#ai sentiment analysis
Explore tagged Tumblr posts
Text
AI-Based Sentiment Analysis for Customer Feedback
0 notes
Text
Bossware is unfair (in the legal sense, too)

You can get into a lot of trouble by assuming that rich people know what they're doing. For example, might assume that ad-tech works – bypassing peoples' critical faculties, reaching inside their minds and brainwashing them with Big Data insights, because if that's not what's happening, then why would rich people pour billions into those ads?
https://pluralistic.net/2020/12/06/surveillance-tulip-bulbs/#adtech-bubble
You might assume that private equity looters make their investors rich, because otherwise, why would rich people hand over trillions for them to play with?
https://thenextrecession.wordpress.com/2024/11/19/private-equity-vampire-capital/
The truth is, rich people are suckers like the rest of us. If anything, succeeding once or twice makes you an even bigger mark, with a sense of your own infallibility that inflates to fill the bubble your yes-men seal you inside of.
Rich people fall for scams just like you and me. Anyone can be a mark. I was:
https://pluralistic.net/2024/02/05/cyber-dunning-kruger/#swiss-cheese-security
But though rich people can fall for scams the same way you and I do, the way those scams play out is very different when the marks are wealthy. As Keynes had it, "The market can remain irrational longer than you can remain solvent." When the marks are rich (or worse, super-rich), they can be played for much longer before they go bust, creating the appearance of solidity.
Noted Keynesian John Kenneth Galbraith had his own thoughts on this. Galbraith coined the term "bezzle" to describe "the magic interval when a confidence trickster knows he has the money he has appropriated but the victim does not yet understand that he has lost it." In that magic interval, everyone feels better off: the mark thinks he's up, and the con artist knows he's up.
Rich marks have looong bezzles. Empirically incorrect ideas grounded in the most outrageous superstition and junk science can take over whole sections of your life, simply because a rich person – or rich people – are convinced that they're good for you.
Take "scientific management." In the early 20th century, the con artist Frederick Taylor convinced rich industrialists that he could increase their workers' productivity through a kind of caliper-and-stopwatch driven choreographry:
https://pluralistic.net/2022/08/21/great-taylors-ghost/#solidarity-or-bust
Taylor and his army of labcoated sadists perched at the elbows of factory workers (whom Taylor referred to as "stupid," "mentally sluggish," and as "an ox") and scripted their motions to a fare-the-well, transforming their work into a kind of kabuki of obedience. They weren't more efficient, but they looked smart, like obedient robots, and this made their bosses happy. The bosses shelled out fortunes for Taylor's services, even though the workers who followed his prescriptions were less efficient and generated fewer profits. Bosses were so dazzled by the spectacle of a factory floor of crisply moving people interfacing with crisply working machines that they failed to understand that they were losing money on the whole business.
To the extent they noticed that their revenues were declining after implementing Taylorism, they assumed that this was because they needed more scientific management. Taylor had a sweet con: the worse his advice performed, the more reasons their were to pay him for more advice.
Taylorism is a perfect con to run on the wealthy and powerful. It feeds into their prejudice and mistrust of their workers, and into their misplaced confidence in their own ability to understand their workers' jobs better than their workers do. There's always a long dollar to be made playing the "scientific management" con.
Today, there's an app for that. "Bossware" is a class of technology that monitors and disciplines workers, and it was supercharged by the pandemic and the rise of work-from-home. Combine bossware with work-from-home and your boss gets to control your life even when in your own place – "work from home" becomes "live at work":
https://pluralistic.net/2021/02/24/gwb-rumsfeld-monsters/#bossware
Gig workers are at the white-hot center of bossware. Gig work promises "be your own boss," but bossware puts a Taylorist caliper wielder into your phone, monitoring and disciplining you as you drive your wn car around delivering parcels or picking up passengers.
In automation terms, a worker hitched to an app this way is a "reverse centaur." Automation theorists call a human augmented by a machine a "centaur" – a human head supported by a machine's tireless and strong body. A "reverse centaur" is a machine augmented by a human – like the Amazon delivery driver whose app goads them to make inhuman delivery quotas while punishing them for looking in the "wrong" direction or even singing along with the radio:
https://pluralistic.net/2024/08/02/despotism-on-demand/#virtual-whips
Bossware pre-dates the current AI bubble, but AI mania has supercharged it. AI pumpers insist that AI can do things it positively cannot do – rolling out an "autonomous robot" that turns out to be a guy in a robot suit, say – and rich people are groomed to buy the services of "AI-powered" bossware:
https://pluralistic.net/2024/01/29/pay-no-attention/#to-the-little-man-behind-the-curtain
For an AI scammer like Elon Musk or Sam Altman, the fact that an AI can't do your job is irrelevant. From a business perspective, the only thing that matters is whether a salesperson can convince your boss that an AI can do your job – whether or not that's true:
https://pluralistic.net/2024/07/25/accountability-sinks/#work-harder-not-smarter
The fact that AI can't do your job, but that your boss can be convinced to fire you and replace you with the AI that can't do your job, is the central fact of the 21st century labor market. AI has created a world of "algorithmic management" where humans are demoted to reverse centaurs, monitored and bossed about by an app.
The techbro's overwhelming conceit is that nothing is a crime, so long as you do it with an app. Just as fintech is designed to be a bank that's exempt from banking regulations, the gig economy is meant to be a workplace that's exempt from labor law. But this wheeze is transparent, and easily pierced by enforcers, so long as those enforcers want to do their jobs. One such enforcer is Alvaro Bedoya, an FTC commissioner with a keen interest in antitrust's relationship to labor protection.
Bedoya understands that antitrust has a checkered history when it comes to labor. As he's written, the history of antitrust is a series of incidents in which Congress revised the law to make it clear that forming a union was not the same thing as forming a cartel, only to be ignored by boss-friendly judges:
https://pluralistic.net/2023/04/14/aiming-at-dollars/#not-men
Bedoya is no mere historian. He's an FTC Commissioner, one of the most powerful regulators in the world, and he's profoundly interested in using that power to help workers, especially gig workers, whose misery starts with systemic, wide-scale misclassification as contractors:
https://pluralistic.net/2024/02/02/upward-redistribution/
In a new speech to NYU's Wagner School of Public Service, Bedoya argues that the FTC's existing authority allows it to crack down on algorithmic management – that is, algorithmic management is illegal, even if you break the law with an app:
https://www.ftc.gov/system/files/ftc_gov/pdf/bedoya-remarks-unfairness-in-workplace-surveillance-and-automated-management.pdf
Bedoya starts with a delightful analogy to The Hawtch-Hawtch, a mythical town from a Dr Seuss poem. The Hawtch-Hawtch economy is based on beekeeping, and the Hawtchers develop an overwhelming obsession with their bee's laziness, and determine to wring more work (and more honey) out of him. So they appoint a "bee-watcher." But the bee doesn't produce any more honey, which leads the Hawtchers to suspect their bee-watcher might be sleeping on the job, so they hire a bee-watcher-watcher. When that doesn't work, they hire a bee-watcher-watcher-watcher, and so on and on.
For gig workers, it's bee-watchers all the way down. Call center workers are subjected to "AI" video monitoring, and "AI" voice monitoring that purports to measure their empathy. Another AI times their calls. Two more AIs analyze the "sentiment" of the calls and the success of workers in meeting arbitrary metrics. On average, a call-center worker is subjected to five forms of bossware, which stand at their shoulders, marking them down and brooking no debate.
For example, when an experienced call center operator fielded a call from a customer with a flooded house who wanted to know why no one from her boss's repair plan system had come out to address the flooding, the operator was punished by the AI for failing to try to sell the customer a repair plan. There was no way for the operator to protest that the customer had a repair plan already, and had called to complain about it.
Workers report being sickened by this kind of surveillance, literally – stressed to the point of nausea and insomnia. Ironically, one of the most pervasive sources of automation-driven sickness are the "AI wellness" apps that bosses are sold by AI hucksters:
https://pluralistic.net/2024/03/15/wellness-taylorism/#sick-of-spying
The FTC has broad authority to block "unfair trade practices," and Bedoya builds the case that this is an unfair trade practice. Proving an unfair trade practice is a three-part test: a practice is unfair if it causes "substantial injury," can't be "reasonably avoided," and isn't outweighed by a "countervailing benefit." In his speech, Bedoya makes the case that algorithmic management satisfies all three steps and is thus illegal.
On the question of "substantial injury," Bedoya describes the workday of warehouse workers working for ecommerce sites. He describes one worker who is monitored by an AI that requires him to pick and drop an object off a moving belt every 10 seconds, for ten hours per day. The worker's performance is tracked by a leaderboard, and supervisors punish and scold workers who don't make quota, and the algorithm auto-fires if you fail to meet it.
Under those conditions, it was only a matter of time until the worker experienced injuries to two of his discs and was permanently disabled, with the company being found 100% responsible for this injury. OSHA found a "direct connection" between the algorithm and the injury. No wonder warehouses sport vending machines that sell painkillers rather than sodas. It's clear that algorithmic management leads to "substantial injury."
What about "reasonably avoidable?" Can workers avoid the harms of algorithmic management? Bedoya describes the experience of NYC rideshare drivers who attended a round-table with him. The drivers describe logging tens of thousands of successful rides for the apps they work for, on promise of "being their own boss." But then the apps start randomly suspending them, telling them they aren't eligible to book a ride for hours at a time, sending them across town to serve an underserved area and still suspending them. Drivers who stop for coffee or a pee are locked out of the apps for hours as punishment, and so drive 12-hour shifts without a single break, in hopes of pleasing the inscrutable, high-handed app.
All this, as drivers' pay is falling and their credit card debts are mounting. No one will explain to drivers how their pay is determined, though the legal scholar Veena Dubal's work on "algorithmic wage discrimination" reveals that rideshare apps temporarily increase the pay of drivers who refuse rides, only to lower it again once they're back behind the wheel:
https://pluralistic.net/2023/04/12/algorithmic-wage-discrimination/#fishers-of-men
This is like the pit boss who gives a losing gambler some freebies to lure them back to the table, over and over, until they're broke. No wonder they call this a "casino mechanic." There's only two major rideshare apps, and they both use the same high-handed tactics. For Bedoya, this satisfies the second test for an "unfair practice" – it can't be reasonably avoided. If you drive rideshare, you're trapped by the harmful conduct.
The final prong of the "unfair practice" test is whether the conduct has "countervailing value" that makes up for this harm.
To address this, Bedoya goes back to the call center, where operators' performance is assessed by "Speech Emotion Recognition" algorithms, a psuedoscientific hoax that purports to be able to determine your emotions from your voice. These SERs don't work – for example, they might interpret a customer's laughter as anger. But they fail differently for different kinds of workers: workers with accents – from the American south, or the Philippines – attract more disapprobation from the AI. Half of all call center workers are monitored by SERs, and a quarter of workers have SERs scoring them "constantly."
Bossware AIs also produce transcripts of these workers' calls, but workers with accents find them "riddled with errors." These are consequential errors, since their bosses assess their performance based on the transcripts, and yet another AI produces automated work scores based on them.
In other words, algorithmic management is a procession of bee-watchers, bee-watcher-watchers, and bee-watcher-watcher-watchers, stretching to infinity. It's junk science. It's not producing better call center workers. It's producing arbitrary punishments, often against the best workers in the call center.
There is no "countervailing benefit" to offset the unavoidable substantial injury of life under algorithmic management. In other words, algorithmic management fails all three prongs of the "unfair practice" test, and it's illegal.
What should we do about it? Bedoya builds the case for the FTC acting on workers' behalf under its "unfair practice" authority, but he also points out that the lack of worker privacy is at the root of this hellscape of algorithmic management.
He's right. The last major update Congress made to US privacy law was in 1988, when they banned video-store clerks from telling the newspapers which VHS cassettes you rented. The US is long overdue for a new privacy regime, and workers under algorithmic management are part of a broad coalition that's closer than ever to making that happen:
https://pluralistic.net/2023/12/06/privacy-first/#but-not-just-privacy
Workers should have the right to know which of their data is being collected, who it's being shared by, and how it's being used. We all should have that right. That's what the actors' strike was partly motivated by: actors who were being ordered to wear mocap suits to produce data that could be used to produce a digital double of them, "training their replacement," but the replacement was a deepfake.
With a Trump administration on the horizon, the future of the FTC is in doubt. But the coalition for a new privacy law includes many of Trumpland's most powerful blocs – like Jan 6 rioters whose location was swept up by Google and handed over to the FBI. A strong privacy law would protect their Fourth Amendment rights – but also the rights of BLM protesters who experienced this far more often, and with far worse consequences, than the insurrectionists.
The "we do it with an app, so it's not illegal" ruse is wearing thinner by the day. When you have a boss for an app, your real boss gets an accountability sink, a convenient scapegoat that can be blamed for your misery.
The fact that this makes you worse at your job, that it loses your boss money, is no guarantee that you will be spared. Rich people make great marks, and they can remain irrational longer than you can remain solvent. Markets won't solve this one – but worker power can.
Image: Cryteria (modified) https://commons.wikimedia.org/wiki/File:HAL9000.svg
CC BY 3.0 https://creativecommons.org/licenses/by/3.0/deed.en
#pluralistic#alvaro bedoya#ftc#workers#algorithmic management#veena dubal#bossware#taylorism#neotaylorism#snake oil#dr seuss#ai#sentiment analysis#digital phrenology#speech emotion recognition#shitty technology adoption curve
2K notes
·
View notes
Text
AI-Driven Sentiment Analysis Solutions by Marketways Arabia
Discover the power of AI driven sentiment analysis with Marketways Arabia. As a leading provider of cutting-edge AI solutions, we specialize in transforming raw customer feedback into actionable insights. By leveraging advanced machine learning algorithms and natural language processing, our sentiment analysis tools enable businesses to understand emotions, opinions, and trends expressed in text data.
Marketways Arabia’s AI-driven sentiment analysis can help you monitor customer satisfaction, improve brand reputation, and drive strategic decision-making. From analyzing social media conversations to processing customer reviews, we ensure you stay ahead in a competitive market by unlocking valuable insights hidden within data.
Our innovative solutions are designed to cater to businesses of all sizes and industries, empowering them to create more personalized and impactful customer experiences. With a focus on accuracy, speed, and scalability, Marketways Arabia delivers unmatched results in sentiment analysis.Transform the way you understand and engage with your audience. Choose Marketways Arabia to harness the full potential of AI-driven sentiment analysis and take your business to new heights.
0 notes
Text
Wawasan Pelanggan Berbasis AI untuk Pemasaran yang Tepat Sasaran
Di era digital yang semakin kompleks, memahami pelanggan menjadi kunci keberhasilan pemasaran. Perusahaan yang dapat mengidentifikasi kebutuhan, preferensi, dan perilaku pelanggan memiliki keunggulan kompetitif yang signifikan. Namun, dengan jumlah data yang terus bertambah, menganalisis informasi secara manual menjadi tantangan besar. Di sinilah kecerdasan buatan (AI) memainkan peran penting. AI…
#AI in small businesses#AI marketing#AI-driven campaigns#customer insights#customer loyalty#data ethics#future of AI marketing#hyper-personalization#machine learning tools#marketing automation#omnichannel marketing#personalized marketing#predictive analytics#ROI improvement#sentiment analysis
0 notes
Text
Turn feedback into action with StratosIQ! Discover insights that matter—like this clothing retailer who improved customer satisfaction by addressing sizing issues. Make smarter decisions, faster.
#business#ai#ai tools#ai generated#ai insights#sentiment analysis#competitor analysis#ai platform#market insights
0 notes
Text
ConvertML: AI-Generated Insights for Streamlined Decision-Making
Discover ConvertML's innovative AI-generated insights that streamline decision-making. Automate complex analytics workflows to gain actionable customer insights and recommendations swiftly. Save time and resources while making informed decisions effortlessly with ConvertML's advanced capabilities.
0 notes
Text
Demystifying Encoder and Decoder Components in Transformer Models

A recent report says that 52.4% of businesses are already embracing Generative AI to make their work life easier while cutting down costs. In case you’re out of the marathon, it’s time for your organization to deepen the understanding of Generative AI and Large Language Models (LLMs). You can start exploring the various forms of GenAI, beginning with the encoder and decoder components of transformer models emerging as one of the leading innovations.
Wondering what exactly are transformer models?
A transformer model is a type of neural network that understands the meaning of words by looking at how they relate to each other in a sentence.
For example: In the sentence "The cat sat on the mat," the model recognizes that "cat" and "sat" are connected, helping it understand that the sentence is about a cat sitting.
Such models have opened new possibilities, enabling AI-driven innovations as it can help with tasks like -

Onwards toward the roles of each component!
Role of Encoder in Transformer Models
Encoder in transformer models plays an important role in processing the input sequence and generating a response that captures its meaning and context.
This is how it works:
1. Input Embedding: The process begins by feeding the input sequence, usually made up of embeddings, into the encoder. These embeddings represent the meaning of each word in a multi-dimensional space.
2. Positional Encoding: Since transformer models do not have built-in sequential information, positional encoding is added to the input embeddings. This helps the model understand the position of each word within the sequence.
3. Self-Attention Mechanism: The heart of the encoder is the self-attention mechanism, which assesses the importance of each word in relation to others in the sequence. Each word considers all other words, dynamically calculating attention weights based on their relationships.
4. Multi-Head Attention: To capture various aspects of the input, self-attention is divided into multiple heads. Each head learns different relationships among the words, enabling the model to identify more intricate patterns.
5. Feed-Forward Neural Network: After the self-attention mechanism processes the input, the output is then sent through a feed-forward neural network.
6. Layer Normalization and Residual Connections: To improve training efficiency and mitigate issues like vanishing gradients, layer normalization and residual connections are applied after each sub-layer in the encoder.
Next, get to know how decoders work!
Role of Decoder in Transformer Models The primary function of the decoder is to create the output sequence based on the representation provided by the encoder.
Here’s how it works:
1. Input Embedding and Positional Encoding: Here, first the target sequence is embedded, and positional encoding is added to indicate word order.
2. Masked Self-Attention: The decoder employs masked self-attention, allowing each word to focus only on the previous words. This prevents future information from influencing outputs during model training.
3. Encoder-Decoder Attention: The decoder then attends to the encoder's output, helping it focus on relevant parts of the input when generating words.
4. Multi-Head Attention and Feed-Forward Networks: Like the encoder, the decoder uses multiple self-attention heads and feed-forward networks for processing.
5. Layer Normalization and Residual Connections: These techniques are applied after each sub-layer to improve training and performance.
6. Output Projection: The decoder's final output is projected into a probability distribution over the vocabulary, selecting the word with the highest probability as the next output.
So, the integration of these components in the Transformer architecture allows efficient handling of input sequences and the creation of output sequences. This versatility makes it exceptionally suited for a wide range of tasks in natural language processing and other GenAI applications.
Wish to learn more about LLMs and its perks for your business? Reach us at Nitor Infotech.
#transformers#Sentiment Analysis#nlp#Deep Learning#generative ai#deep learning#large language models#ai models#gen ai#artificial intelligence models#model artificial intelligence#ai deep learning#llm models#transformers models#blog#software development#software services
0 notes
Text
O.J. Simpson’s Twists of Fate: From Cancer Battles to Infamous Trials
In May 2023, O.J. Simpson shared a video on X (formerly known as Twitter), revealing that he had recently “caught cancer” and undergone chemotherapy. Although he didn’t specify the type of cancer, he expressed optimism about beating it. Fast forward to February 2024, when a Las Vegas television station reported that Simpson was once again receiving treatment for an unspecified cancer. In a…
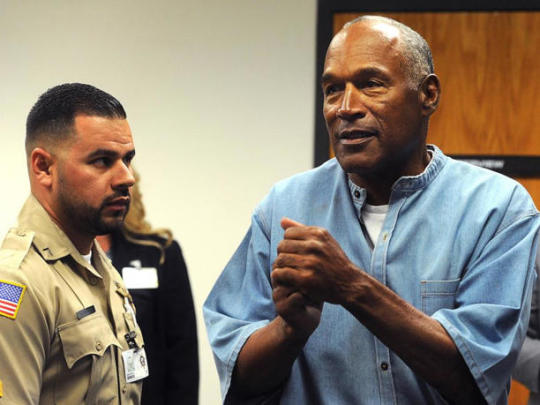
View On WordPress
#AI News#biometric identification#case prediction#crime prevention#document analysis#ethical AI#facial recognition#forensic analysis#kaelin#kato#legal research#machine learning#News#nicole simpson#O.J. Simpson#predictive policing#ron goldman#sentiment analysis
0 notes
Text
Unveiling the Power of AI for Customer Sentiment Analysis
Introduction
In the rapidly evolving landscape of business, understanding customer sentiment is paramount for success. The rise of Artificial Intelligence (AI) has revolutionized various aspects of business operations, and one of the most significant applications is in sentiment analysis. This article explores how AI is harnessed for customer sentiment analysis, the advancements in AI-based sentiment analysis, and the pivotal role played by AI development services in this domain.
The Significance of Customer Sentiment Analysis
Customer sentiment analysis involves gauging the feelings, opinions, and attitudes of customers towards a product, service, or brand. Traditionally, businesses relied on surveys, feedback forms, and reviews to gather this information. However, with the advent of AI, the process has become more efficient, accurate, and timely.
Understanding customer sentiment is crucial for businesses aiming to enhance customer satisfaction, improve products or services, and make informed decisions. AI facilitates the automatic analysis of vast amounts of textual data, enabling businesses to gain insights into customer sentiments on a real-time basis.
AI-Based Sentiment Analysis: An Overview
1. Natural Language Processing (NLP)
AI utilizes Natural Language Processing (NLP) algorithms to decipher and understand human language. NLP enables machines to comprehend the nuances of language, including context, tone, and sentiment. Through sentiment analysis AI models can classify text data into categories like positive, negative, or neutral, providing a comprehensive overview of customer opinions.
2. Machine Learning Models
Machine Learning (ML) plays a pivotal role in training sentiment analysis models. These models learn from historical data, becoming adept at recognizing patterns and sentiments. As more data is fed into the system, the accuracy of predictions improves. ML-based sentiment analysis adapts to evolving language trends and is capable of handling diverse datasets.
3. Deep Learning
Deep Learning, a subset of ML, involves neural networks with multiple layers to simulate human decision-making. In sentiment analysis, deep learning models can capture intricate relationships within data, enhancing the accuracy of sentiment classification. This allows for a more nuanced understanding of customer sentiments, going beyond simple positive or negative categorizations.
AI Development Services in Sentiment Analysis
1. Customized Solutions
AI development services offer businesses tailored solutions for sentiment analysis based on their unique requirements. These services involve developing AI models specific to the industry, brand, or product, ensuring accurate and relevant results. Customization allows businesses to focus on the aspects of sentiment that matter most to their operations.
2. Integration with Existing Systems
AI development services seamlessly integrate sentiment analysis capabilities into existing business systems. This integration facilitates a smooth transition to AI-powered sentiment analysis, minimizing disruptions to daily operations. Businesses can leverage their existing data repositories to enhance the efficiency and accuracy of sentiment analysis.
3. Continuous Improvement
AI development services not only build AI models but also ensure continuous improvement. Regular updates and adaptations are essential to keep sentiment analysis models relevant in a dynamic market. AI developers refine algorithms based on feedback and emerging trends, guaranteeing that businesses stay at the forefront of sentiment analysis capabilities.
Conclusion
The utilization of AI for customer sentiment analysis has transformed the way businesses interpret and respond to customer feedback. From NLP and machine learning to deep learning, AI brings a range of sophisticated tools to decipher the intricate language of customer sentiments. AI development services further empower businesses by providing customized solutions, seamless integrations, and continuous improvement.
As businesses strive to stay competitive in an ever-evolving market, harnessing the power of AI for sentiment analysis is not just a choice but a necessity. The insights derived from AI-driven sentiment analysis empower businesses to make data-driven decisions, enhance customer satisfaction, and ultimately thrive in the dynamic landscape of modern commerce.
1 note
·
View note
Text
Turn Feedback into Fortune: How to Leverage Sentiment Analysis for Growth

https://www.odioiq.com/turn-feedback-into-fortune-how-to-leverage-sentiment-analysis-for-growth/
0 notes
Text
Use no-code machine learning to derive insights from product reviews using Amazon SageMaker Canvas sentiment analysis and text analysis models
Exciting news! 🎉 Want to use machine learning to gain insights from product reviews without any coding or ML expertise? Check out this step-by-step guide on how to leverage Amazon SageMaker Canvas sentiment analysis and text analysis models. 🤩 According to Gartner, 85% of software buyers trust online reviews as much as personal recommendations. With machine learning, you can analyze large volumes of customer reviews and uncover valuable insights to improve your products and services. 💡 Amazon SageMaker Canvas offers ready-to-use AI models for sentiment analysis and text analysis of product reviews, eliminating the need for ML specialists. In this blog post, we provide sample datasets and walk you through the process of leveraging these models. 📈 Elevate your company with AI and stay competitive. Don't miss out on this opportunity to derive insights from product reviews. Check out the blog post here: [Link](https://ift.tt/tRcfO83) Stay updated on AI and ML trends by following Itinai on Twitter @itinaicom. And for a free consultation, join our AI Lab in Telegram @aiscrumbot. 🚀 #AI #MachineLearning #AmazonSageMakerCanvas #ProductReviews #Insights List of Useful Links: AI Scrum Bot - ask about AI scrum and agile Our Telegram @itinai Twitter - @itinaicom
#itinai.com#AI#News#Use no-code machine learning to derive insights from product reviews using Amazon SageMaker Canvas sentiment analysis and text analysis mod#AI News#AI tools#AWS Machine Learning Blog#Gavin Satur#Innovation#LLM#t.me/itinai Use no-code machine learning to derive insights from product reviews using Amazon SageMaker Canvas sentiment analysis and text
0 notes
Text
Transforming Noisy Investor Reactions into Actionable Financial Insights with ChatGPT
Disclaimer : The information in this post is intended for informational purposes only and should not be considered investment advice. Prior to making any investment decisions, conduct your own research and consult with a financial advisor. (Images generated by author with DALL·E / Bing Image Creator) In the finance world, earnings season is always a busy time for investors. Once those quarterly…
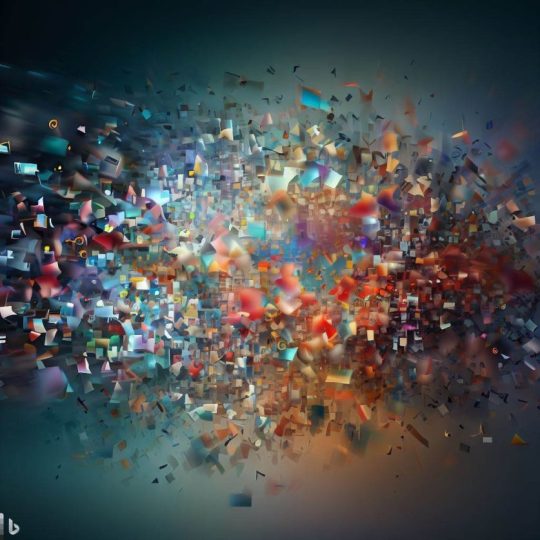
View On WordPress
#AI in finance#AI-driven investment insights#ChatGPT insights#Financial intelligence#Financial market commentary#Investment decision-making#Investor sentiment#Investor sentiment analysis#Investor sentiment trends#Market analysis with AI#Share buyback
0 notes
Text
Unlock Business Insights with AI Driven Sentiment Analysis from Market Ways Arabia
In the digital age, understanding customer sentiment is more important than ever. With AI driven sentiment analysis, businesses can gain valuable insights into consumer emotions, opinions, and preferences, which can significantly enhance decision-making and customer engagement strategies. Market Ways Arabia offers cutting-edge AI driven sentiment analysis solutions designed to help organizations stay ahead of the curve.
Market Ways Arabia’s AI-powered tools analyze vast amounts of data from social media, reviews, surveys, and other online sources to determine the sentiment behind customer interactions. By leveraging natural language processing (NLP) and machine learning technologies, our sentiment analysis models can accurately classify text data into categories such as positive, negative, or neutral. This enables businesses to understand their customers better and tailor their strategies accordingly. Whether you're in retail, hospitality, healthcare, or any other sector, AI driven sentiment analysis can be used to improve customer service, brand perception, and marketing effectiveness. By identifying trends in customer feedback, businesses can quickly respond to issues, capitalize on positive sentiment, and make informed decisions that align with customer expectations.
At Market Ways Arabia, we believe that harnessing the power of AI driven sentiment analysis is a game-changer for businesses looking to drive growth and innovation. Our solutions are tailored to meet the specific needs of your business, ensuring that you get the most out of your data and can take proactive measures to enhance customer satisfaction and loyalty.
Transform your customer engagement and business strategies today with Market Ways Arabia AI driven sentiment analysis. Contact us to learn more and start leveraging the power of AI for actionable insights that can propel your business forward.
0 notes
Text
LLMs and their impact: using language models to advance data science
In the ever-evolving landscape of data science, language models have emerged as powerful tools that hold the potential to revolutionize how we process and interpret vast amounts of textual data. Among these language models, Large Language Models (LLMs) have emerged as the forefront of cutting-edge technology, enabling us to tackle complex natural language processing tasks with unprecedented accuracy and efficiency. In this blog, we will explore how LLMs have impacted data science and how they continue to shape the future of the field.
What are Large Language Models (LLMs)?
Large Language Models, or LLMs, are advanced artificial intelligence models that can understand, generate, and manipulate human language. These models are typically trained on massive datasets, containing billions of words, enabling them to learn intricate patterns and structures of language. They employ deep learning techniques, such as the Transformer architecture, to process text and make predictions with exceptional proficiency.
Applications of LLMs in Data Science
Natural Language Understanding (NLU): LLMs excel in NLU tasks, such as sentiment analysis, named entity recognition, and text classification. With their ability to comprehend context, they can infer the intended meaning of a sentence or document more accurately than traditional methods.
Language Generation: LLMs can generate human-like text, including articles, stories, and poetry. This capability finds application in content creation, chatbots, and virtual assistants.
Machine Translation: LLMs have significantly improved machine translation systems, allowing for more accurate and contextually appropriate translations across multiple languages.
Text Summarization: With LLMs, data scientists can develop robust automatic summarization algorithms that extract key information from lengthy documents, improving efficiency and comprehension.
Question-Answering Systems: LLMs enable the development of advanced question-answering systems that can comprehend complex queries and provide accurate responses.
Enhancing Data Science with LLMs
Pre-trained Models: LLMs are often pre-trained on vast datasets, making them a valuable resource for data scientists. Pre-trained models can be fine-tuned on specific tasks, saving time and computational resources.
Improved Feature Extraction: LLMs can extract high-level features from text data, offering more informative representations for downstream tasks, such as sentiment analysis or image captioning.
Data Augmentation: Data augmentation techniques using LLMs can generate synthetic data to enhance the robustness and generalization of machine learning models.
Domain-Specific Applications: LLMs can be fine-tuned on domain-specific datasets, making them adaptable to specialized industries, such as healthcare, finance, and law.
Challenges and Ethical Considerations
While LLMs have significantly advanced data science, they are not without challenges and ethical implications:
Data Bias: Pre-training on large datasets can lead to inherent biases present in the data, potentially perpetuating societal prejudices.
Overfitting: LLMs may overfit to the training data, leading to unrealistic outputs or incorrect predictions.
Model Size and Resource Requirements: Large LLMs demand substantial computational power and memory, making them inaccessible to many researchers.
Misinformation and Fake Content: Language models can inadvertently generate false information, which can be exploited to spread misinformation or create fake content.
Conclusion
Language models, particularly Large Language Models (LLMs), have revolutionized data science by providing powerful tools to process, understand, and generate human language. Their applications are diverse and continually expanding, with promising opportunities to enhance various NLP tasks and other data science applications. However, to fully realize the potential of LLMs, addressing challenges related to bias, overfitting, and ethical considerations is essential. As we move forward, it is crucial to use these models responsibly and transparently, ensuring that their impact on data science and society at large remains positive and transformative.
#Language models#LLMs (Large Language Models)#Natural language processing#Data science#Machine learning#Text generation#AI applications#Text analysis#Data processing#Information extraction#Knowledge representation#Semantic understanding#Text classification#Sentiment analysis#Data-driven insights#Predictive modeling#Text summarization#Feature extraction
0 notes
Text
In this tutorial, we will explore how to perform sentiment analysis using Python with three popular libraries — NLTK, TextBlob, and VADER.
#machine learning#data science#python#sentiment analysis#natural language processing#NLTK#TextBlob#VADER#tutorial#medium#medium writers#artificial intelligence#ai#data analysis#data scientist#data analytics#computer science
1 note
·
View note
Text
ConvertML - Leading Predictive Customer Insights Platform for Advanced Analytics
ConvertML empowers businesses with seamless data integration and advanced analytics. Our platform delivers predictive customer insights, combining qualitative and quantitative data for actionable strategies. With an intuitive dashboard and AI-driven tools, ConvertML transforms raw data into powerful, real-time business decisions.
0 notes