#Vehicle Comparison
Explore tagged Tumblr posts
Text
#Toyota Fortuner#Ford Endeavour#Mg Gloster#Toyota Fortuner Vs Ford Endeavour#Ford Endeavour Vs Mg Gloster#Fortuner Vs Endeavour#Car review#Car comparison#SUV Comparison#Vehicle Comparison
0 notes
Note
Hi quick question, do you have maybe a rough reference for Sunrazor's height?? Like in comparison to Sides and Sunny maybe. So I could whittle out the difference between her and Comet? If you don't mind I know your busy..
Or maybe even how you decided on their height difference??? I know your making something involving the two of them :D
Funny enough, I was actually about to ask you about that lol
Here’s a height reference thing I started but never finished with some of my other OCs

Please ignore how messy everything is haha
I’d say the average height for bots are somewhere between Leoblast (the second guy to the last) and Valkyrie. She’s like in the middle though.
If I had to guess I’d put Sunstreaker around this tall compared to her. Once again. Using the same logic that says Soundwave can turn into a cassette player and Megatron into a gun, she can be big.
I also just really love myself some big bulky characters that can just throw people around.

I swear she’s freakishly big for a reason. It’s important for the story. Trust.
I hope this kinda helps. I also haven’t completely locked down the sizes. This is just kinda like… a general idea? Basically, it could change but it probably wouldn’t be too dramatic or anything.
#transformer oc#height comparison#you get to see more of my children#she also does transform into a really big truck so#I mean it’s not without reason to put her there#gonna nerd out for a moment here haha#I got the inspiration for Sunrazor after I noticed our lack of truck alt modes in transformers#and as a very proud truck owner myself. I decided I’d help fix that#my truck is a Ram 1500. on the vehicle and truck classification that’s a class 2#that class includes things like cargo vans and minivans#Sunrazor is based of of a truck that would fall into class 3#basically any vehicle that ranges from 10000lbs-14000lbs#the average weight of most cars is like 4000lbs#Lamborghini’s weigh in at like 3700lbs generally speaking… so yeah#she’s big#sorry for the rant#I just really like trucks#also sorry I took so long to reply lol#asks
5 notes
·
View notes
Text
love when you see a content creator you hate come on your feed and then they say something that is immediately so stupid that you close the app.
anyway I just saw that linguistics tiktok guy say “a lot of people are comparing the ban of tiktok to the burning of the library of alexandria”
#like I get sort of where that’s coming from. but don’t do that.#tiktok isn’t an information source it’s a public forum. compare it to other vehicles of free speech.#not a fucking. archive of factual information like a library#tiktok#tiktok ban#idk the nyt did comparisons of the tiktok ban to historical events better than this shit
5 notes
·
View notes
Text
I have been spending and objectively disturbing amount of time on the war thunder forums...
#Like fucking hell man this is embarrassing#need to get some stats and comparisons for vehicle performance somewhere I guess#They're all a bit... enthusiastic about this though
5 notes
·
View notes
Text
Sometimes I think about if the CH reboot took the late 2010s cartoon route of doing episodic shit for the first few seasons and then slowly introducing serialized elements instead of pretending it was still a parody when they clearly took their characters and storylines seriously too much for it to actually be one.
#clone high#clone high reboot#text post#like it starts OFF still being the same parody but slowly introduces plotpoints and characters that come back later#even though in the moment they pretend they aren’t important/are one-offs#so you’re blindsided by the reveals but you can also clearly go back and pinpoint when they were set up#I genuinely think they COULD pull it off if they had a good enough writing staff#uuh but they wanted to write their / everyone fic into the show instead#but I get it would be weird for a cartoon that was primarily a parody of a#genre of show or one specific show to introduce serialization even if slowly while also fleshing out the characters meant to be#used as vehicles for jokes…#…#(this is where the Venture Bros comparison goes)#if the show that started off as what if Scooby Doo but they can swear and the kids are inept#can do it I know you can too Clone High#I KNOW you have capable writers because a bunch of them wrote the old season one which was AMAZING
3 notes
·
View notes
Text
Hunyuan-Large and the MoE Revolution: How AI Models Are Growing Smarter and Faster
New Post has been published on https://thedigitalinsider.com/hunyuan-large-and-the-moe-revolution-how-ai-models-are-growing-smarter-and-faster/
Hunyuan-Large and the MoE Revolution: How AI Models Are Growing Smarter and Faster
Artificial Intelligence (AI) is advancing at an extraordinary pace. What seemed like a futuristic concept just a decade ago is now part of our daily lives. However, the AI we encounter now is only the beginning. The fundamental transformation is yet to be witnessed due to the developments behind the scenes, with massive models capable of tasks once considered exclusive to humans. One of the most notable advancements is Hunyuan-Large, Tencent’s cutting-edge open-source AI model.
Hunyuan-Large is one of the most significant AI models ever developed, with 389 billion parameters. However, its true innovation lies in its use of Mixture of Experts (MoE) architecture. Unlike traditional models, MoE activates only the most relevant experts for a given task, optimizing efficiency and scalability. This approach improves performance and changes how AI models are designed and deployed, enabling faster, more effective systems.
The Capabilities of Hunyuan-Large
Hunyuan-Large is a significant advancement in AI technology. Built using the Transformer architecture, which has already proven successful in a range of Natural Language Processing (NLP) tasks, this model is prominent due to its use of the MoE model. This innovative approach reduces the computational burden by activating only the most relevant experts for each task, enabling the model to tackle complex challenges while optimizing resource usage.
With 389 billion parameters, Hunyuan-Large is one of the most significant AI models available today. It far exceeds earlier models like GPT-3, which has 175 billion parameters. The size of Hunyuan-Large allows it to manage more advanced operations, such as deep reasoning, generating code, and processing long-context data. This ability enables the model to handle multi-step problems and understand complex relationships within large datasets, providing highly accurate results even in challenging scenarios. For example, Hunyuan-Large can generate precise code from natural language descriptions, which earlier models struggled with.
What makes Hunyuan-Large different from other AI models is how it efficiently handles computational resources. The model optimizes memory usage and processing power through innovations like KV Cache Compression and Expert-Specific Learning Rate Scaling. KV Cache Compression speeds up data retrieval from the model’s memory, improving processing times. At the same time, Expert-Specific Learning Rate Scaling ensures that each part of the model learns at the optimal rate, enabling it to maintain high performance across a wide range of tasks.
These innovations give Hunyuan-Large an advantage over leading models, such as GPT-4 and Llama, particularly in tasks requiring deep contextual understanding and reasoning. While models like GPT-4 excel at generating natural language text, Hunyuan-Large’s combination of scalability, efficiency, and specialized processing enables it to handle more complex challenges. It is adequate for tasks that involve understanding and generating detailed information, making it a powerful tool across various applications.
Enhancing AI Efficiency with MoE
More parameters mean more power. However, this approach favors larger models and has a downside: higher costs and longer processing times. The demand for more computational power increased as AI models grew in complexity. This led to increased costs and slower processing speeds, creating a need for a more efficient solution.
This is where the Mixture of Experts (MoE) architecture comes in. MoE represents a transformation in how AI models function, offering a more efficient and scalable approach. Unlike traditional models, where all model parts are active simultaneously, MoE only activates a subset of specialized experts based on the input data. A gating network determines which experts are needed for each task, reducing the computational load while maintaining performance.
The advantages of MoE are improved efficiency and scalability. By activating only the relevant experts, MoE models can handle massive datasets without increasing computational resources for every operation. This results in faster processing, lower energy consumption, and reduced costs. In healthcare and finance, where large-scale data analysis is essential but costly, MoE’s efficiency is a game-changer.
MoE also allows models to scale better as AI systems become more complex. With MoE, the number of experts can grow without a proportional increase in resource requirements. This enables MoE models to handle larger datasets and more complicated tasks while controlling resource usage. As AI is integrated into real-time applications like autonomous vehicles and IoT devices, where speed and low latency are critical, MoE’s efficiency becomes even more valuable.
Hunyuan-Large and the Future of MoE Models
Hunyuan-Large is setting a new standard in AI performance. The model excels in handling complex tasks, such as multi-step reasoning and analyzing long-context data, with better speed and accuracy than previous models like GPT-4. This makes it highly effective for applications that require quick, accurate, and context-aware responses.
Its applications are wide-ranging. In fields like healthcare, Hunyuan-Large is proving valuable in data analysis and AI-driven diagnostics. In NLP, it is helpful for tasks like sentiment analysis and summarization, while in computer vision, it is applied to image recognition and object detection. Its ability to manage large amounts of data and understand context makes it well-suited for these tasks.
Looking forward, MoE models, such as Hunyuan-Large, will play a central role in the future of AI. As models become more complex, the demand for more scalable and efficient architectures increases. MoE enables AI systems to process large datasets without excessive computational resources, making them more efficient than traditional models. This efficiency is essential as cloud-based AI services become more common, allowing organizations to scale their operations without the overhead of resource-intensive models.
There are also emerging trends like edge AI and personalized AI. In edge AI, data is processed locally on devices rather than centralized cloud systems, reducing latency and data transmission costs. MoE models are particularly suitable for this, offering efficient processing in real-time. Also, personalized AI, powered by MoE, could tailor user experiences more effectively, from virtual assistants to recommendation engines.
However, as these models become more powerful, there are challenges to address. The large size and complexity of MoE models still require significant computational resources, which raises concerns about energy consumption and environmental impact. Additionally, making these models fair, transparent, and accountable is essential as AI advances. Addressing these ethical concerns will be necessary to ensure that AI benefits society.
The Bottom Line
AI is evolving quickly, and innovations like Hunyuan-Large and the MoE architecture are leading the way. By improving efficiency and scalability, MoE models are making AI not only more powerful but also more accessible and sustainable.
The need for more intelligent and efficient systems is growing as AI is widely applied in healthcare and autonomous vehicles. Along with this progress comes the responsibility to ensure that AI develops ethically, serving humanity fairly, transparently, and responsibly. Hunyuan-Large is an excellent example of the future of AI—powerful, flexible, and ready to drive change across industries.
#ai#AI efficiency#AI energy efficiency#AI in finance#AI in healthcare#ai model#AI model comparison#AI models#AI scalability#AI systems#AI-powered diagnostics#Analysis#applications#approach#architecture#artificial#Artificial Intelligence#autonomous#autonomous vehicles#billion#cache#change#Cloud#code#complexity#compression#computer#Computer vision#contextual understanding#cutting
0 notes
Text
Porsche Macan EV 4S: Performance Meets Luxury in an Electric SUV
₹1.39 Cr Powertrain and Performance The Macan EV 4S stands out for its cutting-edge electric powertrain: Dual Motor System: The Permanent Magnet Synchronous Motors (PMSM) on the front and rear axles deliver exceptional 430 kW of combined power and an impressive 820 Nm torque, ensuring instantaneous acceleration and smooth power delivery. Performance: With a 0-100 km/h sprint in just 4.1…
#AWD Electric SUV#Electric SUV#Electric SUV Comparison#EV Sports SUV#High-Performance Electric Vehicle#Luxury EV#Macan EV Interior#Macan EV Range#Performance EV#Porsche Adaptive Suspension#Porsche EV Charging#Porsche EV Features#Porsche Macan Electric#Porsche Macan EV 4S#Porsche Macan Review
0 notes
Text
#Car Reviews#Eco-friendly Vehicles#Emission Scores#environmentally friendly#Green Vehicles#India 2024#Low Emissions Cars#price comparison#specifications#Sustainable Cars
0 notes
Text
#Car Reviews#Eco-friendly Vehicles#Emission Scores#environmentally friendly#Green Vehicles#India 2024#Low Emissions Cars#price comparison#specifications#Sustainable Cars
0 notes
Text
Ready to buy a car but torn between bank or dealer financing? Discover the ultimate showdown of pros and cons in our engaging guide! Make the smartest choice and drive away with the best deal. Don’t miss out—read now and secure your dream car effortlessly!
#Car loan options#Auto financing#Loan interest rates#Vehicle financing#Auto loan terms#Bank loan advantages#Dealer financing benefits#Car dealership financing#Personal loans for cars#Auto loan approval process#Credit score impact#Financing incentives#Loan comparison#Financing deals#Auto loan repayment#Down payment options#APR comparison#Loan flexibility#Financing discounts#Purchase vs. lease#Loan pre-approval#Auto finance advice#Negotiating car loans#Financial institutions#Loan application process
0 notes
Text
Comparing Car Insurance Quotes: Find The Best Deal
Navigating the complex world of car insurance can be daunting, but comparing quotes from multiple providers is the key to finding the best deal. This comprehensive guide will walk you through the process of gathering personalized car insurance quotes, evaluating coverage options, and ultimately securing the most affordable policy that meets your unique needs. Whether you’re a first-time buyer or…

View On WordPress
#Affordable car insurance#Auto insurance savings#Best car insurance quotes#Car insurance comparison#Car insurance coverage#Comparing insurance providers#Finding the right coverage#Insurance premium comparison#Online insurance quotes#Vehicle insurance rates
0 notes
Text
BYD Seal Takes on Ireland: Real-World Range Tested
The rise of Chinese electric vehicles (EVs) is undeniable, with brands like BYD challenging established players like Tesla. But how do these Chinese EVs perform in real-world conditions? A recent test by the YouTube channel “Neo EV Review Ireland” sheds light on the capabilities of the BYD Seal, a direct competitor to the Tesla Model 3. BYD Seal vs. Tesla Model 3: Specs on Paper The BYD Seal…
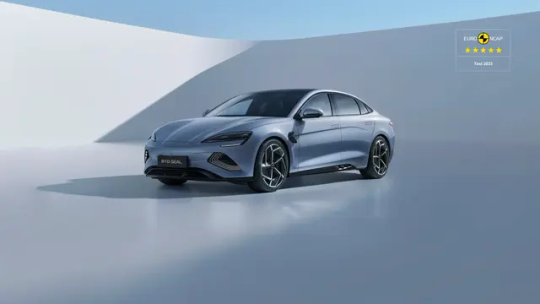
View On WordPress
#BYD Seal#China EV Market#Electric Vehicle Comparison#Electric Vehicles (EV)#Ireland#Real-World Range Test#Tesla Model 3#WLTP Rating
0 notes
Text
2024 Subaru Solterra - TDP Review
The 2024 Subaru Solterra marks Subaru’s ambitious entry into the electric vehicle market. As the brand’s first all-electric car, the Solterra aims to blend Subaru’s well-known ruggedness with the modern demands of electric mobility. Built on the same platform as the Toyota BZ4X and Lexus RZ, the Solterra is designed to offer a unique take on the electric SUV segment. Its debut in Australia comes…
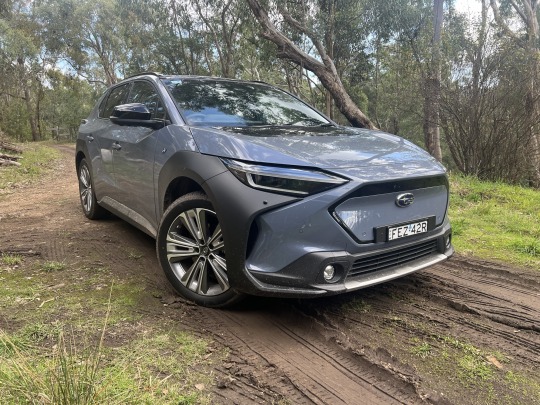
View On WordPress
#2024 Subaru#AC charging#all-wheel drive#Australian market#AWD#battery#Charging#Comfort#competitive comparison#connectivity#DC fast charging#driving experience#dual motors#electric car#electric SUV#electric vehicle#energy efficiency#EV#EV Market#first drive#ground clearance#Hyundai Ioniq 5#Infotainment#interior#Kia EV6#maintenance#off-road#performance#pricing#range
0 notes
Text
Understanding Zero Depreciation in Car Insurance: What You Need to Know

When it comes to car insurance, many vehicle owners are seeking ways to maximize their protection while minimizing out-of-pocket costs during claims. This has led to the rising popularity of “Zero Depreciation” add-ons in car insurance policies, especially in India. In this blog, we’ll explore what Zero Depreciation is, why it’s beneficial, and how it differs from traditional car insurance coverage. We’ll also discuss how to add it to your car insurance policy, the cost implications, and the best times to consider purchasing it.
What is Zero Depreciation in Car Insurance?
Zero Depreciation, also known as “Nil Depreciation” or “Bumper-to-Bumper” coverage, is an add-on to a car insurance policy that ensures the full cost of repairing or replacing car parts without accounting for depreciation. Depreciation is the reduction in value of a car’s components over time due to wear and tear. In a standard car insurance policy, the claim amount for repairs is reduced by the depreciation value of the parts, which means that you would have to pay the difference out-of-pocket.
With Zero Depreciation, the insurance company covers the full cost of repairs or replacements, providing greater financial protection for the insured. This add-on is particularly beneficial for those who own newer cars or high-end vehicles where parts replacement can be costly.
Benefits of Zero Depreciation Add-On
Higher Claim Amount:Â Since depreciation is not deducted from the claim, you receive a higher reimbursement for repairs or replacements, resulting in lower out-of-pocket expenses.
Comprehensive Coverage:Â Zero Depreciation covers all types of parts, including metal, rubber, plastic, and fiber. This comprehensive approach provides greater security for vehicle owners.
Better for High-End Cars:Â If you have a premium or luxury car, Zero Depreciation is particularly useful, as the cost of replacing parts can be substantial.
Reduced Stress During Claims: With Zero Depreciation, you won’t have to worry about complex depreciation calculations. This simplifies the claims process, allowing you to focus on getting your car repaired.
Difference Between Zero Depreciation and Third-Party Car Insurance
It’s essential to understand the distinction between Zero Depreciation and third-party car insurance. Third-party car insurance is mandatory in India and covers liabilities to others in case of an accident. It does not cover damage to your own vehicle. On the other hand, Zero Depreciation is an add-on to a comprehensive car insurance policy, providing additional coverage for your own car without considering depreciation.
Cost Implications of Zero Depreciation
Zero Depreciation comes at an additional cost, typically increasing the premium by 10–15%. However, the actual cost depends on various factors, including the car’s make and model, age, driving history, and location. While it may seem expensive, the benefits often outweigh the additional premium, especially if you have a newer car or are prone to accidents.
When to Consider Zero Depreciation
Zero Depreciation might not be necessary for everyone. Here are some scenarios when it would be beneficial:
New Car Owners:Â If your car is new (usually less than five years old), Zero Depreciation provides better coverage.
Luxury Car Owners:Â If you own a high-end or premium car, the cost of parts replacement can be high, making Zero Depreciation a smart choice.
Frequent Drivers:Â If you drive frequently or in high-traffic areas, the risk of accidents is greater, and Zero Depreciation can provide additional security.
Those Seeking Comprehensive Protection:Â If you want comprehensive protection without worrying about depreciation, this add-on is ideal.
How to Get Zero Depreciation in Your Car Insurance Policy
Adding Zero Depreciation to your car insurance policy is simple. When purchasing or renewing your car insurance online, look for the option to add Zero Depreciation to your comprehensive policy. Compare different insurers to find the best rates and coverage options. Consider reading customer reviews and checking the claim settlement ratio to ensure a smooth experience.
Conclusion
Zero Depreciation is a valuable add-on for car insurance policies, providing comprehensive coverage by eliminating the impact of depreciation on claim amounts. It offers greater financial protection and peace of mind, especially for newer and premium car owners. While it comes with an additional cost, the benefits can outweigh the expense in many cases. Whether you’re renewing your policy or buying a new one, consider the value of Zero Depreciation in ensuring complete protection for your vehicle.
#insurance#car insurance policy#car insurance comparison#car insurance online#car insurance#vehicle insurance#car insurance company#insurance company
0 notes
Text
You know who I do feel bad for in all this?
The person who turned Luigi in. I don't agree with what they did, but I get it. If they are working for McDonald's, they are probably at minimum wage. Full time (which they probably weren't, cause fuck McDonalds probably doesnt want to have to give benefits)yearly pay for min wage is $15,000. The rewards together were $60,000.
Can you imagine how much that would change their life? FOUR TIMES their yearly wage. I am so incredibly privileged in comparison, but four times my yearly wage would change so many things for me.
You think about your family, and how much that could help. You think about your living situation. You think about rent and food prices and a vehicle. All of that would be so much easier, even for a little bit of time.
And then to find out that you probably won't get the money on technicalities. And if you do, you definitely won't get all of it. And even then, it will take at least a year.
Being told that you would get something that would change your life, the life of your children, and it's bullshit. Yet another reason you can't trust the cops.
And it also shows another way that authorities are so fucking stupid about this whole thing. Any trust people had in them is being blown out of the water. It is so incredibly clear the difference in ways people are treated. Children are being killed in school and nothing, but one CEO gets shot and it's considered terrorism. A CEO who profited off the deaths of thousands. But that person is more important than all us regular people. And now this reward BS. Do they actually think ANYONE is gonna help them again after seeing how they are screwing this person over
And eric fucking adams being in that fucking perp walk. That bastard has spent the past several months talking "innocent until proven guilty" and "due process"...but only when it comes to committing his own crimes. Not someone else's where he can pretend that he is some sort of hero.
It is amazing how people who are in power and people who think thay they can gain power by protecting the rich are so out of touch.
5K notes
·
View notes
Text
AI Language Showdown: Comparing the Performance of C++, Python, Java, and Rust
New Post has been published on https://thedigitalinsider.com/ai-language-showdown-comparing-the-performance-of-c-python-java-and-rust/
AI Language Showdown: Comparing the Performance of C++, Python, Java, and Rust
The choice of programming language in Artificial Intelligence (AI) development plays a vital role in determining the efficiency and success of a project. C++, Python, Java, and Rust each have distinct strengths and characteristics that can significantly influence the outcome. These languages impact everything from the performance and scalability of AI systems to the speed at which solutions can be developed and deployed.
As AI continues to advance and succeed across various industries, be it healthcare, finance, autonomous vehicles, or creative fields like art and music, understanding the nuances of these programming languages becomes increasingly important. The correct language can enhance an AI project’s ability to handle complex tasks, optimize processes, and create innovative solutions. In fact, the choice of programming language is not just a technical decision but a strategic one because it significantly impacts the future of AI-driven advancements.
Brief History and Evolution of Each Language
The history and evolution of each of the four languages is briefly presented below:
C++
Bjarne Stroustrup developed C++ in the early 1980s to enhance the C programming language. By combining C’s efficiency and performance with object-oriented features, C++ quickly became a fundamental tool in system software, game development, and other high-performance applications.
In AI, C++ is highly valued for its ability to efficiently manage low-level operations and handle memory. These qualities are significant in areas that require real-time processing, such as robotics and autonomous systems. Although complex, the language’s support for manual memory management enables precise performance optimization, especially in tasks where every millisecond matters. With its speed and low-level control, C++ is an excellent choice for AI applications that demand high computational power and real-time responsiveness.
Python
Guido van Rossum developed Python in the late 1980s, emphasizing simplicity and readability. Its clear syntax and dynamic typing have made it a preferred choice among developers, particularly in AI and data science. Python’s rise in AI is mainly attributable to its rich ecosystem of libraries, such as TensorFlow, PyTorch, and Scikit-learn, which have become essential tools in machine learning and deep learning.
Python’s framework is built to simplify AI development, making it accessible to both beginners and experts. Its flexibility and a large and active community promote continuous innovation and broad adoption in AI research. Python’s simplicity and powerful libraries have made it the leading language for developing AI models and algorithms.
Java
Java, developed by James Gosling and released by Sun Microsystems in 1995, is a high-level, object-oriented language that has gained recognition for its platform independence. Java’s “write once, run anywhere” principle has made it popular for building large-scale, cross-platform applications.
Java is particularly well-suited for enterprise-level AI solutions, where integration with big data technologies like Hadoop and Spark is often required. Its robust performance, scalability, and strong ecosystem make Java an excellent choice for AI applications that need to handle significant volumes of data and integrate with existing enterprise systems. Java’s capacity to effectively manage complex, large-scale projects has made it a reliable option for developing AI solutions that prioritize scalability and integration.
Rust
Rust is a systems programming language developed by Mozilla Research and first released in 2010. It was designed with a strong focus on memory safety and performance, using a unique ownership model to manage memory without relying on garbage collection. Rust’s emphasis on safety and concurrency has gained attention in the AI community, especially for applications that require parallel processing and real-time performance.
Although Rust is relatively new compared to C++, Python, and Java, it quickly gained attention in AI development. Its ability to deliver high performance while avoiding common programming errors, such as memory leaks and data races, makes it an attractive choice for AI applications where safety and efficiency are crucial. As its framework continues to grow, Rust is being increasingly adopted for AI tasks, particularly in edge computing and the Internet of Things (IoT), where performance and reliability are essential.
Performance Comparison
Performance comparison is done based on execution speed, memory management, parallelism and concurrency.
Execution Speed
Execution speed is critical in AI, particularly in applications requiring real-time processing or handling large datasets.
C++ leads in execution speed due to its low-level operations and minimal runtime overhead. Rust, emphasizing performance and safety, offers comparable speed while ensuring memory safety.
Java, though slightly slower than C++ and Rust due to JVM overhead, still performs well in enterprise environments where speed is balanced with scalability.
Despite its slower execution speed, Python remains popular due to its extensive library support and ease of development. However, for performance-critical applications, Python often relies on libraries like NumPy and TensorFlow, which are implemented in C or C++ to boost performance.
Memory Management
Memory management is another critical aspect of AI, especially for large-scale applications that process vast amounts of data.
C++ provides manual memory management, offering developers fine-grained control over resource allocation, essential in optimizing performance. However, this control can lead to memory leaks and other errors if not managed carefully. Rust addresses these issues with its ownership model, which ensures memory safety while maintaining performance.
Java uses automatic garbage collection, simplifying memory management but potentially introducing latency during garbage collection cycles. Python’s garbage collection is also automatic, which, while convenient, can lead to performance bottlenecks in memory-intensive applications.
Parallelism and Concurrency
Parallelism and concurrency are increasingly crucial in AI due to the need to process large datasets and perform complex computations simultaneously.
Rust’s approach to concurrency, which emphasizes safety, sets it apart from C++ and Java, where concurrency can lead to data races and other issues if not handled carefully.
C++ offers powerful parallelism tools but requires careful management to avoid concurrency-related bugs. Java provides a robust threading model, making it suitable for enterprise AI applications that require reliable concurrency.
While capable of parallelism, Python is limited by the Global Interpreter Lock (GIL), which can hinder proper parallel execution in multi-threaded applications. However, Python can exhibit parallelism through multiprocessing and external libraries like Dask.
Performance Aspect C++ Python Java Rust      Execution Speed Fast, low-level operations, minimal runtime overhead Slower often relies on C/C++ libraries for speed Moderate JVM overhead can introduce latency Comparable to C++, emphasis on performance Memory Management Manual control can optimize for performance Automatic garbage collection can lead to bottlenecks Automatic garbage collection introduces latency The ownership model ensures safety, no garbage collection Parallelism & Concurrency Powerful tools require careful management Limited by GIL, can use multiprocessing Robust threading model, suitable for enterprise Safe concurrent programming, emphasis on safety
Ease of Development and Productivity
This comparison is done based on the parameters, such as learning curve, library and framework support, and development speed.
Learning Curve
The learning curve for each language varies significantly, impacting developer productivity and project timelines.
Python is widely regarded as the most accessible language, particularly for beginners and developers transitioning from other languages. Its straightforward syntax and extensive documentation make it an ideal starting point for AI development.
With its clear structure and strong typing, Java offers a moderate learning curve, particularly for developers with experience in object-oriented programming. C++ presents a steeper learning curve due to its complexity and manual memory management, requiring a deeper understanding of low-level operations.
While offering safety and performance benefits, Rust has a steep learning curve due to its unique ownership model and strict compiler rules, which can be challenging for developers accustomed to other languages.
Library and Framework Support
Library and framework support is critical in AI development, as it directly impacts the ease of implementing complex algorithms and models.
Python excels in this aspect, with a vast ecosystem of libraries and frameworks specifically designed for AI and machine learning. TensorFlow, PyTorch, Scikit-learn, and Keras are just a few examples of the powerful tools available to Python developers. Java also offers a robust ecosystem, particularly for enterprise AI solutions, with libraries like Weka, Deeplearning4j, and Apache Mahout.
C++ has fewer AI-specific libraries but benefits from its performance. It can also use libraries like Caffe and TensorFlow for high-performance AI tasks. Rust, a newer language, has a growing but still limited selection of AI libraries, with efforts like the Rust Machine Learning library (rust-ml) community working to expand its capabilities.
Development Speed
Development speed is often a trade-off between ease of use and performance.
Python leads in development speed due to its simplicity, readability, and extensive library support. This allows developers to quickly prototype and iterate on AI models. Java, while more verbose than Python, offers robust tools and frameworks that streamline development for large-scale AI applications, making it suitable for enterprise environments.
On the other hand, C++, with its complexity and manual memory management, C++ requires more time and effort to develop AI applications but offers unparalleled performance in return. Despite its steep learning curve, Rust promotes efficient and safe code, which can lead to faster development once developers are familiar with the language. However, Rust’s relative lack of AI-specific libraries can slow down development compared to Python.
Ecosystem and Community Support
Open-source contributions and industry adoption are among the factors that help assess the ecosystem in general of a programming language.
Open-Source Contributions
The strength of a programming language’s ecosystem and community support is often reflected in the number of active open-source projects and repositories available for AI development. Python dominates this space, with many AI-related open-source projects and an active community contributing to the continuous improvement of libraries like TensorFlow, PyTorch, and Scikit-learn.
Java also benefits from a robust open-source community, with projects like Weka, Deeplearning4j, and Apache Mahout offering robust tools for AI development. C++ has a more specialized community focused on high-performance computing and AI applications requiring real-time processing, with projects like Caffe and TensorFlow. Rust’s community is rapidly growing and concentrates on safe AI development, but it is still in the early stages compared to the more established languages.
Industry Adoption
Industry adoption is a critical factor in determining the relevance and longevity of a programming language in AI development. Python’s widespread adoption in AI research and industry makes it a popular language for most AI projects, from startups to tech giants like Google and Facebook.
On the other hand, with its substantial presence in enterprise environments, Java is commonly used for AI solutions that require integration with existing systems and large-scale data processing. C++ is a preferred choice for AI applications in industries that require high performance, such as autonomous vehicles, robotics, and gaming. Rust, while newer and less widely adopted, is gaining attention in industries prioritizing memory safety and concurrency, such as systems programming and IoT.
Real-World Use Cases
Below, some real-world applications of each of these programming languages are briefly presented:
C++ in AI: Autonomous Vehicles and Robotics
C++ is widely used in the development of AI for autonomous vehicles and robotics, where real-time processing and high performance are critical. Companies like Tesla and NVIDIA employ C++ to develop AI algorithms that enable self-driving cars to process sensor data, make real-time decisions, and navigate complex environments. Robotics applications also benefit from C++’s ability to handle low-level hardware operations, ensuring precise control and fast response times in object recognition and manipulation tasks.
Python in AI: Deep Learning and Research
Due to its rich libraries and frameworks, Python has become synonymous with AI research and deep learning. Google’s TensorFlow and Facebook’s PyTorch, written in Python, are among the most widely used tools for developing deep learning models. Python’s simplicity and ease of use make it the preferred language for researchers and data scientists, enabling rapid prototyping and experimentation with complex neural networks.
Java in AI: Enterprise AI Solutions
Java’s platform independence and scalability make it ideal for enterprise AI solutions that require integration with existing systems and large-scale data processing. Companies like IBM and Oracle use Java to develop AI applications on diverse platforms, from on-premises servers to cloud-based infrastructures.
Rust in AI: Edge Computing and IoT AI Applications
Rust’s emphasis on safety and concurrency makes it suitable for AI applications in edge computing and the Internet of Things (IoT). Companies like Microsoft are exploring Rust to develop AI algorithms that run on resource-constrained devices, where memory safety and performance are critical. Rust’s ability to handle concurrent tasks safely and efficiently makes it ideal for IoT applications that require real-time data processing and decision-making at the edge, reducing latency and improving responsiveness in AI-driven systems.
The Bottom Line
In conclusion, choosing the right programming language for AI development is essential and can greatly influence a project’s performance, scalability, and overall success. Each of the four languages discussed has distinct advantages, making them suitable for different aspects of AI work.
Recommendations Based on Different AI Project Needs
Best Language for High-Performance AI: C++ remains the top choice for AI applications that demand high computational power and real-time processing, such as robotics and autonomous systems.
Best Language for Rapid Development: Python’s ease of use and rich ecosystem make it the best language for rapid development and experimentation in AI, particularly in research and deep learning.
Best Language for Enterprise AI: Java’s scalability and robust ecosystem make it ideal for enterprise AI solutions that require integration with existing systems and large-scale data processing.
Best Language for Future-Proofing AI Projects: Rust’s focus on safety and concurrency makes it the best language for future-proofing AI projects, particularly in critical areas of memory safety and performance.
#1980s#ai#AI development#AI models#AI research#AI systems#Algorithms#amp#Apache#applications#approach#Art#artificial#Artificial Intelligence#attention#autonomous#autonomous systems#autonomous vehicles#Big Data#bugs#Building#C#Cars#Cloud#code#Community#Companies#comparison#complexity#computing
0 notes