#quadratic scaling
Explore tagged Tumblr posts
Text
X spells in magic the gathering are where the fact it was designed by a maths guy really pops. Majority of the maths in magic is "can I pay cost" or "is damage dealt enough to kill a creature/player", so playing around with that number being variable rather than fixed makes some sense, and maths has X as a default variable, so you get fireball
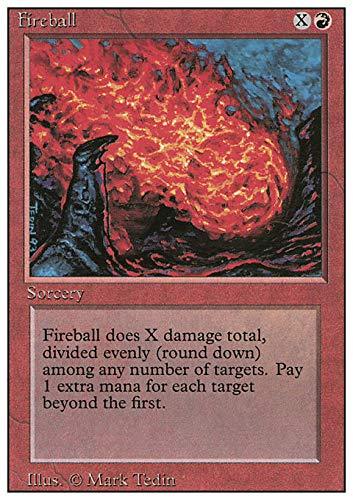
right there in the first set - variable cost for variable damage, 1:1. This spell is great for ending games, which makes up for it's poor rate for the time (see lightning bolt, where you pay 1 mana for 3 damage)
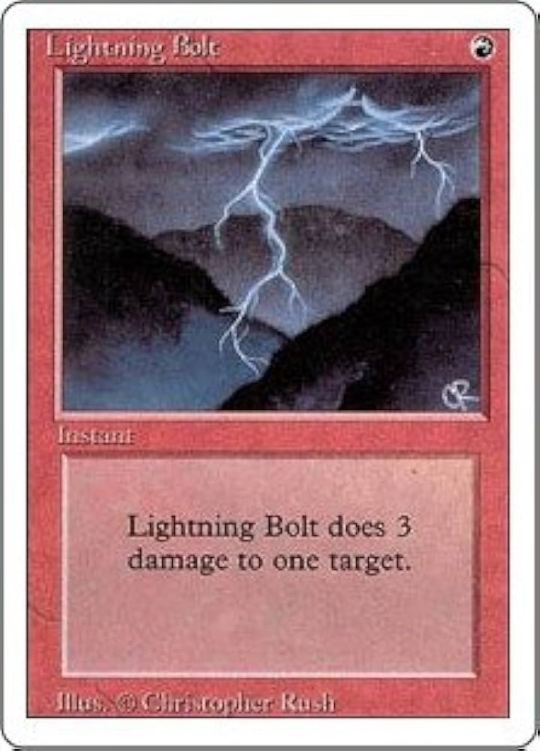
So now you can use X for the cost to give a linear scaling effect, such as additional counters on creatures
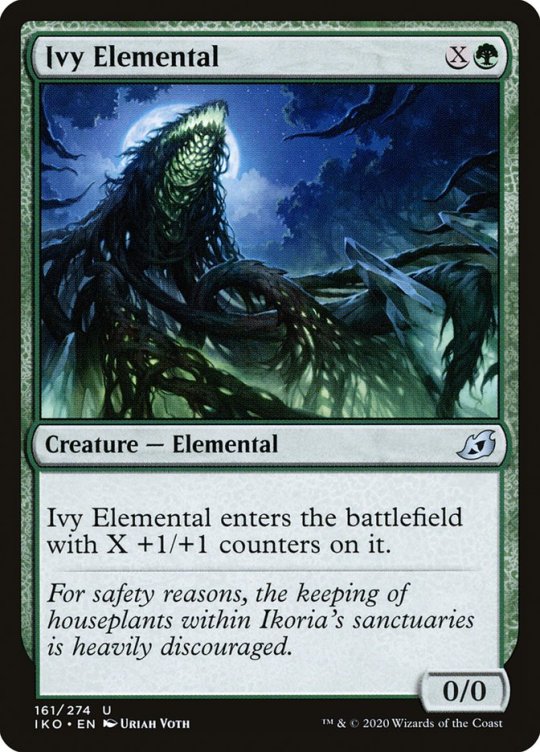
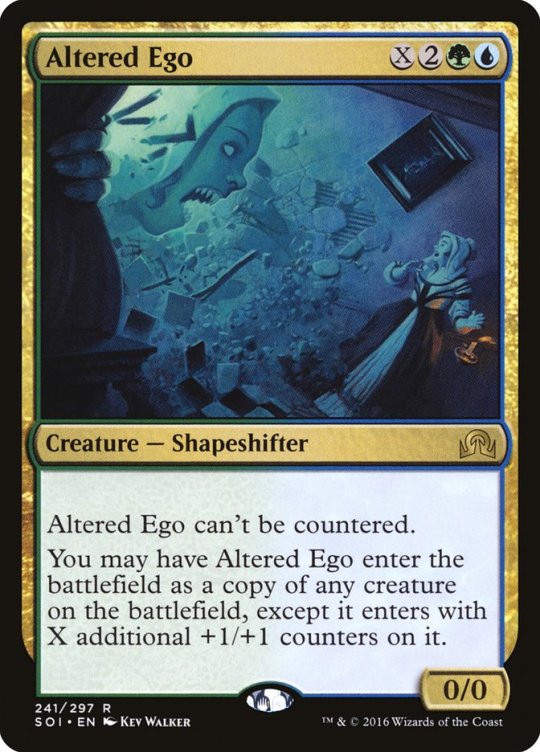
Where we get really mathsy next is the relatively few examples of effectively "do something X times, X times" for example:
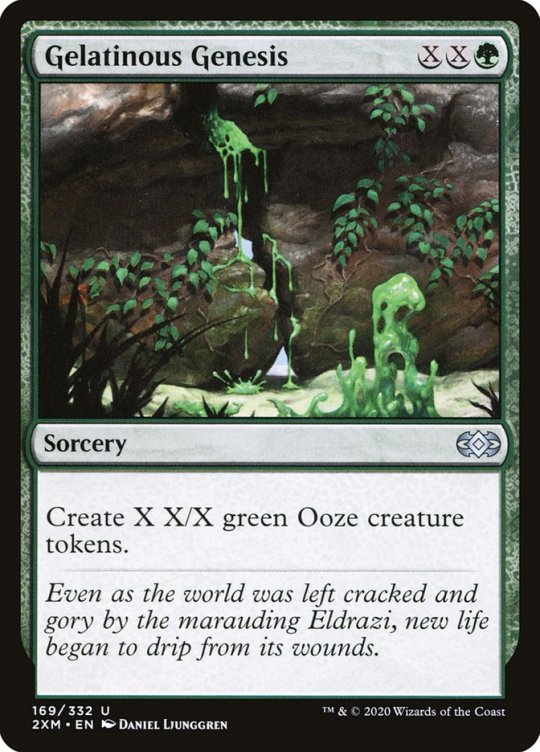
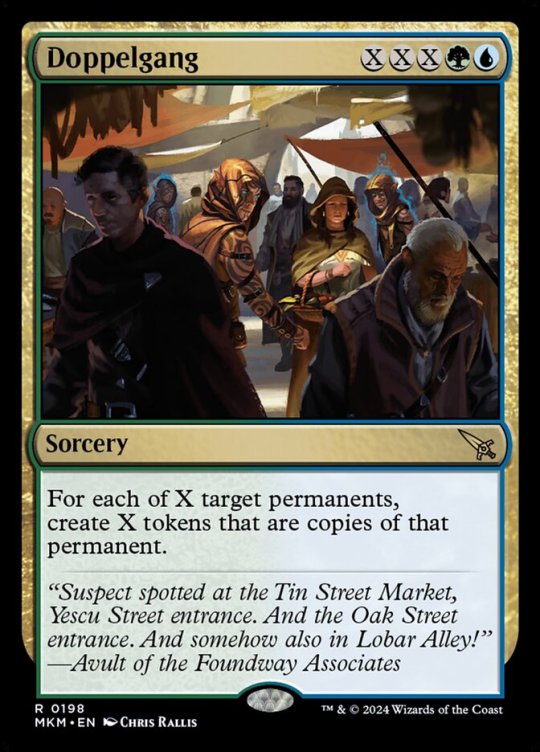
These two cards are roughly equivalent in what they do to the previous two - but appear to scale at a much worse rate, having to pay two or three times the cost for each time you do "X" but the trick here is these aren't actually scaling linearly, they're scaling quadratically, making them much more effective than they seem.
Anyway all this to say that sometimes evaluating how good a magic card can require understanding quadratic scaling!*
*but almost never will because these effects are almost certainly intentionally costed too highly and attached to traditionally uncompetitive effects, likely so that players don't actually have to think about quadratic scaling. This is probably for the best. Also there's one card that scales exponentially (2 to the power of X) rather than quadratically (X to the power of 2). It's literally called exponential growth.
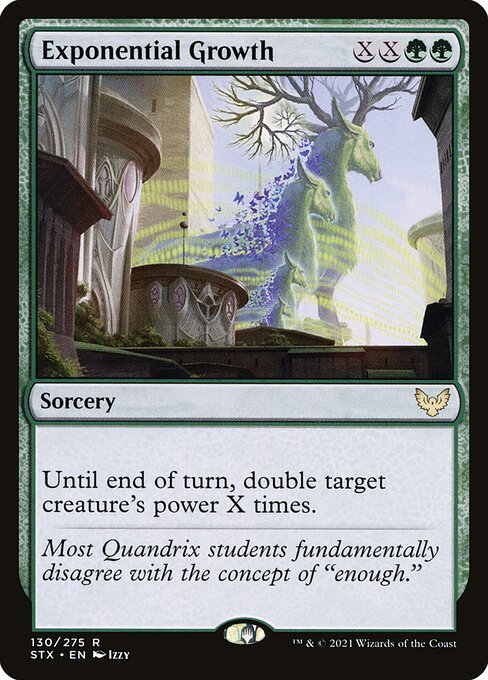
#mtg#magic the gathering#quadratic scaling#richard Garfield phd was a maths professor but maths guy gets the point across#exponential growth#maths#just throwing tags on here. hashtag whatever#i hope this auto does read more. sorry if this has taken forever to scroll past.
50 notes
¡
View notes
Text
Ukugyps orcesi Lo Coco et al., 2024 (new genus and species)
(Type quadrate [bone in the back of the skull] of Ukugyps orcesi [scale bar = 10 mm], from Lo Coco et al., 2024)
Meaning of name: Ukugyps = reign of death [in Quechua] vulture [in Latin]; orcesi = for Gustavo OrcĂŠs VillagĂłmez [Ecuadorian biologist]
Age: Pleistocene (Late Pleistocene), between 17,800â19,000 years ago
Where found: Tablazo Formation (La Carolina tar deposits), Santa Elena Province, Ecuador
How much is known: A partial right quadrate (bone in the back of the skull).
Notes: Ukugyps was an American vulture, around the size of the extant king vulture (Sarcoramphus papa). Although it is based on very little fossil material, it can be distinguished from all other Pleistocene American vultures for which the quadrate is known.
Reference: Lo Coco, G.E., F.L. AgnolĂn, and J.L.R. CarriĂłn. 2024. New records of Pleistocene birds of prey from Ecuador. Journal of Ornithology advance online publication. doi: 10.1007/s10336-024-02229-1
32 notes
¡
View notes
Text
I really wish there was an "any standard" format in magic. It feels like it would very neatly address the issues of non-rotating formats having to deal with a quadratically-scaling number of interactions that make these formats have absurd power levels, while still allowing new cards and strategies to enter the meta. I think it would prevent older decks from being permanently power-scaled out of the meta, because old cards aren't directly competing against new cards -- cards can only be directly replaced by cards from the same standard, so many weaker older cards can be carried by stronger cards from the same standard, hopefully leading to greater deck diversity.
13 notes
¡
View notes
Text
scaling up the context window by eliminating quadratic overhead of the transformer architecture?
77 notes
¡
View notes
Note
this isnât a husbandry question, but do you know why snakes are considered only a suborder taxonomically rather than a full order? It seems like the order squamates is diverse enough that Iâd expect it be split into one order for snakes and another for lizards
The general consensus among herpetologists is that squamates, while a very diverse group, have similarities strong enough that it's more useful to consider them all part of the same order rather than split it up. There are actually three suborders in Squamata: snakes, lizards, and amphisbaenians. They all have things that tell us they're members of the same lineage:
Scales. Squamates are also called "scaled reptiles" becuase they all have scaly skin that must be shed every so often. This makes squamates distinct from other reptiles, like crocodilians, who have scutes instead.
Kinetic quadrate bones - they all have bones in their skulls that make it possible to move the upper jaw independently from the rest of the skull. Not every squamate can, but their skull structure makes it possible.
The potential for venom. Not every squamate is venomous (not by a long shot, only about 15% of snakes are venomous and that number is even smaller for lizards), but something like 60% of all squamates belong to clades with venomous members somewhere in their lineage.
Taken all together, these three things that all squamates share tell us that they are extremely closely related and considering them as one order altogether can tell us a great deal about how all squamates evolved. If we considered snakes as their own order instead of a suborder, then we'd lose some perspective on how interrelated all three suborders are.
If we take a look at the single sister order to Squamata, the rhynchocephalians, we can see why no squamates are distinct enough to warrant becoming their own order. Rhynchocephalians, unlike all squamates, have a distinct tooth type, have fused skull bones, do not have the potential for venom, and have a unique skeletal structure including hourglass-shaped vertebrae and gastralia. Snakes are a little different from other squamates, but they're not that different!
95 notes
¡
View notes
Text
CUPID đđš
IN WHICH our cast plays cupid for their loser (endearingly) friends who canât seem to take their own relationship advice.
CHAPTER 002. sneaky little tax evader (smau + written) đ§

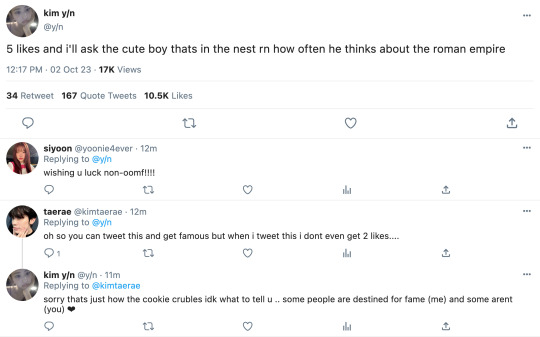
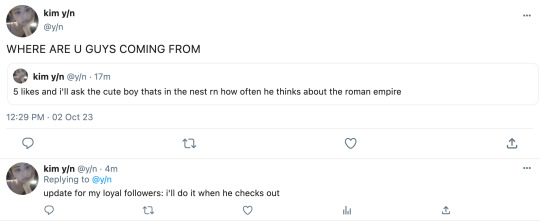
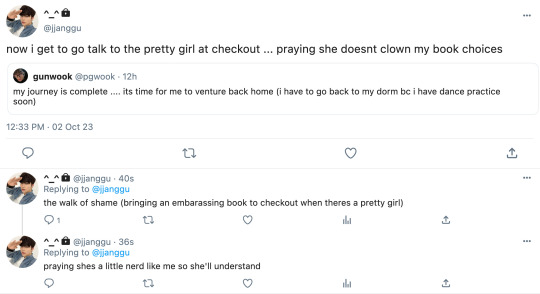
âHI!â you greet the boy that youâve been not-so-secretly eyeing for the past hour and a half.
âHi!â he smiles. you internally melt and almost donât notice the hands belonging to the boy in front of you that are giving you a book to check out. He doesnât break eye contact once. Usually, that would be a little odd and would kind of sketch you out but thereâs just something about his big brown eyes that makes you feel safe.
âWill that be all for you today?â you ask. Youâre the one to finally break eye contact, but only to quickly look down so you can locate the barcode and scan it. Your eyes graze over the cover, âThe Crisis of the 3rd Century? Interesting choiceâŚâ You must have a quizzical look on your face because he laughs and itâs the most beautiful sound you think youâve ever heard.Â
âI know. It's one of my favorite things to whip out at trivia night. No one ever sees it coming! First, itâs the Quadratic Formula ⌠easy peasy ⌠and then Boom! Itâs the near collapse of Ancient Rome! Well, that and the fact that Henry David Thoreau committed tax evasion. Multiple times.âÂ
âOh my gosh, no way⌠no one ever knows about that! Every time I reference it, my friends think I'm some sort of nerd. Not that youâre a nerd or anything.â you couldâve rambled on and on but a thought pops up and stops you in your tracks. âI'm sorry, I just have to ask. Exactly how often do you think about the Roman Empire, and Iâm not talking about The Gladiator (2000) with Russell Crowe all oiled up Roman Empire. Iâm talking like actual Roman Empire?â
âTrust me ⌠you donât want to know. â the pretty, brown haired boy replies while trying his best to look serious. It doesnât work though because a few seconds later, he bursts into the same laughter as before and you swear your world stops for a second. All you can do is pray that he doesnât hear your heart about to beat right out of your chest. In an attempt to hide your rosy cheeks, you look away from him and towards the register which now displays his total.Â
âYour total is going to be $15.89 today, cash or card?â
He pulls out a $20 from what seems to be an off-brand Lightning McQueen wallet and hands it to you. You take it, put it in the register, and give him back his change. He takes it from you with a smile that youâre convinced could bring world peace and drops all $4.11 into the tip jar sitting on the counter in front of you. He says âHave a good evening.â to which you reply âyou too.â and watch him walk out the door, bag and cup in hand.
âWoah, your cheeks are redder than a tomato. Be honest, on a scale of 1 to 10, how down bad are you?â Taerae, your best friend, questions as he walks over from his post at Bluebird, the in-store cafe that sits right by the checkout and also happens to give him the perfect view of what just went down.
You sigh and dramatically place a hand to your forehead as if youâre swooning. âI fear Iâm in love and I donât even know his name.â
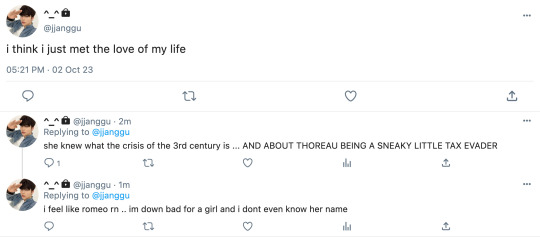
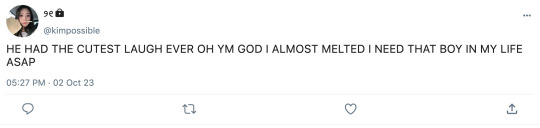
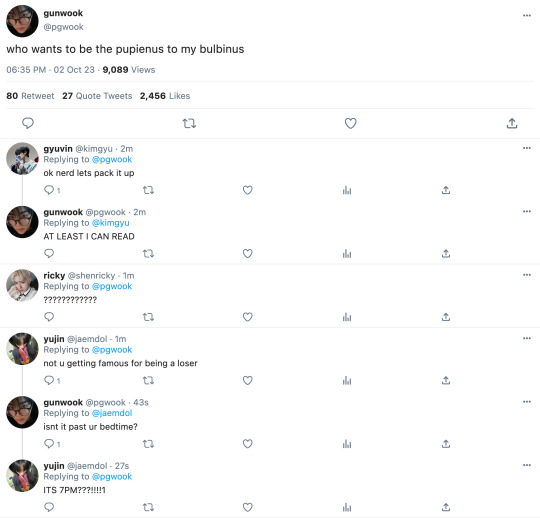
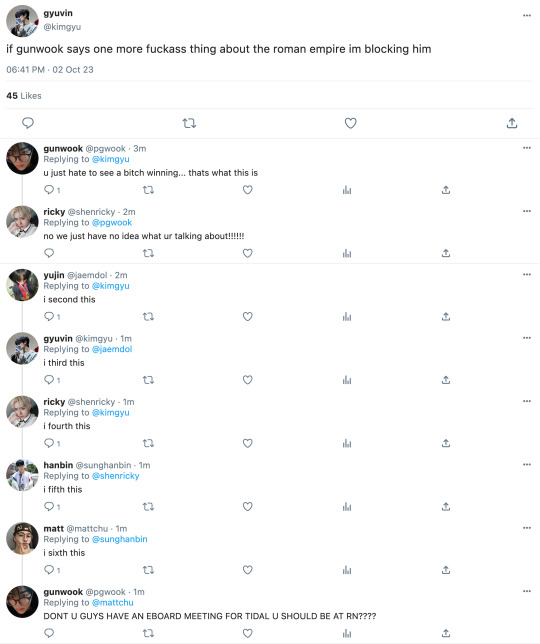
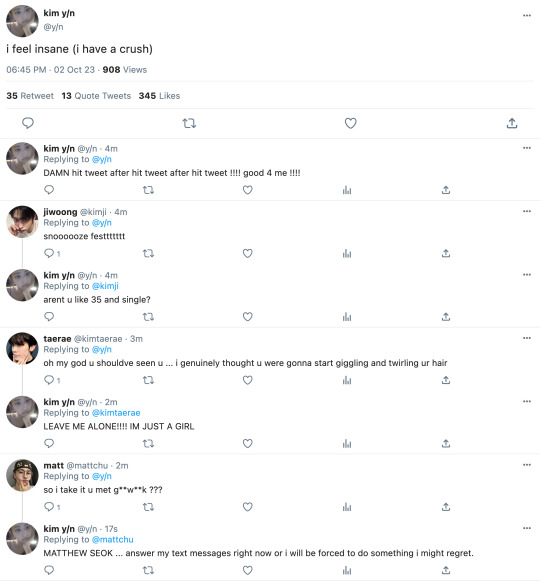

đ â
mlist. previous. next.

ââ
TAGLIST:Â @annoyingbitch83 @vernonburger @doiedecimal @taekwondoes @imthisclosetokms @kaynunu (bold can't be tagged ; pls check your settings + make sure u can be tagged ... ty ^_^)
want to join? -> taglist form . đŤ

#prkwook#002. cupid đ đš#zerobaseone#park gunwook#zb1 gunwook#gunwook x reader#park gunwook imagines#park gunwook x reader#zb1 fluff#zerobaseone imagines#zb1 x reader#zerobaseone fluff#zerobaseone x reader#gunwook fluff#park gunwook smau#gunwook imagine#gunwook smau#zb1 imagines#zb1 scenarios#zb1 smau#zb1#zerobaseone smau#cupid đ đš
46 notes
¡
View notes
Quote
Life is not an orderly progression, self-contained like a musical scale or a quadratic equation... If one is to record one's life truthfully, one must aim at getting into the record of it something of the disorderly discontinuity which makes it so absurd, unpredictable, bearable.
Leonard Woolf
#Leonard Woolf#quotelr#quotes#literature#lit#biography#bloomsbury#bloomsbury-group#leonard-woolf#life#writing
3 notes
¡
View notes
Text
Stanotte ho fatto un sogno abbastanza particolare Era sera e mi trovavo a casa mia, a primo piano, c'erano tanti amici e parenti vari Ad un certo punto vedo che è già orario di cena e mi ricordo che i miei cugini avevano prenotato in un locale Mi affaccio al balcone e vedo che i miei suddetti cugini sono giÚ per strada, già in auto Quindi, invece di scendere dalle scale di casa e uscire, per fare prima scavalco la ringhiera del balcone e mi butto giÚ Il fatto è che nel sogno, non era una cosa nuova per me ma qualcosa che avevo già fatto in passato e a cui ero abituato, inoltre i miei genitori che mi guardavano farlo erano piuttosto tranquilli CosÏ mi lancio dal balcone, atterro in piedi facilmente e senza dolore, tutto a posto Cerco di entrare in auto che c'è mio fratello ma fa il classico scherzetto di accelerare un po' ogni volta che sto per entrare, nel mentre che lo sportello resta aperto, anche se per lui era per sbrigarsi, cioè per non tenere l'auto ferma Ad una certa infatti mi rompo e faccio un tratto a piedi Finalmente si arriva in questo locale, entriamo ed è terribile... à una stanza sola molto grande con un lungo tavolo tutto per noi, però sembra tutto molto vecchio e molto trascurato, il pavimento fatto interamente con mattonelle quadrate, è molto irregolare, pieno di buche e con diverse mattonelle mancanti In tutto ciò oltre a noi cugini c'erano altri conoscenti che dovevano mangiare con noi, due delle quali stavano litigando abbastanza intensamente ed erano una bambina e una ragazza adulta che era amica della madre della bambina Però in realtà al di fuori del sogno non so chi siano, non so nemmeno se esistano davvero Io mi sposto dalla stanza del locale verso fuori, per non sentire la litigata piÚ che altro, anche il locale ha un balcone e anche il locale è a primo piano proprio come casa mia CosÏ mi affaccio e guardo per strada e vedo che i miei cugini sono rimasti a piano terra che stavano aiutando qualcuno a spostare cose, forse i gestori del locale Allora penso, piuttosto che stare qui ad aspettare e sentire quelle che litigano, scendo giÚ e li aiuto e anche in questo caso mi verrebbe da buttarmi giÚ dal balcone, ma alla fine non faccio nulla e il sogno finisce lÏ
2 notes
¡
View notes
Text
Tag yourself Fatui cabbage addition!!

Goodest boy cabbage
- good cook
- very wholesome
- likes to stab things
- has never done anything wrong ever
- orange
Greedy cabbage
- âI like moneyâ
- loan shark
- probably has teeth marks on his cabb-ose
- Dottore ate his ass is what Iâm saying
Cotton-candy cabbage
- blue
- very soft and fluffy
- sharp teeth (likes to bite)
- secretly kidnaps baby cabbages in the dead of night to throw them into a pot and test new stew recipes. I can still hear their screams.
- very soft and fluffy
Cow cabbage
- bites H A R D
- quadratic scaling but actually he just multiplies indefinitely
- is a plague
- heeheeemoomoo
Robo-cabbage
- the actual cabbage is the ear
- uses the bodies of dead cabbages to form her taxi cabbage
- always annoyed
Angel cabbage đĽşđâ¤ď¸đĽ°đ
- can manipulate minds (allegedly)
- the most beautiful singer and adorable being to ever bless cabbagekind
- likes scrapbooking
Arleccabbage
- the chef probably barks for her
- but what else is new
- hidden stash of baby cabbages she uses to feed the dottores
- does not like scapbooking
Daddage
- old man but cabbage
- slightly past expiration date
- found a gremlin cabbage outside the garden
- âorange is a normal cabbage color, he wonât taste any different. Dottore, stop trying to eat him.â
- orange is not a normal cabbage color
Dilf cabbage
- got nommed on
- was not very tasty
- might be rotting
Cabbatano
- is that actually a cabbage /gen /srs
- I think itâs looking at me
- help
Ghost cabbage
- like a ghost pepper
-except cabbage
- hot like pepper, dead like ghost
- remembers the cabbaclysm
- rest in peppers
Missingno.
- guys I swear there was another cabbage here
#genshin impact#Fatui#fatui harbingers#except theyâre cabbages#pierro genshin#Dottore#capitano#Columbina#damslette#Pulcinella#scaramouche#wanderer genshin#sandrone#Signora#la signora#pantalone#regrator#Arlecchino#the knave#tartaglia#childe
45 notes
¡
View notes
Text
Interesting Papers for Week 15, 2023
Cross-scale excitability in networks of quadratic integrate-and-fire neurons. Avitabile, D., Desroches, M., & Ermentrout, G. B. (2022). PLOS Computational Biology, 18(10), e1010569.
Modulation of working memory duration by synaptic and astrocytic mechanisms. Becker, S., Nold, A., & Tchumatchenko, T. (2022). PLOS Computational Biology, 18(10), e1010543.
Mathematical relationships between spinal motoneuron properties. Caillet, A. H., Phillips, A. T., Farina, D., & Modenese, L. (2022). eLife, 11, e76489.
The pupillometry of the possible: an investigation of infantsâ representation of alternative possibilities. Cesana-Arlotti, N., Varga, B., & TĂŠglĂĄs, E. (2022). Philosophical Transactions of the Royal Society B: Biological Sciences, 377(1866).
Olfactory responses of Drosophila are encoded in the organization of projection neurons. Choi, K., Kim, W. K., & Hyeon, C. (2022). eLife, 11, e77748.
Postsynaptic burst reactivation of hippocampal neurons enables associative plasticity of temporally discontiguous inputs. Fuchsberger, T., Clopath, C., Jarzebowski, P., Brzosko, Z., Wang, H., & Paulsen, O. (2022). eLife, 11, e81071.
Immature olfactory sensory neurons provide behaviourally relevant sensory input to the olfactory bulb. Huang, J. S., Kunkhyen, T., Rangel, A. N., Brechbill, T. R., Gregory, J. D., Winson-Bushby, E. D., ⌠Cheetham, C. E. J. (2022). Nature Communications, 13(1), 6194.
Humans Can Track But Fail to Predict Accelerating Objects. Kreyenmeier, P., Kämmer, L., Fooken, J., & Spering, M. (2022). ENeuro, 9(5).
Ventrolateral Prefrontal Cortex Contributes to Human Motor Learning. Kumar, N., Sidarta, A., Smith, C., & Ostry, D. J. (2022). ENeuro, 9(5).
Magnitude-sensitive reaction times reveal non-linear time costs in multi-alternative decision-making. Marshall, J. A. R., Reina, A., Hay, C., Dussutour, A., & Pirrone, A. (2022). PLOS Computational Biology, 18(10), e1010523.
Differences in temporal processing speeds between the right and left auditory cortex reflect the strength of recurrent synaptic connectivity. Neophytou, D., Arribas, D. M., Arora, T., Levy, R. B., Park, I. M., & Oviedo, H. V. (2022). PLOS Biology, 20(10), e3001803.
Structured random receptive fields enable informative sensory encodings. Pandey, B., Pachitariu, M., Brunton, B. W., & Harris, K. D. (2022). PLOS Computational Biology, 18(10), e1010484.
Obsessive-compulsive disorder is characterized by decreased Pavlovian influence on instrumental behavior. Peng, Z., He, L., Wen, R., Verguts, T., Seger, C. A., & Chen, Q. (2022). PLOS Computational Biology, 18(10), e1009945.
The value of confidence: Confidence prediction errors drive value-based learning in the absence of external feedback. Ptasczynski, L. E., Steinecker, I., Sterzer, P., & Guggenmos, M. (2022). PLOS Computational Biology, 18(10), e1010580.
Psychedelics and schizophrenia: Distinct alterations to Bayesian inference. Rajpal, H., Mediano, P. A. M., Rosas, F. E., Timmermann, C. B., Brugger, S., Muthukumaraswamy, S., ⌠Jensen, H. J. (2022). NeuroImage, 263, 119624.
Visual working memory recruits two functionally distinct alpha rhythms in posterior cortex. Rodriguez-Larios, J., ElShafei, A., Wiehe, M., & Haegens, S. (2022). ENeuro, 9(5).
Pitfalls in post hoc analyses of population receptive field data. Stoll, S., Infanti, E., de Haas, B., & Schwarzkopf, D. S. (2022). NeuroImage, 263, 119557.
Event-related microstate dynamics represents working memory performance. Tamano, R., Ogawa, T., Katagiri, A., Cai, C., Asai, T., & Kawanabe, M. (2022). NeuroImage, 263, 119669.
Rule-based and stimulus-based cues bias auditory decisions via different computational and physiological mechanisms. Tardiff, N., Suriya-Arunroj, L., Cohen, Y. E., & Gold, J. I. (2022). PLOS Computational Biology, 18(10), e1010601.
Correcting the hebbian mistake: Toward a fully error-driven hippocampus. Zheng, Y., Liu, X. L., Nishiyama, S., Ranganath, C., & OâReilly, R. C. (2022). PLOS Computational Biology, 18(10), e1010589.
#science#Neuroscience#computational neuroscience#Brain science#research#cognition#cognitive science#neurons#neural networks#neural computation#neurobiology#psychophysics#scientific publications
23 notes
¡
View notes
Text
genshin spiderbit
Cellbit - dendro catalyst, 3 attack string, 2 modes, 'pen' mode and 'knife' mode, gets stacks when attacking with pen mode that are used up to buff when the skill is used. burst has the same 2 modes, pen mode gives buffs to the team and knife mode augments his own damage and does lots of damage. damage mainly comes from autos. when has 3 stacks of pen, if the character is changed and is a meele weapon, their attacks will be imbued with dendro. if 'knife' autos come in contact with pyro they will deal extra dendro damage depending on the number of stacks. his autos also have a slightly smaller icd (1 sec instead of 2.5, every other attack instead of every third)
Roier - pyro sword, 4 attack string, each button press is 2 damage instances, skill is a dash like yelan that marks and connects the enemies in a web-like thing. the web periodically pulses, dealing pyro damage and pulling the enemies towards the center of the web. without anything, the web pulses 3 time (7.5 secs), but if a burning or pyro swirl reaction is triggered the duration of the web is extended, up to 25 secs. burst is coordinated attacks like xingqiu or yelan for around 7 seconds. if the attacks hit an enemy marked by the web, the web will pulse, and when the burst ends, the web timer is reset. has a lot about quadratic scaling in his kit
#qsmp#qsmp cellbit#qsmp roier#just posting for the sake of posting#i need to think about the passives scaling the ascension stats...#ill prolly do it other day#genshin impact#genshin#shiros genshin fankit
8 notes
¡
View notes
Note
đť
(Oh man, the mortifying ordeal of actually having to pick something to talk about when I have so many ideas...)
Uh, OK, I'm talking about galactic algorithms, I've decided! Also, there are some links peppered throughout this post with some extra reading, if any of my simplifications are confusing or you want to learn more. Finally, all logarithms in this post are base-2.
So, just to start from the basics, an algorithm is simply a set of instructions to follow in order to perform a larger task. For example, if you wanted to sort an array of numbers, one potential way of doing this would be to run through the entire list in order to find the largest element, swap it with the last element, and then run though again searching for the second-largest element, and swapping that with the second-to-last element, and so on until you eventually search for and find the smallest element. This is a pretty simplified explanation of the selection sort algorithm, as an example.
A common metric for measuring how well an algorithm performs is to measure how the time it takes to run changes with respect to the size of the input. This is called runtime. Runtime is reported using asymptotic notation; basically, a program's runtime is reported as the "simplest" function which is asymptotically equivalent. This usually involves taking the highest-ordered term and dropping its coefficient, and then reporting that. Again, as a basic example, suppose we have an algorithm which, for an input of size n, performs 7nÂł + 9n² operations. Its runtime would be reported as Î(nÂł). (Don't worry too much about the theta, anyone who's never seen this before. It has a specific meaning, but it's not important here.)
One notable flaw with asymptotic notation is that two different functions which have the same asymptotic runtime can (and do) have two different actual runtimes. For an example of this, let's look at merge sort and quick sort. Merge sort sorts an array of numbers by splitting the array into two, recursively sorting each half, and then merging the two sub-halves together. Merge sort has a runtime of Î(nlogn). Quick sort picks a random pivot and then partitions the array such that items to the left of the pivot are smaller than it, and items to the right are greater than or equal to it. It then recursively does this same set of operations on each of the two "halves" (the sub-arrays are seldom of equal size). Quick sort has an average runtime of O(nlogn). (It also has a quadratic worst-case runtime, but don't worry about that.) On average, the two are asymptotically equivalent, but in practice, quick sort tends to sort faster than merge sort because merge sort has a higher hidden coefficient.
Lastly (before finally talking about galactic algorithms), it's also possible to have an algorithm with an asymptotically larger runtime than a second algorithm which still has a quicker actual runtime that the asymptotically faster one. Again, this comes down to the hidden coefficients. In practice, this usually means that the asymptotically greater algorithms perform better on smaller input sizes, and vice versa.
Now, ready to see this at its most extreme?
A galactic algorithm is an algorithm with a better asymptotic runtime than the commonly used algorithm, but is in practice never used because it doesn't achieve a faster actual runtime until the input size is so galactic in scale that humans have no such use for them. Here are a few examples:
Matrix multiplication. A matrix multiplication algorithm simply multiplies two matrices together and returns the result. The naive algorithm, which just follows the standard matrix multiplication formula you'd encounter in a linear algebra class, has a runtime of O(n³). In the 1960s, German mathematician Volker Strassen did some algebra (that I don't entirely understand) and found an algorithm with a runtime of O(n^(log7)), or roughly O(n^2.7). Strassen's algorithm is now the standard matrix multiplication algorithm which is used nowadays. Since then, the best discovered runtime (access to paper requires university subscription) of matrix multiplication is now down to about O(n^2.3) (which is a larger improvement than it looks! -- note that the absolute lowest possible bound is O(n²), which is theorized in the current literature to be possible), but such algorithms have such large coefficients that they're not practical.
Integer multiplication. For processors without a built-in multiplication algorithm, integer multiplication has a quadratic runtime. The best runtime which has been achieved by an algorithm for integer multiplication is O(nlogn) (I think access to this article is free for anyone, regardless of academic affiliation or lack thereof?). However, as noted in the linked paper, this algorithm is slower than the classical multiplication algorithm for input sizes less than n^(1729^12). Yeah.
Despite their impracticality, galactic algorithms are still useful within theoretical computer science, and could potentially one day have some pretty massive implications. P=NP is perhaps the largest unsolved problem in computer science, and it's one of the seven millennium problems. For reasons I won't get into right now (because it's getting late and I'm getting tired), a polynomial-time algorithm to solve the satisfiability problem, even if its power is absurdly large, would still solve P=NP by proving that the sets P and NP are equivalent.
Alright, I think that's enough for now. It has probably taken me over an hour to write this post lol.
5 notes
¡
View notes
Text
The grades I think I would get if I was in the pirate training program
Bbad spelling: 10/10 I would fucking ace that class. My spelling is so horrible that in fourth grade I was put in the âspecial classâ for spelling. I canât spell their properly for fuckâs sake, I always misspell it as thier.Â
Basic burglary: 6/10 I think Iâd be pretty good at stealing and even better at gaslighting the victims like âWhere is your bracelet? I havenât seen it. No, you didnât put it on today. Oh, this? This was a gift from my cousin. Why does it have your name? It's a hand me down duh, my cousin has the same name as you. If youâre that upset about losing your bracelet, I can help you look for it, because Iâm just that nice.â
Baseball: 0/10 I will activate my quiet, nerd, introvert cloak of invisibility (standing so still that adults forget I exist)Â during all sports. Itâs worked before, it will work again.Â
Beginner Burping: 2/10 I could do it, just not very well.
Boarding an enemy ship: 1.5/10 - 6/10 This one sounds like it requires a lot of stamina and depending on if Iâm in a more manic or depressive episode my grade would fluctuate. Â
Dragon training: 1/10 I would have a bunch of fun debating my dragon about the meaning of life and free will. I would not train the dragon, I would educate them. My dragon would be able to do quadratics and tell you why true democracy can never work on a large scale, but would not be able to catch fish.
Frightening foreigners: 5/10 I can be scary when I want to be, however my brand of frightening isâŚdifferent from the vikings. It would also require quite a bit of effort, half the time Iâd just end up being a resting bitch face nihilist.Â
Hammer throwing: 1.5 /10 I have been working out regularly, so I could probably pick up the hammer and toss it like ½ a foot in front of me, but not much more.Â
Herding reindeer on dragonback: 3.5/10 I would be so-so at it (a bit below because I donât love getting near large animals)Â
Herding sheep: 4/10 I feel like sheep would be easier to get closer to.Â
Hunting-With-Bows-and-Arrows-on-Skis: 1/10 Iâm a shit archer but not a terrible skier (which would be the only thing saving me from a 0/10)Â
Pointless graffiti: 8/10 I would get 2 points taken off for accidentally creating moving and meaningful graffiti.Â
Robbery: 4.5/10: I am good at stealth and plans, but I can be clumsy.Â
Senseless Violence: 5/10 I am not normally a violent person, but if need be I wonât hesitate to throw hands. My older brother would fight with me when we were kids for fun. I also have anger issues.Â
Shipbuilding: 4/10 I would not follow the instructions but through sheer luck, determination, and will power would get a working boat, that is simultaneously an artistic masterpiece.Â
Shouting: 1.5/10 I literally canât raise my voice nine times out of ten because of my trauma. Iâd also probably start crying in the middle of class.Â
Spitting: 0/10 Iâm not even trying on this one.Â
Sword Fighting: 4/10 Iâd be average at it (just slightly below)
Sword Fighting at Sea: 3/10 Iâd be even worse due to the rockiness of the boat.Â
Advanced rudery: 7/10 I âŚwatch way too much r/roast me.Â
Weaponry: 2/10 Iâm not sure what this class entails but I doubt Iâd be good at it.Â
15 notes
¡
View notes
Photo
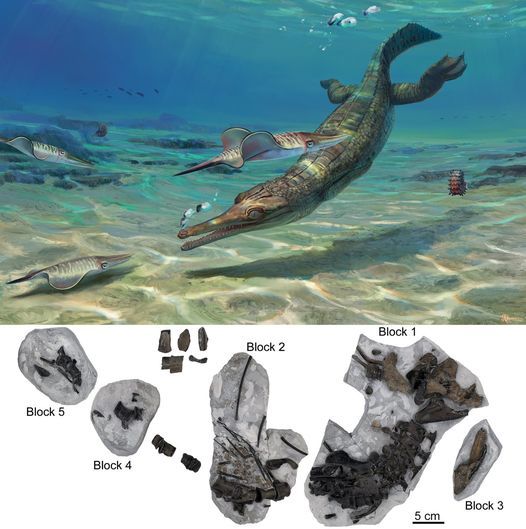
A new early diverging thalattosuchian (Crocodylomorpha) from the Early Jurassic (Pliensbachian) of Dorset, U.K. and implications for the origin and evolution of the group
Eric W. Wilberg,Pedro L. Godoy,Elizabeth F. Griffiths,Alan H. Turner &Roger B. J. Benson
ABSTRACT
Among archosaurs, thalattosuchian crocodylomorphs experienced the most extensive adaptations to the marine realm. Despite significant attention, the phylogenetic position of the group remains uncertain. Thalattosuchians are either the sister-group to Crocodyliformes, basal mesoeucrocodylians, or nest among longirostrine neosuchians.Â
The earliest definite thalattosuchians are Toarcian, and already possess many synapomorphies of the group. All phylogenetic hypotheses imply a ghost lineage extending at least to the Sinemurian, and a lack of older or more plesiomorphic forms may contribute to the uncertain phylogenetic placement of the group.Â
Here we describe a new species, Turnersuchus hingleyae, gen. et sp. nov., from the early Pliensbachian Belemnite Marl Member of the Charmouth Mudstone Formation (Dorset, U.K.). The specimen includes partially articulated cranial, mandibular, axial, and appendicular elements. It can be attributed to Thalattosuchia based on the following features: distinct fossa on the posterolateral corner of the squamosal; broad ventrolateral process of the otoccipital covering the dorsal surface of the quadrate; large supratemporal fenestrae lacking a flattened skull table; broadly exposed prootic; orbital process of quadrate lacking bony attachment with the braincase. This specimen represents the earliest thalattosuchian currently known from diagnostic material.Â
Phylogenetic analyses of two published datasets recover Turnersuchus as the earliest diverging thalattosuchian, and sister to Teleosauroideaâ+âMetriorhynchoidea. Bayesian tip-dating analyses suggest a Rhaetian or Sinemurian divergence of Thalattosuchia from other crocodylomorphs, depending on topology, with confidence intervals spanning from the Norian to the Pliensbachian.Â
The new specimen extends the fossil record of Thalattosuchia, but the time-scaling analyses demonstrate that a significant ghost lineage remains.
Read the paper here:
https://www.tandfonline.com/doi/abs/10.1080/02724634.2022.2161909
23 notes
¡
View notes
Text
in wow im like hmm ok given unholy death knight's quadratic scaling i need to save army for a large pull and also save mirror for army and use unholy assault right at the start so i have enough wounds to instantly pop apoc and get the 4set bonus magi and make sure to put defile down before building my wounds back up to maximize cleave and then in bg3 ive just been like idfk man one of you go over there and handle it
#and then gale dies#and i pop all my health pots to get through a single encounter#ive put all my points into zugzugging high apm gameplay and now in turn based i feel like a fucking caveman#the udk gameplay described above happens in like 5-10 seconds at the start of a pull i loooooove it
4 notes
¡
View notes
Text
Hausdorff dimension in fractals
Doubling a line makes 2 lines. Doubling a square makes 4 squares. Doubling a cube makes 8 cubes.
Have you heard of the square-cube law? If you double an object's linear dimensions its volume is multiplied by 8, but its surface area is multiplied by 4. For an object of dimension D, doubling its linear dimensions multiplies the object by 2^D. D is the object's Hausdorff dimension. Unlike definitions of dimension that rely on orthogonality, D can take non-integer values. And it does, for some of your favorite fractals!
This SierpiĹski gasket is made of 3 subunits, each of which has half its linear dimensions. That means when you double a gasket's linear dimensions, you triple its area. That's more than a line gets but less than a square gets. The gasket has dimension log_2(3) â 1.585. This fractal is one-and-a-half dimensional!
One gets the sense that SierpiĹski's carpet is more substantial than his gasket. It's composed of 8 subunits at 1:3 scale, so 3^D = 8 and D â 1.893. It's almost 2-dimensional. Much closer than the gasket at least.
Oh god, act casual. It's elementary automaton #150. Want to take a crack at it? Answer under the cut.
This animal is made of 2 half-scale subunits and 4 quarter-scale subunits. If we let the little subunits have area 1, the big subunits have 2^D and the whole shebang is 4^D. We obtain 4+2*2^D=4^D. The quadratic formula gives 2^D=1+sqrt(5), so Dâ1.694.
Now I intend to get #150 tattooed on my torso. If a 3 x 3 in tat is $100 then by my calculations the 6 x 6 in should be 100*2^D = $323.61 instead of $400. But hey, I don't make the rules!
5 notes
¡
View notes