#AI data governance
Explore tagged Tumblr posts
Text
Monetizing Research for AI Training: The Risks and Best Practices
New Post has been published on https://thedigitalinsider.com/monetizing-research-for-ai-training-the-risks-and-best-practices/
Monetizing Research for AI Training: The Risks and Best Practices


As the demand for generative AI grows, so does the hunger for high-quality data to train these systems. Scholarly publishers have started to monetize their research content to provide training data for large language models (LLMs). While this development is creating a new revenue stream for publishers and empowering generative AI for scientific discoveries, it raises critical questions about the integrity and reliability of the research used. This raises a crucial question: Are the datasets being sold trustworthy, and what implications does this practice have for the scientific community and generative AI models?
The Rise of Monetized Research Deals
Major academic publishers, including Wiley, Taylor & Francis, and others, have reported substantial revenues from licensing their content to tech companies developing generative AI models. For instance, Wiley revealed over $40 million in earnings from such deals this year alone. These agreements enable AI companies to access diverse and expansive scientific datasets, presumably improving the quality of their AI tools.
The pitch from publishers is straightforward: licensing ensures better AI models, benefitting society while rewarding authors with royalties. This business model benefits both tech companies and publishers. However, the increasing trend to monetize scientific knowledge has risks, mainly when questionable research infiltrates these AI training datasets.
The Shadow of Bogus Research
The scholarly community is no stranger to issues of fraudulent research. Studies suggest many published findings are flawed, biased, or just unreliable. A 2020 survey found that nearly half of researchers reported issues like selective data reporting or poorly designed field studies. In 2023, more than 10,000 papers were retracted due to falsified or unreliable results, a number that continues to climb annually. Experts believe this figure represents the tip of an iceberg, with countless dubious studies circulating in scientific databases.
The crisis has primarily been driven by “paper mills,” shadow organizations that produce fabricated studies, often in response to academic pressures in regions like China, India, and Eastern Europe. It’s estimated that around 2% of journal submissions globally come from paper mills. These sham papers can resemble legitimate research but are riddled with fictitious data and baseless conclusions. Disturbingly, such papers slip through peer review and end up in respected journals, compromising the reliability of scientific insights. For instance, during the COVID-19 pandemic, flawed studies on ivermectin falsely suggested its efficacy as a treatment, sowing confusion and delaying effective public health responses. This example highlights the potential harm of disseminating unreliable research, where flawed results can have a significant impact.
Consequences for AI Training and Trust
The implications are profound when LLMs train on databases containing fraudulent or low-quality research. AI models use patterns and relationships within their training data to generate outputs. If the input data is corrupted, the outputs may perpetuate inaccuracies or even amplify them. This risk is particularly high in fields like medicine, where incorrect AI-generated insights could have life-threatening consequences. Moreover, the issue threatens the public’s trust in academia and AI. As publishers continue to make agreements, they must address concerns about the quality of the data being sold. Failure to do so could harm the reputation of the scientific community and undermine AI’s potential societal benefits.
Ensuring Trustworthy Data for AI
Reducing the risks of flawed research disrupting AI training requires a joint effort from publishers, AI companies, developers, researchers and the broader community. Publishers must improve their peer-review process to catch unreliable studies before they make it into training datasets. Offering better rewards for reviewers and setting higher standards can help. An open review process is critical here. It brings more transparency and accountability, helping to build trust in the research. AI companies must be more careful about who they work with when sourcing research for AI training. Choosing publishers and journals with a strong reputation for high-quality, well-reviewed research is key. In this context, it is worth looking closely at a publisher’s track record—like how often they retract papers or how open they are about their review process. Being selective improves the data’s reliability and builds trust across the AI and research communities.
AI developers need to take responsibility for the data they use. This means working with experts, carefully checking research, and comparing results from multiple studies. AI tools themselves can also be designed to identify suspicious data and reduce the risks of questionable research spreading further.
Transparency is also an essential factor. Publishers and AI companies should openly share details about how research is used and where royalties go. Tools like the Generative AI Licensing Agreement Tracker show promise but need broader adoption. Researchers should also have a say in how their work is used. Opt-in policies, like those from Cambridge University Press, offer authors control over their contributions. This builds trust, ensures fairness, and makes authors actively participate in this process.
Moreover, open access to high-quality research should be encouraged to ensure inclusivity and fairness in AI development. Governments, non-profits, and industry players can fund open-access initiatives, reducing reliance on commercial publishers for critical training datasets. On top of that, the AI industry needs clear rules for sourcing data ethically. By focusing on reliable, well-reviewed research, we can build better AI tools, protect scientific integrity, and maintain the public’s trust in science and technology.
The Bottom Line
Monetizing research for AI training presents both opportunities and challenges. While licensing academic content allows for the development of more powerful AI models, it also raises concerns about the integrity and reliability of the data used. Flawed research, including that from “paper mills,” can corrupt AI training datasets, leading to inaccuracies that may undermine public trust and the potential benefits of AI. To ensure AI models are built on trustworthy data, publishers, AI companies, and developers must work together to improve peer review processes, increase transparency, and prioritize high-quality, well-vetted research. By doing so, we can safeguard the future of AI and uphold the integrity of the scientific community.
#000#2023#academia#Academic publishers and AI#adoption#agreement#ai#AI data governance#AI data integrity#AI data quality#AI development#AI industry#AI model reliability#AI models#ai tools#ai training#AI training data#amp#Artificial Intelligence#Business#business model#China#Community#Companies#content#covid#data#data transparency#databases#datasets
0 notes
Text
Latest AI Regulatory Developments:
As artificial intelligence (AI) continues to transform industries, governments worldwide are responding with evolving regulatory frameworks. These regulatory advancements are shaping how businesses integrate and leverage AI technologies. Understanding these changes and preparing for them is crucial to remain compliant and competitive. Recent Developments in AI Regulation: United Kingdom: The…
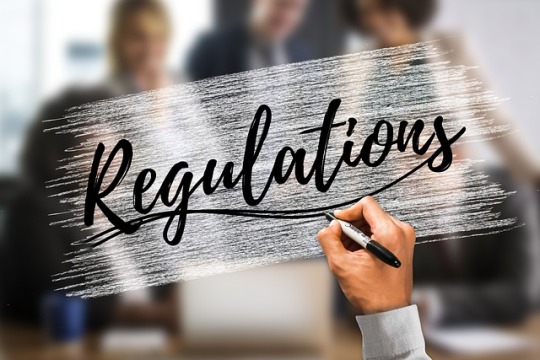
View On WordPress
#AI#AI compliance#AI data governance#AI democratic values#AI enforcement#AI ethics#AI for humanity#AI global norms#AI human rights#AI industry standards#AI innovation#AI legislation#AI penalties#AI principles#AI regulation#AI regulatory framework#AI risk classes#AI risk management#AI safety#AI Safety Summit 2023#AI sector-specific guidance#AI transparency requirements#artificial intelligence#artificial intelligence developments#Bletchley Declaration#ChatGPT#China generative AI regulation#Department for Science Innovation and Technology#EU Artificial Intelligence Act#G7 Hiroshima AI Process
1 note
·
View note
Text
"wrapped look bad. wrapped is AI" oh my god dont you get it. AI is not one thing. it's not some computer guy they dreamed up. it's not one algorithm. everything is AI. nothing is AI. it's all computer generated content. all of it. it is this year. it was last year & the year before that.
#treating AI like a boogeyman will not stop the energy sucking data centers#It will not stop government funded misinformation campaigns#learn how to use it TODAY#learn how to use critica thinking TODAY#obligatory I don't support AI generated art statement
10 notes
·
View notes
Text


I don’t plan on using any ai services, but if you need MORE reason not to….
#aligned with t***p 🤢🤢#Funny how the government is suddenly worried when another country wants our data but if it’s US based it’s fine….#anti ai#anti generative ai#us politics
5 notes
·
View notes
Text
My oh my. From ABC News Australia: "Facebook admits to scraping every Australian adult user's public photos and posts to train AI, with no opt-out option."
A summary:
"The company does not offer Australians an opt out option like it does in the EU, because it has not been required to do so under privacy law."
https://www.abc.net.au/news/2024-09-11/facebook-scraping-photos-data-no-opt-out/104336170
BTW the ABC News website has links to Facebook, Instagram and X but no Fediverse profiles. So their posts are used to train #Llama
#privacy#invasion of privacy#meta#facebook#social networks#social media#auslaw#artificial intelligence#fuck ai#anti ai#ausgov#politas#auspol#tasgov#taspol#australia#fuck neoliberals#neoliberal capitalism#anthony albanese#albanese government#mark zuckerberg#fuck mark zuckerberg#class war#data privacy#oppression#repression#boycott facebook#fuck facebook#free all oppressed peoples#oppressor
5 notes
·
View notes
Text
Why Did India’s Finance Ministry Restrict the Use of AI Tools in Offices? A Closer Look at the Decision
In a significant move, India’s Finance Ministry recently issued an advisory restricting the use of artificial intelligence (AI) tools, such as ChatGPT, Bard, and other generative AI platforms, in government offices. This decision has sparked widespread debate, with many questioning the rationale behind it. Why would a government, in an era of rapid technological advancement, curb the use of tools that promise efficiency and innovation? Let’s delve into the logic and reasoning behind this decision, including the geopolitical implications and the growing global AI race, particularly with China. Read more
#Finance Ministry India AI ban#AI tools restriction India#data security and AI#geopolitical AI race#China AI development#AI governance India#ChatGPT and DeepSeek ban in government#AI and national security#indigenous AI solutions#ethical AI use in government.
2 notes
·
View notes
Text
Elon Musk’s Ally Pushes for ‘AI-First’ Strategy in Government Agency
Elon Musk’s Ally Pushes for ‘AI-First’ Strategy in Government Agency In a groundbreaking statement, a close ally of Elon Musk has revealed that embracing an “AI-first” approach is the future for a key government agency. This bold vision outlines how artificial intelligence (AI) will shape the operations and policies of government entities, especially those tied to technology and national…
#AI adoption#AI collaboration#AI in defense#AI in government#AI strategy#AI technology#AI-driven government#AI-first#artificial intelligence#cybersecurity#data analysis#Elon Musk#future of AI#government agency#government reform#Musk&039;s vision#national security#public sector#public services#tech industry#technology innovation
2 notes
·
View notes
Text
Mark your calendar for these health tech conferences in 2024-2025

- By InnoNurse Staff -
Interested in health technology-related events for fall 2024 and 2025? Fierce Healthcare has compiled a list of key conferences, both virtual and in-person, scheduled for the upcoming seasons.
Read more at Fierce Healthcare
///
Other recent news and insights
Lapsi transforms the stethoscope into a health tracking data platform (TechCrunch)
UK: The Department of Health and Social Care set to review clinical risk standards for digital health technologies (Digital Health)
AI-based cancer test determines if chemotherapy is needed (The Financial Express)
New tool enhances microscopic imaging by eliminating motion artifacts (UC Berkeley/Tech Xplore)
Researchers integrate a fast optical coherence tomography system into neurosurgical microscopes (Optica)
AI model achieves clinical-expert-level accuracy in complex medical scans (UCLA/Medical Xpress)
Bioinformatics reveals the hidden prevalence of repeat expansion disorders (Queen Mary University of London/Medical Xpress)
Ultrasound detects 96% of ovarian cancers in postmenopausal women (University of Birmingham)
AI ‘liquid biopsies’ using cell-free DNA and protein biomarkers could improve early ovarian cancer detection (Johns Hopkins Technology Ventures)
Mammograms show potential for detecting heart disease (UC San Diego/Medical Xpress)
IMRT and proton therapy provide similar quality of life and tumor control for prostate cancer patients (American Society for Radiation Oncology/Medical Xpress)
Machine learning enhances MRI video quality (Graz University of Technology/Medical Xpress)
Robotic surgery for colorectal cancer reduces pain and accelerates recovery (Beth Israel Deaconess Medical Center)
Global human brain mapping project releases its first data set (Allen Institute)
AI could speed up PCR tests, aiding faster DNA diagnostics and forensics (Flinders University/Medical Xpress)
AI-powered apps may detect depression through eye snapshots (Stevens Institute of Technology/Medical Xpress)
#events#health tech#digital health#medtech#biotech#health informatics#data science#neuroscience#imaging#radiology#diagnostics#ai#robotics#cancer#lapsi#government#uk
2 notes
·
View notes
Text
Why Quantum Computing Will Change the Tech Landscape
The technology industry has seen significant advancements over the past few decades, but nothing quite as transformative as quantum computing promises to be. Why Quantum Computing Will Change the Tech Landscape is not just a matter of speculation; it’s grounded in the science of how we compute and the immense potential of quantum mechanics to revolutionise various sectors. As traditional…
#AI#AI acceleration#AI development#autonomous vehicles#big data#classical computing#climate modelling#complex systems#computational power#computing power#cryptography#cybersecurity#data processing#data simulation#drug discovery#economic impact#emerging tech#energy efficiency#exponential computing#exponential growth#fast problem solving#financial services#Future Technology#government funding#hardware#Healthcare#industry applications#industry transformation#innovation#machine learning
2 notes
·
View notes
Text
Recently stumbled across this remnant of a trend in my meme folder and I do not remember making this at all
#diary of a wimpy kid#greg heffley#shitpost#meme#anime#ai#reminder that this whole website was a front for a company developing facial id software for the Chinese government to obtain data
2 notes
·
View notes
Text
Eric Schmidt: AI misuse poses an ‘extreme risk’
New Post has been published on https://thedigitalinsider.com/eric-schmidt-ai-misuse-poses-an-extreme-risk/
Eric Schmidt: AI misuse poses an ‘extreme risk’
Eric Schmidt, former CEO of Google, has warned that AI misuse poses an “extreme risk” and could do catastrophic harm.
Speaking to BBC Radio 4’s Today programme, Schmidt cautioned that AI could be weaponised by extremists and “rogue states” such as North Korea, Iran, and Russia to “harm innocent people.”
Schmidt expressed concern that rapid AI advancements could be exploited to create weapons, including biological attacks. Highlighting the dangers, he said: “The real fears that I have are not the ones that most people talk about AI, I talk about extreme risk.”
Using a chilling analogy, Schmidt referenced the al-Qaeda leader responsible for the 9/11 attacks: “I’m always worried about the Osama bin Laden scenario, where you have some truly evil person who takes over some aspect of our modern life and uses it to harm innocent people.”
He emphasised the pace of AI development and its potential to be co-opted by nations or groups with malevolent intent.
“Think about North Korea, or Iran, or even Russia, who have some evil goal … they could misuse it and do real harm,” Schmidt warns.
Oversight without stifling innovation
Schmidt urged governments to closely monitor private tech companies pioneering AI research. While noting that tech leaders are generally aware of AI’s societal implications, they may make decisions based on different values from those of public officials.
“My experience with the tech leaders is that they do have an understanding of the impact they’re having, but they might make a different values judgement than the government would make.”
Schmidt also endorsed the export controls introduced under former US President Joe Biden last year to restrict the sale of advanced microchips. The measure is aimed at slowing the progress of geopolitical adversaries in AI research.
Global divisions around preventing AI misuse
The tech veteran was in Paris when he made his remarks, attending the AI Action Summit, a two-day event that wrapped up on Tuesday.
The summit, attended by 57 countries, saw the announcement of an agreement on “inclusive” AI development. Signatories included major players like China, India, the EU, and the African Union.
However, the UK and the US declined to sign the communique. The UK government said the agreement lacked “practical clarity” and failed to address critical “harder questions” surrounding national security.
Schmidt cautioned against excessive regulation that might hinder progress in this transformative field. This was echoed by US Vice-President JD Vance who warned that heavy-handed regulation “would kill a transformative industry just as it’s taking off”.
This reluctance to endorse sweeping international accords reflects diverging approaches to AI governance. The EU has championed a more restrictive framework for AI, prioritising consumer protections, while countries like the US and UK are opting for more agile and innovation-driven strategies.
Schmidt pointed to the consequences of Europe’s tight regulatory stance, predicting that the region would miss out on pioneering roles in AI.
“The AI revolution, which is the most important revolution in my opinion since electricity, is not going to be invented in Europe,” he remarked.
Prioritising national and global safety
Schmidt’s comments come against a backdrop of increasing scrutiny over AI’s dual-use potential—its ability to be used for both beneficial and harmful purposes.
From deepfakes to autonomous weapons, AI poses a bevy of risks if left without measures to guard against misuse. Leaders and experts, including Schmidt, are advocating for a balanced approach that fosters innovation while addressing these dangers head-on.
While international cooperation remains a complex and contentious issue, the overarching consensus is clear: without safeguards, AI’s evolution could have unintended – and potentially catastrophic – consequences.
(Photo by Guillaume Paumier under CC BY 3.0 license. Cropped to landscape from original version.)
See also: NEPC: AI sprint risks environmental catastrophe
Want to learn more about AI and big data from industry leaders? Check out AI & Big Data Expo taking place in Amsterdam, California, and London. The comprehensive event is co-located with other leading events including Intelligent Automation Conference, BlockX, Digital Transformation Week, and Cyber Security & Cloud Expo.
Explore other upcoming enterprise technology events and webinars powered by TechForge here.
#adversaries#agile#agreement#ai#ai & big data expo#ai action summit#AI development#ai governance#AI research#amp#approach#Artificial Intelligence#automation#autonomous#BBC#biden#Big Data#california#CEO#China#Cloud#Companies#comprehensive#conference#cyber#cyber security#data#deepfakes#development#Digital Transformation
5 notes
·
View notes
Text
At the California Institute of the Arts, it all started with a videoconference between the registrar’s office and a nonprofit.
One of the nonprofit’s representatives had enabled an AI note-taking tool from Read AI. At the end of the meeting, it emailed a summary to all attendees, said Allan Chen, the institute’s chief technology officer. They could have a copy of the notes, if they wanted — they just needed to create their own account.
Next thing Chen knew, Read AI’s bot had popped up inabout a dozen of his meetings over a one-week span. It was in one-on-one check-ins. Project meetings. “Everything.”
The spread “was very aggressive,” recalled Chen, who also serves as vice president for institute technology. And it “took us by surprise.”
The scenariounderscores a growing challenge for colleges: Tech adoption and experimentation among students, faculty, and staff — especially as it pertains to AI — are outpacing institutions’ governance of these technologies and may even violate their data-privacy and security policies.
That has been the case with note-taking tools from companies including Read AI, Otter.ai, and Fireflies.ai.They can integrate with platforms like Zoom, Google Meet, and Microsoft Teamsto provide live transcriptions, meeting summaries, audio and video recordings, and other services.
Higher-ed interest in these products isn’t surprising.For those bogged down with virtual rendezvouses, a tool that can ingest long, winding conversations and spit outkey takeaways and action items is alluring. These services can also aid people with disabilities, including those who are deaf.
But the tools can quickly propagate unchecked across a university. They can auto-join any virtual meetings on a user’s calendar — even if that person is not in attendance. And that’s a concern, administrators say, if it means third-party productsthat an institution hasn’t reviewedmay be capturing and analyzing personal information, proprietary material, or confidential communications.
“What keeps me up at night is the ability for individual users to do things that are very powerful, but they don’t realize what they’re doing,” Chen said. “You may not realize you’re opening a can of worms.“
The Chronicle documented both individual and universitywide instances of this trend. At Tidewater Community College, in Virginia, Heather Brown, an instructional designer, unwittingly gave Otter.ai’s tool access to her calendar, and it joined a Faculty Senate meeting she didn’t end up attending. “One of our [associate vice presidents] reached out to inform me,” she wrote in a message. “I was mortified!”
23K notes
·
View notes
Text
How to Use Telemetry Pipelines to Maintain Application Performance.
Sanjay Kumar Mohindroo Sanjay Kumar Mohindroo. skm.stayingalive.in Optimize application performance with telemetry pipelines—enhance observability, reduce costs, and ensure security with efficient data processing. 🚀 Discover how telemetry pipelines optimize application performance by streamlining observability, enhancing security, and reducing costs. Learn key strategies and best…
#AI-powered Observability#Anonymization#Application Performance#Cloud Computing#Cost Optimization#Cybersecurity#Data Aggregation#Data Filtering#Data Normalization#Data Processing#Data Retention Policies#Debugging Techniques#DevOps#digital transformation#Edge Telemetry Processing#Encryption#GDPR#HIPAA#Incident Management#IT Governance#Latency Optimization#Logging#Machine Learning in Observability#Metrics#Monitoring#News#Observability#Real-Time Alerts#Regulatory Compliance#Sanjay Kumar Mohindroo
0 notes
Text
#Tags:AI in Government#AI-Driven Governance#Apple-Starlink Integration#Big Tech Monopoly#Corporate-Government Merger#Cybersecurity#Data Privacy and Control#Digital Dependence#Digital Surveillance#Elon Musk and Government Influence#facts#life#Musk's Expanding Empire#New World Order (NWO)#Podcast#Ransomware#Satellite-Based Surveillance#serious#SpaceX and Telecommunications#straight forward#Technocratic Control#truth#upfront#website
0 notes
Text
Social networks in 2024 are giving the public every reason not to use them
#social networks#social media#artificial intelligence#data scraping#fuck ai#anti artificial intelligence#anti ai#class war#ausgov#politas#auspol#tasgov#taspol#australia#fuck neoliberals#neoliberal capitalism#anthony albanese#albanese government
5 notes
·
View notes
Text
Google Play Protect 2025: Revocarea Automată a Permisiunilor și Noile Măsuri de Securitate Android
Google a anunțat pe 29 ianuarie 2025 mai multe măsuri noi pentru îmbunătățirea securității dispozitivelor Android. Printre cele mai importante schimbări se numără revocarea automată a permisiunilor aplicațiilor periculoase, protecția avansată împotriva aplicațiilor instalate din surse terțe și introducerea de insigne de verificare pentru aplicațiile oficiale guvernamentale și VPN-urile de…
#Android 11#Android malware#Android security#aplicații guvernamentale#Aplicații Periculoase#atacuri cibernetice#automatic permission revocation#bam#cyber attacks#dangerous apps#data protection#diagnoza#Google AI#Google AI security#Google Play Protect#Google Play Protect update#government apps#insigne verificare aplicații#Malware Android#neamt#online safety#Play Store#Play Store safety#protecție date#revocare automată permisiuni#roman#securitate Android#sideloading#sideloading risks#siguranța online
0 notes