#Advanced Data Analysis
Explore tagged Tumblr posts
Text
Future Of AI In Software Development
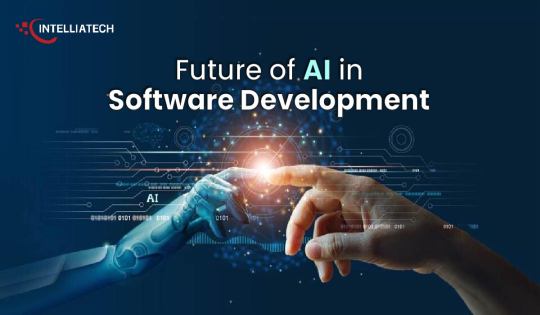
The usage of AI in Software Development has seen a boom in recent years and it will further continue to redefine the IT industry. In this blog post, we’ll be sharing the existing scenario of AI, its impacts and benefits for software engineers, future trends and challenge areas to help you give a bigger picture of the performance of artificial intelligence (AI). This trend has grown to the extent that it has become an important part of the software development process. With the rapid evolvements happening in the software industry, AI is surely going to dominate.
Read More
#Accountability#Accuracy Accuracy#Advanced Data Analysis#artificial intelligence#automated testing#Automation#bug detection#code generation#code reviews#continuous integration#continuous deployment#cost savings#debugging#efficiency#Enhanced personalization#Ethical considerations#future trends#gartner report#image generation#improved productivity#job displacement#machine learning#natural language processing#privacy privacy#safety#security concerns#software development#software engineers#time savings#transparency
0 notes
Text
Prior to the election, I recall some Rightwing Analyst predicting that Orange Palpatine would win with over 50% of the vote while Harris barely gets 40%.
Orange Man did win in both Popular and Electoral Votes but it wasn’t the landslide that said Analyst predicted.
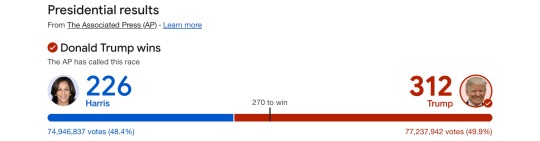
Reminder:
People who shifted Right did so mostly because of Economic Concerns .
Yes, social issues related to Gender and Ethnicity may have been elements in places but they’re not the sole determining factors.
Dems focusing on appeasing corporate CEO’s and the like did NOT help them in the slightest.
It’s my honest belief that Dems really need to repair their image of truly being the Party that stands for “Working Class” individuals.
The current “Status Quo” must be undone and replaced with a new normal of average folks being empowered instead of oligarchs.
Dems must do this in order to have a meaningful future.
#us election#election 2024#autopsy#analysis#my two cents#usa politics#political#thoughts#opinion#my take#just putting it out there#something to consider#dont give up#keep fighting#we will not go back#donotobey#do not obey in advance#nevergiveup#never stop fighting#stand and fight#data#think about it#thinking things#be wise#us politics#the bigger picture
7 notes
·
View notes
Text
This is the part where all the people who followed me for RotB Mirage memes and babygirl jokes discover I'm actually the Extremely Niche Fucked Up AUs and Profound Angst guy and I am so so sorry for that ksjhdjks
#this is in regards to the Data Ghosts AU#But also just in general....#frostbitten and bloodstained. they have electric souls. depressing ass analysis. I have a Lot of terrible things planned in ADKoR sjkhd#I'm the tragedies guy I'm sorry!!#I find inevitable heartbreak more compelling than happy endings!! I'm a romantic in a blood and gore acearo way and it shows!!!!#I apologize in advance for the psychic damage :'//
14 notes
·
View notes
Text
The Impact of AI on Everyday Life: A New Normal
The impact of AI on everyday life has become a focal point for discussions among tech enthusiasts, policymakers, and the general public alike. This transformative force is reshaping the way we live, work, and interact with the world around us, making its influence felt across various domains of our daily existence. Revolutionizing Workplaces One of the most significant arenas where the impact…
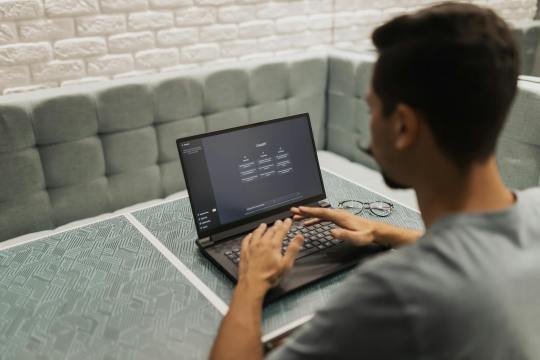
View On WordPress
#adaptive learning#AI accessibility#AI adaptation#AI advancements#AI algorithms#AI applications#AI automation#AI benefits#AI capability#AI challenges#AI collaboration#AI convenience#AI data analysis#AI debate#AI decision-making#AI design#AI diagnostics#AI discussion#AI education#AI efficiency#AI engineering#AI enhancement#AI environment#AI ethics#AI experience#AI future#AI governance#AI healthcare#AI impact#AI implications
1 note
·
View note
Text
Swarm Enterprises
Website: https://swarm.enterprises/
Address: San Francisco, California
Phone: +1 (504) 249-8350
Swarm Enterprises: Revolutionizing Decision-Making with Cutting-Edge Technology
Swarm Enterprises is at the forefront of innovation, harnessing the power of swarm intelligence algorithms to redefine the way decisions are made. Inspired by the coordinated movements of natural swarms, these algorithms deliver unparalleled precision and intelligent recommendations. Clients are empowered to embrace data-driven decision-making, resulting in heightened efficiency and superior outcomes.
The company's secret weapon lies in its utilization of machine learning techniques, where massive data sets are transformed into invaluable insights. By training algorithms on both historical and real-time data, Swarm Enterprises uncovers hidden patterns, emerging trends, and subtle anomalies that often elude human analysis. This extraordinary capability allows clients to unearth concealed opportunities, minimize risks, and gain an undeniable competitive edge.
Swarm Enterprises doesn't stop there; they seamlessly integrate IoT (Internet of Things) devices into their offerings, enabling real-time data collection and analysis. These connected physical objects grant clients the ability to remotely monitor and manage various aspects of their operations. This groundbreaking technology not only streamlines proactive maintenance but also enhances operational efficiency and boosts overall productivity.
In a world where informed decisions are paramount, Swarm Enterprises is a trailblazer, providing the tools and insights necessary for success in an increasingly data-centric landscape.
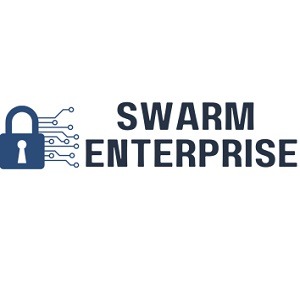
#Bot Detection & Defense in San Francisco#Real-time bot detection near me#Security against live botnet traffic#Browser Feature Signatures#Performance Profiling#Automated Behavior Analysis#Advanced Analysis Technique#Integration & Results#Data & Insights#Simplified Integration#Accuracy and Precision#Real-time Reporting
2 notes
·
View notes
Text
The Transformative Benefits of Artificial Intelligence
Title: The Transformative Benefits of Artificial Intelligence Artificial Intelligence (AI) has emerged as one of the most revolutionary technologies of the 21st century. It involves creating intelligent machines that can mimic human cognitive functions such as learning, reasoning, problem-solving, and decision-making. As AI continues to advance, its impact is felt across various industries and…
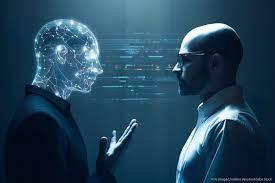
View On WordPress
#Advancements in Education#AI Advantages#AI Benefits#artificial intelligence#Customer Experience#Data Analysis#Data Analytics#Decision-Making#Efficiency and Productivity#Energy Management#Ethical AI Deployment.#Healthcare Transformation#Machine Learning#Personalized Learning#Personalized User Experiences#Robotics in Healthcare#Smart Cities#Smart Technology#Smart Traffic Management#Sustainable Development
2 notes
·
View notes
Text
Learn Advanced Excel for Data Analysis | Master Excel Skills Online
Unlock the full potential of Microsoft Excel by learning advanced techniques tailored for data analysis. This comprehensive course is designed for professionals, analysts, and students who want to gain hands-on expertise in using Excel for making data-driven decisions. Explore powerful tools like PivotTables, Power Query, VLOOKUP, XLOOKUP, data visualization, dynamic charts, conditional formatting, and advanced formulas. Whether you're preparing dashboards, cleaning data, or performing complex analyses, this training will give you the edge to turn raw data into meaningful insights. Learn at your own pace and elevate your data analysis skills with Excel today!
0 notes
Text
What are the 5 main functions of Excel?

When exploring what are the 5 main functions of Excel, it’s clear that this powerful spreadsheet application dramatically enhances the productivity of students, professionals, and business owners alike. TCCI-Tririd Computer Coaching Institute aims to help students and working professionals improve their Excel skills for career benefits.
1. Data Entry and Management
A simple, systematic means of data entry, organization, and management of huge amounts of data is provided by Excel. Users can fill out cells with numbers, text, or dates and present that cell information in some system in rows and columns. The management of complex datasets is made easier through such functions, like data validation, sorting, and filtering.
2. Data Analysis and Representation
Excel's ability to analyze data with the use of pivot tables, charts, and graphs is one of its most powerful functions. Charts such as bar, pie, line, and scatter can be created by users to present data trends and insights, thereby enhancing the value of their reports and presentations.
3. Mathematical and Logical Calculations
A wide range of built-in mathematical, statistical, and logical functions are provided in Excel, such as:
SUM()- Adds values in a range.
AVERAGE() - Computes the average of the numbers selected.
IF() - Is used for logical operations that depend on conditions.
VLOOKUP() & HLOOKUP() - Looks for values inside a table.
4: Data Formatting and Customization:
Excel provides a number of features to format its presentation of data, including:
When conditional formatting can be applied, set specific values to be highlighted;
Cell formatting options (bold, italics, colors);
Be able to adjust column and row width/height for easier reading.
All of these elements will enhance the appearance and tidy presentation of data.
5. Automation with Macros and Formulas
With macros and VBA (Visual Basic for Applications), processes can be automated for tasks that are repetitive. Recording macros can help users automate the repetitive tasks, thus improving their efficiency. On the other hand, complex formulas and functions can be set for tasks requiring calculations and immediate results.
Learn Excel at TCCI-Tririd Computer Coaching Institute
TCCI offers a complete Advanced Excel training course designed to make students and professionals well versed in these important functions. Whether you start as a beginner or semi-expert, you will find assistance in lots of practical training to enhance your Excel skills from our expert trainers.
So why wait? Master Excel through TCCI-Tririd Computer Coaching Institute and uplift your career with one of our specialized Excel courses!
Location: Bopal & Iskon-Ambli Ahmedabad, Gujarat
Call now on +91 9825618292
Get information from: https://tccicomputercoaching.wordpress.com/
#Main Functions of Excel#Excel Training at TCCI#Advanced Excel Features#Learn Excel for Data Analysis#Best Excel Coaching Institute
0 notes
Text
#GPU Market#Graphics Processing Unit#GPU Industry Trends#Market Research Report#GPU Market Growth#Semiconductor Industry#Gaming GPUs#AI and Machine Learning GPUs#Data Center GPUs#High-Performance Computing#GPU Market Analysis#Market Size and Forecast#GPU Manufacturers#Cloud Computing GPUs#GPU Demand Drivers#Technological Advancements in GPUs#GPU Applications#Competitive Landscape#Consumer Electronics GPUs#Emerging Markets for GPUs
0 notes
Text
Twitter Advanced Search helps you find specific tweets or accounts on X (Twitter). This is a very powerful tool built into the X platform. And there are many different ways in which you can utilize the full potential of Twitter's advanced search. Any digital marketer can benefit from this and can track different campaigns on X.
#twitter advanced search#twitter tool#twitter data#hashtag analytics#historical twitter data#twitter dataset#hashtag analysis#historical data
1 note
·
View note
Text
How to use COPILOT in Microsoft Word | Tutorial
This page contains a video tutorial by Reza Dorrani on how to use Microsoft 365 Copilot in Microsoft Word. The video covers: Starting a draft with Copilot in Word. Adding content to an existing document using Copilot. Rewriting text with Copilot. Generating summaries with Copilot. Overall, using Copilot as a dynamic writing companion to enhance productivity in Word. Is there something…
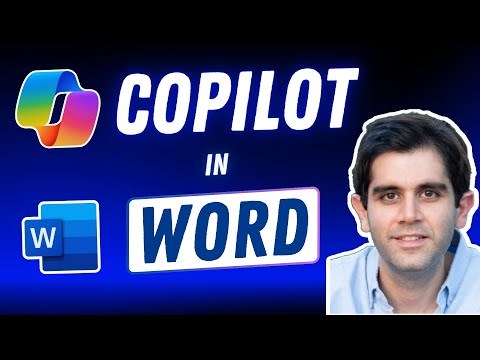
View On WordPress
#Advanced Excel#Automation tools#Collaboration#copilot#Data analysis#Data management#Data visualization#Excel#Excel formulas#Excel functions#Excel skills#Excel tips#Excel tutorials#MIcrosoft Copilot#Microsoft Excel#Microsoft Office#Microsoft Word#Office 365#Power BI#productivity#Task automation
1 note
·
View note
Text
Saryu Nayyar, CEO and Founder of Gurucul – Interview Series
New Post has been published on https://thedigitalinsider.com/saryu-nayyar-ceo-and-founder-of-gurucul-interview-series/
Saryu Nayyar, CEO and Founder of Gurucul – Interview Series
Saryu Nayyar is an internationally recognized cybersecurity expert, author, speaker and member of the Forbes Technology Council. She has more than 15 years of experience in the information security, identity and access management, IT risk and compliance, and security risk management sectors.
She was named EY Entrepreneurial Winning Women in 2017. She has held leadership roles in security products and services strategy at Oracle, Simeio, Sun Microsystems, Vaau (acquired by Sun) and Disney. Saryu also spent several years in senior positions at the technology security and risk management practice of Ernst & Young.
Gurucul is a cybersecurity company that specializes in behavior-based security and risk analytics. Its platform leverages machine learning, AI, and big data to detect insider threats, account compromise, and advanced attacks across hybrid environments. Gurucul is known for its Unified Security and Risk Analytics Platform, which integrates SIEM, UEBA (User and Entity Behavior Analytics), XDR, and identity analytics to provide real-time threat detection and response. The company serves enterprises, governments, and MSSPs, aiming to reduce false positives and accelerate threat remediation through intelligent automation.
What inspired you to start Gurucul in 2010, and what problem were you aiming to solve in the cybersecurity landscape?
Gurucul was founded to help Security Operations and Insider Risk Management teams obtain clarity into the most critical cyber risks impacting their business. Since 2010 we’ve taken a behavioral and predictive analytics approach, rather than rules-based, which has generated over 4,000+ machine learning models that put user and entity anomalies into context across a variety of different attack and risk scenarios. We’ve built upon this as our foundation, moving from helping large Fortune 50 companies solve Insider Risk challenges, to helping companies gain radical clarity into ALL cyber risk. This is the promise of REVEAL, our unified and AI-Driven Data and Security Analytics platform. Now we’re building on our AI mission with a vision to deliver a Self-Driving Security Analytics platform, using Machine Learning as our foundation but now layering on Generative and Agentic AI capabilities across the entire threat lifecycle. The goal is for analysts and engineers to spend less time in the myriad in complexity and more time focused on meaningful work. Allowing machines to amplify the definition of their day-to-day activities.
Having worked in leadership roles at Oracle, Sun Microsystems, and Ernst & Young, what key lessons did you bring from those experiences into founding Gurucul?
My leadership experience at Oracle, Sun Microsystems, and Ernst & Young strengthened my ability to solve complex security challenges and provided me with an understanding of the challenges that Fortune 100 CEOs and CISOs face. Collectively, it allowed me to gain a front-row seat the technological and business challenges most security leaders face and inspired me to build solutions to bridge those gaps.
How does Gurucul’s REVEAL platform differentiate itself from traditional SIEM (Security Information and Event Management) solutions?
Legacy SIEM solutions depend on static, rule-based approaches that lead to excessive false positives, increased costs, and delayed detection and response. Our REVEAL platform is fully cloud-native and AI-driven, utilizing advanced machine learning, behavioral analytics, and dynamic risk scoring to detect and respond to threats in real time. Unlike traditional platforms, REVEAL continuously adapts to evolving threats and integrates across on-premises, cloud, and hybrid environments for comprehensive security coverage. Recognized as the ‘Most Visionary’ SIEM solution in Gartner’s Magic Quadrant for three consecutive years, REVEAL redefines AI-driven SIEM with unmatched precision, speed, and visibility. Furthermore, SIEMs struggle with a data overload problem. They are too expensive to ingest everything needed for complete visibility and even if they do it just adds to the false positive problem. Gurucul understands this problem and it’s why we have a native and AI-driven Data Pipeline Management solution that filters non-critical data to low-cost storage, saving money, while retaining the ability to run federated search across all data. Analytics systems are a “garbage in, garbage out” situation. If the data coming in is bloated, unnecessary or incomplete then the output will not be accurate, actionable or ultimately trusted.
Can you explain how machine learning and behavioral analytics are used to detect threats in real time?
Our platform leverages over 4,000 machine learning models to continuously analyze all relevant datasets and identify anomalies and suspicious behaviors in real time. Unlike legacy security systems that rely on static rules, REVEAL uncovers threats as they emerge. The platform also utilizes User and Entity Behavior Analytics (UEBA) to establish baselines of normal user and entity behavior, detecting deviations that could indicate insider threats, compromised accounts, or malicious activity. This behavior is further contextualized by a big data engine that correlates, enriches and links security, network, IT, IoT, cloud, identity, business application data and both internal and external sourced threat intelligence. This informs a dynamic risk scoring engine that assigns real-time risk scores that help prioritize responses to critical threats. Together, these capabilities provide a comprehensive, AI-driven approach to real-time threat detection and response that set REVEAL apart from conventional security solutions.
How does Gurucul’s AI-driven approach help reduce false positives compared to conventional cybersecurity systems?
The REVEAL platform reduces false positives by leveraging AI-driven contextual analysis, behavioral insights, and machine learning to distinguish legitimate user activity from actual threats. Unlike conventional solutions, REVEAL refines its detection capabilities over time, improving accuracy while minimizing noise. Its UEBA detects deviations from baseline activity with high accuracy, allowing security teams to focus on legitimate security risks rather than being overwhelmed by false alarms. While Machine Learning is a foundational aspect, generative and agentic AI play a significant role in further appending context in natural language to help analysts understand exactly what is happening around an alert and even automate the response to said alerts.
What role does adversarial AI play in modern cybersecurity threats, and how does Gurucul combat these evolving risks?
First all we’re already seeing adversarial AI being applied to the lowest hanging fruit, the human vector and identity-based threats. This is why behavioral, and identity analytics are critical to being able to identify anomalous behaviors, put them into context and predict malicious behavior before it proliferates further. Furthermore, adversarial AI is the nail in the coffin for signature-based detection methods. Adversaries are using AI to evade these TTP defined detection rules, but again they can’t evade the behavioral based detections in the same way. SOC teams are not resourced adequately to continue to write rules to keep pace and will require a modern approach to threat detection, investigation and response. Behavior and context are the key ingredients. Finally, platforms like REVEAL depend on a continuous feedback loop and we’re constantly applying AI to help us refine our detection models, recommend new models and inform new threat intelligence our entire ecosystem of customers can benefit from.
How does Gurucul’s risk-based scoring system improve security teams’ ability to prioritize threats?
Our platform’s dynamic risk scoring system assigns real-time risk scores to users, entities, and actions based on observed behaviors and contextual insights. This enables security teams to prioritize critical threats, reducing response times and optimizing resources. By quantifying risk on a 0–100 scale, REVEAL ensures that organizations focus on the most pressing incidents rather than being overwhelmed by low-priority alerts. With a unified risk score spanning all enterprise data sources, security teams gain greater visibility and control, leading to faster, more informed decision-making.
In an age of increasing data breaches, how can AI-driven security solutions help organizations prevent insider threats?
Insider threats are an especially challenging security risk due to their subtle nature and the access that employees possess. REVEAL’s UEBA detects deviations from established behavioral baselines, identifying risky activities such as unauthorized data access, unusual login times, and privilege misuse. Dynamic risk scoring also continuously assesses behaviors in real time, assigning risk levels to prioritize the most pressing insider risks. These AI-driven capabilities enable security teams to proactively detect and mitigate insider threats before they escalate into breaches. Given the predictive nature of behavioral analytics Insider Risk Management is race against the clock. Insider Risk Management teams need to be able to respond and collaborate quickly, with privacy top-of-mind. Context again is critical here and appending behavioral deviations with context from identity systems, HR applications and all other relevant data sources gives these teams the ammunition to quickly build and defend a case of evidence so the business can respond and remediate before data exfiltration occurs.
How does Gurucul’s identity analytics solution enhance security compared to traditional IAM (identity and access management) tools?
Traditional IAM solutions focus on access control and authentication but lack the intelligence and visibility to detect compromised accounts or privilege abuse in real time. REVEAL goes beyond these limitations by leveraging AI-powered behavioral analytics to continuously assess user risk, dynamically adjust risk scores, and enforce adaptive access entitlements, minimizing misuse and illegitimate privileges. By integrating with existing IAM frameworks and enforcing least-privilege access, our solution enhances identity security and reduces the attack surface. The problem with IAM governance is identity system sprawl and the lack of interconnectedness between different identity systems. Gurucul gives teams a 360° view of their identity risks across all identity infrastructure. Now they can stop rubber stamping access but rather take risk-oriented approach to access policies. Furthermore, they can expedite the compliance aspect of IAM and demonstrate a continuous monitoring and fully holistic approach to access controls across the organization.
What are the key cybersecurity threats you foresee in the next five years, and how can AI help mitigate them?
Identity-based threats will continue to proliferate, because they have worked. Adversaries are going to double-down on gaining access by logging in either via compromising insiders or attacking identity infrastructure. Naturally insider threats will continue to be a key risk vector for many businesses, especially as shadow IT continues. Whether malicious or negligent, companies will increasingly need visibility into insider risk. Furthermore, AI will accelerate the variations of conventional TTPs, because adversaries know that is how they will be able to evade detections by doing so and it will be low cost for them to creative adaptive tactics, technics and protocols. Hence again why focusing on behavior in context and having detection systems capable of adapting just as fast will be crucial for the foreseeable future.
Thank you for the great interview, readers who wish to learn more should visit Gurucul.
#000#access control#access management#account compromise#Accounts#Advanced attacks#adversaries#Agentic AI#ai#AI-powered#alerts#ammunition#amp#Analysis#Analytics#anomalies#applications#approach#Attack surface#authentication#author#automation#Behavior#Big Data#bridge#Building#Business#Business application#CEO#CISOs
0 notes
Text
Mastering Predictive Analysis Techniques Through Advanced Data Science Programs
Predictive analysis techniques are revolutionizing industries by enabling data-driven decision-making. Whether you’re aiming to enhance customer satisfaction, streamline operations, or manage risks, mastering these methods is crucial. Enrolling in the Best Data Science Training in Delhi, Noida, Lucknow, Nagpur, and other cities in India can help you develop expertise in predictive modeling, machine learning algorithms, and data preprocessing.
Read more: https://reshukhushi.wordpress.com/2025/02/03/mastering-predictive-analysis-techniques-through-advanced-data-science-programs/
0 notes
Text
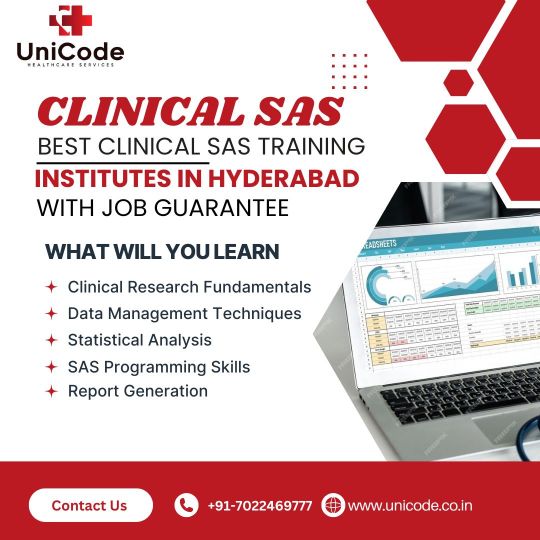
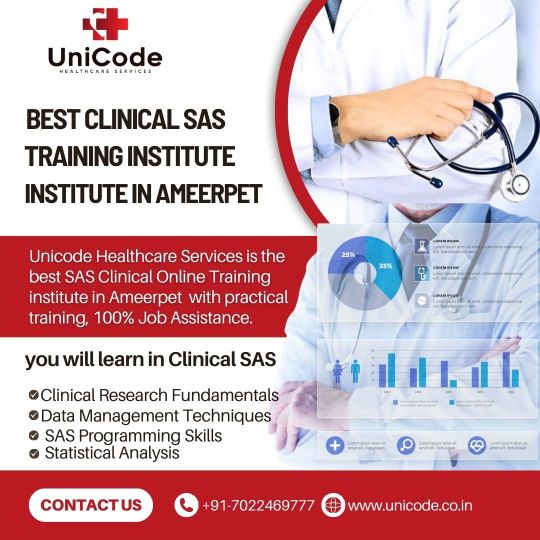
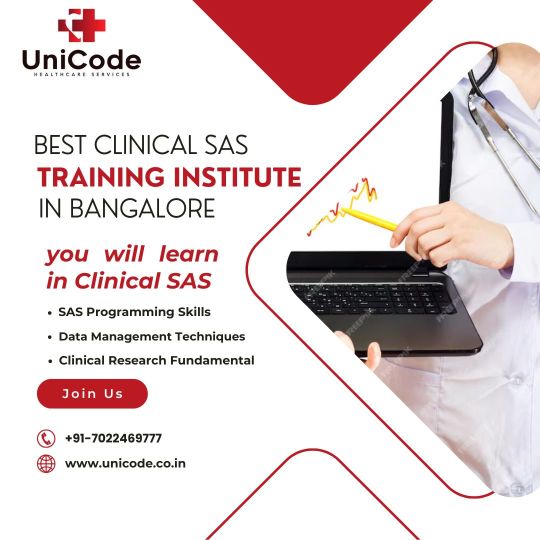
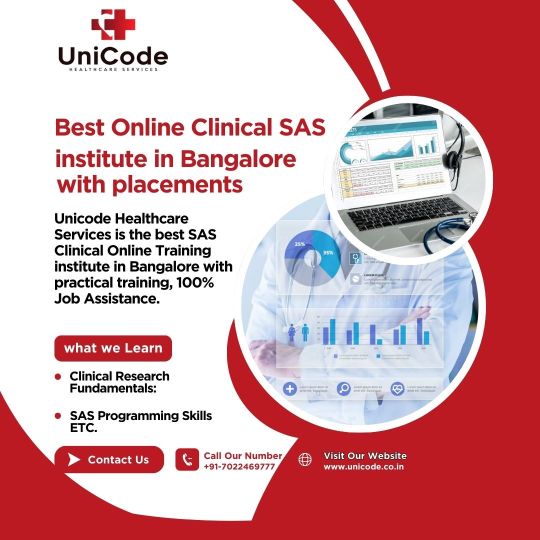
#Best Clinical SAS Training Institute in Hyderabad#Unicode Healthcare Services stands out as the top Clinical SAS training institute in Ameerpet#Hyderabad. Our comprehensive program is tailored to provide a deep understanding of Clinical SAS and its various features. The curriculum i#analytics#reporting#and graphical presentations#catering to both beginners and advanced learners.#Why Choose Unicode Healthcare Services for Clinical SAS Training?#Our team of expert instructors#with over 7 years of experience in the Pharmaceutical and Healthcare industries#ensures that students gain practical knowledge along with theoretical concepts. Using real-world examples and hands-on projects#we prepare our learners to effectively use Clinical SAS in various professional scenarios.#About Clinical SAS Training#Clinical SAS is a powerful statistical analysis system widely used in the Pharmaceutical and Healthcare industries to analyze and manage cl#and reporting.#The program includes both classroom lectures and live project work#ensuring students gain practical exposure. By completing the training#participants will be proficient in data handling#creating reports#and graphical presentations.#Course Curriculum Highlights#Our Clinical SAS course begins with the fundamentals of SAS programming#including:#Data types#variables#and expressions#Data manipulation using SAS procedures#Techniques for creating graphs and reports#Automation using SAS macros#The course also delves into advanced topics like CDISC standards
0 notes
Text
#R Programming Assignment Help#R Programming Homework Help#Expert Help with R Programming Assignments#Online R Programming Homework Solutions#Custom R Programming Assignment Assistance#R Programming Data Analysis Help#Professional R Programming Tutors Online#Help with R Programming Projects#Affordable R Programming Assignment Support#R Programming Statistical Analysis Help#R Coding Assignment Help#Debugging R Programming Homework#Advanced R Programming Solutions#Machine Learning with R Assignment Help#R Programming Assistance for Students
0 notes
Text
Learn Advanced Excel for Data Analysis | Master Excel Skills for Insights
Unlock the full potential of Microsoft Excel by learning advanced techniques tailored for data analysis. This comprehensive course dives deep into powerful Excel features like PivotTables, advanced formulas, Power Query, Power Pivot, data visualization, and what-if analysis. Designed for professionals, analysts, and students, this training will equip you with the skills to clean, manipulate, and interpret complex datasets with ease. Whether you're preparing business reports, forecasting trends, or making data-driven decisions, mastering advanced Excel functions will give you the edge you need in today’s data-driven world. Start your journey to becoming an Excel power user and transform raw data into actionable insights.
0 notes