#real-time learning analytics
Explore tagged Tumblr posts
Text
Living Intelligence: The Convergence of AI, Biotechnology, and Advanced Sensors in Education
By Ariel Can the classroom of the future actually learn from students? Imagine an environment where lessons adapt in real-time to each student’s needs, where smart sensors monitor cognitive load, and where AI-driven tutors adjust content dynamically to ensure mastery. This is the emerging reality of Living Intelligence—a cutting-edge blend of artificial intelligence, biotechnology, and advanced…
#adaptive learning environments#ai#AI in education#AI-assisted grading#AI-driven curriculum development#AI-driven lesson plans#AI-powered tutors#AR and VR in education#artificial intelligence in schools#biometric feedback in education#biotechnology in education#cognitive engagement tracking#digital learning transformation#education#educational neuroscience#educational technology trends#ethical AI in education#future of learning#future of STEM education#immersive learning experiences#integrating AI in schools#interactive learning environments#learning#machine learning in schools#next-gen education technology#personalized learning with AI#predictive analytics in education#real-time learning analytics#real-time student assessment#smart classrooms
0 notes
Text
How DeepSeek AI Revolutionizes Data Analysis
1. Introduction: The Data Analysis Crisis and AI’s Role2. What Is DeepSeek AI?3. Key Features of DeepSeek AI for Data Analysis4. How DeepSeek AI Outperforms Traditional Tools5. Real-World Applications Across Industries6. Step-by-Step: Implementing DeepSeek AI in Your Workflow7. FAQs About DeepSeek AI8. Conclusion 1. Introduction: The Data Analysis Crisis and AI’s Role Businesses today generate…
#AI automation trends#AI data analysis#AI for finance#AI in healthcare#AI-driven business intelligence#big data solutions#business intelligence trends#data-driven decisions#DeepSeek AI#ethical AI#ethical AI compliance#Future of AI#generative AI tools#machine learning applications#predictive modeling 2024#real-time analytics#retail AI optimization
3 notes
·
View notes
Text
make it make sense that I enjoy doing my job (especially the tedious parts) when I am off the clock, but as soon as I am supposed to be working I'd rather be doing LITERALLY anything else????
#like im having so much fun prepping all my TEAMS posts for Monday and doing SLEUTHING to figure out who owns this one meeting rn#but come Monday im gonna whine and groan and try my best to avoid my job as much as is reasonable. like??#and this happens often. I love doing remedial tasks at 2am. plugging shit into the glossaries that I dont care about woooooo hell yeah.#doing a quick audit on Tuesday at 10pm? yes. easy. takes 30 mins at MOST.#but like. ask me to update glossaries or make posts on TEAMS or do adults between 7am and 4:30 pm??? ABSOLUTELY NOT I will drag my FEET#If I really wanna psychoanalyze myself... I think that what's happening is that my work isnt interesting to me and I find it too easy#and really boring. and if im bored and dont care. it HURTS its SO HARD its PAINFUL to drag my brain through the mud to do it#and so I thus hate my job. BUT. the exact work I do for my job is what I ENJOY doing in my real life. I love organizing and scheduling#and prepping and alll that shit. like I work as an admin assistant at my job but like. I LOVE BEING AN ADMINISTRATOR FOR MY REAL LIFE!!#so when im off the clock and im in *sort my life out and prep for the future* mode of COURSE work is fun!! thats how I get my dopamine!!!#but I dont wanna be doing that ALL THE TIME cause like. tbh its kinda a stress response. so like. I want to do work that fills other needs.#I wanna do work that makes me hyperfixate and get super curious and challenges me and makes me think analytically and learn a ton#but my job doesnt do that. and my brain thus sorts the work I get paid to do as work that I do on my own time#thus I am really productive when im off the clock and dont do SHIT during the times I put down on my timesheet that I am working#shit still gets done but like.... at what cost?.#googoogajoob
2 notes
·
View notes
Text
Role of Technology in Modern Portfolio Management Services
Technology has reshaped portfolio management services, enhancing investment analysis, strategy optimization, and client experience. By utilizing artificial intelligence and automation, portfolio managers can make faster, more accurate decisions, addressing the complexities of modern financial markets.
Portfolio Management Technology Evolution
Traditional portfolio management depended heavily on basic analysis, intuition, and limited computational tools. Now, algorithms and machine learning provide deeper insights and more reliable decision-making. Key challenges addressed by technology include:
Navigating market complexity and volatility
Managing information overload from vast data streams
Improving risk assessment accuracy
Efficient portfolio rebalancing
Enhanced client communication and reporting
Major Technological Innovations in Portfolio Management
Artificial Intelligence and Machine Learning AI-driven systems now analyze large volumes of market data, identifying patterns and trends beyond human capabilities. Key advantages include:
Greater accuracy in forecasting market movements
Anomaly detection in trading patterns
Multi-variable portfolio optimization
Real-time adjustments to changing conditions
Automated Portfolio Rebalancing Smart rebalancing systems continuously monitor portfolio drift, making trades autonomously to maintain optimal asset allocation. They also optimize trade timing to reduce transaction costs and ensure tax-efficient rebalancing.
Real-Time Risk Analytics Advanced risk management systems offer:
Stress testing of portfolios under different market conditions
Automated real-time computation of risk metrics
Early detection of hidden risk factors
Portfolio strategy fine-tuning to adjust risk profiles
Benefits of Technology-Enabled Portfolio Management
Organizations that use advanced portfolio management services see numerous benefits, including:
45% reduction in portfolio analysis time
35% increase in risk-adjusted returns
60% decrease in manual data processing
40% improvement in client satisfaction scores
Data Analytics for Informed Decisions
Advanced data analytics provide a comprehensive view of market trends through:
Live market data insights
Sentiment analysis of social media and news
Economic indicator tracking with correlation analysis
Competitive landscape monitoring
Portfolio Optimizers
Modern portfolio management solutions can be tailored to individual client needs, offering:
Customized risk profiles
Goal-based investment strategies
Tax-aware trading
Constraint management based on investment goals
Detailed performance attribution and factor analysis
Multilevel reporting and style drift monitoring
ESG and Sustainable Investing
Advanced ESG Integration ESG (Environmental, Social, and Governance) technology offers:
Automated scoring and screening based on ESG factors
Impact measurement and reporting
Identification of sustainable investment opportunities
Climate risk assessment and monitoring
Enhancing the Client Experience
Technology improves the client experience with:
Interactive portfolio dashboards
Customized reporting
Mobile access to portfolio information
Automated client communications
Tools for assessing individual risk preferences
Cost Efficiency and Scalability
Tech-driven portfolio management reduces operational costs and enhances scalability by:
Minimizing manual intervention
Increasing the capacity and accuracy of portfolio managers
Improving trade reconciliation precision
Supporting regulatory compliance
Future Trends in Portfolio Management Technology
Emerging technologies will continue to shape portfolio management, including:
Blockchain Applications Blockchain enhances portfolio management through:
Improved transaction security
Faster settlement processes
Transparent asset ownership records
Execution of smart contracts
Advanced Data Analytics New data analytics techniques include:
Natural language processing in market research
Alternative data integration
Predictive analytics
Real-time market intelligence
Moving Toward a Technology-Intensive Portfolio Management Future
Investment professionals and firms are encouraged to:
Evaluate their current portfolio management processes
Identify areas for technological enhancement
Analyze solution providers for a seamless technology transition
Monitor performance metrics to optimize productivity
Embracing Technological Change
Portfolio management services are evolving with increased technology integration, which is crucial for gaining a competitive edge. Financial professionals must understand how this shift impacts both their workflows and their clients.
Ready to transform portfolio management with technology? Consult financial technology experts to explore advanced solutions for optimizing investment strategies and achieving superior client results.
0 notes
Text
AI HIGH TICKET COMMISSIONS
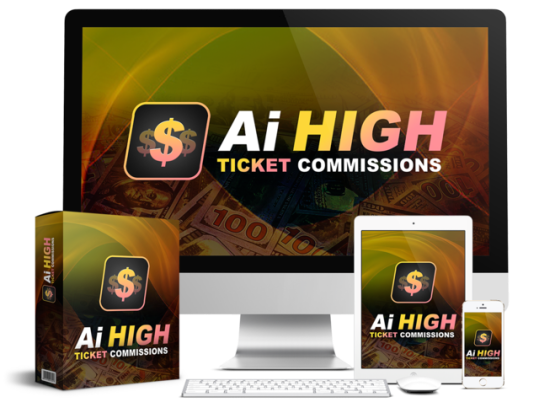
#Our advanced AI platform offers automated tools that optimize your sales strategy#ensuring you close high-value deals effortlessly.#By leveraging our AI tools#you’ll not only save time but also increase your conversion rates#allowing you to earn higher commissions without the extra effort.#Perfect for sales professionals#entrepreneurs#and marketers looking to maximize their income and streamline their processes.#‘Since using this AI platform#my commissions have doubled!’ – Sarah#Top Sales Rep.#it’s important to highlight key features and benefits that appeal to potential buyers. Here are some suggestions for how to structure your d#1. **Attention-Grabbing Intro**#- Start with a bold statement or question to capture interest.#- Example: “Unlock your earning potential with our exclusive AI-driven commission program!”#2. **Product Overview**#- Briefly describe what the product is and what it does.#- Example:#3. **Key Features**#- **Smart Analytics**: Utilize data-driven insights to identify your best prospects.#- **Seamless Integration**: Effortlessly connect with your existing CRM and marketing tools.#4. **Benefits**#- Explain how these features translate into real-world benefits.#5. **Target Audience**#- Identify who will benefit most from the product.#6. **Testimonials or Success Stories**#- Include quotes or case studies from satisfied customers.#7. **Call to Action**#- Encourage readers to take the next step#whether it's signing up or learning more.
1 note
·
View note
Text
youtube
#Oncology education#artificial intelligence#AI in healthcare#cancer diagnostics#machine learning#precision medicine#personalized medicine#radiomics#predictive analytics#cancer treatment#genomics#immunotherapy#medical education#AI-driven tools#data-driven decision-making#healthcare technology#cancer research#real-time data analysis#oncology training#future of oncology.#Youtube
0 notes
Text
How Can Data Science Predict Consumer Demand in an Ever-Changing Market?

In today’s dynamic business landscape, understanding consumer demand is more crucial than ever. As market conditions fluctuate, companies must rely on data-driven insights to stay competitive. Data science has emerged as a powerful tool that enables businesses to analyze trends and predict consumer behavior effectively. For those interested in mastering these techniques, pursuing an AI course in Chennai can provide the necessary skills and knowledge.
The Importance of Predicting Consumer Demand
Predicting consumer demand involves anticipating how much of a product or service consumers will purchase in the future. Accurate demand forecasting is essential for several reasons:
Inventory Management: Understanding demand helps businesses manage inventory levels, reducing the costs associated with overstocking or stockouts.
Strategic Planning: Businesses can make informed decisions regarding production, marketing, and sales strategies by accurately predicting consumer preferences.
Enhanced Customer Satisfaction: By aligning supply with anticipated demand, companies can ensure that they meet customer needs promptly, improving overall satisfaction.
Competitive Advantage: Organizations that can accurately forecast consumer demand are better positioned to capitalize on market opportunities and outperform their competitors.
How Data Science Facilitates Demand Prediction
Data science leverages various techniques and tools to analyze vast amounts of data and uncover patterns that can inform demand forecasting. Here are some key ways data science contributes to predicting consumer demand:
1. Data Collection
The first step in demand prediction is gathering relevant data. Data scientists collect information from multiple sources, including sales records, customer feedback, social media interactions, and market trends. This comprehensive dataset forms the foundation for accurate demand forecasting.
2. Data Cleaning and Preparation
Once the data is collected, it must be cleaned and organized. This involves removing inconsistencies, handling missing values, and transforming raw data into a usable format. Proper data preparation is crucial for ensuring the accuracy of predictive models.
3. Exploratory Data Analysis (EDA)
Data scientists perform exploratory data analysis to identify patterns and relationships within the data. EDA techniques, such as data visualization and statistical analysis, help analysts understand consumer behavior and the factors influencing demand.
4. Machine Learning Models
Machine learning algorithms play a vital role in demand prediction. These models can analyze historical data to identify trends and make forecasts. Common algorithms used for demand forecasting include:
Linear Regression: This model estimates the relationship between dependent and independent variables, making it suitable for predicting sales based on historical trends.
Time Series Analysis: Time series models analyze data points collected over time to identify seasonal patterns and trends, which are crucial for accurate demand forecasting.
Decision Trees: These models split data into branches based on decision rules, allowing analysts to understand the factors influencing consumer demand.
5. Real-Time Analytics
In an ever-changing market, real-time analytics becomes vital. Data science allows businesses to monitor consumer behavior continuously and adjust forecasts based on the latest data. This agility ensures that companies can respond quickly to shifts in consumer preferences.
Professionals who complete an AI course in Chennai gain insights into the latest machine learning techniques used in demand forecasting
Why Pursue an AI Course in Chennai?
For those looking to enter the field of data science and enhance their skills in predictive analytics, enrolling in an AI course in Chennai is an excellent option. Here’s why:
1. Comprehensive Curriculum
AI courses typically cover essential topics such as machine learning, data analysis, and predictive modeling. This comprehensive curriculum equips students with the skills needed to tackle real-world data challenges.
2. Hands-On Experience
Many courses emphasize practical, hands-on learning, allowing students to work on real-world projects that involve demand forecasting. This experience is invaluable for building confidence and competence.
3. Industry-Relevant Tools
Students often learn to use industry-standard tools and software, such as Python, R, and SQL, which are essential for conducting data analysis and building predictive models.
4. Networking Opportunities
Enrolling in an AI course in Chennai allows students to connect with peers and industry professionals, fostering relationships that can lead to job opportunities and collaborations.
Challenges in Predicting Consumer Demand
While data science offers powerful tools for demand forecasting, organizations may face challenges, including:
1. Data Quality
The accuracy of demand predictions heavily relies on the quality of data. Poor data quality can lead to misleading insights and misguided decisions.
2. Complexity of Models
Developing and interpreting predictive models can be complex. Organizations must invest in training and resources to ensure their teams can effectively utilize these models.
3. Rapidly Changing Markets
Consumer preferences can shift rapidly due to various factors, such as trends, economic changes, and competitive pressures. Businesses must remain agile to adapt their forecasts accordingly.
The curriculum of an AI course in Chennai often includes hands-on projects that focus on real-world applications of predictive analytics
Conclusion
Data science is revolutionizing how businesses predict consumer demand in an ever-changing market. By leveraging advanced analytics and machine learning techniques, organizations can make informed decisions that drive growth and enhance customer satisfaction.
For those looking to gain expertise in this field, pursuing an AI course in Chennai is a vital step. With a solid foundation in data science and AI, aspiring professionals can harness these technologies to drive innovation and success in their organizations.
#predictive analytics#predictivemodeling#predictiveanalytics#predictive programming#consumer demand#consumer behavior#demand analysis#machinelearning#machine learning#technology#data science#ai#artificial intelligence#Data science course#AI course#AI course in Chennai#Data science course in Chennai#Real-Time Analytics#Data Collection#Data Cleaning
0 notes
Text
Ethical and Privacy Issues in Big Data
The ethical dilemmas of Big Data Analytics revolve around three crucial aspects: privacy, security, and bias.
Developing ethical AI and responsible data handling practices is crucial for navigating the challenges of Big Data Analytics. This involves establishing clear guidelines for data use, conducting impact assessments, and fostering a culture of ethical behavior within organizations.
We are a team of experts who help clients reach a wider audience online. We design websites that improve user experience and meet customer expectations.
Feather Softwares is a pro in the field of social media marketing, enabling businesses to get more consumers. We make ads that are paid on social media to increase sales and to share useful content that helps customer.
Are you looking for a more impactful brand? Feather Softwares provide you with high-level instructional content that makes you appear as a leader in your field, as well as the improvement of your online visibility through SEO. This, in turn, facilitates the customers' discovery of your business.
For Business Enquiries- https://formfacade.com/sm/xvjfh3dkM For Course Enquiries - https://formfacade.com/sm/RD0FNS_ut

#data analytics#data mining services#Predictive Analytics#machine learning#Real-time Analytics#digital marketing#marketing#seo#graphic design#online visibility#ecommerce
0 notes
Text
Smarter Trucking: The Tech Transforming the Road Ahead
Trucking is evolving fast, thanks to some cutting-edge technology that’s making life on the road a whole lot smarter. Let’s talk about how companies are using AI, machine learning, cloud computing, and the Internet of Things (IoT) to make everything from route optimization to driver behavior analysis and capacity utilization more efficient. First up, AI and machine learning. These technologies…

View On WordPress
#AI in trucking#business#capacity utilization#cargo optimization#cash flow management#cloud computing#connected vehicles#data-driven decisions#driver behavior#driver safety#fleet management#fleet performance#Freight#freight industry#Freight Revenue Consultants#fuel efficiency#IoT#logistics#logistics technology#machine learning#operational efficiency#predictive analytics#real-time data#Route Optimization#small carriers#smart trucking#supply chain efficiency#Telematics#Trucking#trucking analytics
0 notes
Text
Living Intelligence: The Convergence of AI, Biotechnology, and Advanced Sensors in Education
By Ariel Can the classroom of the future actually learn from students? Imagine an environment where lessons adapt in real-time to each student’s needs, where smart sensors monitor cognitive load, and where AI-driven tutors adjust content dynamically to ensure mastery. This is the emerging reality of Living Intelligence—a cutting-edge blend of artificial intelligence, biotechnology, and advanced…
#adaptive learning environments#ai#AI in education#AI-assisted grading#AI-driven curriculum development#AI-driven lesson plans#AI-powered tutors#AR and VR in education#artificial intelligence in schools#biometric feedback in education#biotechnology in education#cognitive engagement tracking#digital learning transformation#education#educational neuroscience#educational technology trends#ethical AI in education#future of learning#future of STEM education#immersive learning experiences#integrating AI in schools#interactive learning environments#learning#machine learning in schools#next-gen education technology#personalized learning with AI#predictive analytics in education#real-time learning analytics#real-time student assessment#smart classrooms
0 notes
Text
21st Century Project Management: Navigating Challenges with Monitoring and Controlling
Discover how to master the monitoring and controlling phase of project management with cutting-edge tools and strategies. Stay ahead in the 21st century! Read our latest guide & subscribe for more insights from Hafsa Reasoner's empowered learning journey.
#21stcenturybusinessstrategies#artificialintelligenceinprojectmanagement#EmpoweredJourney#HafsaReasoner#KPIs#machinelearninginprojectmanagement#modernprojectmanagementtools#monitoringandcontrollingphase#performancemetrics#professionaldevelopmentinprojectmanagement#projectmanagement#projectsuccess#projecttracking#realtimedataanalytics#resourceallocationoptimization#riskmanagement#21st-century business strategies#artificial intelligence in project management#Empowered Journey#Hafsa Reasoner#machine learning in project management#modern project management tools#monitoring and controlling phase#performance metrics#professional development in project management#project management#project success#project tracking#real-time data analytics
0 notes
Text
Unveiling the Power of Delta Lake in Microsoft Fabric
Discover how Microsoft Fabric and Delta Lake can revolutionize your data management and analytics. Learn to optimize data ingestion with Spark and unlock the full potential of your data for smarter decision-making.
In today’s digital era, data is the new gold. Companies are constantly searching for ways to efficiently manage and analyze vast amounts of information to drive decision-making and innovation. However, with the growing volume and variety of data, traditional data processing methods often fall short. This is where Microsoft Fabric, Apache Spark and Delta Lake come into play. These powerful…
#ACID Transactions#Apache Spark#Big Data#Data Analytics#data engineering#Data Governance#Data Ingestion#Data Integration#Data Lakehouse#Data management#Data Pipelines#Data Processing#Data Science#Data Warehousing#Delta Lake#machine learning#Microsoft Fabric#Real-Time Analytics#Unified Data Platform
0 notes
Text
The Impact of Big Data Analytics on Business Decisions
Introduction
Big data analytics has transformed the way of doing business, deciding, and strategizing for future actions. One can harness vast reams of data to extract insights that were otherwise unimaginable for increasing the efficiency, customer satisfaction, and overall profitability of a venture. We steer into an in-depth view of how big data analytics is equipping business decisions, its benefits, and some future trends shaping up in this dynamic field in this article. Read to continue
#Innovation Insights#TagsAI in Big Data Analytics#big data analytics#Big Data in Finance#big data in healthcare#Big Data in Retail#Big Data Integration Challenges#Big Data Technologies#Business Decision Making with Big Data#Competitive Advantage with Big Data#Customer Insights through Big Data#Data Mining for Businesses#Data Privacy Challenges#Data-Driven Business Strategies#Future of Big Data Analytics#Hadoop and Spark#Impact of Big Data on Business#Machine Learning in Business#Operational Efficiency with Big Data#Predictive Analytics in Business#Real-Time Data Analysis#trends#tech news#science updates#analysis#adobe cloud#business tech#science#technology#tech trends
0 notes
Text
How Generative AI is Improving Business Forecast Accuracy

Reference : How Generative AI is Improving Business Forecast Accuracy - Medium
The age of digital transformation is upon us, and organizations are actively searching for inventive methods of outperforming rivals. One of the most revolutionary achievements in this regard is the inclusion of Generative AI into BI systems. Generative AI — a sub-category of AI that can create new data samples that are similar to a given set of data — is the revolutionary in forecasting and planning that BI uses. This article shows how generative AI is going to change the way we use business intelligence for forecasting and planning, its advantages, applications and ethical challenges.
The development of Business Intelligence
However, to start with the place of AI in BI forecasting and planning, it is important to comprehend the development of BI and its role in modern operation. Being a term that encompasses different tools, applications and methodologies, Business Intelligence enable an organization to gathering, analyzing and interpreting data to make the right decisions. Traditional BI platforms were mainly based on descriptive and diagnostic analytics with the focus on past performance and identifying prevailing trends.
Hence, with companies appreciating more and more the crucial role of predictive and prescriptive analytics for future success and competitive advantage, there is a heightened requirement for progressively complicated and competent BI tools. It is at this point where generative AI is brought into the equation, characterized by high-level capabilities capable of reshaping BI forecasting and planning strategies.
Through Generative AI in BI Forecasting and Planning, its capabilities can be utilized.
Enhanced Predictive Analytics
Generative AI uniquely increases the efficiency of predictive analytics through the use of complex data sets with advanced machine learning algorithms that factor out the accuracy of predictive models. It is true that unlike the traditional predictive analytics which mostly rely on predetermined algorithms and patterns, the power of AI is in its ability to create new data points and imaginary characters. This opens new opportunities for businesses to know the changing trends of the market better than their competitors and therefore become more efficient.
Generative AI is capable of identifying hidden patterns and subtle relationships contained in big and complex data sets which traditional BI tools fail to catch. Through the crunching of different variables and factors, generative AI can determine business’ insights into the market trends, customer behavior and possible threats and opportunities so that they can make decisions with aim of making the business to be successful.
Scenario Simulation
One of the further developments of AI generative technology is the scenario simulation which facilitates the forecasting and planning strategizing. Generative AI is capable of simulating multiple business scenarios due to its capability to generate synthetic datasets which are based on historical data. This way businesses are able to check and compare alternative strategies and their expected consequences allowing them to make wise decisions in the course of their planning process.
Realistic and accurate simulation by generative AI help to identify eccentric risks and probable openings, estimate the direction of different factors and see that business strategy is sturdy and responsive. This leads to increased agility and durability of enterprises, which allows them to follow quickly the rapidly flowing changes of market conditions and to grab new business opportunities.
Personalized Insights
The AI technologies also generates the personalized responses by analyzing the user’s behavior and inclination. Such an approach helps to uncover the most appropriate marketing and sales directions, which leads to great chances to increase among clients and their loyalty.
Revealing customer data, e.g. shopping history, browsing behavior and interaction with marketing campaigns, through sophisticated data analysis generative AI can find shortcomings and trends and craft personalized offers and recommendations for customers. It helps in planning and implementing marketing and sales strategies, thus it creates consumer engagement and sales growth.
Automating Routine Tasks
Generative AI might even be able to run the whole of the forecasting and planning activities, including data collection, processing and report writing. It gives BI professional additional spare time to focus more on strategic and analytical applications rather than spending it on simple data arrangement.
Generative AI in automation can help companies reduce routinary and time-consuming jobs and help them to grow in operations’ efficiency, cut down on operational costs and make their decision-making quicker. By doing this BI team productivity and performance will show up eventually allowing the team members to deliver more value to the organization.
Real-time Analytics
Generative AI does real-time analytics to keep tabs on the market updates and, consequently, helps a company to act in a timely manner, whenever there is a need for any market adjustments. However, this ability may be critically vital for industrial sectors that have very volatile markets such as retail, finance, and health care.
Thanks to real-time data analysis, generative AI brings business with a unique opportunity to spot and address emergent trends early, find new prospects, and stay informed about their key performance indicators in order to maximize performance and avoid losses on the spot. Technological advancement gives businesses a real edge of fast-decision making and flexibility, and it helps them to take the most of their opportunities.
Improved Data Quality
Generative AI has a great potential of boosting dat quality through detection and correction of such errors as clashing, inconsistency and outliers in data sets. As a result of this, forecasting will have a stronger fundament and would be more reliable and accurate, which minimizes the risk of making hasty decisions that are based on incomplete information.
Through enhancing data quality, generative AI gives to the businesses the opportunity to acquire better decisions thanks more to evidence and veracity, better shape the predictive models’ reliability and accuracy, as well as to enhance the efficiency of the forecasting and planning processes. This improves the accuracy and trustworthiness of the information promoted by BI which helps the businesses make informed decisions with vigour.
Ethical Considerations
Even if generative AI in BI can bring about positive outcomes in forecasting and planning, one should also think about AI ethic issues which might arise and hinder the implementation of this technology. Enterprises should pay special attention that AI models are trained and applied with data collected and used in accordance with the data ethical norms, privacy and compliance regulations established by the lawmakers.
Data Privacy and Security
The AI of the future relies on getting access to relevant and numerous data sets to create meaningful and valued outputs. Companies must have data privacy and security policies to be aware of threats of data misuse, unauthorized access and breaches. Those policies must ensure that only authorized personnel could access sensitive and confidential information of others.
Transparency and Accountability
Therefore, generative AI, which has complex machine learning algorithms to achieve their goals and yield outcomes that are sometimes difficult to decode is one of the advanced technologies of AI. The realm of ethics should include but not be limited to the notion of how the AI “black boxes” function, how decision making comes about, or how any possible biases are identified and dealt with.
Fairness and Bias
AI that is able to creatively could unwittingly therefore keep and amplify the current unfavorable and unfair indications, which is present in the training data for the model. Organizations should eliminate bias and identify mechanisms that can modulate the bias and promote equality. Thus, A.I. must generate unbiased and equitable information.
Conclusion
In the meantime, generative AI is making BI more efficient with imperative analytics, allowing to simulate with different scenarios, wherever applicable providing specific insights on an individual level, automating the routine tasks, availability of real-time analytics, increment in the quality of the data as well as securing the competitive advantage. However, businesses should indeed manage not only the operative questions, but also the ethical aspects confirming due performance when working with data in order to take the best from generative AI in BI.
The prominence of generative AI in today’s business sphere is unimaginable. Businesses always modernize and adapt to changing business environments. This calls for businesses to implement outputs of generative AI in their BI systems into lately. Through the inclusive implementation of the transforming impact of AI with the ethics keeping quiet, companies can become successful because of the cut-throat competition and the fast moving of businesses, in the business world.
#AI Generated Insights#AI Powered Data Analytics#Business Intelligence Platforms#Generative AI Into BI Systems#Development Of Business Intelligence#Role Of Predictive Analytics#AI Based Analytics Solutions#AI Powered Functionality#Power Of AI#Traditional BI Tools#Advanced Technologies Of AI#Machine Learning Algorithms#AI Based Predictive Analysis#Business Intelligence Practices#Data Analysis#Real Time Data Analysis#Business Intelligence Development
0 notes
Text
From predictions to planning, generative AI is redefining business intelligence. Learn how it’s revolutionizing forecasting strategies.
#Generative AI into BI Systems#Development of Business Intelligence#Role of Predictive Analytics#Power of AI#Traditional BI Tools#Advanced Technologies of AI#Machine Learning Algorithms#Data Analysis#Real Time Data Analysis
0 notes
Text
Big Data vs. Traditional Data: Understanding the Differences and When to Use Python

In the evolving landscape of data science, understanding the nuances between big data and traditional data is crucial. Both play pivotal roles in analytics, but their characteristics, processing methods, and use cases differ significantly. Python, a powerful and versatile programming language, has become an indispensable tool for handling both types of data. This blog will explore the differences between big data and traditional data and explain when to use Python, emphasizing the importance of enrolling in a data science training program to master these skills.
What is Traditional Data?
Traditional data refers to structured data typically stored in relational databases and managed using SQL (Structured Query Language). This data is often transactional and includes records such as sales transactions, customer information, and inventory levels.
Characteristics of Traditional Data:
Structured Format: Traditional data is organized in a structured format, usually in rows and columns within relational databases.
Manageable Volume: The volume of traditional data is relatively small and manageable, often ranging from gigabytes to terabytes.
Fixed Schema: The schema, or structure, of traditional data is predefined and consistent, making it easy to query and analyze.
Use Cases of Traditional Data:
Transaction Processing: Traditional data is used for transaction processing in industries like finance and retail, where accurate and reliable records are essential.
Customer Relationship Management (CRM): Businesses use traditional data to manage customer relationships, track interactions, and analyze customer behavior.
Inventory Management: Traditional data is used to monitor and manage inventory levels, ensuring optimal stock levels and efficient supply chain operations.
What is Big Data?
Big data refers to extremely large and complex datasets that cannot be managed and processed using traditional database systems. It encompasses structured, unstructured, and semi-structured data from various sources, including social media, sensors, and log files.
Characteristics of Big Data:
Volume: Big data involves vast amounts of data, often measured in petabytes or exabytes.
Velocity: Big data is generated at high speed, requiring real-time or near-real-time processing.
Variety: Big data comes in diverse formats, including text, images, videos, and sensor data.
Veracity: Big data can be noisy and uncertain, requiring advanced techniques to ensure data quality and accuracy.
Use Cases of Big Data:
Predictive Analytics: Big data is used for predictive analytics in fields like healthcare, finance, and marketing, where it helps forecast trends and behaviors.
IoT (Internet of Things): Big data from IoT devices is used to monitor and analyze physical systems, such as smart cities, industrial machines, and connected vehicles.
Social Media Analysis: Big data from social media platforms is analyzed to understand user sentiments, trends, and behavior patterns.
Python: The Versatile Tool for Data Science
Python has emerged as the go-to programming language for data science due to its simplicity, versatility, and robust ecosystem of libraries and frameworks. Whether dealing with traditional data or big data, Python provides powerful tools and techniques to analyze and visualize data effectively.
Python for Traditional Data:
Pandas: The Pandas library in Python is ideal for handling traditional data. It offers data structures like DataFrames that facilitate easy manipulation, analysis, and visualization of structured data.
SQLAlchemy: Python's SQLAlchemy library provides a powerful toolkit for working with relational databases, allowing seamless integration with SQL databases for querying and data manipulation.
Python for Big Data:
PySpark: PySpark, the Python API for Apache Spark, is designed for big data processing. It enables distributed computing and parallel processing, making it suitable for handling large-scale datasets.
Dask: Dask is a flexible parallel computing library in Python that scales from single machines to large clusters, making it an excellent choice for big data analytics.
When to Use Python for Data Science
Understanding when to use Python for different types of data is crucial for effective data analysis and decision-making.
Traditional Data:
Business Analytics: Use Python for traditional data analytics in business scenarios, such as sales forecasting, customer segmentation, and financial analysis. Python's libraries, like Pandas and Matplotlib, offer comprehensive tools for these tasks.
Data Cleaning and Transformation: Python is highly effective for data cleaning and transformation, ensuring that traditional data is accurate, consistent, and ready for analysis.
Big Data:
Real-Time Analytics: When dealing with real-time data streams from IoT devices or social media platforms, Python's integration with big data frameworks like Apache Spark enables efficient processing and analysis.
Large-Scale Machine Learning: For large-scale machine learning projects, Python's compatibility with libraries like TensorFlow and PyTorch, combined with big data processing tools, makes it an ideal choice.
The Importance of Data Science Training Programs
To effectively navigate the complexities of both traditional data and big data, it is essential to acquire the right skills and knowledge. Data science training programs provide comprehensive education and hands-on experience in data science tools and techniques.
Comprehensive Curriculum: Data science training programs cover a wide range of topics, including data analysis, machine learning, big data processing, and data visualization, ensuring a well-rounded education.
Practical Experience: These programs emphasize practical learning through projects and case studies, allowing students to apply theoretical knowledge to real-world scenarios.
Expert Guidance: Experienced instructors and industry mentors offer valuable insights and support, helping students master the complexities of data science.
Career Opportunities: Graduates of data science training programs are in high demand across various industries, with opportunities to work on innovative projects and drive data-driven decision-making.
Conclusion
Understanding the differences between big data and traditional data is fundamental for any aspiring data scientist. While traditional data is structured, manageable, and used for transaction processing, big data is vast, varied, and requires advanced tools for real-time processing and analysis. Python, with its robust ecosystem of libraries and frameworks, is an indispensable tool for handling both types of data effectively.
Enrolling in a data science training program equips you with the skills and knowledge needed to navigate the complexities of data science. Whether you're working with traditional data or big data, mastering Python and other data science tools will enable you to extract valuable insights and drive innovation in your field. Start your journey today and unlock the potential of data science with a comprehensive training program.
#Big Data#Traditional Data#Data Science#Python Programming#Data Analysis#Machine Learning#Predictive Analytics#Data Science Training Program#SQL#Data Visualization#Business Analytics#Real-Time Analytics#IoT Data#Data Transformation
0 notes