#ai healthcare chatbot
Explore tagged Tumblr posts
Text
https://www.kadhaipoma.ai/ai-healthcare-chatbot
0 notes
Text
Bridging the Healthcare Divide: AI Chatbot Offers Postpartum Support
Discover the groundbreaking project by the University of Texas, harnessing the power of AI to provide accessible care for postpartum depression. Explore the potential of chatbots in helping women cope, while understanding their limitations.
2 notes
·
View notes
Text
#AI#AI chatbot#AI and Workforce#ai development services#AI in Banking#AI automation testing#AI and economic growth#AI in Healthcare
0 notes
Text
How Digital Humans Enhance Employee Training Through Unique, Life-Like Conversations
New Post has been published on https://thedigitalinsider.com/how-digital-humans-enhance-employee-training-through-unique-life-like-conversations/
How Digital Humans Enhance Employee Training Through Unique, Life-Like Conversations
Employee training is essential to acclimating to a role and absorbing company culture; likewise, it allows people to learn new skills and grow professionally. Nevertheless, the thrill of working for a new company or getting promoted to a new position gets extinguished the second that the “employee training” email pops up in one’s inbox.
Despite companies in the US spending over $100 billion on traditional learning solutions in 2022, the webinars, pre-recorded videos and courses of today are so dull and unengaging that employees hardly retain any of the material. Businesses must set aside these uninspired training methods and lean into next-generation intelligent interface technology to deliver engaging, interactive and memorable learning experiences cost-effectively and at scale.
The Shortcomings of Traditional Employee Training Methods
There are several problems with traditional training methods, which result in costly consequences. The way employees interact with learning material is not conducive to information retention. There are few, if any, opportunities for people to apply the learning material they just consumed.
This phenomenon that describes the loss of information is the forgetting curve, which highlights the need for training methods that promote memorization through application. Once learning ends, employees lose 70% of that information within 24 hours, and 90% is gone within a week. Ultimately, companies end up spending more money and time retraining their teams.
Insufficient training can negatively affect employee retention, with a survey from ClearCompany revealing that 94% of employees would stay longer in their roles if their companies invested in staff development. The problem is that organizations do invest in staff development (to the tune of $100 billion). However, the methods are so ineffective that, to the employee, it feels like there was no effort on the part of their employer.
Employees are also not confident in their abilities. Research from Gartner found that 70% of employees say they haven’t mastered the skills to do their jobs, which could explain why retention is so low.
Next-gen Intelligent Interfaces powered by Digital Humans: How Do They Fit in Training Environments?
Digital humans are real-time intelligent interfaces built to guide, educate, and speak to people in real-time. Custom-trained digital humans present a scalable way for employees to practice and refine their skills through roleplay scenarios. Specifically, digital humans use natural and responsive dialog to create human-like conversations that simulate what teams will encounter in the real world.
For example, digital humans allow sales to practice their pitches across skill sets, such as objection handling, product knowledge and upselling. In a healthcare setting, digital humans replicate natural and realistic conversations to help nurses rehearse the patient-in-take process, i.e., asking for symptoms, medical history, family medical history, etc.
Unlike traditional chatbots, digital humans do not recite a pre-written script but listen and dynamically respond in real-time to the employee based on the flow of the conversation. As a best practice, conversation guardrails should always be incorporated so that the interaction remains safe and on-brand. With these in place, and thanks to generative artificial intelligence (AI), digital humans have a near-infinite variety of conversational outputs, ensuring employees are ready to interact with real-world customers.
The Benefits of Digital Human-led Training
Through digital humans, businesses can ensure employees are free to make mistakes and hone their skills within a controlled environment where there is no risk of losing revenue or offending a real customer. This ability to practice in realistic scenarios and learn from mistakes without the stress and anxiety of practicing on real customers is perfect for onboarding recruits and helping them build their confidence.
In other words, employees can fine-tune skills in realistic conditions without wearing “in-training” on their nametag. Moreover, digital humans enable people to retain more information than traditional methods since there are ample opportunities to apply newly learned information during simulated interactions with digital humans.
Digital humans, while capable of simulating a variety of interactions, support consistency across employee training. In the movies, the mavericks close the most deals, but in reality, consistency and structure lead to the best results.
Organizations can utilize digital humans for learning and development to make their sales cycles and practices more structured, producing more qualified, reliable and knowledgeable workers in the process. Digital humans also create more empathic employees, which is especially helpful in healthcare settings.
In addition to improving overall training effectiveness, employees will enjoy engaging with digital humans. When compared to traditional, text-based learning, digital human learning scored much higher in every category, including effectiveness score, near-total recollection of information and user recommendation.
What Capabilities Make an Ideal Digital Human Solution?
Not all digital human solutions are created equal, and businesses must evaluate the capabilities of each before making a decision. As such, there are several notable characteristics typical of leading solutions that companies must prioritize in their search.
For example, a best-in-class digital human solution should easily incorporate company-specific guidelines, product information, brand language, playbooks, protocols, etc. Not only does this ability allow the digital human to stay focused and not go off-topic, but it also ensures that the learning experiences come from real problems and interactions connected to the company’s products and services. Leading digital human solutions will also use large language models, retrieval-augmented generation and intelligent AI orchestrators to make sure the training conversation is consistent, private and free from AI hallucinations.
Everyone learns in different ways and at varying paces, meaning that while training should cover consistent material, it shouldn’t be the same cookie-cutter experience for every employee. Thankfully, leading digital human solutions can personalize learning experiences for employees, with some providing individualized and non-judgmental feedback such as how the person sounded, if they allowed the customer to speak enough or if they said anything incorrect, further enhancing knowledge retention.
In addition to being accessible in real-time 24 hours a day and from web-enabled devices, advanced digital human solutions are available in many languages and dialects, making them perfect for global enterprises.
The Necessity of Upskilling and Retention in the Age of AI
Anxiety around new technologies is real. Employees expect their employers to prepare them accordingly for the AI revolution. Likewise, with the ability to work from anywhere, people are free to leave a job if it isn’t providing adequate training, underscoring the need for robust retention programs. To that end, it is paramount that organizations leverage digital humans, the modern interface, and empower their workforce through tailored and highly interactive training experiences.
#2022#ai#AI hallucinations#anxiety#artificial#Artificial Intelligence#billion#chatbots#Companies#company culture#courses#deals#development#devices#dialog#digital human#digital humans#email#employee retention#Employee Training#employees#Enterprises#Environment#gartner#generative#generative artificial intelligence#Global#guidelines#hallucinations#healthcare
0 notes
Text

Transforming Healthcare with Intelligent Chatbots
Discover how our cutting-edge chatbot solution is revolutionizing the healthcare sector! With features like automated medication reminders, appointment scheduling, and real-time patient assistance, we are enhancing patient engagement and streamlining operations.
Patients can access medical information and assistance 24/7, ensuring they receive the support they need whenever they need it. By leveraging the power of artificial intelligence, we're revolutionizing the healthcare industry and providing a personalized, seamless experience for patients.
Join the trend towards AI-driven healthcare innovation! Our chatbot not only improves access to medical information but also ensures a personalized experience for every patient.
Website: https://www.vinnovatetechnologies.com/ Book a demo at: [email protected]
Let’s embrace the future of healthcare together!
0 notes
Text
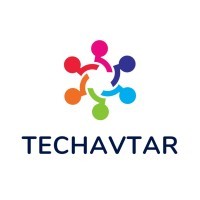
#Top Technology Services Company in India#Generative AI Development Company#AI Calling Software#AI Software Development Company#Best Chatbot Service Company#AI Calling Software Development#AI Automation Software#Best AI Chat Bot Development Company#AI Software Dev Company#Top Web Development Company in India#Top Software Services Company in India#Best Product Design Company in World#Best Cloud and Devops Company#Best Analytic Solutions Company#Best Blockchain Development Company in India#Best Tech Blogs in 2024#Creating an AI-Based Product#Custom Software Development for Healthcare#NexaCalling Best AI Calling Bot#Best ERP Management System#WhatsApp Bulk Sender#Neighborhue Frontend Vercel
0 notes
Text
The Future of AI in Healthcare: Transforming Patient Care and Medical Research
Introduction
The future of AI in healthcare is poised to revolutionize how we approach patient care and medical research. As artificial intelligence technologies continue to advance, their integration into the healthcare sector promises to enhance diagnostics, improve treatment outcomes, and streamline operational processes. In this blog, we will explore the transformative potential of AI in healthcare and its implications for patients, providers, and researchers.
Enhancing Patient Care
One of the most significant impacts of AI in healthcare is its ability to enhance patient care. AI-powered tools are being developed to assist healthcare providers in diagnosing diseases more accurately and quickly. For example, machine learning algorithms can analyze medical imaging data, such as X-rays and MRIs, to identify conditions like tumors and fractures with remarkable precision. This not only leads to earlier diagnoses but also allows for timely interventions that can save lives.
Moreover, AI can personalize treatment plans based on individual patient data. By analyzing a patient’s genetic information, medical history, and lifestyle factors, AI systems can recommend tailored therapies that are more effective and have fewer side effects. This approach, known as precision medicine, represents a significant shift from the traditional one-size-fits-all model, empowering healthcare providers to deliver more effective care.
Streamlining Operations
In addition to improving patient outcomes, AI is also transforming the operational side of healthcare. Administrative tasks, such as scheduling appointments and managing patient records, can be automated through AI-driven systems. This not only reduces the burden on healthcare staff but also minimizes the risk of errors, ensuring that patients receive timely and coordinated care.
AI chatbots and virtual assistants are becoming increasingly popular in healthcare settings, providing patients with immediate responses to their inquiries and guiding them through administrative processes. This enhances patient engagement and satisfaction while freeing up healthcare professionals to focus on more complex tasks.
Advancements in Medical Research
The future of AI in healthcare also holds immense promise for medical research. AI algorithms can analyze vast amounts of data from clinical trials, electronic health records, and genomic studies, uncovering patterns and insights that would be impossible for humans to detect. This capability accelerates the drug discovery process, enabling researchers to identify promising candidates more quickly and efficiently.
Furthermore, AI can facilitate the development of new therapies by simulating how drugs interact with biological systems. This not only shortens the research timeline but also reduces costs, ultimately bringing life-saving treatments to patients faster.
Ethical Considerations
Despite the numerous benefits of AI in healthcare, it is essential to address the ethical considerations that accompany its adoption. Issues related to data privacy, algorithmic bias, and the need for transparency in AI decision-making must be prioritized to ensure that these technologies are used responsibly. Establishing clear guidelines and regulatory frameworks will be crucial in navigating these challenges and fostering trust among patients and providers.
Conclusion
In conclusion, the future of AI in healthcare is bright, with the potential to transform patient care and medical research dramatically. From enhancing diagnostic accuracy and personalizing treatments to streamlining operations and accelerating research, AI technologies are set to redefine the healthcare landscape. However, it is crucial to address the ethical implications of these advancements to ensure that AI serves as a force for good in the healthcare sector. As we embrace this technological evolution, the ultimate goal remains clear: to improve health outcomes and enhance the quality of care for patients worldwide.
0 notes
Text
Embrace the Future of Healthcare. Experience the benefits of generative AI in healthcare with Botgo. Discover more at botgo.io and see how we’re driving innovation in patient care! 𝐒𝐰𝐢𝐭𝐜𝐡 𝐭𝐨 𝐁𝐨𝐭𝐠𝐨 𝐍𝐨𝐰! 𝗖𝐨𝐧𝐭𝐚𝐜𝐭 𝐮𝐬 𝐭𝐨𝐝𝐚𝐲 𝐟𝐨𝐫 𝐚 𝐟𝐫𝐞𝐞 𝟔𝟎 𝐝𝐚𝐲𝐬 𝐭𝐫𝐢𝐚𝐥, 𝐃𝐞𝐦𝐨 & 𝐐𝐮𝐨𝐭𝐞𝐬! 𝗙𝗼𝗿 𝗺𝗼𝗿𝗲 𝗶𝗻𝗳𝗼𝗿𝗺𝗮𝘁𝗶𝗼𝗻:👇 🌐𝗩𝗶𝘀𝗶𝘁 𝗨𝘀: https://botgo.io
0 notes
Text
#chat#chatbot#communication#conversational ai#ai agents#ai agents for business#conversational ai vendor#conversational ai in dubai#conversational ai for healthcare
0 notes
Text
Ensuring Data Security and Privacy in AI Healthcare Chatbots
The adoption of technology like AI healthcare chatbots is rising swiftly. These chatbots can significantly improve care delivery, making processes more efficient and helping patients manage their health better. However, securing personal health information becomes crucial as we rely more on AI for healthcare services.
In this blog, we discuss simple, effective ways to ensure data security and privacy in chatbot development for healthcare.
Why is Data Security Important in AI Healthcare Chatbots?
AI healthcare chatbots handle sensitive data, including medical histories, personal health information, and other confidential data that patients share during interactions. This data is highly sensitive, and any breach could lead to significant privacy violations and other consequences for patients. Therefore, maintaining the highest level of security and privacy is not just a technical requirement but a moral imperative.
Essential Measures for Securing AI Healthcare Chatbots
Implement Strong Authentication and Authorization Procedures
One of the first steps in securing a chatbot development for healthcare is to ensure that access to the chatbot and its data is tightly controlled. Use strong authentication mechanisms to verify the identity of chatbot users. Additionally, implementing role-based access controls can ensure that individuals only access the data necessary for their role.
Use Data Encryption
Encrypting data is crucial. All data, whether at rest or in transit, should be encrypted. This means that even if data is intercepted, it cannot be read without the decryption key. Using strong, up-to-date encryption protocols can significantly reduce the risk of data breaches.
Ensure Compliance with Regulations
AI healthcare chatbots must comply with health information privacy regulations such as HIPAA in the United States or GDPR in Europe. These regulations provide a framework for data protection that includes assessing risks, implementing security measures, and ensuring transparency in data processing activities.
Regular Security Audits and Vulnerability Assessments
Conduct regular security audits and vulnerability assessments to identify and address potential security weaknesses in the chatbot system. This proactive approach helps in spotting issues before malicious parties can exploit them.
Provide Data Privacy Training
Human error is a significant security risk. Providing regular training on data privacy best practices to everyone involved in the development and management of AI healthcare chatbots can reduce this risk. Training should cover secure data handling, recognising phishing attempts, and other relevant security topics.
Implement Secure Development Practices
Secure development practices should be a priority from the initial design to the deployment and maintenance of AI healthcare chatbots. This includes regular software updates, stringent testing for security vulnerabilities, and the use of secure coding practices.
Conclusion
As AI's role in healthcare continues to expand, ensuring the security and privacy of data within AI healthcare chatbots is more critical than ever. Implementing strong security measures and adhering to best practices in chatbot development for healthcare can protect sensitive patient information from potential threats.
By fostering a secure and trustworthy environment, healthcare providers can maximise the benefits of AI healthcare chatbots, enhancing patient care without compromising data privacy.
1 note
·
View note
Text
Hanooman AI model for Indian languages unveiled
Mumbai: Seetha Mahalaxmi Healthcare (SML), in collaboration with the IIT Bombay-led BharatGPT initiative, has introduced ‘Hanooman AI,’ a suite of Indic large language models proficient in 22 Indian languages. Supported by Mukesh Ambani’s Reliance Industries and other engineering institutions across India, the consortium is gearing up to launch its inaugural ChatGPT-style service, BharatGPT, next month.
Led by IIT Bombay and supported by seven other IITs, the BharatGPT ecosystem enjoys backing from the Department of Science and Technology, SML, and Reliance Jio.
Read More - https://apacnewsnetwork.com/2024/02/hanooman-ai-model-for-indian-languages-unveiled/
#ai hanooman#AI model for Indian#ai model hanooman#BharatGPT#bharatgpt hanooman#ChatGPT-style service#Hanooman#hanooman ai#hanooman ai model#Hanooman AI model for Indian languages#hanooman bharatgpt#hanooman chatbot#IIT Bombay-led BharatGPT#Mukesh Ambani's Reliance Industries#Seetha Mahalaxmi Healthcare
0 notes
Text
Scrubs and Stadiums
This month’s episode of “News You Can Use” on HealthcareNOWRadio features news from the month of February 2024 News You Can Use with your Hosts Dr Craig Joseph and Dr Nick van Terheyden The show that gives you a quick insight into the latest news, twists, turns and debacles going on in healthcare withmy friend and co-host Craig Joseph, MD (@CraigJoseph) Chief Medical Officer at Nordic Consulting…
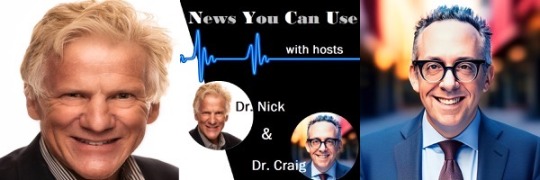
View On WordPress
#AI#Ask Me Anything#Bigdata#Blockchain#ChatBots#connectedhealth#Cybersecurity#Digital Health#DigitalHealth#disruption#education#ehealth#ehrs#EMR#fraud#FutureofHealthcare#futurism#HCIT#Healthcare#Healthcare Reform#healthcarecosts#healthdata#HealthIT#healthtech#HIT#hospitals#Incremental#Incremental Healthcare#IncrementalHealth#Innovation
1 note
·
View note
Text
Revolutionizing Patient Education with “X-Plain Health AI”
Imagine a world where every patient's educational journey is uniquely tailored to their health needs, language, and learning style. This is not a distant dream but a present reality, thanks to the groundbreaking advancements in AI with “X-Plain Health AI,” a custom GPT app redefining the landscape of patient education. How is this innovative technology transforming how we understand and interact with healthcare information? Let's explore the future, which is already here.
What are Custom GPTs? Custom GPTs are a specialized iteration of the Generative Pre-trained Transformer models designed specifically for targeted applications. Unlike general-purpose models like ChatGPT, custom GPTs are fine-tuned with specific datasets, tailored instructions, and integrated functionalities to cater to particular needs. This specialization enables them to produce outputs that are highly adaptive and aligned with specific objectives, making them invaluable in fields like patient education.
Traditional vs. AI-based Patient Education
In traditional settings, patient education relies on printed materials, videos, and digital resources, which often fall short in addressing the diverse needs of patients. These methods, while informative, lack the personalization necessary for effective healthcare communication. Custom GPTs revolutionize this domain by offering tailored, dynamic, and interactive educational content. This AI-driven approach aligns with individual health conditions, literacy levels, and cultural backgrounds, enhancing patient understanding, engagement, and adherence to medical guidance.
Consider the case of Laila, a 52-year-old woman recently diagnosed with type 2 diabetes. She's overwhelmed with the flood of generic information available online. That's where 'X-Plain Health AI' comes in. Unlike traditional GPT models like ChatGPT, our custom GPT is fine-tuned with vast medical data. When Laila uses X-Plain Health AI, she receives personalized information. The system adjusts the complexity of the language to match her understanding and even considers her cultural background. This means Laila gets tailored advice on managing her diabetes, dietary recommendations suited to her lifestyle, and explanations of medical terms in a way she can easily comprehend. It's like having a virtual health educator, always ready to provide information that’s specifically relevant to her, rather than a one-size-fits-all approach.
X-Plain Health AI
Custom GPTs for patient education can generate detailed, specific information about a patient's condition and treatment options, adjust the complexity of the language to suit the patient's literacy level, facilitate interactive learning sessions for heightened engagement, and offer materials in multiple languages, considering cultural nuances.
Released on February 5, 2024, X-Plain Health AI is a pioneering custom GPT model developed by the Patient Education Institute. Accessible through the ChatGPT Store, it promises unprecedented personalization in patient education. What does “X-Plain Health AI” offer beyond what ChatGPT can do? Some key features include:
Prompting users to set their preferences for language, content depth, difficulty level, and other parameters.
Ask users to select a health topic, then offer targeted questions to refine information to specific interests like causes, symptoms, alternative treatments, risks, coping strategies, support options, and more.
Adjusting the complexity of the text according to the user’s preferences and previous interactions.
Engage with users to explain complex terms, present statistics, or compare treatment options tailored to their specific requests.
Asking questions to verify understanding.
Using our extensive database of peer-reviewed, up-to-date content, which includes over 25 million words, a video library with over 2,500 videos, and 2,500 interactive quizzes for comprehension testing, ensuring that users receive up-to-date information in their preferred educational format.
Upon the user’s request, display one of our +10,000 illustrations and +100,000 slides to show diagrams and anatomical and procedural illustrations (in progress).
Here is a scenario illustrating how X-Plain Health AI interacts with a user: John, a 40-year-old man, logs into X-Plain Health AI.
— X-Plain Health AI: "Hello! How may I assist you today? Do you have any specific health topics or questions in mind? Also, would you like me to address you by a name?”
— John: “You can call me John. I want to learn about managing high blood pressure.”
— X-Plain Health AI: “Welcome, John! To provide you with personalized information about managing high blood pressure, I have a few questions for you. First, what is your preferred language for communication?"
— John: "English, please."
— X-Plain Health AI: "Great, English it is. How would you rate your understanding of high blood pressure management on a scale from 1 to 5?"
— John: "Probably around a 2."
— X-Plain Health AI: "Understood, we'll keep things straightforward. Are you seeking information on causes, symptoms, complications, diet, exercise, medication, or all of these topics?"
— John: "Mostly diet and exercise."
— X-Plain Health AI: "Okay. I can provide dietary guidelines and exercise tips that effectively manage high blood pressure. Before we proceed, do you have any dietary restrictions or physical limitations I should be aware of?"
— John: "I'm a vegetarian and have a knee injury, so nothing too strenuous."
— X-Plain Health AI: "Thanks for sharing that, John. I'll tailor the information accordingly. [Provides tailored advice on a vegetarian diet and low-impact exercises suitable for someone with a knee injury]. Would you like me to ask a few questions to ensure you've understood the information clearly?"
— John: "Yes, that would be helpful."
— X-Plain Health AI: "Alright, let's start with a simple one: Can you name two vegetables that are particularly beneficial for managing high blood pressure?"
— John: [Responds with his answers]
— X-Plain Health AI: "That's correct! [Provides feedback on his answers]. Let’s try another question to see how you’re doing with the exercise recommendations."
Challenges and Considerations
The deployment of custom GPTs in patient education presents challenges, particularly in privacy and information accuracy. Ensuring compliance with healthcare privacy laws, such as HIPAA, and maintaining the accuracy of the information provided by these AI models are of utmost importance. It's crucial to continually remind users that AI-generated content should be seen as supplementary to professional medical advice, not a replacement.
In OpenAI's custom GPT environment, users have control over their data. If they permit ChatGPT to learn from their interactions, the chats with custom GPTs remain private and are not shared with the creators. A key challenge is ensuring GPT models adhere to healthcare privacy laws like HIPAA. ChatGPT and custom GPT models consistently remind users not to share any personally identifiable information during conversations. Users also have the option to adjust their settings to prevent ChatGPT from retaining their chat history. However, these settings may not be straightforward and could be inadvertently altered during updates or when opting into new services. In addition, the field is rapidly developing, and so are OpenAI’s privacy and data training policies.
Two years following the launch of X-Plain in 1995, we established a clear distinction between Clinical X-Plain and Public X-Plain. Clinical X-Plain serves a crucial role in clinical environments, offering informed consent, discharge instructions, and specific guidance on medications and home care directly to patients. In contrast, Public X-Plain is a patient education resource available on healthcare institutions' websites, assisting patients in learning about and preparing for clinical visits. In this context, X-Plain Health AI is envisioned as a public-facing tool accessible online to aid patients in understanding and preparing for healthcare encounters. It is distinct from the discharge documents that healthcare providers typically hand to patients following outpatient, inpatient, or emergency room visits.
Monetization
The introduction of the GPT Store by OpenAI marks a significant shift in the landscape of AI application development. Like an app store for AI technologies, this platform allows creators to publish and potentially monetize their GPT models. Although still in its infancy, the revenue-sharing model indicates a promising avenue for creators to benefit financially from their innovative AI solutions in healthcare.
What do we know so far? OpenAI covers the cost of tokens for GPTs in the OpenAI GPT Store. This means neither the user nor the creator of a custom GPT bears the token cost. However, access to the GPT Store and its features is limited to ChatGPT Plus Subscribers and OpenAI Enterprise customers.
The monetization model for OpenAI's GPT Store is still unspecified and vague. OpenAI states that it will involve revenue sharing with creators, where creators are paid based on the usage and utility of their custom GPTs. Initially, the revenue model may start with a straightforward revenue share, the specifics of which are yet to be detailed. Later, there might be options for subscription-based access to individual GPTs, depending on demand.
These monetization policies are dynamic and tentative; for the most current monetization models, check OpenAI's official website.
Our patient education solutions are exclusively licensed to healthcare institutions and stakeholders, not directly to patients. However, with OpenAI's proposed model for monetization, we are poised to indirectly offer X-Plain Health AI to patients and individual users for the first time, a departure from our traditional approach of licensing only to healthcare service providers. Given the importance of ensuring accuracy and privacy, we will maintain X-Plain Health AI as a public patient education tool rather than a clinical one until these critical factors are fully guaranteed.
The Future
Looking ahead, the potential of AI in patient education is immense. Future developments in custom GPTs are expected to include a deeper understanding of language nuances, more sophisticated bias mitigation techniques, and enhanced integration with other applications.
Regarding its integration with other applications, future custom GPT models for patient education will offer capabilities such as:
Seamlessly combining several aspects of patient care, including treatment planning, patient education, and follow-up care.
Dynamically adapting educational content in real-time based on the user's preferences, interaction style, and updates in their health records.
Utilize predictive analytics to identify potential health risks and deliver tailored educational content to patients accordingly.
Enhancing patient engagement and understanding using augmented reality in educational materials.
Final Notes
As custom AI models like X-Plain Health AI evolve, it’s crucial to steer their integration and governance in patient engagement proactively. To ensure these advancements serve the best interests of all involved, consider the following:
Healthcare thought leaders should commit to ongoing research and develop oversight processes. Regular evaluations of custom GPTs for accuracy, privacy protection, and clinical effectiveness are essential. This continuous monitoring facilitates responsible innovation that truly benefits patients.
Policymakers must act promptly to establish data governance laws specifically for healthcare AI. These regulations should balance patient rights protection with the encouragement of empowering innovations.
Patients should be encouraged to learn about and use AI-powered educational tools. They need to recognize that, while valuable, these tools are not replacements for professional medical advice.
By fostering frameworks that promote accountability in tandem with technological advancement, we can revolutionize how individuals learn about and manage their health.
As we stand on the brink of a new healthcare age, X-Plain Health AI represents a pivotal shift from one-size-fits-all to a world where patient education is as adapted as a tailored suit.
#AI-in-Patient-Education#Custom-GPT-for-Healthcare#X-Plain-Health-AI#Interactive-Patient-Education#Patient-Engagement#Patient-Empowerment#Patient-Education-Chatbots#Adaptive-Learning
0 notes
Text
Ambience Healthcare secures $70M in funding for its AI assistant with OpenAI and Kleiner Perkins at the helm
- By InnoNurse Staff -
Ambience Healthcare, a startup bridging artificial intelligence and healthcare, has secured $70 million in funding to expand its "operating system" for healthcare organizations, focusing primarily on outpatient medical services in the U.S.
Major backers include Kleiner Perkins, OpenAI’s Startup Fund, Andreessen Horowitz, and Optum Ventures. The company, founded by Michael Ng and Nikhil Buduma, aims to streamline administrative tasks for clinicians through products like AutoScribe and AutoCDI, catering to various ambulatory specialties while aiming to eventually incorporate diagnosis capabilities, amidst a competitive landscape in AI-driven healthcare solutions.
Read more at TechCrunch
#Ambience Healthcare#primary care#health tech#health it#digital health#ai#usa#openai#chatbot#medtech#outpatient
0 notes
Text
5 Challenges of AI in Healthcare
New Post has been published on https://thedigitalinsider.com/5-challenges-of-ai-in-healthcare/
5 Challenges of AI in Healthcare
Imagine a world where your smartwatch not only tracks your steps but also predicts a heart attack before it happens. It’s closer to reality than you think.
Artificial intelligence (AI) integration in healthcare has begun, unlocking many use cases for healthcare providers and patients. The AI healthcare software and hardware market is expected to surpass $34 billion by 2025 globally.
Among the technology and processes indicative of these investments in healthcare include:
Robotic nurses to aid surgeons.
Wearables for real-time health monitoring.
Medical AI chatbots for enhanced self-care.
Predictive diagnosis based on existing health symptoms.
However, these applications also come with complex challenges. This blog will explore the five challenges in implementing AI in healthcare, their solutions, and their benefits.
Challenges of Using AI in Healthcare
Physicians, doctors, nurses, and other healthcare providers face many challenges integrating AI into their workflows, from displacement of human labor to data quality issues.
1. Displacement of Human Employees
There is a growing concern that AI could replace healthcare professionals, including job displacement, an outdated skillset, and mental and financial hardships. This potential shift may deter medical groups from adopting AI, causing them to forego many benefits.
The challenge lies in balancing the integration of AI for routine tasks and retaining human expertise for complex patient care, where empathy and critical thinking are irreplaceable.
2. Ethical and Privacy Issues
Obtaining informed consent from patients on how AI systems will use their data can be complex, especially when the public does not fully understand the underlying logic. Some providers might also disregard ethics and use patient data without permission.
Additionally, biases in training data could result in unequal treatment suggestions or misdiagnosis. This discrepancy can disproportionately affect vulnerable groups.
For example, an algorithm that predicts which patients need more intensive care based on healthcare costs rather than actual illness. This incorrectly attributed a lower disease burden to black people.
Furthermore, AI’s ability to identify individuals through large amounts of genome data, even when personal identifiers are removed, poses a risk to patient confidentiality.
3. Lack of Digital Training and Adoption Barriers
A major problem is that medical students receive insufficient training on AI tools and theory. This unpreparedness makes adopting AI difficult during their internships and work.
Another significant barrier is the reluctance of some individuals to embrace digital technologies. Many people still prefer traditional, in-person consultations due to multiple reasons, such as:
The relatable nature of human interactions.
Uniqueness neglect by AI.
The higher perceived value of human doctors, etc.
This resistance is often compounded by a general lack of awareness about AI and its potential benefits, particularly in developing countries.
4. Professional Liabilities
The use of AI systems in decision-making introduces new professional liabilities for healthcare providers, raising questions about ownership if AI initiatives are ineffective. For example, doctors can defer treatment plans to AI without taking responsibility for failed patient examinations.
Furthermore, while machine learning (ML) algorithms can offer personalized treatment recommendations, the lack of transparency in these algorithms complicates individual accountability.
Additionally, reliance on AI could lead to complacency among healthcare professionals, who might defer to computerized decisions without applying their clinical judgment.
5. Interoperability Problems and Data Quality Issues
Data from different sources can often fail to integrate seamlessly. Inconsistency in data formats across systems makes it difficult to access and process information efficiently, creating information silos.
Moreover, poor data quality—such as incomplete or inaccurate records—can lead to flawed AI analysis, ultimately compromising patient care.
Considering these challenges, how can healthcare organizations leverage the full potential of AI?
Solutions to Healthcare AI Problems
Solving the challenges introduced by AI involves a top-down approach. It begins with ensuring that data analysts thoroughly vet datasets used to train AI algorithms to eliminate biases and low-quality data. Transparency with patients regarding AI’s role in their treatment is also crucial to increase adoption.
An example is the Mayo Clinic, which used an algorithm that analyzed over 60,000 images to detect pre-cancerous signs. The algorithm’s accuracy was 91% compared to a human expert’s.
Apart from fixing old datasets, health regulatory bodies, such as the European Medicines Agency (EMA), must collect new, error-free data representing diverse populations to enhance accuracy. OpenAPS is an example of an initiative to create an inclusive open-source collection of systems to treat type 1 diabetes accurately.
Additionally, hospitals should enhance training and education for healthcare professionals. Educational authorities can also extend this specialized training to universities to prepare future practitioners.
This initiative will ensure familiarity with and expertise in AI tools and reduce resistance to their adoption in a professional setting. For example, Intuitive Surgical Ltd’s investment in the da Vinci system has helped doctors in over 5 million surgeries.
Investing in modern data integration tools, such as Astera and Fivetran, with built-in data quality features will also help. These tools remove siloed data and improve interoperability. They also enable data validation to ensure AI algorithms have clean data to analyze.
To effectively integrate AI systems into healthcare, medical institutions must balance leveraging AI and preserving human expertise. Adopting hybrid approaches like human-in-the-loop (HITL) models can help alleviate fears of job displacement. This approach will also ease patient concerns about AI involvement while allowing workers to improve productivity.
And, what are the benefits of successful AI integration within healthcare?
Benefits of AI in Healthcare
AI provides many benefits in the healthcare industry, including improved diagnosis and higher work efficiency:
1. Enhanced Diagnostic Accuracy
AI is transforming diagnostic processes by rapidly analyzing medical images, lab results, and patient data with remarkable precision. This ability to process large amounts of information quickly leads to early, potentially more accurate diagnoses, improving disease management.
2. Personalized Treatment Plans
AI-powered deep learning algorithms can process extensive datasets to create personalized treatment plans tailored to individual patients. This customization improves the efficacy of treatments and minimizes side effects by addressing each patient’s specific needs based on extensive sample data.
3. Operational Efficiency
By automating administrative tasks such as scheduling appointments and billing, AI allows healthcare providers to spend more time and effort on direct patient care. This shift reduces the burden of routine tasks, cuts costs, streamlines operations, and improves overall efficiency.
4. Improved Patient Monitoring
AI-powered tools, including wearable devices, offer continuous patient monitoring, providing real-time alerts and insights. For example, these devices can alert medical services in case of an unusually high heartbeat, which could indicate a physical injury or heart condition.
This proactive approach enables healthcare providers to respond swiftly to changes in a patient’s condition, improving disease management and overall patient care.
Looking Ahead
Emerging technologies, like virtual reality (VR) in medicine, will play a critical role. Many healthcare tasks, from diagnostics to treatment, will be AI-powered, enhancing access to care patient outcomes.
However, healthcare authorities must balance AI’s benefits and challenges to ensure ethical and effective integration into patient care. This will transform the healthcare delivery systems in the long term.
Explore Unite.ai for more resources on AI and healthcare.
#000#ai#AI chatbots#AI in healthcare#AI integration#AI systems#ai tools#AI-powered#alerts#algorithm#Algorithms#Analysis#applications#approach#artifical intelligence#artificial#Artificial Intelligence#awareness#barrier#billion#Blog#challenge#chatbots#continuous#data#Data Integration#data integration tools#data quality#data validation#datasets
1 note
·
View note
Text

AI in Healthcare: Uses, Examples, & Benefits
The integration of AI in healthcare is transforming the industry, offering unprecedented opportunities to enhance patient care, improve diagnostic accuracy, and streamline operations. From medical imaging and personalized treatment plans to virtual health assistants and drug discovery, AI is driving innovation and efficiency across all aspects of healthcare.
As AI technology continues to evolve, its applications in healthcare will expand, providing even more tools to support medical professionals and improve patient outcomes. Embracing AI in healthcare is not just about adopting new technologies; it’s about reimagining the future of medicine and unlocking its full potential to benefit patients worldwide.
youtube
#ai#AI in Healthcare#Hosptals#Medical#Medical innovation#Healthcare Techonology#Chatbot#OCR Technology#Generative AI#Youtube
0 notes