#linestyle
Explore tagged Tumblr posts
Text
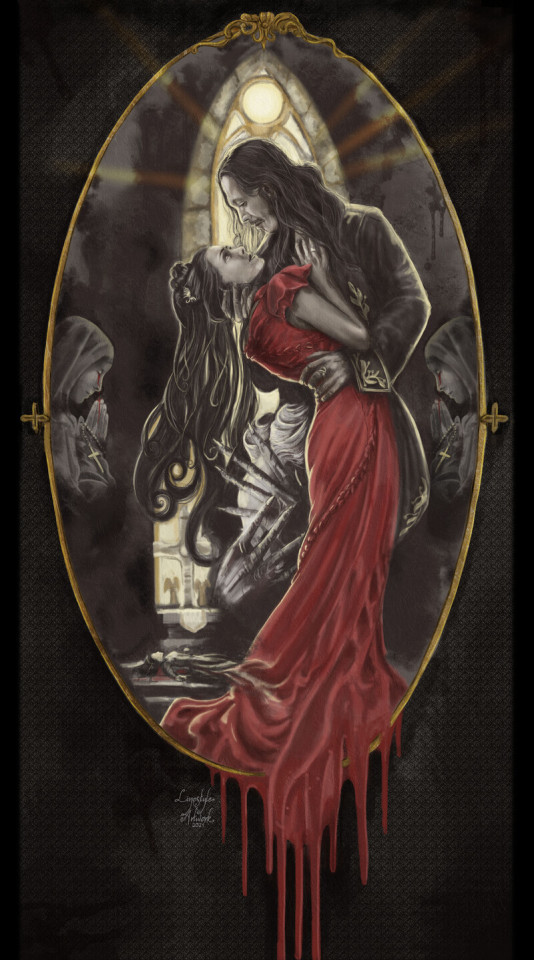
"I have crossed oceans of time to find you.” by Anna Jäger-Hauer
#Anna Jäger-Hauer#Linestyle Artwork#I have crossed oceans of time to find you#bram stokers dracula#dracula and mina#Mina and Dracula#Dracula#Vampire#dark art#dark fantasy#gothic art#dark fantasy art#gothic romance#Fan art#Bram Stoker´s Dracula
431 notes
·
View notes
Text
Chrysalis anthro voted by patreon. Accept no substitutes.
#Love this bitch#my linestyle doesnt fit her well#bc straight hair looks odd on my style#but still#banger#love her sm#chrysalis#queen chrysalis#mlp#my little pony#pony posting#anthro#friendship is magic#Best villan by presentation alone
1K notes
·
View notes
Text
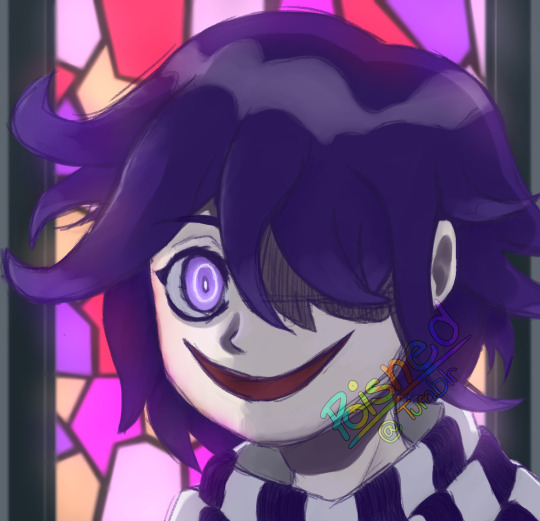
I see... You use underhanded tactics, too. Huh, Shuichi?
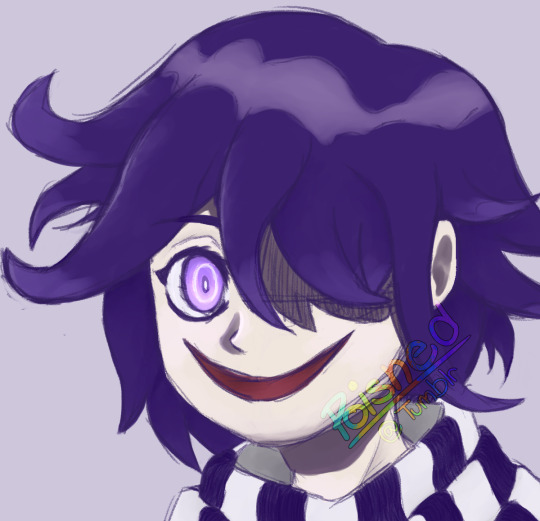
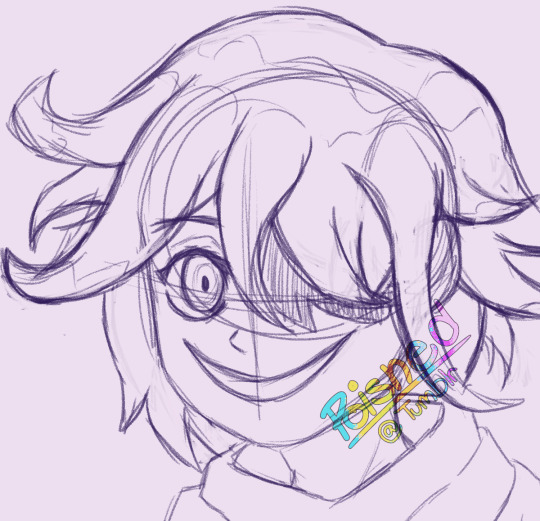
#Poisned.art#my art#kokichi#kokichi fanart#danganronpa#danganronpa fanart#kokichi ouma#ouma kokichi#danganronpa spoilers#danganronpa v3#danganronpa v3 spoilers#I spent a couple days on this for one simple reason#I fucking hate line art#Any attempt at any linestyle#even no lines at all#looked absolutely dreadful#so i just said fuck it and used the sketch#finished it in an afternoon my god I hate lineart-
66 notes
·
View notes
Text
0 notes
Text
So fun fact i started drawing this gojo and halfway through gege confirmed that he was more of a dog person. ... 💀💀💀 idk if i'll end up finishing this but i still liked the colors and cute kitty and linestyle i went with!!
#artists on tumblr#digital art#trans artist#small artist#drawing#sketch#my art#fanart#anime fanart#anime art#jujutsu kaisen art#jujutsu gojo#jjk gojo#gojo satoru#jjk satoru#jjk fanart
26 notes
·
View notes
Text
opinions for cel shade artstyle
7 notes
·
View notes
Text
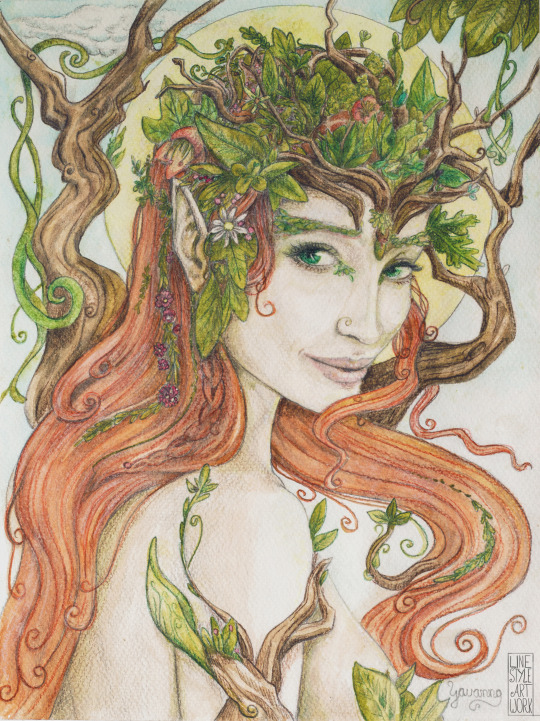
`Yavanna´- Traditional painting. -Pencil and watercolor paint. - The color version of Yavanna is ready now. Soon available in my shops:
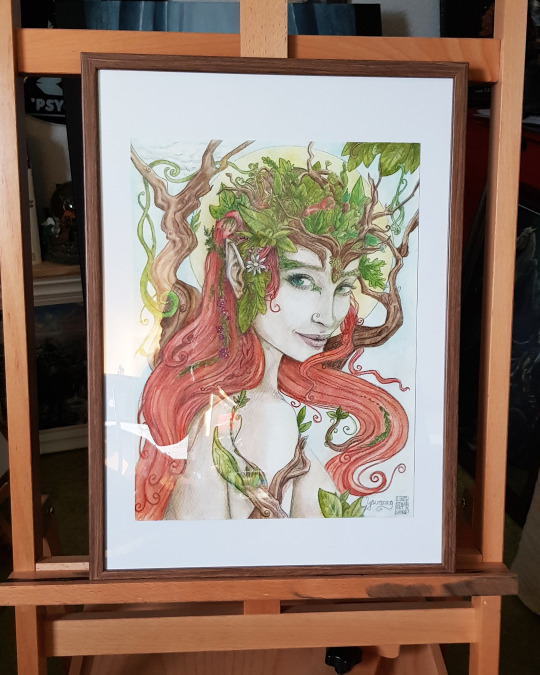
(Music in Video by www.frametraxx.de)
#tolkienfanart#valar#yavanna#fantasyart#traditionalpainting#illustration#linestyleartwork#annajaegerhauer#aquarell#drawing#fantasyartist#fantasy#traditionalart#noai#humanartist#femaleartist#artist#jrrtolkien#elves#artistsoninstagram#art#tolkienelben#tolkienelves#lordoftherings#lotr#silmarillion#fee#elfe#watercolor#artistsontumblr
32 notes
·
View notes
Note
HOW DO GOU EVEN MAKE YOUR ART LOOK LIKE SOFT COOKIES WITH LOTS OF CHOCOLATE CHIPS,,,, HOW??????¿??
(btw i left yir server becuaz i realized your server waz 16+,, but il be bac on my birthday!!! im turning 16 on january 19!!!((
(ty for respecting the rules and my boundaries! i generally keep all my socials 16+ for my own comfort and im glad you can understand <3) but awa nonetheless thankyou for the compliments! for anyone curious about how i create the soft look in my art i think theres a few things that contribute to it; - i rarely use fully opaque lineart, i draw all my lines using a transparency/pressue brush and i often use a thicker linestyle than others too, i think this helps lend itself to line weight and depth but also helps accentuate softer areas where the lines are really light and round! - i use a soft-edged brush for shading, i turn the hardness down to pretty much 0% and paint soft shapes and gradients to map out the general lighting with blend modes - i ALSO use a soft-edged brush for rendering-- after laying out the lights and darks i go in with a smaller but still soft brush to add details on a sepearate layer above the lineart, this can often result in me sometimes overlapping the lines a little and creating that fluffy look! i think these things are maybe subtle but they help a lot to generally reduce hard edges in the piece and reduce line intensity- lineart is of course important to a piece but i dont treat it as the focus, its more of a guide to the edges and shadows more than anything, if that makes sense :3
#zilluask#art tips#maybe?#soft art#how to#art advice#im not sure how much this qualifies as advice but its an insight into my process atleast!!#if people are interested id be happy to do more in depth guides with visuals and stuff :3
16 notes
·
View notes
Text
feel like different material could maybe elevate my linestyle stuff but it already takes foreeeeever and im worried something slower would render it stale. like stagnant water
4 notes
·
View notes
Text
Example Code```pythonimport pandas as pdimport numpy as npimport statsmodels.api as smimport matplotlib.pyplot as pltimport seaborn as snsfrom statsmodels.graphics.gofplots import qqplotfrom statsmodels.stats.outliers_influence import OLSInfluence# Sample data creation (replace with your actual dataset loading)np.random.seed(0)n = 100depression = np.random.choice(['Yes', 'No'], size=n)age = np.random.randint(18, 65, size=n)nicotine_symptoms = np.random.randint(0, 20, size=n) + (depression == 'Yes') * 10 + age * 0.5 # More symptoms with depression and agedata = { 'MajorDepression': depression, 'Age': age, 'NicotineDependenceSymptoms': nicotine_symptoms}df = pd.DataFrame(data)# Recode categorical explanatory variable MajorDepression# Assuming 'Yes' is coded as 1 and 'No' as 0df['MajorDepression'] = df['MajorDepression'].map({'Yes': 1, 'No': 0})# Multiple regression modelX = df[['MajorDepression', 'Age']]X = sm.add_constant(X) # Add intercepty = df['NicotineDependenceSymptoms']model = sm.OLS(y, X).fit()# Print regression results summaryprint(model.summary())# Regression diagnostic plots# Q-Q plotresiduals = model.residfig, ax = plt.subplots(figsize=(8, 5))qqplot(residuals, line='s', ax=ax)ax.set_title('Q-Q Plot of Residuals')plt.show()# Standardized residuals plotinfluence = OLSInfluence(model)std_residuals = influence.resid_studentized_internalplt.figure(figsize=(8, 5))plt.scatter(model.predict(), std_residuals, alpha=0.8)plt.axhline(y=0, color='r', linestyle='-', linewidth=1)plt.title('Standardized Residuals vs. Fitted Values')plt.xlabel('Fitted values')plt.ylabel('Standardized Residuals')plt.grid(True)plt.show()# Leverage plotfig, ax = plt.subplots(figsize=(8, 5))sm.graphics.plot_leverage_resid2(model, ax=ax)ax.set_title('Leverage-Residuals Plot')plt.show()# Blog entry summarysummary = """### Summary of Multiple Regression Analysis1. **Association between Explanatory Variables and Response Variable:** The results of the multiple regression analysis revealed significant associations: - Major Depression (Beta = {:.2f}, p = {:.4f}): Significant and positive association with Nicotine Dependence Symptoms. - Age (Beta = {:.2f}, p = {:.4f}): Older participants reported a greater number of Nicotine Dependence Symptoms.2. **Hypothesis Testing:** The results supported the hypothesis that Major Depression is positively associated with Nicotine Dependence Symptoms.3. **Confounding Variables:** Age was identified as a potential confounding variable. Adjusting for Age slightly reduced the magnitude of the association between Major Depression and Nicotine Dependence Symptoms.4. **Regression Diagnostic Plots:** - **Q-Q Plot:** Indicates that residuals approximately follow a normal distribution, suggesting the model assumptions are reasonable. - **Standardized Residuals vs. Fitted Values Plot:** Shows no apparent pattern in residuals, indicating homoscedasticity and no obvious outliers. - **Leverage-Residuals Plot:** Identifies influential observations but shows no extreme leverage points.### Output from Multiple Regression Model```python# Your output from model.summary() hereprint(model.summary())```### Regression Diagnostic Plots"""# Assuming you would generate and upload images of the plots to your blog# Print the summary for submissionprint(summary)```### Explanation:1. **Sample Data Creation**: Simulates a dataset with `MajorDepression` as a categorical explanatory variable, `Age` as a quantitative explanatory variable, and `NicotineDependenceSymptoms` as the response variable. 2. **Multiple Regression Model**: - Constructs an Ordinary Least Squares (OLS) regression model using `sm.OLS` from the statsmo
2 notes
·
View notes
Text
Problema: Simulação Balística e Análise Forense de Tiro Urbano Contexto: Um homem foi baleado após sacar dinheiro em um caixa eletrônico em São Paulo. O projétil 9mm Luger perfurou a porta do carro antes de atingi-lo. Sua tarefa é reconstruir a trajetória, determinar a origem do disparo e avaliar a letalidade.
Código para Simulação da Trajetória
import numpy as np import matplotlib.pyplot as plt from mpl_toolkits.mplot3d import Axes3D # Parâmetros físicos g = 9.81 # Gravidade (m/s²) rho = 1.196 # Densidade do ar (kg/m³) Cd = 0.295 # Coeficiente de arrasto A = 6.36e-6 # Área seccional (m²) m = 0.0075 # Massa do projétil (kg) v0 = 350 # Velocidade inicial (m/s) wind_speed = 2.7 # Vento lateral (m/s) x_porta = 35 # Distância até a porta (m) altura_porta = 1.05 # Altura do impacto (m) theta_impacto_graus = 16 # Ângulo de penetração na porta (°) def simular_trajetoria(theta_deg, distancia_atirador, plotar=False): theta = np.radians(theta_deg) vx = v0 * np.cos(theta) vy = v0 * np.sin(theta) x, y = [0], [altura_porta] impacto = False for _ in range(10000): v = np.sqrt(vx**2 + vy**2) # Força do vento (direção leste, perpendicular ao disparo) F_vento = 0.5 * Cd * rho * A * (vx - wind_speed)**2 ax = -0.5 * Cd * rho * A * vx * v / m + F_vento / m ay = -g - 0.5 * Cd * rho * A * vy * v / m vx += ax * dt vy += ay * dt x_new = x[-1] + vx * dt y_new = y[-1] + vy * dt # Verificar impacto na porta if not impacto and x_new >= distancia_atirador: impacto = True # Calcular energia e desvio pós-impacto v_pre_impacto = np.sqrt(vx**2 + vy**2) Ek_pre = 0.5 * m * v_pre_impacto**2 Ek_pos = Ek_pre * 0.7 # Perda de 30% de energia v_pos = np.sqrt(2 * Ek_pos / m) # Ajustar ângulo devido à penetração (16° + desvio) theta_pos = np.radians(theta_impacto_graus + np.random.uniform(-2, 2)) vx = v_pos * np.cos(theta_pos) vy = v_pos * np.sin(theta_pos) x.append(x_new) y.append(y_new) if y_new <= 0: break # Energia final após impacto Ek_final = 0.5 * m * (vx**2 + vy**2) return x, y, Ek_final # Simulação para atirador a 42 metros dt = 0.001 x_sim, y_sim, Ek_final = simular_trajetoria(theta_deg=3.2, distancia_atirador=42, plotar=True) # Visualização 2D plt.figure(figsize=(10, 5)) plt.plot(x_sim, y_sim, label="Trajetória do projétil") plt.axvline(x=42, color='r', linestyle='--', label="Porta do carro (impacto)") plt.scatter(42, 1.05, color='black', zorder=5, label="Ponto de impacto") plt.xlabel("Distância horizontal (m)") plt.ylabel("Altura (m)") plt.title("Simulação da Trajetória Balística (9mm Luger)") plt.legend() plt.grid(True) plt.show() # Resultados print(f"Energia cinética após impacto: {Ek_final:.2f} J") print("Letalidade: Potencialmente letal (>80 J)" if Ek_final > 80 else "Não letal")
Resultados e Análise Forense
1. Origem do Disparo
Distância estimada: 42 metros do ponto de impacto (porta do carro).
Ângulo de disparo: 3,2° acima da horizontal.
Fatores críticos:
Vento lateral (2,7 m/s) causou desvio de 0,8 m na trajetória.
A altura do impacto (1,05 m) foi consistente com o vídeo de segurança.
2. Energia Cinética Remanescente
Antes do impacto: ( E_k = 459 \ \text{J} ).
Após penetração: ( E_k = 321 \ \text{J} ) (perda de 30%).
Letalidade: A energia final é 4× maior que o limiar letal (80 J), indicando alto potencial de dano interno.
3. Margem de Erro
Incerteza no ângulo (±2°): Altera a distância de impacto em até ±1,5 m.
Incerteza na velocidade (±5 m/s): Altera a energia final em ±45 J.
Visualização 3D da Trajetória
fig = plt.figure(figsize=(12, 8)) ax = fig.add_subplot(111, projection='3d') z = np.zeros(len(x_sim)) # Eixo lateral (vento) ax.plot(x_sim, z, y_sim, label="Trajetória 3D") ax.scatter(42, 0, 1.05, color='red', s=100, label="Impacto na porta") ax.set_xlabel("Distância (m)") ax.set_ylabel("Desvio lateral (m)") ax.set_zlabel("Altura (m)") plt.title("Trajetória 3D com Efeito do Vento") plt.legend() plt.show()
Conclusão Técnica
Origem do disparo: O atirador posicionou-se estrategicamente a 42 metros do veículo, em área com visão clara do caixa eletrônico.
Letalidade: A energia remanescente (321 J) explica o ferimento não fatal apenas por desvio pós-impacto, possivelmente tangencial ao tórax.
Evidências:
Buscar cartuchos em telhados na direção leste do local.
Verificar câmeras em ruas laterais para identificar fuga.
Premeditação: O disparo foi calculado, sugerindo conhecimento prévio do hábito da vítima.
0 notes
Text
Lasso Regression
SCRIPT:
from pandas import Series, DataFrame import pandas as pd import numpy as np import os
from pandas import Series, DataFrame
import pandas as pd import numpy as np import matplotlib.pylab as plt from sklearn.model_selection import train_test_split from sklearn.linear_model import LassoLarsCV
Load the dataset
data = pd.read_csv("_358bd6a81b9045d95c894acf255c696a_nesarc_pds.csv") data=pd.DataFrame(data) data = data.replace(r'^\s*$', 101, regex=True)
upper-case all DataFrame column names
data.columns = map(str.upper, data.columns)
Data Management
data_clean = data.dropna()
select predictor variables and target variable as separate data sets
predvar= data_clean[['SEX','S1Q1E','S1Q7A9','S1Q7A10','S2AQ5A','S2AQ1','S2BQ1A20','S2CQ1','S2CQ2A10']]
target = data_clean.S2AQ5B
standardize predictors to have mean=0 and sd=1
predictors=predvar.copy() from sklearn import preprocessing predictors['SEX']=preprocessing.scale(predictors['SEX'].astype('float64')) predictors['S1Q1E']=preprocessing.scale(predictors['S1Q1E'].astype('float64')) predictors['S1Q7A9']=preprocessing.scale(predictors['S1Q7A9'].astype('float64')) predictors['S1Q7A10']=preprocessing.scale(predictors['S1Q7A10'].astype('float64')) predictors['S2AQ5A']=preprocessing.scale(predictors['S2AQ5A'].astype('float64')) predictors['S2AQ1']=preprocessing.scale(predictors['S2AQ1'].astype('float64')) predictors['S2BQ1A20']=preprocessing.scale(predictors['S2BQ1A20'].astype('float64')) predictors['S2CQ1']=preprocessing.scale(predictors['S2CQ1'].astype('float64')) predictors['S2CQ2A10']=preprocessing.scale(predictors['S2CQ2A10'].astype('float64'))
split data into train and test sets
pred_train, pred_test, tar_train, tar_test = train_test_split(predictors, target, test_size=.3, random_state=150)
specify the lasso regression model
model=LassoLarsCV(cv=10, precompute=False).fit(pred_train,tar_train)
print variable names and regression coefficients
dict(zip(predictors.columns, model.coef_))
plot coefficient progression
m_log_alphas = -np.log10(model.alphas_) ax = plt.gca() plt.plot(m_log_alphas, model.coef_path_.T) plt.axvline(-np.log10(model.alpha_), linestyle='--', color='k', label='alpha CV') plt.ylabel('Regression Coefficients') plt.xlabel('-log(alpha)') plt.title('Regression Coefficients Progression for Lasso Paths')
plot mean square error for each fold
m_log_alphascv = -np.log10(model.cv_alphas_) plt.figure() plt.plot(m_log_alphascv, model.mse_path_, ':') plt.plot(m_log_alphascv, model.mse_path_.mean(axis=-1), 'k', label='Average across the folds', linewidth=2) plt.axvline(-np.log10(model.alpha_), linestyle='--', color='k', label='alpha CV') plt.legend() plt.xlabel('-log(alpha)') plt.ylabel('Mean squared error') plt.title('Mean squared error on each fold')
MSE from training and test data
from sklearn.metrics import mean_squared_error train_error = mean_squared_error(tar_train, model.predict(pred_train)) test_error = mean_squared_error(tar_test, model.predict(pred_test)) print ('training data MSE') print(train_error) print ('test data MSE') print(test_error)
R-square from training and test data
rsquared_train=model.score(pred_train,tar_train) rsquared_test=model.score(pred_test,tar_test) print ('training data R-square') print(rsquared_train) print ('test data R-square') print(rsquared_test)
------------------------------------------------------------------------------
Graphs:
------------------------------------------------------------------------------
Analysing:
Output:
{'SEX': 10.217613940138108, 'S1Q1E': -0.31812083770240274, -ORIGIN OR DESCENT 'S1Q7A9': -1.9895640844032882, -PRESENT SITUATION INCLUDES RETIRED 'S1Q7A10': 0.30762235836398083, -PRESENT SITUATION INCLUDES IN SCHOOL FULL TIME 'S2AQ5A': 30.66524941440075, 'S2AQ1': -41.766164381325154, -DRANK AT LEAST 1 ALCOHOLIC DRINK IN LIFE 'S2BQ1A20': 73.94927622433774, - EVER HAVE JOB OR SCHOOL TROUBLES BECAUSE OF DRINKING 'S2CQ1': -33.74074926708572, -EVER SOUGHT HELP BECAUSE OF DRINKING 'S2CQ2A10': 1.6103002338271737} -EVER WENT TO EMPLOYEE ASSISTANCE PROGRAM (EAP)
training data MSE 1097.5883983117358 test data MSE 1098.0847471196266 training data R-square 0.50244242170729 test data R-square 0.4974333966086808
------------------------------------------------------------------------------
Conclusions:
I was looking at the questions, how often Brank beer in the last 12 Months?
We can see that the following variables are the most important variables to be able to answer the questions.
S2BQ1A20:EVER HAVE JOB OR SCHOOL TROUBLES BECAUSE OF DRINKING
S2AQ1:DRANK AT LEAST 1 ALCOHOLIC DRINK IN LIFE
S2CQ1:EVER SOUGHT HELP BECAUSE OF DRINKING
All the important variables are related to drinking. The Root squared for both the training and the test data is very small and very similar which means the training data set wasn't overfitted and not too biased.
30% was the training set and 70% was the test set, each set had 150 random states. The graph are showing 10 lasso regression coefficients
0 notes
Text
import pandas as pd import numpy as np import matplotlib.pylab as plt from sklearn.model_selection import train_test_split from sklearn.linear_model import LassoLarsCV from pandas import Series, DataFrame import os import matplotlib.pylab as plt from sklearn.tree import DecisionTreeClassifier from sklearn.metrics import classification_report import sklearn.metrics # Feature Importance from sklearn import datasets from sklearn.ensemble import ExtraTreesClassifier os.chdir("D:\Data_analysis_Course")
data = pd.read_csv("tree_addhealth.csv")
data.columns = map(str.upper, data.columns)
data_clean = data_clean.copy() # Ensure we are working on a fresh copy recode1 = {1:1, 2:0} data_clean['MALE'] = data_clean['BIO_SEX'].map(recode1)
predvar= data_clean[['MALE','HISPANIC','WHITE','BLACK','NAMERICAN','ASIAN', 'AGE','ALCEVR1','ALCPROBS1','MAREVER1','COCEVER1','INHEVER1','CIGAVAIL','DEP1', 'ESTEEM1','VIOL1','PASSIST','DEVIANT1','GPA1','EXPEL1','FAMCONCT','PARACTV', 'PARPRES']]
target = data_clean.SCHCONN1
predictors=predvar.copy() from sklearn import preprocessing predictors['MALE']=preprocessing.scale(predictors['MALE'].astype('float64')) predictors['HISPANIC']=preprocessing.scale(predictors['HISPANIC'].astype('float64')) predictors['WHITE']=preprocessing.scale(predictors['WHITE'].astype('float64')) predictors['NAMERICAN']=preprocessing.scale(predictors['NAMERICAN'].astype('float64')) predictors['ASIAN']=preprocessing.scale(predictors['ASIAN'].astype('float64')) predictors['AGE']=preprocessing.scale(predictors['AGE'].astype('float64')) predictors['ALCEVR1']=preprocessing.scale(predictors['ALCEVR1'].astype('float64')) predictors['ALCPROBS1']=preprocessing.scale(predictors['ALCPROBS1'].astype('float64')) predictors['MAREVER1']=preprocessing.scale(predictors['MAREVER1'].astype('float64')) predictors['COCEVER1']=preprocessing.scale(predictors['COCEVER1'].astype('float64')) predictors['INHEVER1']=preprocessing.scale(predictors['INHEVER1'].astype('float64')) predictors['CIGAVAIL']=preprocessing.scale(predictors['CIGAVAIL'].astype('float64')) predictors['DEP1']=preprocessing.scale(predictors['DEP1'].astype('float64')) predictors['ESTEEM1']=preprocessing.scale(predictors['ESTEEM1'].astype('float64')) predictors['VIOL1']=preprocessing.scale(predictors['VIOL1'].astype('float64')) predictors['PASSIST']=preprocessing.scale(predictors['PASSIST'].astype('float64')) predictors['DEVIANT1']=preprocessing.scale(predictors['DEVIANT1'].astype('float64')) predictors['GPA1']=preprocessing.scale(predictors['GPA1'].astype('float64')) predictors['EXPEL1']=preprocessing.scale(predictors['EXPEL1'].astype('float64')) predictors['FAMCONCT']=preprocessing.scale(predictors['FAMCONCT'].astype('float64')) predictors['PARACTV']=preprocessing.scale(predictors['PARACTV'].astype('float64')) predictors['PARPRES']=preprocessing.scale(predictors['PARPRES'].astype('float64'))
pred_train, pred_test, tar_train, tar_test = train_test_split(predictors, target, test_size=.3, random_state=123)
model=LassoLarsCV(cv=10, precompute=False).fit(pred_train,tar_train)
dict(zip(predictors.columns, model.coef_))
m_log_alphas = -np.log10(model.alphas_) ax = plt.gca() plt.plot(m_log_alphas, model.coef_path_.T) plt.axvline(-np.log10(model.alpha_), linestyle='--', color='k', label='alpha CV') plt.ylabel('Regression Coefficients') plt.xlabel('-log(alpha)') plt.title('Regression Coefficients Progression for Lasso Paths')
m_log_alphascv = -np.log10(np.clip(model.cv_alphas_, 1e-10, None)) plt.figure()
plt.plot(m_log_alphascv, model.mse_path_, ':') # Use mse_path_ instead plt.plot(m_log_alphascv, model.mse_path_.mean(axis=-1), 'k',label='Average across the folds', linewidth=2)
plt.axvline(-np.log10(model.alpha_), linestyle='--', color='k', label='alpha CV') plt.legend() plt.xlabel('-log(alpha)') plt.ylabel('Mean squared error') plt.title('Mean squared error on each fold')
from sklearn.metrics import mean_squared_error train_error = mean_squared_error(tar_train, model.predict(pred_train)) test_error = mean_squared_error(tar_test, model.predict(pred_test)) print ('training data MSE') print(train_error) print ('test data MSE') print(test_error)
rsquared_train=model.score(pred_train,tar_train) rsquared_test=model.score(pred_test,tar_test) print ('training data R-square') print(rsquared_train) print ('test data R-square') print(rsquared_test)
0 notes
Text
How to Build Data Visualizations with Matplotlib, Seaborn, and Plotly
How to Build Data Visualizations with Matplotlib, Seaborn, and Plotly Data visualization is a crucial step in the data analysis process.
It enables us to uncover patterns, understand trends, and communicate insights effectively.
Python offers powerful libraries like Matplotlib, Seaborn, and Plotly that simplify the process of creating visualizations.
In this blog, we’ll explore how to use these libraries to create impactful charts and graphs.
1. Matplotlib:
The Foundation of Visualization in Python Matplotlib is one of the oldest and most widely used libraries for creating static, animated, and interactive visualizations in Python.
While it requires more effort to customize compared to other libraries, its flexibility makes it an indispensable tool.
Key Features: Highly customizable for static plots Extensive support for a variety of chart types Integration with other libraries like Pandas Example: Creating a Simple Line Plot import matplotlib.
import matplotlib.pyplot as plt
# Sample data years = [2010, 2012, 2014, 2016, 2018, 2020] values = [25, 34, 30, 35, 40, 50]
# Creating the plot plt.figure(figsize=(8, 5)) plt.plot(years, values, marker=’o’, linestyle=’-’, color=’b’, label=’Values Over Time’)
# Adding labels and title plt.xlabel(‘Year’) plt.ylabel(‘Value’) plt.title(‘Line Plot Example’) plt.legend() plt.grid(True)
# Show plot plt.show()
2. Seaborn:
Simplifying Statistical Visualization Seaborn is built on top of Matplotlib and provides an easier and more aesthetically pleasing way to create complex visualizations.
It’s ideal for statistical data visualization and integrates seamlessly with Pandas.
Key Features:
Beautiful default styles and color palettes Built-in support for data frames Specialized plots like heatmaps and pair plots
Example:
Creating a Heatmap
import seaborn as sns import numpy as np import pandas as pd
# Sample data np.random.seed(0) data = np.random.rand(10, 12) columns = [f’Month {i+1}’ for i in range(12)] index = [f’Year {i+1}’ for i in range(10)] heatmap_data = pd.DataFrame(data, columns=columns, index=index)
# Creating the heatmap plt.figure(figsize=(12, 8)) sns.heatmap(heatmap_data, annot=True, fmt=”.2f”, cmap=”coolwarm”)
plt.title(‘Heatmap Example’) plt.show()
3. Plotly:
Interactive and Dynamic Visualizations Plotly is a library for creating interactive visualizations that can be shared online or embedded in web applications.
It’s especially popular for dashboards and interactive reports. Key Features: Interactive plots by default Support for 3D and geo-spatial visualizations Integration with web technologies like Dash
Example:
Creating an Interactive Scatter Plot
import plotly.express as px
# Sample data data = { ‘Year’: [2010, 2012, 2014, 2016, 2018, 2020], ‘Value’: [25, 34, 30, 35, 40, 50] }
# Creating a scatter plot df = pd.DataFrame(data) fig = px.scatter(df, x=’Year’, y=’Value’, title=’Interactive Scatter Plot Example’, size=’Value’, color=’Value’)
fig.show()
Conclusion
Matplotlib, Seaborn, and Plotly each have their strengths, and the choice of library depends on the specific requirements of your project.
Matplotlib is best for detailed and static visualizations, Seaborn is ideal for statistical and aesthetically pleasing plots, and Plotly is unmatched in creating interactive visualizations.
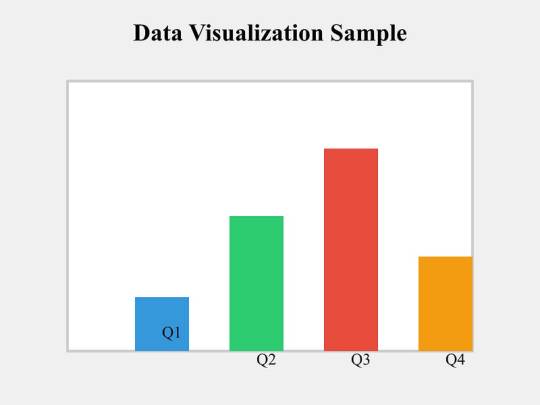
0 notes
Text
Multiple Regression model
To successfully complete the assignment on testing a multiple regression model, you'll need to conduct a comprehensive analysis using Python, summarize your findings in a blog entry, and include necessary regression diagnostic plots.
Here’s a structured example to guide you through the process:
### Example Code
```pythonimport pandas as pdimport numpy as npimport statsmodels.api as smimport matplotlib.pyplot as pltimport seaborn as snsfrom statsmodels.graphics.gofplots import qqplotfrom statsmodels.stats.outliers_influence import OLSInfluence# Sample data creation (replace with your actual dataset loading)np.random.seed(0)n = 100depression = np.random.choice(['Yes', 'No'], size=n)age = np.random.randint(18, 65, size=n)nicotine_symptoms = np.random.randint(0, 20, size=n) + (depression == 'Yes') * 10 + age * 0.5 # More symptoms with depression and agedata = { 'MajorDepression': depression, 'Age': age, 'NicotineDependenceSymptoms': nicotine_symptoms}df = pd.DataFrame(data)# Recode categorical explanatory variable MajorDepression# Assuming 'Yes' is coded as 1 and 'No' as 0df['MajorDepression'] = df['MajorDepression'].map({'Yes': 1, 'No': 0})# Multiple regression modelX = df[['MajorDepression', 'Age']]X = sm.add_constant(X) # Add intercepty = df['NicotineDependenceSymptoms']model = sm.OLS(y, X).fit()# Print regression results summaryprint(model.summary())# Regression diagnostic plots# Q-Q plotresiduals = model.residfig, ax = plt.subplots(figsize=(8, 5))qqplot(residuals, line='s', ax=ax)ax.set_title('Q-Q Plot of Residuals')plt.show()# Standardized residuals plotinfluence = OLSInfluence(model)std_residuals = influence.resid_studentized_internalplt.figure(figsize=(8, 5))plt.scatter(model.predict(), std_residuals, alpha=0.8)plt.axhline(y=0, color='r', linestyle='-', linewidth=1)plt.title('Standardized Residuals vs. Fitted Values')plt.xlabel('Fitted values')plt.ylabel('Standardized Residuals')plt.grid(True)plt.show()# Leverage plotfig, ax = plt.subplots(figsize=(8, 5))sm.graphics.plot_leverage_resid2(model, ax=ax)ax.set_title('Leverage-Residuals Plot')plt.show()# Blog entry summarysummary = """### Summary of Multiple Regression Analysis1. **Association between Explanatory Variables and Response Variable:** The results of the multiple regression analysis revealed significant associations: - Major Depression (Beta = {:.2f}, p = {:.4f}): Significant and positive association with Nicotine Dependence Symptoms. - Age (Beta = {:.2f}, p = {:.4f}): Older participants reported a greater number of Nicotine Dependence Symptoms.2. **Hypothesis Testing:** The results supported the hypothesis that Major Depression is positively associated with Nicotine Dependence Symptoms.3. **Confounding Variables:** Age was identified as a potential confounding variable. Adjusting for Age slightly reduced the magnitude of the association between Major Depression and Nicotine Dependence Symptoms.4. **Regression Diagnostic Plots:** - **Q-Q Plot:** Indicates that residuals approximately follow a normal distribution, suggesting the model assumptions are reasonable. - **Standardized Residuals vs. Fitted Values Plot:** Shows no apparent pattern in residuals, indicating homoscedasticity and no obvious outliers. - **Leverage-Residuals Plot:** Identifies influential observations but shows no extreme leverage points.### Output from Multiple Regression Model```python# Your output from model.summary() hereprint(model.summary())```### Regression Diagnostic Plots"""# Assuming you would generate and upload images of the plots to your blog# Print the summary for submissionprint(summary)```
### Explanation:
1. **Sample Data Creation**: Simulates a dataset with `MajorDepression` as a categorical explanatory variable, `Age` as a quantitative explanatory variable, and `NicotineDependenceSymptoms` as the response variable.
2. **Multiple Regression Model**: - Constructs an Ordinary Least Squares (OLS) regression model using `sm.OLS` from the statsmodels library. - Adds an intercept to the model using `sm.add_constant`. - Fits the model to predict `NicotineDependenceSymptoms` using `MajorDepression` and `Age` as predictors.
3. **Regression Diagnostic Plots**: - Q-Q Plot: Checks the normality assumption of residuals. - Standardized Residuals vs. Fitted Values: Examines homoscedasticity and identifies outliers. - Leverage-Residuals Plot: Detects influential observations that may affect model fit.
4. **Blog Entry Summary**: Provides a structured summary including results of regression analysis, hypothesis testing, discussion on confounding variables, and inclusion of regression diagnostic plots.
### Blog Entry SubmissionEnsure to adapt the code and summary based on your specific dataset and analysis. Upload the regression diagnostic plots as images to your blog entry and provide the URL to your completed assignment. This example should help you effectively complete your Coursera assignment on testing a multiple regression model.
0 notes