture MarketMarket Share
Period Panties (Menstrual Underwear) MarketMarket Growth Rate
Assistive Devices for Vulnerable Groups MarketMarket Forecast
Global Portable Gaming Console MarketMarket Size
Chromebook MarketMarket Growth
Infrared Detector MarketMarket Analysis
Demand Side Platforms (DSP) for Programmatic Advertising from the Mobile Side MarketMarket Size
Global Correlative Light Electron Microscopy (CLEM) for materials science (MS) MarketMarket Share
Global Forensic Technology MarketMarket Growth
Security Screening MarketMarket
Artificial Intelligence-Emotion Recognition MarketMarket Share
Bring-your-own-Device (BYOD) MarketMarket Growth Rate
Packaging Metallized Film MarketMarket Forecast
Global Neuromorphic Chip MarketMarket Size
Elliptical Waveguide Tools MarketMarket Growth
Child Backless Booster Seats MarketMarket Analysis
Hairline Powder MarketMarket Size
Global Popcorn Popper MarketMarket Share
Global 10G Laser Chips MarketMarket Growth
1 note
·
View note
Text
Become A Tech Expert With One Of The Best Private Engineering Colleges In Ranchi
For all engineering students, finding an outstanding university that offers an extraordinary curriculum, industry exposure, and the best private engineering colleges in ranchi. Amity University has risen as one of the top-ranked universities nationwide, and several factors have contributed to the growing demand for this prestigious university. Choosing Amity to pursue tech studies in professional programs like engineering is undoubtedly perfect.
Why is Amity the top engineering university in Ranchi?
Amity University Ranchi has worked hard to provide students with the greatest education possible and has established itself as one of Ranchi's leading engineering universities, with its expert staff, best infrastructure, and hi-tech laboratories. Let's look at the engineering degrees offered and the resources this prestigious university provides for its students:
Training and placement cell
The university works hard to build strong corporate connections that enable students to expand their career opportunities; for that, it has a dedicated placements cell that works day and night. The placement cell of the university organises various campus placement programs within the university itself:
Industry Interaction Placement Cell
Collaborative Research
Business-related leadership lessons
Development Programs
Amity offers various engineering courses for students to take up and build their careers in different technical fields. Below is the list of courses provided by Amity for Bachelor of Technology aspirants.
B.Tech. in Civil Engineering
B.Tech. in Electronics and Communication Engineering
B.Tech. in Mechanical Engineering
B.Tech. in Computer Science Engineering
B.Tech in Mechanical and Robotics, IoT
B.Tech. in Cloud Computing IoT and Blockchain
B.Tech. in Computer Science (Artificial Intelligence and Machine Learning)
B.Tech. in Electronics Engineering and VLSI Design
These technical courses have a very wide scope in the job market, and Amity stands out as the best because it facilitates students with various high in class facilities, which are as follows:
Auditoriums
Hi-Tech Labs
1000 Mbps WiFi
Conference Rooms
Digital Library
Air-Conditioned Classrooms
In addition to this, the university also facilitates students with global exposure, providing opportunities for students to build their careers, and Amity, being one of the top engineering colleges in Ranchi, introduces students to the vibrant learning environment of experiential learning and industry exposure to prepare students to master their skills and excel in the field of engineering.
Doors to various career opportunities open after graduation from Amity University
Students who have an interest in practical programming languages, software, and machines should definitely get into the higher education journey at Amity, one of the best private engineering colleges in Ranchi. With a diverse range of career options, more and more students have developed a strong interest in engineering. Let’s take a look at the major engineering career options:
Data Science and Analytics
Cybersecurity
Artificial Intelligence and Machine Learning
Design and Development
Cloud Support Engineer
Cloud Network Engineer
Amity University Ranchi has emerged as a promising destination for students who wish to make a bright career in engineering. Being one of the best private engineering colleges in Ranchi, this hub of learning wishes to deliver academic excellence to its students. For further details, visit https://amity.edu/ranchi/
Source: https://amityuniversityranchi.blogspot.com/2024/07/become-tech-expert-with-one-of-best.html
#Best private engineering colleges in ranchi#Engineering Colleges in Ranchi#Top Engineering University in Ranchi
0 notes
Text
Artificial Intelligence Foundation Course Overview

Artificial Intelligence (AI) is revolutionizing industries, transforming how businesses operate, and significantly impacting everyday life. Understanding AI’s fundamentals is crucial for anyone looking to advance their career in technology or enhance their business acumen. Our Artificial Intelligence Foundation Course provides a comprehensive introduction to AI, covering essential concepts, techniques, and applications that prepare learners for the rapidly evolving landscape of AI.
Course Objectives and Learning Outcomes
The primary goal of our Artificial Intelligence Foundation Course is to equip participants with a solid understanding of AI principles and practices. By the end of this course, participants will:
Understand the history and evolution of AI: Gain insights into the origins and development of AI, from early theories to modern advancements.
Grasp core AI concepts: Learn key terminologies and concepts, including machine learning, neural networks, deep learning, natural language processing, and robotics.
Develop practical AI skills: Acquire hands-on experience with AI tools and technologies, enabling you to implement AI solutions in real-world scenarios.
Explore AI applications: Discover how AI is applied across various industries, such as healthcare, finance, retail, and manufacturing.
Comprehensive Curriculum
Our course is meticulously designed to cover a wide array of topics, ensuring a thorough understanding of AI. The curriculum includes:
1. Introduction to Artificial Intelligence
Definition and Scope: What is AI? Understanding its scope and impact.
History of AI: Key milestones in the evolution of AI technology.
Types of AI: Narrow AI vs. General AI vs. Superintelligent AI.
2. Core Concepts and Techniques
Machine Learning: Supervised, unsupervised, and reinforcement learning.
Neural Networks: Structure and functioning of neural networks.
Deep Learning: Advanced techniques in deep learning, including convolutional neural networks (CNNs) and recurrent neural networks (RNNs).
Natural Language Processing (NLP): Understanding and processing human language.
Robotics and AI: Integrating AI with robotics for automation and innovation.
3. AI Tools and Technologies
Programming Languages: Introduction to Python and R for AI development.
AI Frameworks: Overview of popular AI frameworks such as TensorFlow, PyTorch, and Keras.
Data Management: Techniques for handling and processing large datasets.
Cloud Platforms: Utilizing cloud services like AWS, Google Cloud, and Azure for AI projects.
4. Practical Applications of AI
Healthcare: AI in medical imaging, diagnosis, and personalized medicine.
Finance: AI for fraud detection, algorithmic trading, and risk management.
Retail: Enhancing customer experience through AI-driven recommendations and inventory management.
Manufacturing: AI in predictive maintenance, quality control, and supply chain optimization.
5. Ethical Considerations and Future Trends
Ethics in AI: Addressing bias, fairness, and transparency in AI systems.
Future Trends: Emerging technologies and the future of AI.
Learning Methodology
Our course combines theoretical knowledge with practical experience, ensuring a well-rounded education. Key components of our learning methodology include:
Interactive Lectures: Engaging lectures delivered by industry experts.
Hands-on Projects: Real-world projects that provide practical experience.
Case Studies: In-depth analysis of successful AI implementations.
Group Discussions: Collaborative learning through group discussions and activities.
Assessments and Quizzes: Regular assessments to track progress and reinforce learning.
Target Audience
The Artificial Intelligence Foundation Course is designed for a diverse audience, including:
Students and Academics: Individuals pursuing studies in computer science, engineering, or related fields.
Professionals: IT professionals, data scientists, and engineers seeking to enhance their AI skills.
Business Leaders: Executives and managers looking to leverage AI for strategic decision-making.
Enthusiasts: Anyone with a keen interest in understanding AI and its potential.
Career Opportunities and Advancement
Completing our Artificial Intelligence Foundation Course opens up numerous career opportunities in various sectors. Some potential career paths include:
AI Engineer: Design and develop AI models and systems.
Data Scientist: Analyze and interpret complex data to inform decision-making.
Machine Learning Engineer: Build and deploy machine learning models.
AI Consultant: Provide strategic advice on AI adoption and implementation.
Research Scientist: Conduct cutting-edge research in AI and related fields.
Why Choose Our Course?
Expert Instructors
Our course is taught by industry veterans and academic experts with extensive experience in AI. Their insights and guidance ensure that participants receive the highest quality education.
Comprehensive Curriculum
We offer a well-rounded curriculum that covers both foundational concepts and advanced topics, ensuring that learners gain a thorough understanding of AI.
Practical Experience
Our emphasis on hands-on learning ensures that participants can apply their knowledge in real-world scenarios, making them job-ready upon course completion.
Flexible Learning
With both online and in-person options, our course is designed to accommodate different learning preferences and schedules, providing flexibility for busy professionals and students.
Supportive Community
Participants join a vibrant community of learners, providing opportunities for networking, collaboration, and continuous learning.
Enroll Today
Join our Artificial Intelligence Foundation Course and embark on a transformative learning journey. Equip yourself with the skills and knowledge to excel in the exciting field of AI. Enroll today and take the first step towards a promising future in AI.
0 notes
Text
Molecular Spectroscopy Market Size 2024 | Anticipating Growth, Trends and Advancements By 2031

The "Molecular Spectroscopy Market" is a dynamic and rapidly evolving sector, with significant advancements and growth anticipated by 2031. Comprehensive market research reveals a detailed analysis of market size, share, and trends, providing valuable insights into its expansion. This report delves into segmentation and definition, offering a clear understanding of market components and drivers. Employing SWOT and PESTEL analyses, the study evaluates the market's strengths, weaknesses, opportunities, and threats, alongside political, economic, social, technological, environmental, and legal factors. Expert opinions and recent developments highlight the geographical distribution and forecast the market's trajectory, ensuring a robust foundation for strategic planning and investment.
What is the projected market size & growth rate of the Molecular Spectroscopy Market?
Market Analysis and Size
Molecular Spectroscopy is a common analytical tool used in universities, research institutions, and academic laboratories worldwide. It is integrated into various scientific disciplines, including chemistry, physics, biology, materials science, and pharmaceutical sciences. Many universities and research institutions have dedicated spectroscopy laboratories equipped with a range of spectroscopic instruments.
Data Bridge Market Research analyses that the global molecular spectroscopy market which was USD 7,221.29 million in 2022, is expected to reach USD 12,688.57 million by 2030, and is expected to undergo a CAGR of 7.3% during the forecast period 2023-2030. This indicates the market value. “Nuclear Magnetic Resonance Spectroscopy” dominates the technology segment of the molecular spectroscopy market owing to the growing demand for better methods of treatment and diagnosis. In addition to the insights on market scenarios such as market value, growth rate, segmentation, geographical coverage, and major players, the market reports curated by the Data Bridge Market Research also include depth expert analysis, patient epidemiology, pipeline analysis, pricing analysis, and regulatory framework.
Browse Detailed TOC, Tables and Figures with Charts which is spread across 350 Pages that provides exclusive data, information, vital statistics, trends, and competitive landscape details in this niche sector.
This research report is the result of an extensive primary and secondary research effort into the Molecular Spectroscopy market. It provides a thorough overview of the market's current and future objectives, along with a competitive analysis of the industry, broken down by application, type and regional trends. It also provides a dashboard overview of the past and present performance of leading companies. A variety of methodologies and analyses are used in the research to ensure accurate and comprehensive information about the Molecular Spectroscopy Market.
Get a Sample PDF of Report - https://www.databridgemarketresearch.com/request-a-sample/?dbmr=global-molecular-spectroscopy-market
Which are the driving factors of the Molecular Spectroscopy market?
The driving factors of the Molecular Spectroscopy market include technological advancements that enhance product efficiency and user experience, increasing consumer demand driven by changing lifestyle preferences, and favorable government regulations and policies that support market growth. Additionally, rising investment in research and development and the expanding application scope of Molecular Spectroscopy across various industries further propel market expansion.
Molecular Spectroscopy Market - Competitive and Segmentation Analysis:
Global Molecular Spectroscopy Market, By Technology (Nuclear Magnetic Resonance Spectroscopy, UV-Visible Spectroscopy, Infrared Spectroscopy, Near-Infrared Spectroscopy, Colour Measurement Spectroscopy, Raman Spectroscopy, Other Technologies), Application (Pharmaceutical Applications, Food and Beverage Testing, Biotechnology and Biopharmaceutical Applications, Environmental Testing, Academic Research, Other Applications) – Industry Trends and Forecast to 2031.
How do you determine the list of the key players included in the report?
With the aim of clearly revealing the competitive situation of the industry, we concretely analyze not only the leading enterprises that have a voice on a global scale, but also the regional small and medium-sized companies that play key roles and have plenty of potential growth.
Which are the top companies operating in the Molecular Spectroscopy market?
Some of the major players operating in the global molecular spectroscopy market are:
Thermo Fisher Scientific Inc. (U.S.)
Bruker (U.S.)
PerkinElmer, Inc. (U.S.)
Agilent Technologies, Inc. (U.S.)
Eurofins Scientific (Luxembourg)
Danaher (U.S.)
Merck KGaA (Germany)
Siemens Healthcare GmbH (Germany)
Medtronic (Ireland)
ABB (Switzerland)
B&W Tek. (U.S.)
Digilab Inc. (U.S.)
Hamamatsu Photonics K.K. (Japan)
HORIBA, Ltd. (Japan)
JASCO International Co., Ltd. (Japan)
JEOL Ltd. (Japan)
Endress+Hauser Group Services AG (Switzerland)
Metrohm India Limited (Switzerland)
Montana Instruments Corporation (U.S.)
Coherent, Inc. (U.S.)
Teledyne Technologies Incorporated (U.S.)
Renishaw plc. (U.K.)
Shimadzu Corporation (Japan)
Short Description About Molecular Spectroscopy Market:
The Global Molecular Spectroscopy market is anticipated to rise at a considerable rate during the forecast period, between 2024 and 2031. In 2023, the market is growing at a steady rate and with the rising adoption of strategies by key players, the market is expected to rise over the projected horizon.
North America, especially The United States, will still play an important role which can not be ignored. Any changes from United States might affect the development trend of Molecular Spectroscopy. The market in North America is expected to grow considerably during the forecast period. The high adoption of advanced technology and the presence of large players in this region are likely to create ample growth opportunities for the market.
Europe also play important roles in global market, with a magnificent growth in CAGR During the Forecast period 2024-2031.
Molecular Spectroscopy Market size is projected to reach Multimillion USD by 2031, In comparison to 2024, at unexpected CAGR during 2024-2031.
Despite the presence of intense competition, due to the global recovery trend is clear, investors are still optimistic about this area, and it will still be more new investments entering the field in the future.
This report focuses on the Molecular Spectroscopy in global market, especially in North America, Europe and Asia-Pacific, South America, Middle East and Africa. This report categorizes the market based on manufacturers, regions, type and application.
Get a Sample Copy of the Molecular Spectroscopy Report 2024
What are your main data sources?
Both Primary and Secondary data sources are being used while compiling the report. Primary sources include extensive interviews of key opinion leaders and industry experts (such as experienced front-line staff, directors, CEOs, and marketing executives), downstream distributors, as well as end-users. Secondary sources include the research of the annual and financial reports of the top companies, public files, new journals, etc. We also cooperate with some third-party databases.
Geographically, the detailed analysis of consumption, revenue, market share and growth rate, historical data and forecast (2024-2031) of the following regions are covered in Chapters
What are the key regions in the global Molecular Spectroscopy market?
North America (United States, Canada and Mexico)
Europe (Germany, UK, France, Italy, Russia and Turkey etc.)
Asia-Pacific (China, Japan, Korea, India, Australia, Indonesia, Thailand, Philippines, Malaysia and Vietnam)
South America (Brazil, Argentina, Columbia etc.)
Middle East and Africa (Saudi Arabia, UAE, Egypt, Nigeria and South Africa)
This Molecular Spectroscopy Market Research/Analysis Report Contains Answers to your following Questions
What are the global trends in the Molecular Spectroscopy market?
Would the market witness an increase or decline in the demand in the coming years?
What is the estimated demand for different types of products in Molecular Spectroscopy?
What are the upcoming industry applications and trends for Molecular Spectroscopy market?
What Are Projections of Global Molecular Spectroscopy Industry Considering Capacity, Production and Production Value? What Will Be the Estimation of Cost and Profit? What Will Be Market Share, Supply and Consumption? What about Import and Export?
Where will the strategic developments take the industry in the mid to long-term?
What are the factors contributing to the final price of Molecular Spectroscopy?
What are the raw materials used for Molecular Spectroscopy manufacturing?
How big is the opportunity for the Molecular Spectroscopy market?
How will the increasing adoption of Molecular Spectroscopy for mining impact the growth rate of the overall market?
How much is the global Molecular Spectroscopy market worth? What was the value of the market In 2020?
Who are the major players operating in the Molecular Spectroscopy market? Which companies are the front runners?
Which are the recent industry trends that can be implemented to generate additional revenue streams?
What Should Be Entry Strategies, Countermeasures to Economic Impact, and Marketing Channels for Molecular Spectroscopy Industry?
Customization of the Report
Can I modify the scope of the report and customize it to suit my requirements? Yes. Customized requirements of multi-dimensional, deep-level and high-quality can help our customers precisely grasp market opportunities, effortlessly confront market challenges, properly formulate market strategies and act promptly, thus to win them sufficient time and space for market competition.
Inquire more and share questions if any before the purchase on this report at - https://www.databridgemarketresearch.com/inquire-before-buying/?dbmr=global-molecular-spectroscopy-market
Detailed TOC of Global Molecular Spectroscopy Market Insights and Forecast to 2031
Introduction
Market Segmentation
Executive Summary
Premium Insights
Market Overview
Molecular Spectroscopy Market By Type
Molecular Spectroscopy Market By Function
Molecular Spectroscopy Market By Material
Molecular Spectroscopy Market By End User
Molecular Spectroscopy Market By Region
Molecular Spectroscopy Market: Company Landscape
SWOT Analysis
Company Profiles
Continued...
Purchase this report – https://www.databridgemarketresearch.com/checkout/buy/singleuser/global-molecular-spectroscopy-market
Data Bridge Market Research:
Today's trends are a great way to predict future events!
Data Bridge Market Research is a market research and consulting company that stands out for its innovative and distinctive approach, as well as its unmatched resilience and integrated methods. We are dedicated to identifying the best market opportunities, and providing insightful information that will help your business thrive in the marketplace. Data Bridge offers tailored solutions to complex business challenges. This facilitates a smooth decision-making process. Data Bridge was founded in Pune in 2015. It is the product of deep wisdom and experience.
Contact Us:
Data Bridge Market Research
US: +1 614 591 3140
UK: +44 845 154 9652
APAC: +653 1251 975
Email:- [email protected]
Browse More Reports:
Global Vegetable Parchment Paper Market – Industry Trends and Forecast to 2028
Global Refrigerated Warehousing Market – Industry Trends and Forecast to 2030
Global Molecular Spectroscopy Market – Industry Trends and Forecast to 2030
Global Egg Protein in Pharmaceuticals Market – Industry Trends and Forecast to 2029
Global Anaplastic Astrocytoma Treatment Market - Industry Trends and Forecast to 2028
#Molecular Spectroscopy Market#Molecular Spectroscopy Market Size#Molecular Spectroscopy Market Share#Molecular Spectroscopy Market Trends
0 notes