#ai sentiment analysis for customer feedback
Explore tagged Tumblr posts
Text
AI-Based Sentiment Analysis for Customer Feedback
0 notes
Text
10 Ways Artificial Intelligence is Transforming the Market Research Industry

Artificial intelligence (AI) is revolutionizing various industries, and the market research solutions sector is no exception. By harnessing the power of AI, companies can gain deeper insights into consumer behavior, optimize their strategies, and make data-driven decisions with greater precision. In this article, we’ll explore 10 transformative ways AI is reshaping the market research industry and how Gen AI development solutions are at the forefront of this evolution.
Understanding What Market Research Necessitates
Market research is essential for businesses seeking to understand consumer needs, preferences, and market dynamics. It involves collecting and analyzing data from various sources to inform strategic decisions. Effective market research solutions help brands grow by uncovering valuable insights into consumer behavior, market trends, and competitive positioning.
10 Ways Artificial Intelligence is Transforming the Market Research Industry
1. Context-Aware Virtual Chatbots
The use of context-aware virtual chatbots has become increasingly popular in market research. These advanced AI-driven tools engage customers in meaningful conversations, gather qualitative feedback, and provide insights into consumer preferences. By automating interactions and analyzing responses, businesses can enhance their market research efforts and increase survey participation.
2. Real-Time Behavior Monitoring
AI-powered tools enable real-time behavior monitoring, offering valuable insights into consumer interactions and product usage patterns. This real-time data helps businesses identify trends, optimize user experiences, and improve customer satisfaction.
3. Unstructured Data Processing
Processing unstructured data from sources like social media, news articles, and multimedia content is a critical aspect of market research. AI-driven solutions excel at unstructured data processing, turning diverse information into actionable insights for market research strategies.
4. Emotion-Driven Profiling
AI technologies enable emotion-driven profiling by analyzing sentiment and emotional responses from customer feedback. This approach helps businesses understand emotional drivers behind consumer behavior and refine their marketing strategies.
5. Predictive Analytics
With predictive analytics, AI helps market researchers forecast future trends, customer behaviors, and market shifts. These insights empower businesses to make informed decisions and stay ahead of the competition.
6. Customer Segmentation
AI enhances customer segmentation by analyzing data to group consumers based on shared characteristics. This segmentation enables businesses to create targeted marketing campaigns and improve customer engagement.
7. Cost-Time Analysis and Optimization
AI solutions streamline market research processes, reducing both cost and time. Through automation and advanced analytics, businesses can conduct comprehensive market studies efficiently, making cost-time optimization accessible to companies of all sizes.
8. Extensive Automation
AI facilitates extensive automation in market research tasks, allowing for continuous data collection and analysis. This automation frees up resources and enables employees to focus on strategic decision-making.
9. Product Performance Simulation
AI-driven product performance simulations allow businesses to test and refine product concepts in virtual environments. These simulations provide valuable insights into product durability, user experience, and potential improvements.
10. Risk Monitoring and Resolution
AI-powered risk monitoring tools help businesses identify potential issues in marketing strategies and product developments. By analyzing data for risk monitoring, businesses can implement proactive measures and ensure resilience against market fluctuations.
Conclusion
AI is a game-changer for the market research industry, offering innovative solutions for data analysis, customer engagement, and strategic planning. By exploring advanced market research solutions and leveraging Gen AI development solutions, businesses can unlock new opportunities and stay competitive in a rapidly evolving market landscape. Embracing these technologies will be crucial for market research professionals looking to drive success in the future.
4 notes
·
View notes
Text
SEMANTIC TREE AND AI TECHNOLOGIES
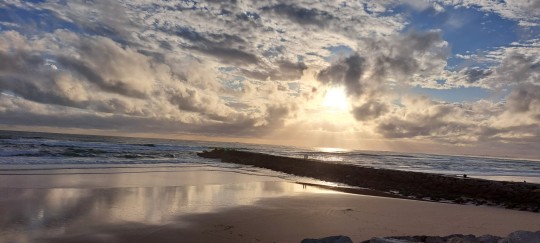
Semantic Tree learning and AI technologies can be combined to solve problems by leveraging the power of natural language processing and machine learning.
Semantic trees are a knowledge representation technique that organizes information in a hierarchical, tree-like structure.
Each node in the tree represents a concept or entity, and the connections between nodes represent the relationships between those concepts.
This structure allows for the representation of complex, interconnected knowledge in a way that can be easily navigated and reasoned about.
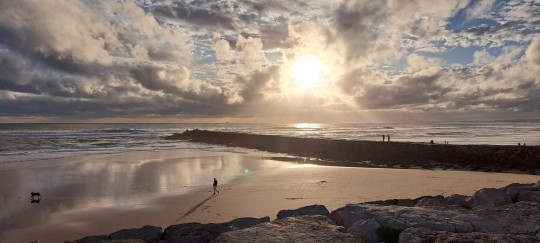


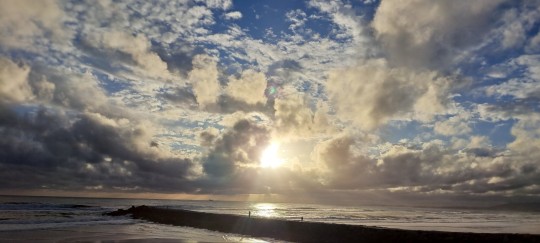

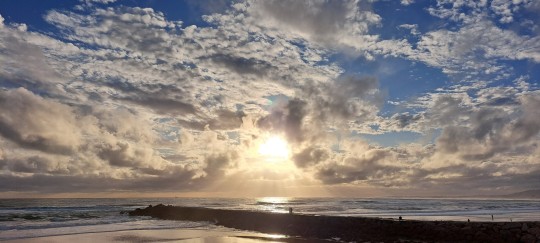
CONCEPTS
Semantic Tree: A structured representation where nodes correspond to concepts and edges denote relationships (e.g., hyponyms, hyponyms, synonyms).
Meaning: Understanding the context, nuances, and associations related to words or concepts.
Natural Language Understanding (NLU): AI techniques for comprehending and interpreting human language.
First Principles: Fundamental building blocks or core concepts in a domain.
AI (Artificial Intelligence): AI refers to the development of computer systems that can perform tasks that typically require human intelligence. AI technologies include machine learning, natural language processing, computer vision, and more. These technologies enable computers to understand reason, learn, and make decisions.
Natural Language Processing (NLP): NLP is a branch of AI that focuses on the interaction between computers and human language. It involves the analysis and understanding of natural language text or speech by computers. NLP techniques are used to process, interpret, and generate human languages.
Machine Learning (ML): Machine Learning is a subset of AI that enables computers to learn and improve from experience without being explicitly programmed. ML algorithms can analyze data, identify patterns, and make predictions or decisions based on the learned patterns.
Deep Learning: A subset of machine learning that uses neural networks with multiple layers to learn complex patterns.
EXAMPLES OF APPLYING SEMANTIC TREE LEARNING WITH AI.
1. Text Classification: Semantic Tree learning can be combined with AI to solve text classification problems. By training a machine learning model on labeled data, the model can learn to classify text into different categories or labels. For example, a customer support system can use semantic tree learning to automatically categorize customer queries into different topics, such as billing, technical issues, or product inquiries.
2. Sentiment Analysis: Semantic Tree learning can be used with AI to perform sentiment analysis on text data. Sentiment analysis aims to determine the sentiment or emotion expressed in a piece of text, such as positive, negative, or neutral. By analyzing the semantic structure of the text using Semantic Tree learning techniques, machine learning models can classify the sentiment of customer reviews, social media posts, or feedback.
3. Question Answering: Semantic Tree learning combined with AI can be used for question answering systems. By understanding the semantic structure of questions and the context of the information being asked, machine learning models can provide accurate and relevant answers. For example, a Chabot can use Semantic Tree learning to understand user queries and provide appropriate responses based on the analyzed semantic structure.
4. Information Extraction: Semantic Tree learning can be applied with AI to extract structured information from unstructured text data. By analyzing the semantic relationships between entities and concepts in the text, machine learning models can identify and extract specific information. For example, an AI system can extract key information like names, dates, locations, or events from news articles or research papers.
Python Snippet Codes for Semantic Tree Learning with AI
Here are four small Python code snippets that demonstrate how to apply Semantic Tree learning with AI using popular libraries:
1. Text Classification with scikit-learn:
```python
from sklearn.feature_extraction.text import TfidfVectorizer
from sklearn.linear_model import LogisticRegression
# Training data
texts = ['This is a positive review', 'This is a negative review', 'This is a neutral review']
labels = ['positive', 'negative', 'neutral']
# Vectorize the text data
vectorizer = TfidfVectorizer()
X = vectorizer.fit_transform(texts)
# Train a logistic regression classifier
classifier = LogisticRegression()
classifier.fit(X, labels)
# Predict the label for a new text
new_text = 'This is a positive sentiment'
new_text_vectorized = vectorizer.transform([new_text])
predicted_label = classifier.predict(new_text_vectorized)
print(predicted_label)
```
2. Sentiment Analysis with TextBlob:
```python
from textblob import TextBlob
# Analyze sentiment of a text
text = 'This is a positive sentence'
blob = TextBlob(text)
sentiment = blob.sentiment.polarity
# Classify sentiment based on polarity
if sentiment > 0:
sentiment_label = 'positive'
elif sentiment < 0:
sentiment_label = 'negative'
else:
sentiment_label = 'neutral'
print(sentiment_label)
```
3. Question Answering with Transformers:
```python
from transformers import pipeline
# Load the question answering model
qa_model = pipeline('question-answering')
# Provide context and ask a question
context = 'The Semantic Web is an extension of the World Wide Web.'
question = 'What is the Semantic Web?'
# Get the answer
answer = qa_model(question=question, context=context)
print(answer['answer'])
```
4. Information Extraction with spaCy:
```python
import spacy
# Load the English language model
nlp = spacy.load('en_core_web_sm')
# Process text and extract named entities
text = 'Apple Inc. is planning to open a new store in New York City.'
doc = nlp(text)
# Extract named entities
entities = [(ent.text, ent.label_) for ent in doc.ents]
print(entities)
```
APPLICATIONS OF SEMANTIC TREE LEARNING WITH AI
Semantic Tree learning combined with AI can be used in various domains and industries to solve problems. Here are some examples of where it can be applied:
1. Customer Support: Semantic Tree learning can be used to automatically categorize and route customer queries to the appropriate support teams, improving response times and customer satisfaction.
2. Social Media Analysis: Semantic Tree learning with AI can be applied to analyze social media posts, comments, and reviews to understand public sentiment, identify trends, and monitor brand reputation.
3. Information Retrieval: Semantic Tree learning can enhance search engines by understanding the meaning and context of user queries, providing more accurate and relevant search results.
4. Content Recommendation: By analyzing the semantic structure of user preferences and content metadata, Semantic Tree learning with AI can be used to personalize content recommendations in platforms like streaming services, news aggregators, or e-commerce websites.
Semantic Tree learning combined with AI technologies enables the understanding and analysis of text data, leading to improved problem-solving capabilities in various domains.
COMBINING SEMANTIC TREE AND AI FOR PROBLEM SOLVING
1. Semantic Reasoning: By integrating semantic trees with AI, systems can engage in more sophisticated reasoning and decision-making. The semantic tree provides a structured representation of knowledge, while AI techniques like natural language processing and knowledge representation can be used to navigate and reason about the information in the tree.
2. Explainable AI: Semantic trees can make AI systems more interpretable and explainable. The hierarchical structure of the tree can be used to trace the reasoning process and understand how the system arrived at a particular conclusion, which is important for building trust in AI-powered applications.
3. Knowledge Extraction and Representation: AI techniques like machine learning can be used to automatically construct semantic trees from unstructured data, such as text or images. This allows for the efficient extraction and representation of knowledge, which can then be used to power various problem-solving applications.
4. Hybrid Approaches: Combining semantic trees and AI can lead to hybrid approaches that leverage the strengths of both. For example, a system could use a semantic tree to represent domain knowledge and then apply AI techniques like reinforcement learning to optimize decision-making within that knowledge structure.
EXAMPLES OF APPLYING SEMANTIC TREE AND AI FOR PROBLEM SOLVING
1. Medical Diagnosis: A semantic tree could represent the relationships between symptoms, diseases, and treatments. AI techniques like natural language processing and machine learning could be used to analyze patient data, navigate the semantic tree, and provide personalized diagnosis and treatment recommendations.
2. Robotics and Autonomous Systems: Semantic trees could be used to represent the knowledge and decision-making processes of autonomous systems, such as self-driving cars or drones. AI techniques like computer vision and reinforcement learning could be used to navigate the semantic tree and make real-time decisions in dynamic environments.
3. Financial Analysis: Semantic trees could be used to model complex financial relationships and market dynamics. AI techniques like predictive analytics and natural language processing could be applied to the semantic tree to identify patterns, make forecasts, and support investment decisions.
4. Personalized Recommendation Systems: Semantic trees could be used to represent user preferences, interests, and behaviors. AI techniques like collaborative filtering and content-based recommendation could be used to navigate the semantic tree and provide personalized recommendations for products, content, or services.
PYTHON CODE SNIPPETS
1. Semantic Tree Construction using NetworkX:
```python
import networkx as nx
import matplotlib.pyplot as plt
# Create a semantic tree
G = nx.DiGraph()
G.add_node("root", label="Root")
G.add_node("concept1", label="Concept 1")
G.add_node("concept2", label="Concept 2")
G.add_node("concept3", label="Concept 3")
G.add_edge("root", "concept1")
G.add_edge("root", "concept2")
G.add_edge("concept2", "concept3")
# Visualize the semantic tree
pos = nx.spring_layout(G)
nx.draw(G, pos, with_labels=True)
plt.show()
```
2. Semantic Reasoning using PyKEEN:
```python
from pykeen.models import TransE
from pykeen.triples import TriplesFactory
# Load a knowledge graph dataset
tf = TriplesFactory.from_path("./dataset/")
# Train a TransE model on the knowledge graph
model = TransE(triples_factory=tf)
model.fit(num_epochs=100)
# Perform semantic reasoning
head = "concept1"
relation = "isRelatedTo"
tail = "concept3"
score = model.score_hrt(head, relation, tail)
print(f"The score for the triple ({head}, {relation}, {tail}) is: {score}")
```
3. Knowledge Extraction using spaCy:
```python
import spacy
# Load the spaCy model
nlp = spacy.load("en_core_web_sm")
# Extract entities and relations from text
text = "The quick brown fox jumps over the lazy dog."
doc = nlp(text)
# Visualize the extracted knowledge
from spacy import displacy
displacy.render(doc, style="ent")
```
4. Hybrid Approach using Ray:
```python
import ray
from ray.rllib.agents.ppo import PPOTrainer
from ray.rllib.env.multi_agent_env import MultiAgentEnv
from ray.rllib.models.tf.tf_modelv2 import TFModelV2
# Define a custom model that integrates a semantic tree
class SemanticTreeModel(TFModelV2):
def __init__(self, obs_space, action_space, num_outputs, model_config, name):
super().__init__(obs_space, action_space, num_outputs, model_config, name)
# Implement the integration of the semantic tree with the neural network
# Define a multi-agent environment that uses the semantic tree model
class SemanticTreeEnv(MultiAgentEnv):
def __init__(self):
self.semantic_tree = # Initialize the semantic tree
self.agents = # Define the agents
def step(self, actions):
# Implement the environment dynamics using the semantic tree
# Train the hybrid model using Ray
ray.init()
config = {
"env": SemanticTreeEnv,
"model": {
"custom_model": SemanticTreeModel,
},
}
trainer = PPOTrainer(config=config)
trainer.train()
```
APPLICATIONS
The combination of semantic trees and AI can be applied to a wide range of problem domains, including:
- Healthcare: Improving medical diagnosis, treatment planning, and drug discovery.
- Finance: Enhancing investment strategies, risk management, and fraud detection.
- Robotics and Autonomous Systems: Enabling more intelligent and adaptable decision-making in complex environments.
- Education: Personalizing learning experiences and providing intelligent tutoring systems.
- Smart Cities: Optimizing urban planning, transportation, and resource management.
- Environmental Conservation: Modeling and predicting environmental changes, and supporting sustainable decision-making.
- Chatbots and Virtual Assistants:
Use semantic trees to understand user queries and provide context-aware responses.
Apply NLU models to extract meaning from user input.
- Information Retrieval:
Build semantic search engines that understand user intent beyond keyword matching.
Combine semantic trees with vector embeddings (e.g., BERT) for better search results.
- Medical Diagnosis:
Create semantic trees for medical conditions, symptoms, and treatments.
Use AI to match patient symptoms to relevant diagnoses.
- Automated Content Generation:
Construct semantic trees for topics (e.g., climate change, finance).
Generate articles, summaries, or reports based on semantic understanding.
RDIDINI PROMPT ENGINEER
#semantic tree#ai solutions#ai-driven#ai trends#ai system#ai model#ai prompt#ml#ai predictions#llm#dl#nlp
3 notes
·
View notes
Text
The Transformative Impact of AI on Data Collection and Analysis
In the ever-evolving landscape of technology, Artificial Intelligence (AI) has emerged as a powerful force reshaping various industries. One of the areas experiencing a profound transformation is data collection and analysis. The integration of AI into these processes has not only increased efficiency but has also unlocked new possibilities for extracting meaningful insights. This article explores the ways in which AI is revolutionizing data collection and analysis, driving innovation across sectors.
Automation and Efficiency:
Traditionally, data collection involved manual efforts, consuming significant time and resources. AI has automated this process, enabling organizations to gather and process vast amounts of data at unprecedented speeds. Machine learning algorithms can sift through massive datasets, identifying patterns, trends, and anomalies far more efficiently than human counterparts. This not only reduces the burden on human labor but also allows organizations to make data-driven decisions in real-time.
Enhanced Accuracy and Predictive Analytics:
AI technologies, particularly machine learning, excel in recognizing complex patterns within data. This capability enhances the accuracy of data analysis and enables predictive analytics. By leveraging historical data, AI models can forecast future trends and outcomes, providing valuable insights for strategic decision-making. This predictive power is invaluable in industries such as finance, healthcare, and marketing, where accurate forecasting is crucial.
Natural Language Processing (NLP) for Unstructured Data:
With the proliferation of unstructured data in the form of text, audio, and video, traditional data analysis methods struggled to extract meaningful information. AI, specifically Natural Language Processing (NLP), has emerged as a game-changer in handling unstructured data. NLP enables machines to understand and interpret human language, making it possible to analyze text, sentiment, and context. This capability is particularly beneficial in customer feedback analysis, social media monitoring, and content categorization.
Internet of Things (IoT) Integration:
The rise of IoT devices has led to an explosion of data generated from various sources, ranging from smart appliances to industrial sensors. AI plays a pivotal role in processing and analyzing this data in real-time. Machine learning algorithms can identify patterns, detect anomalies, and optimize processes based on the continuous stream of IoT-generated data. This synergy between AI and IoT has significant implications for industries like manufacturing, logistics, and smart cities.
Personalization and Customer Insights:
AI-driven data analysis enables organizations to personalize user experiences by understanding individual preferences and behaviors. Through advanced recommendation systems and behavioral analysis, businesses can tailor their products and services to meet the specific needs of their customers. This level of personalization not only enhances customer satisfaction but also improves customer retention and loyalty.
Ethical Considerations and Privacy:
While AI has brought about unprecedented advancements in data collection and analysis, it also raises ethical concerns, particularly related to privacy. The vast amount of personal data being collected requires careful handling to ensure compliance with privacy regulations and to protect individuals' rights. Striking a balance between innovation and ethical considerations remains a critical challenge in the continued development of AI-powered data solutions.
Conclusion:
The integration of AI into data collection and analysis is revolutionizing the way organizations operate and make decisions. The increased efficiency, accuracy, and predictive capabilities offered by AI have far-reaching implications across various industries. As we navigate this transformative era, it is essential to address ethical considerations and privacy concerns to ensure responsible and sustainable use of AI technologies in the realm of data. The ongoing synergy between human intelligence and artificial intelligence promises a future where data-driven insights drive innovation and fuel progress.
To know more:
global market research company
developing a questionnaire for research
market research project management
2 notes
·
View notes
Text
Online Reputation Management Tool: A Must-Have for Business Success
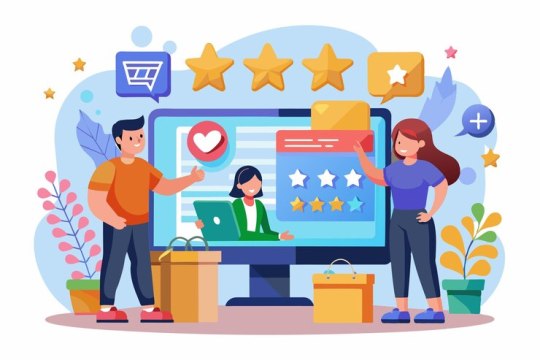
An online reputation management tool is essential for businesses that want to maintain a strong and positive brand image. In today’s digital world, online reviews and customer feedback can make or break a business. By using an effective reputation management tool, businesses can monitor their brand presence, respond to reviews, and build trust among customers. With the help of top review platforms, businesses can track customer feedback across various channels and improve their online credibility.
Why Online Reputation Management Matters
Customers rely on online reviews before making a purchase decision. A business with a good online reputation attracts more customers and builds long-term relationships. On the other hand, negative reviews can harm credibility and reduce sales. This is why businesses need an online reputation management tool to keep track of customer feedback and take necessary actions to improve their brand image.
Key Features of an Online Reputation Management Tool
Review Monitoring on Top Review Platforms Businesses receive feedback from customers across different top review platforms, including Google, Yelp, Trustpilot, and social media channels. An online reputation management tool helps track and manage reviews in one place, making it easier to monitor customer sentiment.
Automated Review Responses Engaging with customer reviews is important for brand reputation. Reputation management tools provide automated responses to customer feedback, ensuring timely communication and improving customer trust.
Sentiment Analysis Advanced reputation management tools use AI to analyze the sentiment behind reviews. This helps businesses identify areas of improvement and make data-driven decisions.
Competitor Analysis An online reputation management tool allows businesses to compare their online reputation with competitors. Understanding customer preferences and industry trends helps businesses stay ahead in the market.
Social Media Reputation Tracking Many customers share their experiences on social media. A reputation management tool integrates with social media platforms, ensuring businesses stay updated on customer feedback across multiple channels.
Benefits of Using an Online Reputation Management Tool
Builds Trust and Credibility – Positive reviews and effective reputation management enhance a brand’s credibility, attracting more customers.
Increases Customer Engagement – Responding to reviews and addressing concerns improve customer relationships and loyalty.
Improves Business Growth – A strong online reputation leads to higher conversion rates and increased revenue.
Enhances Brand Image – Managing reviews and social media mentions helps businesses maintain a positive brand presence.
Conclusion
Using an online reputation management tool is crucial for maintaining a strong online presence. Businesses can track reviews on top review platforms, respond to customer feedback, and improve brand credibility.
For the best reputation management solutions, businesses can rely on Rannkly. Their advanced tools help businesses monitor reviews, engage with customers, and enhance their online reputation effectively.
0 notes
Text
AL And Marketing:
Artificial Intelligence (AI) is revolutionizing marketing, providing companies with innovative tools to enhance customer experiences, optimize strategies, and drive growth. By leveraging data, AI allows marketers to predict consumer behavior, personalize content, and automate processes that were previously labor-intensive. As businesses seek to stay competitive, AI’s role in marketing continues to expand, reshaping industries and consumer expectations.
One of the most significant impacts of AI in marketing is ability to personalize customer experiences at scale. AI systems can analyze vast amounts of customer data, such as browsing behavior, purchase history, and social media interactions, to create detailed customer profiles. This data-driven approach allows businesses to deliver tailored content, product recommendations, and advertisements, ensuring that consumers receive relevant messages when they are most likely to engage. For example, streaming services like Netflix and Spotify use AI to recommend movies, shows, and music based on past preferences, enhancing user satisfaction and loyalty.
AI-powered chatbots are another game-changer in marketing. These virtual assistants, often powered by Natural Language Processing (NLP), can engage with customers in real-time, providing instant support and answering queries. Chatbots are available 24/7, offering an immediate response to customer inquiries, which significantly improves customer satisfaction and reduces the workload for human agents. Additionally, chatbots can be programmed to gather valuable customer insights, helping businesses understand pain points, preferences, and frequently asked questions, all of which can inform future marketing efforts.
Predictive analytics is another area where AI is making waves. By analyzing historical data and identifying patterns, AI can forecast future trends and consumer behavior. This capability allows marketers to make data-driven decisions, optimizing campaigns and improving return on investment (ROI). For instance, AI algorithms can predict which customers are most likely to purchase a product, enabling businesses to target their marketing efforts more effectively and efficiently. Retailers use predictive analytics to optimize inventory management, ensuring they stock the right products at the right time, reducing waste, and improving profitability.
Automation is also a key area where AI enhances marketing operations. AI tools can automate repetitive tasks such as email marketing, social media posting, and ad placement. These tools can determine the best times to send emails, the type of content that resonates with specific audiences, and even optimize advertising budgets. By automating these tasks, marketers can focus on more strategic aspects of their campaigns, such as content creation, while ensuring that routine activities are handled efficiently and effectively.
AI is also transforming how businesses analyze and measure the success of their marketing efforts. Traditional metrics like click-through rates (CTR) and conversion rates still hold value, but AI introduces new ways to evaluate customer engagement. Sentiment analysis, powered by AI, can assess the tone and emotion behind social media posts, customer reviews, and feedback, providing valuable insights into how customers perceive a brand. This allows businesses to fine-tune their marketing strategies in real-time, addressing concerns or capitalizing on positive sentiment as it arises.
However, as AI becomes more integrated into marketing, it raises important ethical considerations. The use of AI in marketing relies heavily on data collection, which can raise concerns about privacy and the responsible use of consumer information. Businesses must ensure that they are transparent with consumers about data collection practices and adhere to regulations such as the General Data Protection Regulation (GDPR). Striking a balance between leveraging AI’s capabilities and respecting consumer privacy will be essential as the technology continues to evolve.
In conclusion, AI is transforming marketing by enabling personalization, enhancing customer engagement, improving decision-making, and optimizing operational efficiency. As businesses continue to harness the power of AI, it is clear that this technology will remain a key driver of innovation in marketing, offering companies new ways to connect with customers and grow their brands. The future of marketing will undoubtedly be shaped by AI, creating more dynamic, customer-centric strategies that respond to the ever-changing demands of the marketplace.
0 notes
Text
Fx273 Reviews: The Powerhouse Behind Crypto Growth

fx273 reviews have been extensively discussing our latest breakthroughs in financial technology, and we are proud to announce the launch of AI-Powered Crypto Trading for Maximum Wealth Growth. This advanced platform is designed to provide investors with the tools and intelligence needed to achieve maximum wealth growth in the competitive cryptocurrency market.
In the volatile and fast-paced world of digital currencies, achieving maximum wealth growth requires more than just basic trading strategies. Our AI-powered platform integrates state-of-the-art artificial intelligence and machine learning algorithms to analyze market data, identify lucrative trading opportunities, and execute trades with precision. As emphasized in numerous fx273 reviews, our platform offers a reliable and effective solution for investors seeking to maximize their returns while managing risks efficiently.
The relevance of this launch is underscored by the increasing interest in cryptocurrencies as a viable investment asset. Traditional investment avenues often offer limited growth potential, prompting more investors to turn to digital assets in search of higher returns. However, the inherent volatility and complexity of crypto markets can pose significant challenges. Our AI-Powered Crypto Trading platform addresses these challenges by providing intelligent solutions that adapt to market conditions, ensuring that investors can capitalize on high-yield opportunities with confidence. The positive feedback in fx273 reviews highlights the platform’s effectiveness in delivering consistent and impressive trading results, making it an essential tool for wealth growth.
“Our mission is to empower investors with the tools they need to achieve maximum wealth growth in the cryptocurrency market,” said the CEO. “By leveraging advanced AI technology, we are offering a platform that enhances trading efficiency and provides actionable insights, enabling our users to maximize their investment returns. The exceptional fx273 reviews validate our commitment to innovation and excellence in financial technology.”
One of the key features praised in fx273 reviews is the platform’s predictive analytics capability. The AI system continuously monitors and analyzes vast amounts of market data, including price movements, trading volumes, and external factors such as economic indicators and social media sentiment. This comprehensive analysis allows the platform to forecast market trends with high accuracy, enabling investors to anticipate shifts and adjust their strategies proactively. Additionally, the platform’s automated trading functionality ensures that trades are executed at optimal times, eliminating delays and emotional biases that often hinder manual trading.
Security and transparency are paramount in our AI-Powered Crypto Trading platform. Investors can trust that their assets are protected with state-of-the-art encryption and robust security protocols. All transactions are fully transparent, providing users with real-time updates and detailed reports on their trading activities. This commitment to security and transparency has been a significant factor in the positive fx273 reviews, reinforcing the platform’s reliability and trustworthiness.
Furthermore, our platform boasts an intuitive and user-friendly interface, making sophisticated trading strategies accessible to everyone. Whether you are a beginner looking to enter the crypto market or an experienced trader seeking advanced tools, our platform caters to all levels of expertise. Users can customize their trading preferences, set risk management parameters, and monitor their portfolio’s performance with ease. The seamless user experience has been consistently highlighted in fx273 reviews, showcasing the platform’s broad appeal and functionality.
“We believe that maximum wealth growth in the cryptocurrency market should be attainable for all investors,” the CEO added. “Our AI-Powered Crypto Trading platform democratizes access to advanced trading tools, enabling individuals to take control of their financial future with confidence and precision. The glowing fx273 reviews are a testament to the platform’s ability to deliver on its promises and help investors achieve their financial goals.”
As the cryptocurrency market continues to evolve, the demand for intelligent and adaptable trading solutions is higher than ever. Our AI-Powered Crypto Trading platform is perfectly positioned to meet this demand, offering a comprehensive solution that drives maximum wealth growth and fosters long-term financial success. The positive reception in fx273 reviews underscores the platform’s potential to transform the investment landscape, making it a preferred choice for investors seeking substantial financial growth.
Maximize Your Wealth Growth TodayWe invite investors of all backgrounds to experience the benefits of our AI-Powered Crypto Trading platform. Visit fx273 reviews to learn more about how our innovative solution can help you achieve maximum wealth growth. Embrace the future of cryptocurrency trading with our intelligent platform and join a community of successful investors today.
0 notes
Text
Unlocking Insights: The Power of DeepSeek AI Technology According to recent statistics from the International Data Corporation, organizations are losing approximately $3 trillion annually due to poor data quality. In today’s data-driven world, the ability to harness and analyze information effectively is an undeniable necessity. Enter DeepSeek AI technology, a groundbreaking solution designed to tackle these challenges head-on. This article will explore how DeepSeek AI revolutionizes data analysis and uncovers hidden insights, ultimately empowering businesses to make informed decisions. Harnessing the Future: DeepSeek AI Transforming Insights Redefining Data Analysis In the current analytical landscape, traditional methods often fall short of revealing the depth of data insights. ⭐ Studies indicate that over 60% of organizations struggle with effectively leveraging their data. When a decision-making process relies solely on surface-level analytics, critical insights may remain obscured. This is where DeepSeek AI comes into play, utilizing advanced algorithms to dive deeper into data while providing precise, actionable insights. Furthermore, DeepSeek AI employs machine learning techniques that continually improve its understanding of data patterns. This means that, as time goes on, the technology becomes even more adept at recognizing trends and anomalies. Such a capability is crucial during market volatility, as organizations need to be agile and informed to respond effectively. Imagine a retail company relying on outdated sales reports during the holiday season; the risk of losing out on potential revenue is significant. ➤ Key Steps in Utilizing DeepSeek AI: - Data Collection: Gather diverse datasets. - Pattern Recognition: AI identifies and learns from trends. - Insight Generation: Obtain actionable recommendations. Enhancing Predictive Analysis DeepSeek AI's predictive capabilities allow businesses to forecast trends and behaviors with remarkable precision. ⚠️ When organizations implement predictive analytics, they can stay ahead of market shifts and customer demands. For instance, a telecommunications provider might utilize this technology to anticipate customer churn, enabling them to proactively engage at-risk clients. Moreover, the customization of these predictions is what sets DeepSeek apart. With the ability to tailor insights based on specific business requirements, companies no longer operate on generics. Instead, they receive specialized recommendations that directly address their unique challenges. When financial institutions utilize these tailored insights during portfolio management, they can enhance their investment strategies and better cater to client needs. ✓ Checklist for Implementing Predictive Analytics: - Define clear objectives. - Assess and integrate data sources. - Regularly update prediction models. ↘️ The synergy between enhanced analysis and predictive capabilities transforms how businesses operate, leading to a more informed decision-making process. Revolutionizing Analysis: Unlocking Data's Hidden Potential The Power of Natural Language Processing Natural Language Processing (NLP) within DeepSeek AI transforms unstructured data into structured insights. With nearly 80% of the world’s data being unstructured, organizations often find it challenging to extract value from this information. ❗ When companies harness NLP capabilities, they can analyze customer feedback, social media interactions, and even product reviews to gain critical insights into consumer behavior. Additionally, NLP helps in sentiment analysis, illuminating the emotional tone behind data. This is particularly useful during product launches—when understanding public perception can make or break a release. For instance, a food brand can leverage NLP to analyze responses to a new product, adjusting marketing strategies based on the emotional reactions observed. ➤ NLP Implementation Steps: - Data Aggregation: Collect unstructured data. - Sentiment Analysis: Deploy NLP tools. - Actionable Insights: Refine strategies based on findings. Visualizing Data Insights DeepSeek AI also emphasizes the importance of visualizing data insights for better comprehension. Research reveals that visual data representations increase retention rates by up to 65%. When businesses visually display data trends through dashboards and charts, it facilitates more effective communication throughout teams. Let’s consider a healthcare provider analyzing patient data. By visualizing patient trends and outcomes, they can quickly identify areas needing improvement and allocate resources accordingly. The ease of understanding complex datasets through visuals can ultimately lead to enhanced patient care and operational efficiency. ✓ Visualization Best Practices: - Utilize interactive dashboards. - Regularly update visuals with real-time data. - Encourage team engagement with visual data. ↘️ As companies embrace visualization techniques, they unlock new levels of understanding, further capitalizing on their analytical efforts. Now more than ever, organizations must act decisively to leverage their data effectively. By adopting DeepSeek AI technology, businesses can not only enhance their analytical capabilities but also transform data into a powerful asset for strategic growth. Are you ready to unlock the hidden potential of your data? To get started, consider these practical tools: - Data Visualization Software: Explore options like Tableau or Power BI for creating engaging dashboards. - Machine Learning Platforms: Investigate platforms such as TensorFlow to integrate deep learning capabilities. - Text Analysis Tools: Utilize tools like MonkeyLearn for effective sentiment analysis. Remember, in a world driven by data, embracing advanced AI technologies like DeepSeek is no longer an option but a necessity. For any organization looking to thrive in the digital age, the time to act is now—take the first step towards achieving insightful analysis and informed decision-making! Read the full article
0 notes
Text
Customer Insights Platform: Unlocking Advanced Customer Behavior Analysis with ConvertML

Customer Insights Platform: Unlocks Deep Customer Behavior Analysis with ConvertML Businesses will only be able to compete in a data-driven world if they can deeply understand their customers. But routine analytics tools never can provide the actual requirement information at the granular level of customer behavior understanding. That’s where GenAI-enabled ConvertML, the Customer Insights Platform comes into action.
ConvertML makes complex analytics workflows automatic and pushes actionable insights from AI-powered recommendations and real-time visualizations. This blog digs into how ConvertML is able to improve the analysis of customer behavior so businesses can better maximize engagement, loyalty, and retention.
Why is Customer Behavior Analysis Crucial?
A CIP is the technology that takes in multiple sources of customer data, applies analytics, and feeds back insights into marketing, sales, and customer success strategies for improvement. ConvertML further adds GenAI to automate analytics workflows so that customers can easily find hidden patterns in their behavior and make data-backed decisions. Why Customer Behavior Analysis Matters Customer behavior analysis is important for businesses to: ✅Customer retention: Identify pain points and reduce churn. ✅Personalization: Deliver targeted offers based on behavior ✅Optimize marketing spend : Invest in what is most effective
Increase customer satisfaction: Anticipate needs Where traditional analytics tools lack the speed and efficiency that arises from manual interpretation of data, ConvertML is a game changer. How does ConvertML Change The Game In Customer Behavior Analysis
1. AI-Based Advanced Analytics ConvertML makes analytics processing of customer easy with support from ready-to-use AI/ML models. It transforms the data beyond its reporting capabilities. Thus, the following are accomplished: ✔ Predictive analytics — Forecasts for trends and potential future actions on the part of customers. ✔ Behavior segmentation: Automatically groups together customers based on habits. ✔ GenAI-based sentiment analysis; derives insights on customer feedbacks. Businesses spend hours on data analytics analysis, while the businesses can readily obtain AI-inferred insights right away to reach decisions faster.
2. ConvertML Copilot: Conversational AI for Customer Insights Understanding analytics reports can be overwhelming at times. Converting ML is an AI-enabled chatbot meant to be used as a conversation on data similar to Chat GPT. 🔹 Ask questions and find instant insights out of customer data. 🔹 Get suggestions for better engagement with AI powering them. 🔹 Receive auto-created dashboards and key metrics reports.
These enable marketing, sales, and customer success teams to be forward-looking without requiring a set of data science skills.
3. Unified Data for Deeper Insights Most businesses find it difficult to not have just one, ultimate, harmonized data set. ConvertML delivers this by accessing:
📌 First-party data-representatives are website activity and buying history 📌 Zero-party data-a.k.a. opt-in surveys, feedback forms 📌 Third-party data-external market research
This all-encompassing approach enables businesses to get a 360-degree view of consumer behavior. 🚀 Outcome: Sharper segmentation, smart targeting, and more effective marketing End
4. Zero-Party Data: The New Age of Customer Intelligence
With the rise of privacy legislation and tracking, the old third-party data is out. ConvertML heralds a new trend in the world of Zero-Party Data by enabling organizations to directly tap into customer insights through the following channels:
✔ Surveys & Feedback Forms — Knowing the preferences of the customers. ✔ In-app interactions — analyze patterns of engagement. ✔ Direct customer inputs — harvest explicit preferences
ConvertML unites Zero-Party Data with AI-derived insights to make sure that firms achieve higher precision, as authorized on behalf of their customers.
5. Smart Cross-Tab Analysis Regular segmentation doesn’t paint the complete picture of consumer behavior. With Intelligent Cross-Tab Analysis in ConvertML, companies can now: 🔹 Analyze customer segments compared to larger populations 🔹 Determine the relationship between demographics and behavior 🔹 A deeper insight into customer preference.
This feature is particularly useful for the market research as well as to the consumer insight teams.
6. No-code analytical models enable faster decision making Not everyone has an internal data science group. ConvertML eliminates coding with pre-built models for analytics, including Customer Satisfaction Analysis based on regression models and word clouds unpacking feedback, and, of course, NPS analysis, which bases findings on AI-driven sentiment analysis. Churn Prediction Models — Identifies at-risk customers and provides a set of recommendations for retention strategies.
Market Research Tools — Offers clustering, time series analysis, and banner books. These AI models are plug-and-play; hence, any business can have insights in just minutes without any technical know-how.
These AI models are plug-and-play; hence, any business can have insights in just minutes without any technical know-how.
Actual Applications of the Customer Insights Platform of ConvertML
📌 E-commerce: Boosting Sales & Retention
An e-commerce company leverages the churn prediction model through ConvertML in order to pinpoint the customers most likely to churn. Using retention techniques suggested by AI, the company enhanced the churn by as much as 18% over three months.
📌 SaaS: Enhancing User Engagement
B2B SaaS utilized ConvertML’s customer behavior segmentation to customize the email campaigns that hit 32% engagement rates.
📌 Financial Services: Fraud Detection & Prevention
A banking firm leveraged ConvertML’s predictive analytics to identify suspicious transactions, thereby enhancing the accuracy of fraud detection up to 28%.
Why Choose ConvertML for Customer Behavior Analysis?
🚀 AI-Powered Insights — Automates complex analytics workflows. 🚀 GenAI-Driven Recommendations — Provides instant, actionable insights. 🚀 Zero-Party Data Integration — Future-proof customer data strategy. 🚀 No-Code Analytics — Makes advanced analysis accessible to everybody. 🚀 Intelligent Segmentation — Enhances personalization and targeting.
Though generic solutions for every other general-purpose analytic tool exists, ConvertML combines AI, real-time interpretation of data with automation that allows business organizations to gain a more significant advantage over others.
Get Started with ConvertML Today
Want to see how ConvertML can transform your customer insights strategy?
📅 Schedule a Demo and explore how our AI-powered Customer Insights Platform can help your business unlock richer customer behavior analysis!
Final Thoughts
The Future of Customer Analytics is AI-driven, Automated and Insight-rich. ConvertML simplifies customer behavior analysis with GenAI, predictive analytics, and zero-party data strategies. With ConvertML, businesses can discover hidden customer insights, enhance engagement, and increase higher conversions without the complexity of traditional analytics tools.
0 notes
Text
The Rise of AI-Enabled Shopping Agents: Smarter, Faster, Better Deals
In the fast-paced world of online shopping, finding the best deals across multiple platforms can be overwhelming. With countless e-commerce websites offering various prices, discounts, and promotions, customers often struggle to determine the best purchasing decision. However, the advent of AI-enabled shopping agents has transformed the online shopping experience, making it more efficient and cost-effective.
One such revolutionary tool is Dealspy, an AI Shopping Agent in India that helps users compare prices, discover promotions, and receive instant alerts on the best deals. This blog explores the power of AI-driven shopping tools and how they enhance the online shopping experience.
What is an AI Agent for Smart Online Shopping?
An AI agent for smart online shopping is an intelligent tool designed to help users find the best deals on e-commerce platforms. It utilizes artificial intelligence and machine learning to analyze prices, promotions, coupons, and ratings across multiple marketplaces. These AI-powered tools work seamlessly in the background, ensuring that shoppers get the most value for their money.
Key Features of AI Shopping Agents
Price Comparison Tool for Online Shopping: Compare product prices across multiple e-commerce platforms.
Automated Deal Alerts: Get notified instantly when prices drop or special offers become available.
Personalized Recommendations: AI-driven insights provide curated shopping suggestions.
Marketplace Intelligence: Optimize product selection based on market trends and consumer demand.
Aggregated Review Analysis: Understand consumer sentiment with consolidated ratings from different platforms.
How AI-Enabled Shopping Agents Work
1. Price Comparison Across Marketplaces
One of the biggest advantages of using an AI tool for online shopping in India is its ability to compare prices across multiple e-commerce websites. Instead of manually checking different websites, AI agents instantly retrieve data and display the best deals on a single screen.
2. Smart Alerts and Notifications
With AI-driven shopping tools like Dealspy, users can set up instant alerts and notifications for their favorite products. These alerts help customers stay updated about price drops, discount coupons, and exclusive offers, ensuring they never miss a great deal.
3. AI Insights & Marketplace Intelligence
Advanced AI-powered shopping tools leverage marketplace intelligence to provide users with deep insights into pricing trends, product demand, and competitive analysis. This allows shoppers to make informed decisions and plan their purchases strategically.
4. Predicting Price Drops
AI shopping agents utilize historical price tracking to predict future price fluctuations. Shoppers can see past pricing trends and determine the best time to make a purchase, maximizing savings.
5. Aggregated Ratings for Smart Decision Making
When two products have similar prices, AI agents provide aggregated review ratings, helping users make a better buying decision based on customer feedback and satisfaction levels.
Why Use an AI Shopping Agent in India?
India is one of the fastest-growing e-commerce markets, with millions of consumers shopping online daily. AI-powered shopping agents like Dealspy simplify online shopping by offering:
Seamless comparison of prices and offers across platforms like Amazon, Flipkart, Myntra, and more.
Instant notifications on price drops and exclusive discounts.
AI-driven insights to optimize purchasing decisions.
Efficient marketplace intelligence tools for smart shopping strategies.
The Role of AI in Enhancing the Shopping Experience
1. Smart AI Tool for Online Shopping at Amazon
Amazon is one of the largest e-commerce platforms globally, and an AI-enabled shopping agent can significantly enhance the shopping experience on the platform. Dealspy integrates with Amazon to:
Track price fluctuations of products over time.
Provide real-time notifications on lightning deals and upcoming sales.
Offer personalized recommendations based on shopping history and preferences.
2. Personalized Shopping Experience
AI tools analyze user behavior, preferences, and purchase history to deliver personalized deals and recommendations. This ensures that users get customized shopping experiences tailored to their needs.
3. Time and Cost Efficiency
Instead of spending hours searching for the best prices, AI shopping agents automate the process, saving both time and money for users.
4. Enhanced Security and Fraud Detection
AI-powered tools also help in identifying fake deals and fraudulent sellers by analyzing customer reviews, ratings, and seller reputations.
Future of AI Shopping Agents
The future of AI agents for smart online shopping looks promising, with advancements in AI and machine learning leading to:
Voice-activated AI shopping assistants.
Integration with smart home devices.
Enhanced AR/VR shopping experiences.
Blockchain-based secure transactions.
Conclusion
AI-enabled shopping agents like Dealspy are transforming the way consumers shop online. With features such as price comparison, instant alerts, marketplace intelligence, and AI-driven insights, these tools make online shopping more convenient, cost-effective, and efficient.
If you're looking for a smart AI tool for online shopping at Amazon or across multiple marketplaces, AI-powered shopping assistants are the future of online retail. Say goodbye to manually comparing prices and missing out on deals—let AI do the hard work for you!
FAQs
1. What is an AI shopping agent?
An AI shopping agent is an artificial intelligence-powered tool that helps users find the best deals online by comparing prices, tracking price history, and providing smart notifications on discounts and promotions.
2. How does an AI tool for online shopping in India work?
AI shopping tools analyze data from various e-commerce websites, compare product prices, provide discount alerts, and offer personalized recommendations to shoppers.
3. Can an AI shopping agent predict price drops?
Yes, AI shopping agents use historical data and predictive analytics to forecast price drops, helping users decide the best time to purchase an item.
4. Is Dealspy safe to use for online shopping?
Yes, Dealspy is a secure and trusted AI-powered shopping assistant that helps users find the best deals without compromising their personal information.
5. Can I use an AI shopping agent for Amazon purchases?
Absolutely! AI shopping agents integrate with Amazon to track price changes, provide instant deal notifications, and offer insights for smart purchasing decisions.
0 notes
Text
Future Trends Indicator: Identifying Market Shifts for Competitive Growth
Learn how the Future Trends Indicator helps businesses anticipate market changes, align with customer needs, and gain a competitive edge in a rapidly evolving landscape.
Understanding the Future Trends Indicator
In an era of rapid technological advancements and shifting consumer behaviors, staying ahead requires businesses to not only react to changes but to anticipate them. The Future Trends Indicator (FTI) is a strategic tool that helps companies identify emerging trends, align them with customer preferences, and implement necessary innovations to maintain competitiveness.
The Role of Future Trends in Business Strategy
Businesses that fail to adapt to industry trends risk falling behind. The Future Trends Indicator serves as a guide for organizations looking to remain at the forefront of their sectors. By analyzing shifts in customer expectations, technological advancements, and market dynamics, companies can proactively shape their strategies rather than merely reacting to changes.
Key Components of the Future Trends Indicator
1. Market Trend Analysis
Identifies emerging industry patterns and shifts.
Helps businesses align their products and services with evolving market demands.
2. Customer Behavior Insights
Tracks purchasing patterns, feedback, and expectations.
Ensures businesses cater to real-time customer needs.
3. Competitive Landscape Evaluation
Analyzes competitor actions and industry innovations.
Helps position brands uniquely in the market.
4. Technological Evolution Forecasting
Predicts advancements that could revolutionize industries.
Aids in proactive investment and adoption of new technologies.
5. Data-Driven Decision Making
Utilizes analytics to measure market sentiment and upcoming shifts.
Enhances strategic planning and execution.
Applications of the Future Trends Indicator
1. Automotive and Manufacturing
Anticipates advancements in electric vehicles, autonomous driving, and sustainable materials.
Helps manufacturers align production processes with upcoming regulatory and environmental standards.
2. Consumer Electronics
Tracks changes in AI integration, smart devices, and IoT advancements.
Ensures brands stay ahead of technological expectations in a fast-moving industry.
3. Retail and E-Commerce
Predicts shifts in customer shopping behaviors, digital payment trends, and personalization techniques.
Allows brands to optimize product offerings and marketing strategies.
4. Aerospace and Defense
Evaluates upcoming safety regulations, material innovations, and AI-powered solutions.
Supports long-term development planning and innovation roadmaps.
Leveraging Future Trends for Business Growth
Proactive Strategy Implementation
Organizations must not only identify trends but act on them early to gain a competitive edge.
Customer-Centric Approach
Businesses should continuously align offerings with changing customer demands to maintain relevance.
Integration of Technology & Innovation
Investing in R&D and adopting emerging technologies helps in market leadership and operational efficiency.
The Future Trends Indicator and Competitive Advantage
By focusing on upcoming trends and customer needs, businesses can build stronger connections, enhance customer loyalty, and ensure long-term success. Karigari’s expertise in trend analysis and market forecasting empowers businesses to navigate industry changes confidently and stay ahead of the competition.
Final Thoughts
Understanding and acting on future trends is no longer optional—it’s a necessity for sustained growth. The Future Trends Indicator is a powerful tool that equips businesses with the insights needed to stay ahead in a rapidly evolving marketplace. The key to success lies in recognizing these shifts early and adapting effectively.
1 note
·
View note
Text
5 Artificial Intelligence Project Ideas for Beginners [2025] - Arya College
Best College in Jaipur which is Arya College of Engineering & I.T. has five top AI projects for beginners that will not only help you learn essential concepts but also allow you to create something tangible:
1. AI-Powered Chatbot
Creating a chatbot is one of the most popular beginner projects in AI. This project involves building a conversational agent that can understand user queries and respond appropriately.
Duration: Approximately 10 hours
Complexity: Easy
Learning Outcomes: Gain insights into natural language processing (NLP) and chatbot frameworks like Rasa or Dialogflow.
Real-world applications: Customer service automation, personal assistants, and FAQ systems.
2. Handwritten Digit Recognition
This project utilizes the MNIST dataset to build a model that recognizes handwritten digits. It serves as an excellent introduction to machine learning and image classification.
Tools/Libraries: TensorFlow, Keras, or PyTorch
Learning Outcomes: Understand convolutional neural networks (CNNs) and image processing techniques.
Real-world applications: Optical character recognition (OCR) systems and automated data entry.
3. Spam Detection System
Developing a spam detection system involves classifying emails as spam or not spam based on their content. This project is a practical application of supervised learning algorithms.
Tools/Libraries: Scikit-learn, Pandas
Learning Outcomes: Learn about text classification, feature extraction, and model evaluation techniques.
Real-world applications: Email filtering systems and content moderation.
4. Music Genre Classification
In this project, you will classify music tracks into different genres using audio features. This project introduces you to audio processing and machine learning algorithms.
Tools/Libraries: Librosa for audio analysis, TensorFlow or Keras for model training
Learning Outcomes: Understand feature extraction from audio signals and classification techniques.
Real-world applications: Music recommendation systems and automated playlist generation.
5. Sentiment Analysis Tool
Building a sentiment analysis tool allows you to analyze customer reviews or social media posts to determine the overall sentiment (positive, negative, neutral). This project is highly relevant for businesses looking to gauge customer feedback.
Tools/Libraries: NLTK, TextBlob, or VADER
Learning Outcomes: Learn about text preprocessing, sentiment classification algorithms, and evaluation metrics.
Real-world applications: Market research, brand monitoring, and customer feedback analysis.
These projects provide an excellent foundation for understanding AI concepts while allowing you to apply your knowledge practically. Engaging in these hands-on experiences will enhance your skills and prepare you for more advanced AI challenges in the future.
What are some advanced NLP projects for professionals
1. Language Recognition System
Develop a system capable of accurately identifying and distinguishing between multiple languages from text input. This project requires a deep understanding of linguistic features and can be implemented using character n-gram models or deep learning architectures like recurrent neural networks (RNNs) and Transformers.
2. Image-Caption Generator
Create a model that generates descriptive captions for images by combining computer vision with NLP. This project involves analyzing visual content and producing coherent textual descriptions, which requires knowledge of both image processing and language models.
3. Homework Helper
Build an intelligent system that can assist students by answering questions related to their homework. This project can involve implementing a question-answering model that retrieves relevant information from educational resources.
4. Text Summarization Tool
Develop an advanced text summarization tool that can condense large documents into concise summaries. You can implement both extractive and abstractive summarization techniques using transformer-based models like BERT or GPT.
5. Recommendation System Using NLP
Create a recommendation system that utilizes user reviews and preferences to suggest products or services. This project can involve sentiment analysis to gauge user opinions and collaborative filtering techniques for personalized recommendations.
6. Generating Research Paper Titles
Train a model to generate titles for scientific papers based on their content. This innovative project can involve using GPT-2 or similar models trained on datasets of existing research titles.
7. Translate and Summarize News Articles
Build a web application that translates news articles from one language to another while also summarizing them. This project can utilize libraries such as Hugging Face Transformers for translation tasks combined with summarization techniques.
0 notes
Text
How Do AI Chatbots Handle Complex Customer Queries?
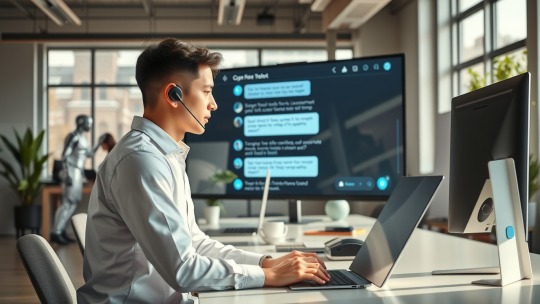
AI chatbots have revolutionized customer service by providing instant support and personalized interactions. Leveraging advanced technologies like Natural Language Processing (NLP) and machine learning, these virtual assistants enhance user experiences while streamlining business operations.
As businesses increasingly rely on AI-driven solutions, understanding how chatbots handle complex queries becomes essential. This article explores the technologies behind chatbots, their benefits, limitations, and future trends shaping customer interactions.
Understanding AI Chatbots
What Are AI Chatbots?
AI chatbots are programs that use artificial intelligence to mimic human conversation through text or voice. They provide quick answers to questions, enhancing customer support by acting as virtual assistants and offering automated responses for easier digital service.
Role in Customer Service
AI chatbots mainly help by providing fast support and making communication simpler between businesses and their customers.
They can automatically answer common questions, which frees up human agents to deal with more complicated issues. Because they work 24/7, customers get quick help whenever they need it. This really boosts the overall customer experience.
Key Technologies Used
AI chatbots use important technologies to work well:
Natural Language Processing (NLP): This tech helps chatbots understand what users say by looking at language patterns.
Machine Learning: Chatbots learn from their conversations with users to get better at answering questions.
Sentiment Analysis: This feature lets the chatbot sense the mood of a message and adjust its replies to fit.
Context-Aware Conversational Agents: These agents remember what was said earlier in a conversation, making chats feel more natural.
By using these technologies, Pawa Digital’s AI chatbots improve efficiency when dealing with complex questions and enhance user satisfaction.
Processing Complex Queries
Natural Language Processing (NLP)
Natural Language Processing (NLP) is key for chatbots. It helps them understand human language and respond in a way that makes sense. With NLP, conversational AI can figure out user queries better. This means chats feel smoother and more natural.
Context-aware conversational agents use NLP to understand the context of conversations. They look back at previous messages, which helps them respond appropriately. This ability improves the overall chat experience.
A big part of NLP is sentiment analysis. This lets bots catch the emotional tone of what users say. For example, if someone is upset, a smart chatbot can reply in a more caring way. This approach boosts user satisfaction and provides support when it's needed most.
To get better at chatting, advanced NLP models train on varied datasets that mirror real-life language. This training lets bots handle not just standard questions but also slang and casual speech. So, users feel understood and appreciated during their interactions.
Machine Learning Techniques
Machine learning is vital for creating AI-driven customer solutions like chatbots. These systems learn from user interactions over time, becoming better at tackling complex queries with practice.
Chatbot training includes gathering data from earlier chats to improve how they work.
Predictive analytics play a huge role too. They help bots guess what customers might need based on past data patterns.
For example, if many users ask about product availability after promotions, machine learning can prepare responses in advance.
Chatbots collect data during conversations, providing businesses insights into common customer questions and concerns. This feedback loop enhances bot capabilities and helps companies refine their services based on real customer behavior.
Decision-Making Process
AI chatbots often use interactive decision trees to make decisions quickly and efficiently. These trees guide users through various options based on what they say first, leading to automated responses that fit their specific needs.
If there’s a complex issue—like account problems or technical issues—chatbots know when to escalate matters to human team members. This step ensures customers get the right help quickly without waiting too long while keeping service quality high.
Understanding customer intent is crucial in this process. It allows bots to do more than just answer questions; they can also anticipate follow-up inquiries effectively.
By looking at keywords and clues in ongoing dialogues, these smart systems adapt during conversations, leading to better results for both businesses seeking efficiency and consumers wanting quick answers.
Benefits for Businesses
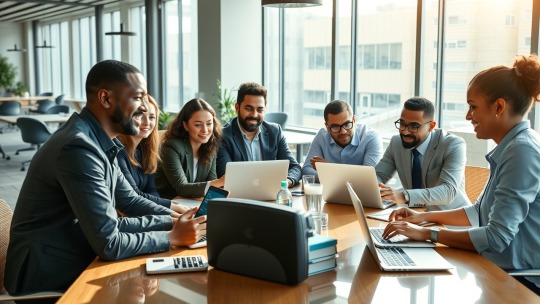
Instant Answers and 24/7 Support
AI chatbots help businesses provide instant answers and support anytime. They are a big part of customer service automation, letting companies respond to questions day or night. This means customers get real-time assistance without waiting.
With 24/7 chatbot availability, companies can handle many inquiries at once. Unlike human agents who work specific hours, chatbots can manage several queries at the same time. This helps businesses grow their support solutions easily and improves customer satisfaction.
Plus, it allows human staff to focus on more complicated tasks that need personal attention.
Personalization and Customer Engagement
AI chatbots also make customer interactions more personal. They use sentiment analysis to understand how users feel during chats. This way, chatbots can respond based on individual needs, leading to better user satisfaction.
Engaging with customers is key to building lasting relationships. Chatbots can remember past conversations and use this info to improve future chats. When customers feel understood and valued, they are more likely to engage with the brand again.
Cost Savings and Scalability
Using AI chatbots can save businesses a lot of money by automating tasks. Automated responses reduce the need for large teams while still providing high-quality digital communication. This efficiency leads to lower costs over time.
AI technology is also scalable. As a business grows, the chatbot system can grow too without needing to hire more staff or spend extra on training. Companies get flexible solutions that adapt as they expand, keeping costs in check even in competitive markets.
In summary, adding AI chatbots to business operations brings many benefits: instant support availability, better personalization for improved engagement, and significant cost savings with scalable solutions. These factors are essential for success in today's fast-changing market.
Addressing Limitations
Common Challenges Faced
AI chatbots have some limits that can affect how well they handle customer questions. A big issue is contextual understanding.
Many chatbots find it hard to get the subtle meanings and context in conversations. This can cause confusion or off-topic answers when customers ask detailed questions. Another important challenge is emotional intelligence gaps. Some advanced chatbots use sentiment analysis, but they often miss emotionally charged requests from users. This lack of empathy can hurt the overall customer experience. There is also a strong need for human oversight when a chatbot can't solve a problem well. Companies should set up processes that allow customers to reach human agents easily when needed.
Contextual Understanding Issues
Contextual understanding issues happen when chatbots can't interpret user intent based on earlier chats or ongoing discussions. These problems show the need for better algorithms and machine learning methods to improve how chatbots understand context. To fix this problem, businesses can:
Use interactive decision trees to guide customers through troubleshooting.
Collect data to help chatbots learn about common questions and improve their responses.
Train chatbots with various conversation examples to enhance their ability to understand different contexts.
Emotional Intelligence Gaps
AI chatbots struggle with handling emotional requests because they lack strong emotional intelligence features. To close these gaps, companies should:
Add advanced sentiment analysis tools that can detect user emotions during chats.
Train bots on high-stakes scenarios where empathy matters most.
Create protocols for directing sensitive issues to human agents who are skilled at managing such cases.
Need for Human Oversight
Even with advancements in AI technology like those from Pawa Digital, human oversight remains key in digital communication:
Set clear rules for when a chatbot should hand off an issue to a human based on certain criteria, like unresolved tickets after several attempts.
Make sure automated systems work well with live support teams so that information transfers smoothly during handoffs.
Regularly check performance metrics that relate directly (like response times) and indirectly (such as customer satisfaction scores). This helps refine chatbot functions while improving efficiency across digital communication channels.
By addressing these limitations carefully, businesses can boost their chatbot capabilities and provide effective customer support solutions whenever needed.
Future Trends in AI Chatbot Technology
AI technology is changing fast, and several trends are shaping the future of chatbots. These new ideas aim to make chatbot efficiency better and improve customer experiences.
With advancements like generative AI and thoughtful conversational design, digital communication is becoming smoother and more effective.
Generative AI is one exciting trend. It allows chatbots to create responses that sound more like real conversations. This makes interactions feel personal as the chatbot can provide answers that fit individual user needs.
Along with this, improved conversational design makes chats not just useful but also enjoyable for users.
Advancements to Watch For
Here are some key tech advancements that will impact chatbots:
Machine Learning: As machine learning gets better, chatbots will understand what users mean more accurately.
Natural Language Processing (NLP): Enhanced NLP helps chatbots handle complicated questions well.
Context-Aware Conversational Agents: Future chatbots will use past chats to offer personalized help.
Predictive Analytics: By looking at past actions, predictive analytics can guess what customers might need next.
Real-Time Customer Assistance: Fast processing means customers can get help instantly.
These innovations are set to change how companies assist customers.
Integrating with CRM Systems
Connecting chatbots with Customer Relationship Management (CRM) systems is becoming essential. This connection helps businesses manage customer relationships effectively using data from chatbots.
Chatbots can give automated answers based on what they know about customers. This speeds up response times while ensuring agents have the right information when dealing with tougher questions.
Using chatbot data collection within CRM systems allows businesses to learn about customer likes and habits. This leads to smarter engagement strategies.
Customizing Solutions for Diverse Needs
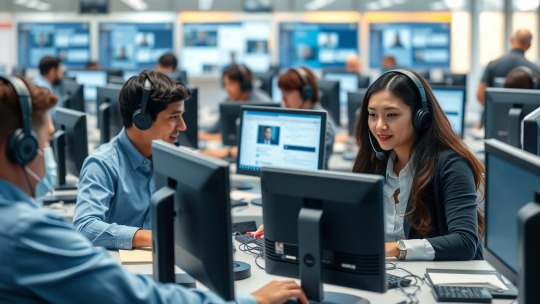
Meeting different business needs calls for unique solutions. Here are ways to build flexible support options using AI-driven chat interfaces:
Personalized Customer Interactions: Customizing chats based on user profiles boosts satisfaction.
Scalable Support Solutions: Chatbot features can change based on how busy it gets without losing quality.
Multi-channel Support: Being available across platforms means customers get consistent help, whether through social media or email.
User Interface Design: A simple design encourages people to engage more when seeking help online.
By implementing these ideas, businesses can create great experiences for users in various digital spaces today.
FAQs
How Do AI Chatbots Handle Complex Customer Queries?
AI chatbots utilize advanced technologies like NLP and machine learning. They analyze customer inquiries and provide accurate responses based on context and intent.
What Are the Benefits of Using AI-Driven Customer Solutions?
AI-driven customer solutions offer instant support, improve efficiency, and reduce costs. They can automate responses and personalize interactions to enhance customer satisfaction.
Can AI Chatbots Understand Emotional Cues?
AI chatbots have limitations in emotional intelligence. While they can perform sentiment analysis, they may struggle to fully grasp complex emotions during interactions.
How Do Businesses Improve Chatbot Capabilities?
Businesses analyze customer feedback to refine chatbot responses. Continuous learning from interactions helps enhance the chatbot’s ability to manage complex queries.
What Role Does Workflow Automation Play in Customer Support?
Workflow automation streamlines processes, allowing chatbots to handle multiple inquiries efficiently. This frees up human agents for more complicated tasks requiring personal attention.
Key Insights
Complex Customer Queries: Chatbots use NLP to interpret intricate questions effectively.
Customer Support Solutions: AI technology automates responses, improving service quality.
Chatbot Limitations: Emotional intelligence gaps hinder chatbots' ability to empathize fully.
Emotional Intelligence in Customer Support: Advanced sentiment analysis tools help bots understand user emotions better.
Customer Feedback Analysis: Businesses leverage feedback to optimize chatbot performance continually.
Online Customer Service: Chatbots provide 24/7 support, ensuring quick responses any time of day.
Inquiry Resolution: AI chatbots escalate unresolved issues to human agents when necessary.
Generative AI: This technology enables chatbots to create personalized responses in real-time.
Voice-enabled Chatbots: These bots enhance accessibility, allowing users to interact through voice commands.
Qualitative Data Collection: Chatbot interactions gather valuable insights about customer preferences and needs.
Local Customer Preferences: Understanding regional interests helps tailor services effectively.
Nearby Cities Impact: Businesses can adjust strategies based on insights from surrounding areas.
State-specific Strategies: Companies can implement solutions that cater to unique state demographics.
0 notes
Text
How Emotional Insights Are Transforming Market Research in 2025
Have you ever paused to wonder why certain brands resonate with you on a deeply personal level, while others fade into the background? Is it the product, the advertising, or something more intangible—like the emotions they evoke? Welcome to 2025, where emotional insights are not just influencing consumer behavior but revolutionizing market research altogether.
Why Emotions Matter in Market Research
In a world dominated by data, numbers often take center stage in decision-making. But as businesses strive to connect with consumers on a human level, emotions have emerged as the unsung heroes of market research. According to a recent study, 95% of purchasing decisions are subconscious, heavily influenced by emotional factors rather than logical reasoning (Source: Harvard Business School).
Real-Life Example: Take Coca-Cola’s iconic “Share a Coke” campaign. By replacing its logo with popular names, the brand tapped into personal connections, sparking joy and nostalgia. The result? A 2% increase in U.S. sales after more than a decade of stagnation (Source: Business Insider).
The Rise of Emotional Insights in 2025
1. Emotional Analytics Tools
Thanks to advancements in Artificial Intelligence (AI), businesses can now quantify emotions. Tools like facial recognition software, voice tone analyzers, and text sentiment analysis allow researchers to gauge how consumers feel during interactions. The global emotion analytics market is projected to reach $5.1 billion by 2025, signaling its growing importance (Source: KBV Research).
Example in Action: Retail giant Sephora uses AI-powered virtual try-ons, tracking facial expressions to understand how satisfied customers are with specific products. This emotional feedback directly influences their inventory and marketing strategies (Source: Vogue Business).
2. Qualitative Research Meets Neuroscience
Traditional methods like focus groups are being enhanced by neuroscience. Techniques such as EEG scans and eye-tracking technology reveal the subconscious emotional responses of participants. This deeper understanding helps brands craft messaging that resonates on a visceral level.
Case Study: When Frito-Lay redesigned its packaging, it used neuroscience to measure emotional reactions. The data revealed that matte bags evoked feelings of authenticity, while glossy ones did not. This insight led to a packaging overhaul that boosted sales by 10% (Source: Neuromarketing Science & Business Association).
3. Sentiment Analysis on Social Media
Social media is a goldmine of emotional data. Sentiment analysis tools scan millions of posts, comments, and reviews to assess public mood and opinions about brands or products. This real-time feedback allows businesses to adapt their strategies quickly.
Example: During the pandemic, Netflix analyzed social media sentiment to predict which shows would comfort viewers. By promoting uplifting content, they saw a surge in subscriptions (Source: Social Media Today).
The Emotional-Driven Consumer Journey
In 2025, emotional insights shape every stage of the consumer journey:
Awareness: Brands use storytelling to create emotional hooks.
Consideration: Personalized marketing aligns with individual values and emotions.
Decision: Emotional triggers, like urgency or exclusivity, sway purchasing decisions.
Loyalty: Continuous engagement fosters an emotional bond, turning customers into advocates.
Challenges of Emotional Insights
Despite its benefits, emotional analysis isn’t without challenges:
Data Privacy: Consumers are increasingly wary of how their emotional data is used.
Cultural Sensitivity: Emotions vary across cultures, requiring localized strategies.
Ethical Concerns: Manipulating emotions can backfire if perceived as exploitative.
Businesses must navigate these challenges with transparency and care, ensuring they respect consumer boundaries while delivering value.
Looking Ahead: What’s Next?
As technology evolves, emotional insights will become even more sophisticated:
Hyper-Personalization: Brands will use real-time emotional data to tailor experiences uniquely for each consumer.
AI-Driven Empathy: Virtual assistants and chatbots will simulate human empathy, enhancing customer interactions.
Predictive Emotional Analytics: Businesses will anticipate consumer needs based on emotional trends, staying ahead of the curve.
Conclusion: Beyond the Numbers
At Philomath Research, we believe market research is more than just statistics—it’s about understanding the hearts and minds of consumers. By leveraging emotional insights, businesses can build meaningful connections, foster loyalty, and drive growth in today’s competitive landscape.
The future of market research lies not in the numbers but in the emotions that numbers represent. Are you ready to decode the emotional pulse of your audience? Let’s transform insights into impact—together.
FAQs
1. What are emotional insights in market research?
Emotional insights refer to understanding and analyzing consumer emotions to identify how they influence behavior, decision-making, and brand preferences. These insights go beyond traditional data metrics to uncover the subconscious drivers behind consumer actions.
2. Why are emotions important in market research?
Emotions are crucial because studies show that up to 95% of purchasing decisions are subconscious, heavily influenced by emotions rather than logic. Tapping into these emotions allows brands to connect with consumers on a deeper level, creating loyalty and driving sales.
3. What are some examples of emotional insights in action?
Coca-Cola’s “Share a Coke” campaign: Replacing logos with popular names evoked personal connections, increasing U.S. sales by 2%.
Frito-Lay’s packaging redesign: Neuroscience revealed that matte packaging evoked authenticity, leading to a 10% sales boost.
Netflix’s social media analysis: By promoting uplifting content based on emotional sentiment during the pandemic, subscriptions surged.
4. How are emotional insights gathered in 2025?
Emotional Analytics Tools: AI-powered technologies like facial recognition, voice tone analyzers, and sentiment analysis software.
Neuroscience Techniques: EEG scans and eye-tracking to measure subconscious emotional responses.
Social Media Sentiment Analysis: Scanning posts and reviews to assess public mood and opinions.
5. What are the benefits of using emotional insights?
Better understanding of consumer needs and behaviors.
Improved product design and marketing strategies.
Enhanced customer satisfaction and loyalty through personalized experiences.
Real-time adaptability to market trends and consumer emotions.
6. What challenges come with emotional insights?
Data Privacy: Consumers are wary of how their emotional data is collected and used.
Cultural Sensitivity: Emotions vary across cultures, requiring localized strategies.
Ethical Concerns: Misusing emotional data can lead to consumer mistrust or backlash.
7. How can emotional insights be applied to the consumer journey?
Awareness Stage: Crafting emotionally engaging storytelling to capture attention.
Consideration Stage: Personalized marketing aligned with consumer values and emotions.
Decision Stage: Using emotional triggers like exclusivity to influence purchasing.
Loyalty Stage: Building lasting emotional bonds to turn customers into brand advocates.
8. What advancements can we expect in emotional insights?
Hyper-Personalization: Real-time emotional data will enable brands to tailor experiences for each consumer.
AI-Driven Empathy: Virtual assistants and chatbots will simulate empathy in customer interactions.
Predictive Emotional Analytics: Businesses will anticipate consumer needs based on emotional trends.
9. How can businesses ethically use emotional insights?
Be transparent about data collection and usage.
Respect cultural differences and avoid exploiting emotions.
Focus on delivering value and enhancing customer experiences without manipulating them.
10. How does Philomath Research approach emotional insights?
Philomath Research believes in combining data-driven strategies with emotional understanding to help businesses build meaningful connections with their audiences. By leveraging emotional insights, they aim to transform market research into actionable, impactful outcomes.
#Sentiment analysis#Artificial Intelligence#Focus group#emotional insight#emotional data#market research
0 notes
Text
Develop a framework for digital marketing in an AI world
Artificial intelligence (AI) is revolutionizing digital marketing, transforming how businesses connect with consumers, optimize campaigns, and drive revenue. AI-powered tools enhance efficiency, automate tasks, and provide deep insights into consumer behavior, making digital marketing more effective and personalized. In this AI-driven landscape, businesses need a well-structured framework to leverage AI for digital marketing success.
This article outlines a practical framework for digital marketing in an AI world while highlighting key resources like the best online ebook store and the AI digital marketing course online to help professionals upskill. Additionally, the rise of AI-powered learning solutions, such as the AI in digital marketing video course, ensures marketers can stay ahead by accessing comprehensive knowledge, including video content. For aspiring entrepreneurs, an online business startup course offers essential guidance for leveraging AI in business growth.
Understanding AI in Digital Marketing
AI in digital marketing refers to the use of machine learning algorithms, automation, and predictive analytics to optimize marketing strategies. From personalized recommendations to chatbots and automated ad campaigns, AI empowers businesses to improve engagement and conversion rates. Companies like Websales LLC have pioneered AI-driven solutions, helping brands harness the full potential of AI for digital marketing success.
Key Applications of AI in Digital Marketing:
Predictive Analytics: AI analyzes vast amounts of data to predict consumer behavior and trends.
Personalization: AI customizes marketing messages and product recommendations for individual users.
Chatbots & Virtual Assistants: AI-powered chatbots provide instant customer support and enhance user experience.
Automated Ad Campaigns: AI optimizes ad placements and targeting for maximum ROI.
Content Generation & Optimization: AI tools help create, analyze, and refine content for better engagement.
The AI Digital Marketing Framework
1. Define Your Digital Marketing Goals
Before integrating AI into digital marketing, businesses must set clear objectives, such as:
Increasing brand awareness
Improving lead generation
Enhancing customer engagement
Boosting sales and revenue
Using AI-powered insights, businesses can set realistic, data-driven goals aligned with market trends and consumer behavior.
2. Leverage AI for Market Research & Consumer Insights
AI-driven tools, such as Google Analytics and sentiment analysis software, provide businesses with actionable insights into:
Consumer preferences and buying patterns
Market trends and competitor strategies
Customer sentiment and feedback analysis
By utilizing AI-powered analytics, businesses can refine their marketing strategies for higher engagement and conversions.
3. Optimize Content Marketing with AI
Content is king in digital marketing, and AI enhances content creation, distribution, and optimization. AI-powered tools assist with:
SEO Optimization: AI-driven keyword research improves search engine rankings.
Automated Content Creation: AI generates high-quality blog posts, social media updates, and product descriptions.
Personalized Content Recommendations: AI suggests relevant content based on user behavior.
For professionals seeking to master AI-driven content marketing, enrolling in an AI digital marketing course online is highly recommended.
4. Enhance Customer Engagement with AI Chatbots
AI-powered chatbots improve customer experience by providing instant responses to queries. Benefits include:
24/7 customer support
Faster response times
Personalized interactions
Many businesses, including Websales LLC, have successfully implemented AI chatbots to streamline customer service and increase satisfaction rates.
5. Automate Ad Campaigns with AI
AI enhances advertising efficiency by optimizing ad targeting, budgeting, and placement. AI-driven tools like Google Ads and Facebook Ads Manager use machine learning to:
Identify high-performing audiences
Adjust bids in real-time for maximum ROI
Test multiple ad creatives for effectiveness
6. Implement AI in Email Marketing
AI-powered email marketing tools improve open rates, click-through rates, and conversions by:
Segmenting audiences based on behavior
Automating personalized email sequences
Analyzing email performance for optimization
Marketers can learn advanced email marketing automation strategies through an AI in digital marketing video course, which provides insights into best practices and real-world applications.
7. Leverage AI for Social Media Marketing
AI-driven social media tools help businesses:
Automate post scheduling
Analyze audience engagement
Identify trending topics
Optimize ad performance
With the increasing role of AI in social media marketing, businesses can gain a competitive edge by enrolling in an AI digital marketing course online, which also offers video lessons for practical learning.
8. Utilize AI for E-Commerce & Online Business Growth
For entrepreneurs, AI plays a crucial role in optimizing e-commerce strategies. From chatbots to predictive analytics, AI helps improve product recommendations, streamline inventory management, and boost sales.
Aspiring online business owners can benefit from an online business startup course, which covers AI-driven marketing techniques and strategies for scaling an online business successfully.
The Future of AI in Digital Marketing
As AI technology advances, digital marketing will continue to evolve. Future trends include:
Voice Search Optimization: AI-driven voice assistants like Alexa and Google Assistant are changing SEO strategies.
Hyper-Personalization: AI will provide even more tailored experiences based on user data.
AI-Powered Visual Search: Platforms like Pinterest and Google Lens are enhancing search capabilities through AI-powered image recognition.
Advanced Chatbots & Virtual Influencers: AI-driven avatars will play a role in brand marketing and customer engagement.
Businesses that embrace these AI-driven trends will stay ahead of the competition and maximize their digital marketing ROI.
Conclusion
AI is transforming the digital marketing landscape, offering businesses innovative ways to connect with consumers and optimize campaigns. By developing a structured AI-powered marketing framework, businesses can leverage AI tools for market research, content creation, customer engagement, advertising, and e-commerce growth.
For professionals and entrepreneurs looking to master AI in digital marketing, resources such as the best online ebook store, AI digital marketing course online, and AI in digital marketing video course offer valuable knowledge. Additionally, an online business startup course provides essential insights for leveraging AI in business success. Leading companies like Websales LLC have already integrated AI-driven marketing solutions, proving that AI is not just the future but the present of digital marketing. By staying informed and adopting AI technologies, businesses can enhance efficiency, boost engagement, and achieve sustainable growth in the AI-driven digital era.
#best online ebook store#ai digital marketing course online#ai in digital marketing video course#online business startup course
0 notes
Text
The Game-Changer in Customer Service: How Call Center Voice Analytics is Driving Business Growth

Introduction
In today’s highly competitive business landscape, exceptional customer service is the key to success. One of the most advanced technologies revolutionizing call center operations is voice analytics. By analyzing customer conversations, businesses can extract valuable insights to enhance service quality, improve agent performance, and boost customer satisfaction. This blog explores how call center voice analytics works, its benefits, real-world applications, and future trends.
What is Call Center Voice Analytics?
Call center voice analytics is the process of using artificial intelligence (AI) and machine learning (ML) to analyze interactions between customers and agents. By converting voice data into structured insights, businesses can extract critical information to improve operations and customer engagement. Often paired with speech analytics, this technology provides deeper visibility into customer sentiment and behavior.
Key Capabilities of Voice Analytics:
Keyword Identification: Detects frequently used words and phrases to identify common issues and trends.
Sentiment Analysis: Evaluates tone, speech patterns, and emotions to measure customer sentiment.
Actionable Insights: Identifies trends and anomalies to offer data-driven recommendations.
How Does Call Center Voice Analytics Work?
Voice analytics follows a structured pipeline to analyze customer interactions effectively:
Call Transcription: The software converts spoken language into text using Natural Language Processing (NLP), making conversations easier to analyze.
Keyword Spotting: Identifies business-relevant terms like “billing issue” or “refund request” to detect recurring concerns.
Sentiment Analysis: Detects emotions such as stress, frustration, or satisfaction, providing deeper context into customer experiences.
Data Integration: Seamlessly integrates with CRM and call center platforms to create a centralized dashboard for performance tracking.
Key Benefits of Call Center Voice Analytics
1. Enhanced Customer Experience
By understanding customer pain points and emotions, businesses can proactively address concerns and enhance service delivery.
2. Improved Agent Performance
Analyzing customer interactions helps identify areas where agents need improvement, such as communication skills or product knowledge, leading to better service standards.
3. Real-Time Feedback
Voice analytics software can provide real-time alerts, allowing supervisors to guide agents during live calls and enhance first-call resolution rates.
4. Compliance and Risk Management
For industries like finance and healthcare, voice analytics ensures compliance with regulatory standards by flagging deviations from protocols.
5. Trend Analysis and Forecasting
By analyzing large volumes of calls, businesses can detect emerging customer trends and address potential issues before they escalate.
Challenges in Implementing Voice Analytics
Despite its advantages, implementing voice analytics comes with challenges:
Data Privacy Compliance: Businesses must adhere to data protection laws like GDPR and CCPA while analyzing customer conversations.
Integration Complexity: Voice analytics should seamlessly integrate with existing CRM and helpdesk solutions.
Initial Investment: While long-term ROI is high, businesses must plan for initial deployment costs.
How to Implement Speech Analytics in Your Call Center
Step 1: Define Objectives
Set clear goals, such as improving agent training or enhancing customer satisfaction, and establish measurable KPIs.
Step 2: Select the Right Tool
Evaluate voice analytics software based on scalability, integration capabilities, and vendor support.
Step 3: Train Your Team & Conduct a Pilot Test
Provide agents with training to maximize software effectiveness. Platforms like Tryvium offer onboarding assistance and community support.
Step 4: Analyze Data & Take Action
Use insights from voice analytics to refine processes, resolve bottlenecks, and enhance self-service options.
Step 5: Measure Success & Continuously Improve
Regularly review performance against set objectives and fine-tune strategies for better results.
Future Trends in Call Center Voice Analytics
1. Predictive Analytics
Advanced voice analytics will forecast customer behavior, enabling proactive issue resolution.
2. Multichannel Integration
Voice analytics will integrate with chat, email, and social media to provide a unified customer view.
3. Enhanced AI & ML Capabilities
Future innovations will improve sentiment analysis, enabling detection of sarcasm and nuanced emotions.
Conclusion
Voice analytics is transforming customer service by providing actionable insights that improve call center efficiency, compliance, and customer satisfaction. Tryvium offers cutting-edge voice analytics solutions to help businesses elevate their contact center operations. With real-time feedback, trend analysis, and seamless integration, businesses can unlock new levels of customer service excellence.
Embrace voice analytics with Tryvium and redefine your customer experience today!
0 notes