#APLS
Explore tagged Tumblr posts
Note
ooooh stark men reacting to you biting HARD on that junction between their neck and shoulder HELLO.
ARF ARF oh my god BARK you awakened her ROOF down girl
NO BUT A EH RFSGA ARG. robb would literally moan, biting you back equally as hard (harder, if you so wish) (you do) (he does) — and you both would be covered in marks from the other by the time you’re finished. he almost likes marking you as much as he likes you marking him 🙂↕️ (freak) (affectionate)
biting him (especially that hard) (he grunts) would help lateseasons!jon flip that switch of man -> wolf in bed, as it not only works him up completely, but it lets him know how much you want this. he’s too much of a sweetheart to be mean without being absolutely sure that’s what you want too (bless him)
AND CREGAN. i just have this vivid image of his brows furrowing + nose scrunching + teeth baring from the (slight) pain of it…. a shaky exhale when it mixes with pleasure omg omgomg. pushing you down into the mattress harder i can’t REMINDING YOU OF HIS STRENGTH OH SEDATE ME
#dippys asks#IM GONNA FLIP MY LID#ARF ATF ARF#APLS#BAKR ANDL BALR#ROOF ROOF#WOOF#GROWLWLLEL#game of thrones#house of the dragon#cregan stark#jon snow#robb stark#cregan stark x reader#robb stark x reader#jon snow x reader#the three musketeers !
333 notes
·
View notes
Text
btw the aphobia on this site is very much still alive. in so many of our tags, you'll find people mocking our terms and our very queerness and identies.
aros and aces and apls are here and queer. aspecs, i love you <3. i love our terms and concepts. they're beautiful. we're beautiful.
190 notes
·
View notes
Text
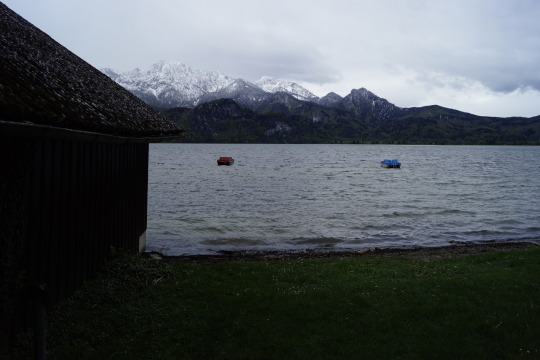
Lake Kochel and mt. Herzogstand on a cold spring day with no tourists around.
5 notes
·
View notes
Text
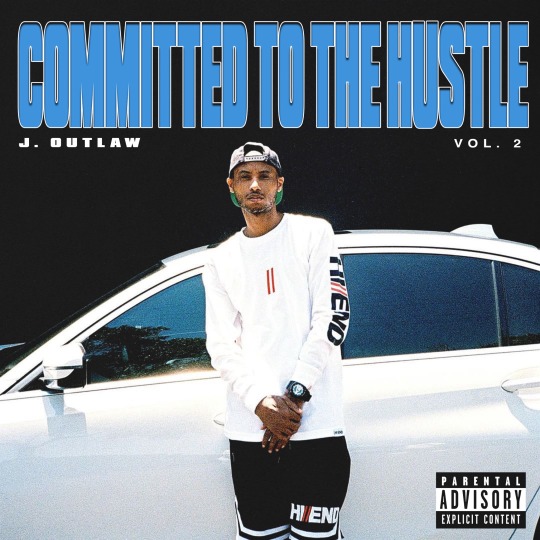
New Project OTW 9/28/87
🎥Teaser For First Visual Release off the project
#havinthangs#mtmg#rte#Roundtripentertainment#kid Gotti#j outlaw#ShawGang#hiphop#musicians#apls#outlaws for life
2 notes
·
View notes
Text
They keep adding aspec identities and the screaming gets progressively longer
A podcast run by an asexual, an aromantic, and an aplatonic called "AAA" and every time an episode starts, one of them welcomes the audience by screaming into the mic
"hello and welcome to AAA!"
5K notes
·
View notes
Text
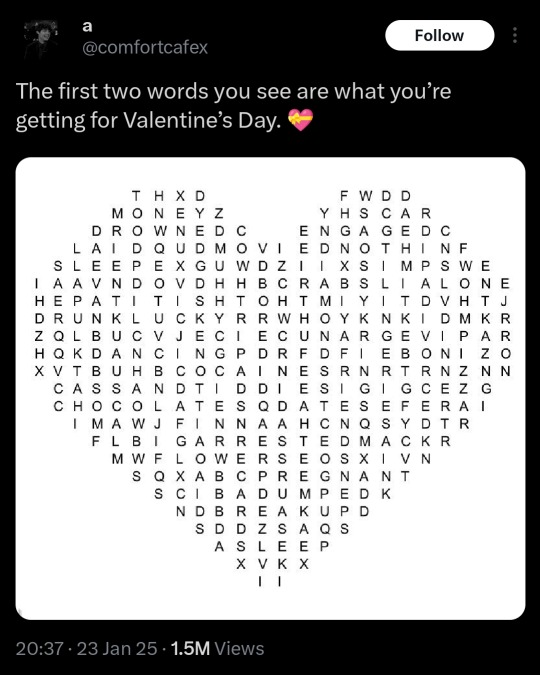
Guess what I saw:
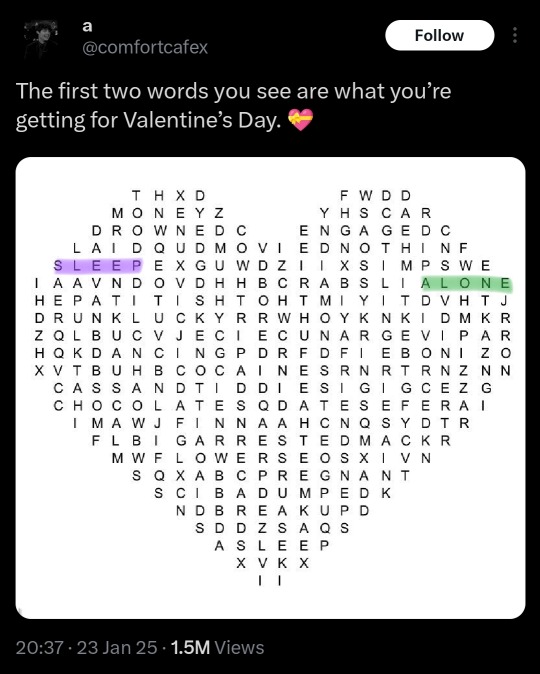
SLEEP, ALONE
SLEEP.
ALONE.
SLEEP ALONE 🗣️🗣️🔥🔥‼️‼️
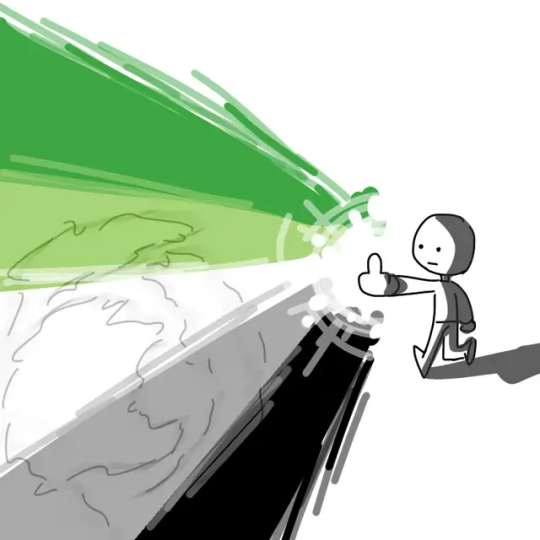
#aromantic#aro#aromantism#arospec#asexual#ace#asexuality#acespec#aroace#aplatonic#apl#aspec#saw this on twitter lmaooo#bro i hope this at least gets some reach ☠️#lemme know if i should add more tags#anyways fuck valentines day#also did you notice that hepatitis is one of the words ☠️#the unlucky people who saw “dies”:#[kal] posts
6K notes
·
View notes
Text
Optimizing LLM Cascades with Prompt Design
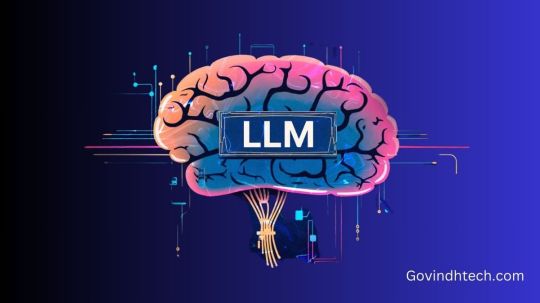
LLM Cascades
The following business outcomes must be achieved by these strategies.
Delivering high-quality answers to a greater number of users initially.
Higher levels of user support should be offered while protecting data privacy.
Enhancing cost and operational effectiveness through timely economization.
Eduardo outlined three methods that developers could use:
Different prompt techniques are used in prompt engineering to produce answers of a higher caliber.
By adding more context to the prompt, retrieval-augmented generation makes it better and less taxing on end users.
The GenAI pipeline moves data more efficiently when prompt economization techniques are used.
Quickly improving the quality of the results while cutting down on the number of model inferencing and associated costs is possible with effective prompting.
Quick engineering: Enhancing model output
Let’s begin by discussing the Learning Based, Creative Prompting, and Hybrid prompt engineering framework for LLM Cascade.
The one shot and few shot prompts are included in the learning-based technique. This method provides the model with context and teaches it through the use of examples in the learning prompt. A zero-shot prompt is one that queries the model using data from its prior training. Context is provided by a one-shot or few-shot prompting, which teaches the model new information so that the LLM produces more accurate results.
More accurate responses can be obtained by using strategies like iterative prompting or negative prompting, which fall under the Creative Prompting category. While iterative prompting offers follow-up prompts that enable the model to learn over the course of the series of prompts, negative prompting sets a boundary for the model’s response.
The above methods can be combined, or not, in the Hybrid Prompting approach.
These approaches have benefits, but there is a catch: in order to generate high-quality prompts, users must possess the necessary knowledge to apply these strategies and provide the necessary context.
LLM 7B
Typically, LLM Cascade are trained using a broad corpus of internet data rather than data unique to your company. Results from the LLM workflow prompt that incorporate enterprise data with retrieval augmented generation (RAG) will be more pertinent. In order to retrieve the prompt context, this workflow entails embedding enterprise data into a vector database. The prompt and the retrieved context are subsequently sent to the LLM, which produces the response. Your data stays private and you avoid paying extra for compute training since RAG allows you to use your data in the LLM without retraining the model.
Early economization: Saving costs and delivering value
The final technique focuses on various prompt strategies to reduce the amount of inferencing needed for the model.
Token summarization lowers costs for APIs that charge by the token by using local models to reduce the number of tokens per user prompt sent to the LLM service.
Answers to frequently asked questions are cached by completion caching, saving inference resources from having to be generated each time the question is posed.
Query concatenation reduces overhead that accumulates per-query, such as pipeline overhead and prefill processing, by combining multiple queries into a single LLM submission.
LLM cascades are designed to execute queries on more basic LLMs initially, rating them according to quality, and only moving on to more expensive, larger models when necessary. By using this method, the average compute requirements per query are decreased.
7B LLM Model
Ultimately, the amount of compute memory and power determines the model throughput. However, accuracy and efficiency are just as important in influencing the outcomes of generative AI as throughput. The above strategies can be combined to create an LLM Cascade prompt architecture that is specific to your company’s requirements.
Although large language models (LLMs) are immensely potent instruments, they can be optimized to function more effectively just like any other tool. Prompt engineering can help in this situation.
Prompt engineering
The skill of crafting input for an LLM Cascade to produce the most accurate and desired result is known as prompt engineering. It basically provides the LLM Cascade with precise instructions and background information for the current task. Prompts with thoughtful design can greatly enhance the following:
Accuracy
A well-crafted prompt can guide the LLM Cascade away from unrelated data and toward the information that will be most helpful for the given task.
Efficiency
The LLM Cascade can determine the solution more quickly and with less computation time and energy if it is given the appropriate context.
Specificity
By giving precise instructions, you can make sure the LLM produces outputs that are suited to your requirements, saving you time from having to sort through pointless data.
Prompt Engineering Technique Examples
Here are two intriguing methods that use prompts to enhance LLM performance
Retrieval-Augmented Generation
This method augments the prompt itself with pertinent background knowledge or data. This can be especially useful for assignments that call for the LLM to retrieve and process outside data.
Emotional Persuasion Prompting
Research indicates that employing persuasive prompts and emotive language can enhance LLM concentration and performance on creative or problem-solving tasks.
You can greatly improve the efficacy and efficiency of LLMs for a variety of applications by combining these strategies and experimenting with various prompt structures.
Read more on govindhtech.com
0 notes
Text
not having sex is morally neutral. having sex is morally neutral
not engaging in romance is morally neutral. engaging in romance is morally neutral
not having friends is morally neutral. having friends is morally neutral
5K notes
·
View notes
Text
people who exclude straight trans people and straight aspec people are my worst enemies. btw
#trans#ace#aro#apl#transgender#asexual#aromantic#aplatonic#im not straight but guys do you not realise how important they are#I love you straight trans people I love you straight aspec people
11K notes
·
View notes
Text
11K notes
·
View notes
Text
people who deserve more respect and recognition:
intersex people
trans men
aromantic people
multigender people
people in qpr's
aplatonic people
#queer#lgbtq#lgbtqia#inclusivity#equality#intersex#trans men#ftm#aromantic#aro#arospec#multigender#qpr#aplatonic#apl#aplspec
2K notes
·
View notes
Text
Imagine
#tumblr please this would be so cool#abrosexual#achillean#agender#alloace#aplatonic#apl#aroace#aroallo#bigender#demiboy#demigender#demigirl#enbian#genderfluid#genderflux#genderqueer#maverique#neutrois#omnisexual#pangender#polysexual#pomosexual#sapphic#toric#trixic#xenogender
7K notes
·
View notes
Text
sure "romantic" isn't the only type of love but also "love" isn't the only type of positive feeling. So maybe stop insisting everyone needs love to be happy and accept that loveless ppl exist? Pretty please?
#man february arrived and im on a roll with these posts#thought to myself 'tumblr could use more loveless-posting'#decided if no one's gonna do it im just gonna do it myself#also this isn't directed at anyone specific im just being salty at random#loveless#loveless aromantic#loveless aro#aromantic#aro#arospec#loveless apl#loveless aplatonic#aplatonic#aplspec#lgbtq#queer stuff
6K notes
·
View notes
Text
The answer to aspecs asking you to stop assuming [thing] about all aspecs is not to start assuming [opposite of thing] about all aspecs btw.
"Stop assuming all AlloAros have a lot of sex (or "are sluts")" does not mean "Start assuming no AlloAro has lots of sex" and also not "No AlloAro ever feels comfortable calling themselves a slut (or whore or w/e)" and vice-versa.
"Stop assuming all aros are loveless and non-partnering" does not mean "start assuming all aros do love ("in non-romantic ways") and are always partnering" and vice-versa.
"Stop assuming all aces are sex-repulsed" does not mean "start assuming all aces are sex-favourable", and vice-versa.
"Stop assuming all aplatonic people want to make friends" does not mean "start assuming no aplatonic people want to make friends" and vice-versa.
"Stop assuming all [aros or aces, mostly*] experience no [romantic or sexual, mostly*] attraction" does not mean "start assuming all [aros or aces] experience some form of [romantic or sexual attraction]" and vice-versa.
[Continue ad infinitum; these are just some examples and listing all things like that would be impossible.]
Just stop making assumptions about people based on one part of their identity. If they decide they want you to know, they'll tell you. If you want to know, you can ask, and maybe they'll give an answer (don't act like you're owed one, tho).
Accept that all people are different and even people under the same queer identity are going to have a vastly different experience; especially vast umbrellas like the aspec-identities. Instead of taking what one aspec person says about their identity as true for everyone under that same identity and then taking everything else as a "contradiction" to that label, or as something that needs another or a different label, simply accept that different people are going to have a different experience even if they use the same words to describe them.
It's really not that hard.
[*I think this may also apply to other aspec-identities (aplatonic, afamilial, atertriary, etc), right? I see these takes mostly inside of and directed at aro- and ace-spaces; but it also seems like it just applies across the board, non-aro and non-ace aspec-identities are just lesser known and thus not discussed as often.]
#aspec#aspec community#aromantic#aro#asexual#ace#aplatonic#apl#alloaro#aroallo#loveless#loveless aro
1K notes
·
View notes
Text
Cishet aspecs are queer.
Cishet aromantics are queer. Cishet asexuals are queer. Cishet aplatonics are queer. Cishet afamilials are queer. Cishet anattractionals are queer.
Aspecs are queer as hell and excluding them only isolates queer people from their community.
#aspec pride#aspec positivity#aspec mafia#aspec#loveless#loveless pride#aromantic#asexual#aplatonic#afamilial#a aesthetic#aemotional#asensual#aqueerplatonic#loveless aplatonic#loveless aro#loveless apl#loveless aromantic#arospec#acespec#aro#ace#anattractional
2K notes
·
View notes