#Randomized Algorithms in Python
Explore tagged Tumblr posts
Text
Day-4: Unlocking the Power of Randomization in Python Lists
Python Boot Camp 2023 - Day-4
Randomization and Python List Introduction Randomization is an essential concept in computer programming and data analysis. It involves the process of generating random elements or sequences that have an equal chance of being selected. In Python, randomization is a powerful tool that allows developers to introduce an element of unpredictability and make programs more dynamic. This article…
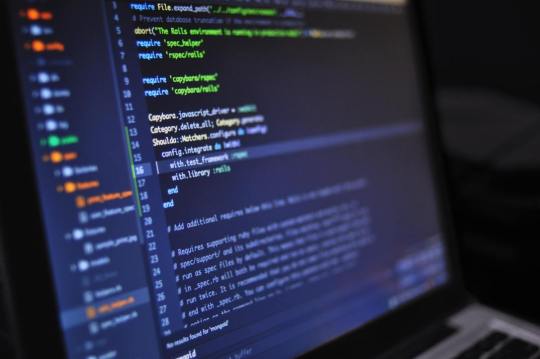
View On WordPress
#Advantages of Randomization in Programming#Dynamic Python Applications#Enhancing User Experience with Randomization#Generating Random Data in Python#How to Shuffle Lists in Python#Python List Data Structure#Python List Manipulation#Python programming techniques#Random Element Selection in Python#Randomization in Python Lists#Randomized Algorithms in Python#Secure Outcomes with Randomization#Unbiased Outcomes in Python#Understanding Non-Deterministic Behavior#Versatility of Randomization in Python
0 notes
Text
I desprately need someone to talk to about this
I've been working on a system to allow a genetic algorithm to create DNA code which can create self-organising organisms. Someone I know has created a very effective genetic algorithm which blows NEAT out of the water in my opinion. So, this algorithm is very good at using food values to determine which organisms to breed, how to breed them, and the multitude of different biologically inspired mutation mechanisms which allow for things like meta genes and meta-meta genes, and a whole other slew of things. I am building a translation system, basically a compiler on top of it, and designing an instruction set and genetic repair mechanisms to allow it to convert ANY hexadecimal string into a valid, operable program. I'm doing this by having an organism with, so far, 5 planned chromosomes. The first and second chromosome are the INITIAL STATE of a neural network. The number and configuration of input nodes, the number and configuration of output nodes, whatever code it needs for a fitness function, and the configuration and weights of the layers. This neural network is not used at all in the fitness evaluation of the organism, but purely something the organism itself can manage, train, and utilize how it sees fit.
The third is the complete code of the program which runs the organism. Its basically a list of ASM opcodes and arguments written in hexadecimal. It is comprised of codons which represent the different hexadecimal characters, as well as a start and stop codon. This program will be compiled into executable machine code using LLVM IR and a custom instruction set I've designed for the organisms to give them a turing complete programming language and some helper functions to make certain processes simpler to evolve. This includes messages between the organisms, reproduction methods, and all the methods necessary for the organisms to develop sight, hearing, and recieve various other inputs, and also to output audio, video, and various outputs like mouse, keyboard, or a gamepad output. The fourth is a blank slate, which the organism can evolve whatever data it wants. The first half will be the complete contents of the organisms ROM after the important information, and the second half will be the initial state of the organisms memory. This will likely be stored as base 64 of its hash and unfolded into binary on compilation.
The 5th chromosome is one I just came up with and I am very excited about, it will be a translation dictionary. It will be 512 individual codons exactly, with each codon pair being mapped between 00 and FF hex. When evaulating the hex of the other chromosomes, this dictionary will be used to determine the equivalent instruction of any given hex pair. When evolving, each hex pair in the 5th organism will be guaranteed to be a valid opcode in the instruction set by using modulus to constrain each pair to the 55 instructions currently available. This will allow an organism to evolve its own instruction distribution, and try to prevent random instructions which might be harmful or inneficient from springing up as often, and instead more often select for efficient or safer instructions.
#ai#technology#genetic algorithm#machine learning#programming#python#ideas#discussion#open source#FOSS#linux#linuxposting#musings#word vomit#random thoughts#rant
8 notes
·
View notes
Text
Tuesday 28th February '23
- JavaScript
I’m currently using Freecodecamp’s ‘JavaScript Algorithms and Data Structures’ course to study the basics of JavaScript. I know some JavaScript already, especially from the lessons from the coding night classes, but I kind of rushed through the lessons to just get the homework done and didn’t give myself enough time to sit down and learn the concepts properly! Today I completed 30 lessons and learnt about arrays, box notation, switch, if-else statements and operators!
- Python
Back on Rep.it and I’m continuing the ‘100 days of Python’ and I got up to day 27. I learnt about using the import keyword and modules such as random, os, and time. I think the challenge has us making an adventure game soon!!
- C#
Watches some youtube videos on C# with SQLite projects because I came up with yet another project idea that has to do with using technologies!
>> note: have a nice day/night and good luck with your studies and in life!
#xc: studies#programming studies#codeblr#progblr#studyblr#coding#programming#studying#python#javascript#csharp#study hard#tech#compsci#study notes#python studies#computer science#csharp studies#javascript studies
142 notes
·
View notes
Text
Oldschool determinants & floating points
Forget those easypeasy 2x2, 3x3, and 4x4 (if you're feeling spicy) formulas for matrix determinants that you forced yourself to memorize in linear algebra. Real ones know that you can get the determinant of any matrix through LU decomposition.
Fun fact though, because Python floating point numbers aren't really the same as floating point numbers in C (python does everything with infinite precision), I was getting different answers for determinants I computed with my LU algorithm versus what NumPy was getting with the algorithm it uses from LAPACK.
For instant with a 99x99 random matrix, the absolute difference in our determinants was over 1 trillion.
BUT
Each determinant was on the order of 10^25 (because the matrices were filled with values from a uniform random distribution and to oversimplify these don't really play nice with det algorithms).
This was my value: -1.3562741025533489e+25
This was NumPy's value: -1.3562741025534902e+25
I bolded the digits where they differed in value. The actual percentage difference between them is basically 0, ~10^-10
I'm sure in some applications this would matter, but for me it doesn't really.
2 notes
·
View notes
Text
How do I learn R, Python and data science?
Learning R, Python, and Data Science: A Comprehensive Guide
Choosing the Right Language
R vs. Python: Both R and Python are very powerful tools for doing data science. R is usually preferred for doing statistical analysis and data visualisations, whereas Python is much more general and currently is very popular for machine learning and general-purpose programming. Your choice of which language to learn should consider your specific goals and preferences.
Building a Strong Foundation
Structured Courses Online Courses and Tutorials: Coursera, edX, and Lejhro offer courses and tutorials in R and Python for data science. Look out for courses that develop theoretical knowledge with practical exercises. Practise your skills with hands-on coding challenges using accompanying datasets, offered on websites like Kaggle and DataCamp.
Books: There are enough books to learn R and Python for data science. You may go through the classical ones: "R for Data Science" by Hadley Wickham, and "Python for Data Analysis" by Wes McKinney.
Learning Data Science Concepts
Statistics: Know basic statistical concepts: probability, distribution, hypothesis testing, and regression analysis.
Cleaning and Preprocessing: Learn how to handle missing data techniques, outliers, and data normalisation.
Data Visualization: Expert libraries to provide informative visualisations, including but not limited to Matplotlib and Seaborn in Python and ggplot2 in R.
Machine Learning: Learn algorithms-Linear Regression, Logistic Regression, Decision Trees, Random Forest, Neural Networks, etc.
Deep Learning: Study deep neural network architecture and how to build and train them using the frameworks TensorFlow and PyTorch.
Practical Experience
Personal Projects: In this, you apply your knowledge to personal projects which help in building a portfolio.
Kaggle Competitions: Participate in Kaggle competitions to solve real-world problems in data science and learn from others.
Contributions to Open-Source Projects: Contribute to some open-source projects for data science in order to gain experience and work with other people.
Other Advice
Join Online Communities: Join online forums or communities such as Stack Overflow and Reddit to ask questions, get help, and connect with other data scientists.
Attend Conferences and Meetups: This is a fantastic way to network with similar working professionals in the field and know the latest trends going on in the industry.
Practice Regularly: For becoming proficient in data science, consistent practice is an indispensable element. Devote some time each day for practising coding challenges or personal projects.
This can be achieved by following the above-mentioned steps and having a little bit of dedication towards learning R, Python, and Data Science.
2 notes
·
View notes
Text
Project Introduction: Text Based Monopoly Game.
Look I'm just going to be frank with you, I am not the smartest individual, nor do I have much experience programming, but what I do have is the gall, the absolute nerve to believe that I can do anything even with very little experience. Some call it the Dunning-Kruger Effect, I like to call it a gift from the All Mighty.
This led me to idea of making a text based version of monopoly with about 2 hours worth of python tutorials, absolutely no understanding of data structures and algorithms, and the help of chatgpt.
So far I have already implemented:
Adding, removing, and naming player. With a required minimum of 2 players and cap of 6 players.
Allowing players to declare bankruptcy
Added a win state when there is only one player who is not bankrupt.
Display the player number, name, and current funds.
Random dice rolls.
Allowing players to move within 40 spaces.
Display on which numbered space the player is on the board along with the name of the space that they are located.
Player automatically collect $200 when they pass go.
They can also end their turn.
What I need to implement:
Buy properties, selling properties, and collecting rent.
Morgeting properties
Buying houses
Chance and community cards.
Jail
Trading
View Current Properties
There are probably other things that need to be added for the list but for the moment those are the most present things.
My plan for the text based game is two parts. 1. Getting the game to work. 2. Is migrating and reworking the code into a discord bot which allows users to play this text based version of Monopoly their servers.
I hope to have updates coming steadily. My current focus is on implementing properties but right now I have no idea where to start or how to efficiently do it. So it is still very much a work in progress.
In dev updates going forwards I'm going to be calling the project Textopoly, once the game is in a playable state I will be posting the code over on github along with the discord bot once it is finished.
Tumbler is going to function for mini updates on my project, official and more detailed updates will be posted on my main blog (https://voidcatstudios.blogspot.com/) but those aren't coming anytime soon.
If you have read this far... thank you very much. I'm still very much a noob programmer, but your support means the world and I hope that as I get more experience and knowledge I'm able to make and share more awesome projects with people like you.
Alright then, this has gotten quite long, have a great rest of your day!
10 notes
·
View notes
Text
100 days of code : day 4
(29/03/2023)
Hello, how are you everyone?
Yesterday I started the 4th I studied about the random module but I had an anxiety attack and I didn't finish. (I'm better)
Today I finished the random and we started the array. But there's still a little bit left to finish. And during the afternoon I had several ideas of things I want to learn and I had a slight outbreak because there are so many things and how to organize myself.
But something I want to share is that I don't feel like I learn from Professor Angela, her teaching is not bad and she gives a lot of exercises.
BUT my head feels that something is missing and I know that I don't really think with it, precisely because the answers are easily accessible, which makes it easier to procrastinate or, in a slight error, look for the answer (no, I don't want moralistic advice on how this is wrong, I have a conscience, I'm just sharing my logic)
And why doesn't it seem to me that I'm learning algorithms and data structure, even though today, for example, I've seen array.
So, accessing the free university on github (I'll make a post, but I'll leave a link here too) I found the Brazilian version and saw a course on Introduction to Computer Science with Python and I loved it, because then I feel like I'm going to algorithms and data structure, and it's taught by the best college in my country (my dream included)
And then for me to stop feeling like a fraud and REALLY try hard.
I decided to make my own roadmap (not the official version yet) It will basically be:
Introduction to computer science part 1 and 2
Exercises from the algorithm course in python (I did it last year, but I really want to do it and make an effort this year)
Graphs
Data structure
Object orientation
programming paradigms
Git and GitHub
Clean Code
Design system
Solid
And only after that go back to 100 days (but now managing to do algorithm exercises for example) So then it would be:
100 days of code
django
Apis
Database
Practice projects.
Another thing I wanted to share (but I'll probably talk more about it in another post) is how the pressure/hurry of wanting to get a job is screwing up my studies.
I WILL NOT be able to learn things effectively on the run.
So I talked to myself and decided that this year I'm going to focus on learning as best I can, but without rushing to get a job (I have the privilege of living with my mother and she supports me) and then next year I'll go back to the call center to pay my bills and then look for a job in the area
I want to feel confident in my code, I want to REALLY know what to do and do it well.
But it won't be in a hurry, so I prefer peace to be able to learn in the best way and everything I want than to freak out and not leave the place.
Anyway, if you've read this essay so far I thank you and I wish you well UHEUHEUHEUHUEH
#100daysofcode#pythonforbeginners#pythonprogramming#pythoncode#coding#javascript#software engineer#software development#computerscience#comp sci#computing#computers#algorithms#datastructure#womanintech#woman in stem#study community#studyspo#study hard#studyblr community#studyblog
25 notes
·
View notes
Text
Explaining Complex Models to Business Stakeholders: Understanding LightGBM
As machine learning models like LightGBM become more accurate and efficient, they also tend to grow in complexity, making them harder to interpret for business stakeholders. This challenge arises as these advanced models, often referred to as "black-box" models, provide superior performance but lack transparency in their decision-making processes. This lack of interpretability can hinder model adoption rates, impede the evaluation of feature impacts, complicate hyper-parameter tuning, raise fairness concerns, and make it difficult to identify potential vulnerabilities within the model.
To explain a LightGBM (Light Gradient Boosting Machine) model, it's essential to understand that LightGBM is a gradient boosting ensemble method based on decision trees. It is optimized for high performance with distributed systems and can be used for both classification and regression tasks. LightGBM creates decision trees that grow leaf-wise, meaning that only a single leaf is split based on the gain. This approach can sometimes lead to overfitting, especially with smaller datasets. To prevent overfitting, limiting the tree depth is recommended.
One of the key features of LightGBM is its histogram-based method, where data is bucketed into bins using a histogram of the distribution. Instead of each data point, these bins are used to iterate, calculate the gain, and split the data. This method is efficient for sparse datasets. LightGBM also employs exclusive feature bundling to reduce dimensionality, making the algorithm faster and more efficient.
LightGBM uses Gradient-based One Side Sampling (GOSS) for dataset sampling. GOSS assigns higher weights to data points with larger gradients when calculating the gain, ensuring that instances contributing more to training are prioritized. Data points with smaller gradients are randomly removed, while some are retained to maintain accuracy. This sampling method is generally more effective than random sampling at the same rate.
As machine learning models like LightGBM become more accurate and efficient, they also tend to grow in complexity, making them harder to interpret for business stakeholders. This challenge arises as these advanced models, often referred to as "black-box" models, provide superior performance but lack transparency in their decision-making processes. This lack of interpretability can hinder model adoption rates, impede the evaluation of feature impacts, complicate hyper-parameter tuning, raise fairness concerns, and make it difficult to identify potential vulnerabilities within the model.
Global and Local Explainability:
LightGBM, a tree-based boosting model, is known for its precision in delivering outcomes. However, its complexity can present challenges in understanding the inner workings of the model. To address this issue, it is crucial to focus on two key aspects of model explainability: global and local explainability.
- Global Explainability: Global explainability refers to understanding the overall behavior of the model and how different features contribute to its predictions. Techniques like feature importance analysis can help stakeholders grasp which features are most influential in the model's decision-making process.
- Local Explainability: Local explainability involves understanding how the model arrives at specific predictions for individual data points. Methods like SHAP (SHapley Additive exPlanations) can provide insights into the contribution of each feature to a particular prediction, enhancing the interpretability of the model at a granular level.
Python Code Snippet for Model Explainability:
To demonstrate the explainability of a LightGBM model using Python, we can utilize the SHAP library to generate local explanations for individual predictions. Below is a sample code snippet showcasing how SHAP can be applied to interpret the predictions of a LightGBM model:
```python
# Import necessary libraries
import shap
import lightgbm as lgb
# Load the LightGBM model
model = lgb.Booster(model_file='model.txt') # Load the model from a file
# Load the dataset for which you want to explain predictions
data = ...
# Initialize the SHAP explainer with the LightGBM model
explainer = shap.TreeExplainer(model)
# Generate SHAP values for a specific data point
shap_values = explainer.shap_values(data)
# Visualize the SHAP values
shap.initjs()
shap.force_plot(explainer.expected_value, shap_values[0], data) ,,,
In this code snippet, we first load the LightGBM model and the dataset for which we want to explain predictions. We then initialize a SHAP explainer with the model and generate SHAP values for a specific data point. Finally, we visualize the SHAP values using a force plot to provide a clear understanding of how each feature contributes to the model's prediction for that data point.
Examples of Using LightGBM in Industries
LightGBM, with its high performance and efficiency, finds applications across various industries, providing accurate predictions and valuable insights. Here are some examples of how LightGBM is utilized in different sectors:
1. Finance Industry:
- Credit Scoring: LightGBM is commonly used for credit scoring models in the finance sector. By analyzing historical data and customer behavior, financial institutions can assess creditworthiness and make informed lending decisions.
- Risk Management: LightGBM helps in identifying and mitigating risks by analyzing market trends, customer data, and other relevant factors to predict potential risks and optimize risk management strategies.
2. Healthcare Industry:
- Disease Diagnosis: LightGBM can be employed for disease diagnosis and prognosis prediction based on patient data, medical history, and diagnostic tests. It aids healthcare professionals in making accurate and timely decisions for patient care.
- Drug Discovery: In pharmaceutical research, LightGBM can analyze molecular data, drug interactions, and biological pathways to accelerate drug discovery processes and identify potential candidates for further testing.
3. E-commerce and Retail:
- Recommendation Systems: LightGBM powers recommendation engines in e-commerce platforms by analyzing user behavior, purchase history, and product preferences to provide personalized recommendations, enhancing user experience and increasing sales.
- Inventory Management: By forecasting demand, optimizing pricing strategies, and managing inventory levels efficiently, LightGBM helps e-commerce and retail businesses reduce costs, minimize stockouts, and improve overall operational efficiency.
4. Manufacturing and Supply Chain:
- Predictive Maintenance: LightGBM can predict equipment failures and maintenance needs in manufacturing plants by analyzing sensor data, production metrics, and historical maintenance records, enabling proactive maintenance scheduling and minimizing downtime.
- Supply Chain Optimization: LightGBM assists in optimizing supply chain operations by forecasting demand, identifying bottlenecks, and streamlining logistics processes, leading to cost savings and improved supply chain efficiency.
5. Marketing and Advertising:
- Customer Segmentation: LightGBM enables marketers to segment customers based on behavior, demographics, and preferences, allowing targeted marketing campaigns and personalized messaging to enhance customer engagement and retention.
- Click-Through Rate Prediction: In digital advertising, LightGBM is used to predict click-through rates for ad placements, optimize ad targeting, and maximize advertising ROI by showing relevant ads to the right audience.
These examples illustrate the versatility and effectiveness of LightGBM in addressing diverse challenges and driving value across industries. By leveraging its capabilities for predictive modeling, optimization, and decision-making, organizations can harness the power of LightGBM to gain a competitive edge and achieve business objectives efficiently.
By leveraging tools like SHAP, data scientists can enhance the explainability of complex models like LightGBM, enabling better communication with business stakeholders and fostering trust in the model's decision-making process.
In the era of advanced machine learning models, achieving model explainability is crucial for ensuring transparency, trust, and compliance with regulatory requirements. By employing techniques like SHAP and focusing on global and local explainability, data scientists can bridge the gap between complex models like LightGBM and business stakeholders, facilitating informed decision-making and fostering a deeper understanding of the model's inner workings.
In summary, LightGBM is a powerful machine learning algorithm that leverages gradient boosting and decision trees to achieve high performance and efficiency in both classification and regression tasks. Its unique features like leaf-wise tree growth, histogram-based data processing, exclusive feature bundling, and GOSS sampling contribute to its effectiveness in handling complex datasets and producing accurate predictions.
2 notes
·
View notes
Text
Chinese regulations require that approved map service providers in China use a specific coordinate system, called GCJ-02 (colloquially Mars Coordinates). Baidu Maps uses yet another coordinate system - BD-09, which seems to be based on GCJ-02.
GCJ-02 (officially Chinese: 地形图非线性保密处理算法; lit. 'Topographic map non-linear confidentiality algorithm') is a geodetic datum used by the Chinese State Bureau of Surveying and Mapping (Chinese: 国测局; pinyin: guó-cè-jú), and based on WGS-84. It uses an obfuscation algorithm which adds apparently random offsets to both the latitude and longitude, with the alleged goal of improving national security.
[...]
Despite the secrecy surrounding the GCJ-02 obfuscation, several open-source projects exist that provide conversions between GCJ-02 and WGS-84, for languages including C#, C, Go, Java, JavaScript, PHP, Python, R, and Ruby.
lol
2 notes
·
View notes
Text
I was making this long ass post (under the cut) asking for help with a homework problem (u guys are my last resort sometimes I swear) where I needed to find the max value of a list, then the next, then the next, etc., without removing elements from the list (because I needed them to coincide with another list. It's a whole thing don't even worry about it), and I didn't know what to DO. I'd been working on this since yesterday...
& then suddenly I go "Well if I can't remove it I can update it so it'll be the lowest no matter what" So in the code, instead of going "REMOVE THIS PLEASE", I go "you are worth nothing now" and set the previous max value to 0 (the values would range from 1.0 to 7.0) and BAM it worked. IT FUCKING WORKED!!!!!!!!!!!!!!! I feel like that gif of a bunch of office guys throwing papers in the air and celebrating and hugging each other except I'm just one guy. Thank u God for my random moments of lucidity <3333
If anyone knows Python and can help:
(Preface that even if u don't know Python / what I'm talking about BUT you read this and have even a vague idea of how to do it, I'd really appreciate ur input OK THX)
Ok so I have to make a program that:
Takes a number n (amount of students) (1)
Takes a name and three grades per student (2)
Calculates each student's average score (3)
Shows the names of the students from highest average to lowest average (4)
I have 1 thru 3 done, and I did them by creating a big list called "school", where I put each "student". Each "student" is also a list containing their name and their three grades. I did it this way so I could reference their names for later.
Then I created another list, called "average", and for each person in the "school", I calculated their average and added them one by one to the "average" list.
After that I made a list called "names", and now I have to check what the max value of the "average" list is. I use the max() function to do this, then grab the index of said max value, which corresponds to the position of the person in the "school" list. Then I add the name of the positions from the "names" list (by doing names.append(school[ind][0]))(ind = index of max value, 0 = name position).
Then, in order for the max value to not be the same all the time I remove said value from the list. So if my "average" list is: [5.0, 6.0, 5.0], and I remove the highest value (6.0), I am left with [5.0, 5.0]. As u can see, this makes it so that the algorithm (?) only works one time, because after that, the list is updated and the positions from the "average" list no longer coincide with the positions from the "school" list.
So I need to find a way to calculate the max value from the "average" list, and then ignore said value in order to find 2nd greatest, 3rd greatest, etc. and then find the position of the value so I can correspond it with the person's name in the "school" list.
If anyone is still here & knows even a semblance of wtf I should do PLEAAAAAAASE tell me!!!!!!!
#I'M SO HAPPY FR. WIN#U can even notice how I was trying to order it in my brain when typing the originial draft (the readmore)...........
3 notes
·
View notes
Text
VE477 Lab 7
Unless specified otherwise, all the programs are expected to be completed in Python or O’caml. In this lab we want to compare three search algorithms. Let A be an array of size n and k be a value to find in A. The first algorithm, RandomSearch, consists in randomly searching A for k. One simply selects a random index i and test if A[i ] = k. If true, then the algorithm returns i and otherwise…
0 notes
Text
Cracking the Code: How Algorithmic Trading Exploits the Symmetrical Triangle for Profitable Breakouts The Secret Weapon of Elite Traders: Algorithmic Trading Meets the Symmetrical Triangle In the world of Forex trading, patterns are everything. And if you’ve ever found yourself staring at charts, desperately trying to decode market movements, let me introduce you to an overlooked gem: the symmetrical triangle. When combined with algorithmic trading, this pattern can transform the way you approach breakouts, giving you a strategic edge that most traders ignore. But before you dive in, let’s make one thing clear: trading isn’t about gut feelings or throwing darts at price charts. It’s about leveraging data, identifying repeatable patterns, and executing precise strategies. And that’s where algo trading shines—removing emotion and maximizing efficiency while targeting high-probability breakout setups. So, how can you harness the power of algorithmic trading to dominate symmetrical triangle breakouts? Buckle up, because we’re about to crack the code. Why the Symmetrical Triangle is a Goldmine for Algo Traders Most traders see symmetrical triangles as just another chart pattern. They know the basics: a period of consolidation followed by a strong breakout. But the real money is in the details—specifically, in using algorithmic strategies to predict, optimize, and capitalize on those breakouts before the masses catch on. Here’s why the symmetrical triangle is an algo trader’s best friend: - Predictability with Measurable Parameters – Unlike random price movements, symmetrical triangles follow a predictable structure of converging trendlines, making them perfect for automated pattern recognition. - Defined Entry & Exit Points – Algo trading thrives on precision, and symmetrical triangles offer clear breakouts with predefined stop-loss and profit targets. - Reduced False Signals – Algorithmic filters can help identify strong volume-backed breakouts, eliminating the noise that traps manual traders. - Automated Risk Management – By setting predefined risk parameters, algorithmic strategies can cut losers fast and let winners ride—a rule most human traders struggle to follow. How to Build an Algo Strategy for Trading Symmetrical Triangles Now that you know why symmetrical triangles are a goldmine, let’s build a step-by-step algorithmic trading strategy to exploit them. Step 1: Identify the Symmetrical Triangle with Code Forget manually drawing lines on your charts. Let your algorithm do the heavy lifting. Using Python (or any preferred language), your script should: - Detect higher lows and lower highs converging into a triangle. - Define the support and resistance lines dynamically. - Filter out weak formations based on timeframe, volume, and volatility. Step 2: Confirm with Volume & Volatility Filters Breakouts without strong volume? Recipe for fakeouts. Your algo should: - Check for an increase in volume by at least 30% above the moving average before executing a trade. - Integrate the Average True Range (ATR) to confirm that volatility is sufficient for a breakout. Step 3: Automate Trade Execution with Stop-Loss & Profit Targets Once your algo detects a breakout, it should: - Execute a buy or sell order once the price breaks the resistance or support. - Set a stop-loss at the opposite end of the triangle (below support for buy trades, above resistance for sell trades). - Calculate the expected move size based on the height of the triangle and set profit targets accordingly. Step 4: Optimize with Machine Learning (Next-Level Tactic) Want to separate yourself from the average algo trader? Train your bot with historical data and machine learning models to: - Identify which symmetrical triangles have the highest success rate. - Avoid breakouts that tend to fail based on past performance. - Adjust risk-reward ratios dynamically based on probability analysis. Case Study: How a Hedge Fund Used Algorithmic Trading to Dominate Triangle Breakouts According to a study by the Bank for International Settlements (BIS), over 70% of Forex trading is now conducted through algorithmic strategies. One hedge fund in London took this approach to the next level: - They trained an AI model on 10 years of symmetrical triangle formations. - Their algorithm only executed trades when volume spiked above 40% of the average. - They set a 2:1 risk-reward ratio, ensuring profitable trades outweighed small losses. - The result? A 38% annual return over three years. Final Thoughts: Why You Need to Automate Your Triangle Breakouts Now Most traders struggle with emotion-driven decision-making, entering breakouts too early or exiting too late. By integrating algorithmic trading with symmetrical triangle setups, you eliminate human error and maximize precision. Want to automate your strategy? - Stay informed with the latest market updates and real-time indicators at StarseedFX. - Learn advanced strategies with free Forex courses at StarseedFX. - Optimize your trading with our smart trading tool at StarseedFX. Trade smarter, not harder. The algorithm is waiting. Are you ready? —————– Image Credits: Cover image at the top is AI-generated Read the full article
0 notes
Text
Building a Ludo Game with Real Money Betting Features
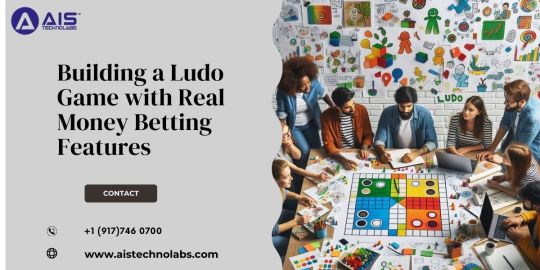
Overview of the Development of Ludo Games
People of all ages love playing the well-known board game Ludo. But as internet gaming has grown in popularity, the classic board game has evolved into a captivating virtual experience. Because it provides an intriguing combination of strategy, competition, and the chance to win real money, Ludo game development with real money betting features is becoming more and more popular these days. We will examine how to create a Ludo game with real money betting elements in this post, going over crucial topics including technological specifications, security protocols, and revenue-generating tactics.
Important Elements of a Real Money Betting Ludo Game
A Ludo game needs essential elements that improve security and user experience in order to succeed in the competitive gaming market. Among the most crucial characteristics are:
Players can compete against actual people all over the world in multiplayer mode.
Secure Payment Gateways: Include dependable payment methods like cryptocurrencies, PayPal, and UPI.
Anti-Fraud Mechanisms: To guarantee fair play, use AI-driven fraud detection systems.
User-Friendly Interface: Make your UI/UX design interesting and simple to use.
Live Chat and Support: Facilitate instant communication and client service.
Create a random number generator (RNG) using the fair play algorithm to guarantee objective results.
Technical Elements of Developing Ludo Games
Selecting the Proper Tech Stack
Building a high-performing Ludo game requires careful consideration of the technology stack. Among the suggested technologies are:
Frontend: HTML5, JavaScript, Unity, and Cocos2d
Backend: PHP, Python, and Node.js
Database: Firebase, MongoDB, and MySQL
Security features include two-factor authentication (2FA) and SSL encryption.
Implementing Real-Time Multiplayer
To keep users interested, a smooth real-time multiplayer experience is essential. Cloud servers and WebSockets can be used to effectively synchronize gaming. To link players with comparable skill levels, AI-based matchmaking can also be used.
Integration of Secure Payments
Integrating secure payment gateways is crucial to guaranteeing safe and easy transactions. User trust is increased with support for many payment methods, including UPI, PayPal, Stripe, and cryptocurrency wallets. A safe betting experience is also guaranteed by adherence to gaming and financial rules.
Strategies for Ludo Betting Game Monetization
When a Ludo game has real money betting features, there are several methods to make money. Among the successful monetization techniques are:
Entry Fees & Commission: Each game has a minor entry cost, and a commission is deducted from the prize fund.
Sponsorships & Advertising: Show tailored advertisements to make money.
Premium avatars, dice designs, and boosters are available through in-game purchases.
Models for Subscriptions: Offer VIP memberships with special advantages.
Legal Aspects and Compliance
Following gaming rules and compliance standards is essential before opening a Ludo game with real money betting. Important things to think about are:
acquiring a gaming license in states where gambling is permitted.
putting in place guidelines for healthy gaming to stop addiction.
guaranteeing data privacy and adherence to other laws, including the GDPR.
Including KYC (identity verification) to stop fraud and gaming by minors.
How to Create a Ludo Game with Betting Elements
1. Market research and planning
Prior to growth, it is essential to comprehend the target audience and market need. Analyze competitors to find USPs (unique selling points).
2. Improving User Experience through UI/UX Design
Player engagement is increased with an interface that is both aesthetically pleasing and simple to use. For a flawless gaming experience, make sure PC and mobile devices are compatible.
3. Testing and Development
Create the fundamental game principles, incorporate betting features, and carry out exhaustive testing to get rid of errors. To safeguard user information and transactions, security testing is essential.
4. Launch and Deployment
Launch the game on several platforms, such as iOS, Android, and the web, following a successful testing phase. To draw users, employ marketing techniques like influencer collaborations and social media promotions.
Future Directions for the Creation of Ludo Games
Following trends might help your Ludo game become more competitive in the ever-changing online gaming market. Among the upcoming trends are:
Integrating blockchain technology to guarantee transaction security and transparency.
AI-Powered Customized Gaming Experience: Increasing player engagement with personalized game recommendations.
Enhancements to VR and AR: Increasing the immersion of the game.
In conclusion
In the expanding online gaming market, investing in Ludo game development with real money betting features might be lucrative. Developers can produce a competitive and enjoyable game by putting in place safe payment methods, interesting UI/UX, and regulatory compliance. AIS Technolabs may assist you if you're searching for a skilled group to create a Ludo game with lots of features. Our skilled developers are experts at creating personalized Ludo games using state-of-the-art technologies. Get in touch with us right now to realize your gaming concept.
Questions and Answers (FAQs)
1. Is creating a Ludo game with real money betting permitted?
Legalities differ from nation to nation. Before starting, it's crucial to review local gambling regulations and secure the required permits.
2. How can I make sure my Ludo betting game is fair?
Fair play can be preserved by putting anti-fraud and Random Number Generator (RNG) procedures into place.
3. Which payment options ought to be included in my Ludo game?
Digital wallets, UPI, PayPal, credit/debit cards, and cryptocurrencies are all well-liked choices.
4. How much time does it take to create a betting Ludo game?
The normal development period is between three and six months, though it might vary based on features and complexity.
5. What are the main obstacles to creating Ludo games?
Obtaining legal licenses, ensuring safe transactions, upholding fair play, and providing consumers with an excellent user interface and user experience are among the challenges.
Blog Source: https://www.knockinglive.com/building-a-ludo-game-with-real-money-betting-features/?snax_post_submission=success
0 notes
Text
Transitioning from Data Analyst to Data Scientist: A Roadmap to Success
In today's data-driven world, businesses rely heavily on data to make informed decisions. While data analysts play a crucial role in interpreting and visualizing data, data scientists go a step further by building predictive models and extracting deeper insights using machine learning. If you are a data analyst looking to transition into a data scientist role, this blog will guide you through the essential steps, required skills, and the best training programs to help you achieve your career goal.
Understanding the Difference: Data Analyst vs. Data Scientist
Before looking into the transition process, it's important to understand the key differences between the two roles:
Data Analysts primarily work with structured data, using tools like Python, SQL, Excel, and visualization platforms (Power BI, Tableau). Their main focus is on reporting, trend analysis, and business intelligence.
Data Scientists go beyond reporting by applying statistical modeling, machine learning, and artificial intelligence to predict outcomes and optimize business strategies. They work with large datasets and use programming languages like Python and R for advanced analytics.
If you are currently a data analyst, making the leap to data science requires upskilling in areas such as machine learning, statistics, and programming. Here’s a structured roadmap to help you make a smooth transition.
1. Strengthen Your Programming Skills
Data analysts often rely on SQL and Excel, but data scientists need proficiency in programming languages like Python and R. These languages are widely used for data manipulation, statistical analysis, and machine learning.
Learn Python: Python is the most popular language for data science due to its simplicity and powerful libraries (Pandas, NumPy, Scikit-learn, TensorFlow).
Master R: R is widely used in academia and research for statistical computing and data visualization.
Enhance your SQL skills: Strong SQL skills are necessary for data extraction and handling large databases.
2. Gain Expertise in Statistics and Mathematics
A strong foundation in statistics and mathematics is essential for data scientists. Unlike data analysts, who primarily focus on descriptive statistics, data scientists need to understand inferential statistics, probability theory, and linear algebra.
Study Probability and Statistics: Concepts like hypothesis testing, confidence intervals, and distributions are fundamental in machine learning.
Learn Linear Algebra and Calculus: Essential for understanding how machine learning algorithms work under the hood.
3. Master Data Manipulation and Visualization
As a data analyst, you may already have experience in data visualization tools. However, data scientists need to go a step further by using Python and R for data manipulation.
Pandas & NumPy (Python): For handling large datasets efficiently.
Matplotlib & Seaborn: To create insightful visualizations.
Power BI & Tableau: If transitioning from analytics, leveraging these tools will be beneficial.
4. Learn Machine Learning Algorithms
Machine learning is the backbone of data science. You need to understand different types of machine learning models and their applications.
Supervised Learning: Regression and classification models (Linear Regression, Decision Trees, Random Forests, SVM, Neural Networks).
Unsupervised Learning: Clustering and dimensionality reduction techniques (K-Means, PCA, Autoencoders).
Deep Learning: Neural networks, CNNs, and RNNs for handling image and text data.
5. Work on Real-World Projects
Practical experience is crucial for a successful transition. Hands-on projects will help you apply your theoretical knowledge and build a strong portfolio.
Kaggle Competitions: Participate in Kaggle challenges to test your skills.
Open Source Contributions: Collaborate on GitHub projects.
Industry Projects: Apply for internships or freelancing gigs in data science.
6. Learn Big Data Technologies
Data scientists often work with massive datasets that require specialized tools for storage and processing.
Hadoop & Spark: For distributed computing and large-scale data processing.
Cloud Platforms (AWS, GCP, Azure): Cloud-based machine learning and data storage.
7. Build a Strong Portfolio and Resume
To stand out in the job market, showcase your data science projects on platforms like GitHub, Kaggle, and LinkedIn.
Create a Portfolio Website: Display your projects, blog posts, and certifications.
Write Technical Blogs: Share insights on data science topics on Medium or personal blogs.
Optimize Your Resume: Highlight your technical skills, certifications, and projects.
8. Obtain a Data Science Certification
Certifications validate your expertise and increase job opportunities.
Google Data Analytics Certificate
IBM Data Science Professional Certificate
AWS Certified Machine Learning Specialty
These certifications from top institutes offer some of the best training and will boost your credibility.
9. Network and Apply for Data Science Jobs
Networking plays a vital role in career transitions. Connect with data science professionals through LinkedIn, attend conferences, and join online communities.
Attend Meetups & Webinars: Engage with data science communities.
Leverage Job Portals: Apply on LinkedIn, Glassdoor, and Indeed.
Consider Internships: Entry-level data science roles or internships can help gain practical experience.
Conclusion
Transitioning from a data analyst to a data scientist is a challenging but rewarding journey. By following this roadmap—mastering programming, statistics, machine learning, and big data technologies—you can successfully shift into a data science role. Enrolling in the best courses and training programs will accelerate your learning and make you job-ready.
Remember, continuous learning and practical experience are key. Start today, work on projects, earn certifications, and network with industry professionals. Your dream job as a data scientist is just a few steps away.
0 notes
Text
Teen Patti Game Development: A Comprehensive Guide
Introduction
Teen Patti, otherwise called Indian Poker, is one of the most well known card games in South Asia, especially in India. With the rising of convenient gaming and online multiplayer stages, Teen Patti has changed from conventional social events to advanced experiences.
This article plunges into the improvement of a Teen Patti game, covering fundamental perspectives like game mechanics, development stack, elements, adaptation, and difficulties.
Understanding the Teen Patti Game
Teen Patti is a three-card game got from Poker and played with a standard 52-card deck. The game starts with players placing an ante or boot amount, and each player is dealt three cards. Players can either play 'blind' (without seeing their cards) or 'seen' (after actually taking a look at their cards). The goal is to have the highest-ranking hand or to feign rivals into collapsing.
Basic Rules of Teen Patti:
Number of Players: Typically 3-6 players.
Wagering Framework: Similar to poker, with blinds, chaal (call), and raise mechanics.
Hand Rankings:
Trail/Three of a Sort (highest)
Straight Flush/Unadulterated Succession
Straight/Succession
Flush/Variety
Pair
High Card
Moves toward Foster a Teen Patti Game
1. Market Research and Planning
Prior to starting development, direct top to bottom research on existing Teen Patti apps. Recognize popular features, user inclinations, and monetization strategies. Characterize your target audience — casual players, high rollers, or professional gamers.
2. Choosing the Right Innovation Stack
Choosing the right innovation stack is crucial for performance, scalability, and user experience.
Front-End: Solidarity, Unreal Motor, React Native, or Shudder
Back-End: Node.js, Python (Django/Flask), Java (Spring Boot)
Database: MySQL, MongoDB, Firebase
Real-Time Communication: WebSockets, Socket.io
Cloud Administrations: AWS, Google Cloud, Azure
Security: SSL Encryption, Anti-Fraud Frameworks
3. Game Plan and UI/UX
A visually appealing and user-friendly interface enhances player engagement. Key plan aspects include:
Intuitive controls for smooth gameplay
Tasteful card plans and animations
Easy-to-navigate menus
Interactive audio cues and background music
4. Core Game Development
a) Game Rationale and Algorithms
Foster hearty game rationale for card appropriation, wagering adjusts, player turns, and win calculations.
b) Multiplayer Integration
Multiplayer functionality is essential. Execute real-time matchmaking, private tables, and social features.
c) Random Number Generator (RNG)
Guarantee fairness utilizing a RNG-affirmed algorithm to rearrange and deal cards.
5. Features to Remember for Teen Patti Game
Various Game Modes: Classic, Joker, AK47, Muflis, and more.
Multiplayer Backing: Play with friends or global players.
Chat and Emojis: In-game chat and emoticon reactions.
Leaderboards and Achievements: Reward top players with rankings.
Allude and Earn: Encourage user development through referrals.
Virtual Money: Chips, gold coins, and daily rewards.
Security and Fair Play: Anti-cheating measures and secure transactions.
6. Testing and Troubleshooting
Prior to launching, thorough testing is required:
Unit Testing: Confirm individual parts.
Integration Testing: Guarantee smooth data stream.
Load Testing: Test performance under heavy traffic.
Beta Testing: Get user feedback for enhancements.
7. Monetization Strategies
A very much planned monetization plan maximizes income. Normal models include:
In-App Purchases: Purchase chips, premium features, and selective game modes.
Ads and Sponsorships: Carry out rewarded ads, interstitials, and banners.
Membership Model: Offer celebrity enrollments with added benefits.
Tournaments and Section Expenses: Charge a passage expense for jackpot tournaments.
8. Launch and Marketing
After development, the game requirements successful marketing for perceivability.
App Store Optimization (ASO): Improve portrayals, catchphrases, and screen captures.
Social Media and Influencers: Advance via YouTube, Instagram, and Facebook.
Paid Campaigns: Run Google Ads, Facebook Ads, and force to be reckoned with collaborations.
Referral Programs: Encourage users to welcome friends for rewards.
9. Post-Launch Updates and Maintenance
Constant updates keep the game new and engaging.
Add new game modes and features.
Fix messes with and advance performance.
Gather user feedback and improve gameplay.
Challenges in Teen Patti Game Development
1. Fair Play and Security
Guaranteeing a cheat-verification framework with RNG-ensured algorithms and anti-hacking measures.
2. Regulatory Compliance
Various nations have varying laws on web based gambling. Guarantee legal compliance and acquire necessary licenses.
3. User Maintenance
Keeping players engaged requires regular updates, occasions, and social components.
4. Scalability
Handling a large number of simultaneous players demands productive server architecture and cloud arrangements.
Conclusion
Teen Patti game development is a thrilling and profitable endeavor, yet it requires strategic planning, the right innovation, and persistent updates. With an engaging UI, vigorous security, and compelling marketing, engineers can create a fruitful and high-netting Teen Patti gaming platform. By zeroing in on user experience and fair gameplay, your Teen Patti game can stand out in the cutthroat market and attract a loyal player base.
#Casino#Gambling#Betting#Slots#Poker#Blackjack#Roulette#Jackpot#Vegas#CasinoLife#CasinoGames#Gambler
0 notes
Text
UNLOCKING THE POWER OF AI WITH EASYLIBPAL 2/2
EXPANDED COMPONENTS AND DETAILS OF EASYLIBPAL:
1. Easylibpal Class: The core component of the library, responsible for handling algorithm selection, model fitting, and prediction generation
2. Algorithm Selection and Support:
Supports classic AI algorithms such as Linear Regression, Logistic Regression, Support Vector Machine (SVM), Naive Bayes, and K-Nearest Neighbors (K-NN).
and
- Decision Trees
- Random Forest
- AdaBoost
- Gradient Boosting
3. Integration with Popular Libraries: Seamless integration with essential Python libraries like NumPy, Pandas, Matplotlib, and Scikit-learn for enhanced functionality.
4. Data Handling:
- DataLoader class for importing and preprocessing data from various formats (CSV, JSON, SQL databases).
- DataTransformer class for feature scaling, normalization, and encoding categorical variables.
- Includes functions for loading and preprocessing datasets to prepare them for training and testing.
- `FeatureSelector` class: Provides methods for feature selection and dimensionality reduction.
5. Model Evaluation:
- Evaluator class to assess model performance using metrics like accuracy, precision, recall, F1-score, and ROC-AUC.
- Methods for generating confusion matrices and classification reports.
6. Model Training: Contains methods for fitting the selected algorithm with the training data.
- `fit` method: Trains the selected algorithm on the provided training data.
7. Prediction Generation: Allows users to make predictions using the trained model on new data.
- `predict` method: Makes predictions using the trained model on new data.
- `predict_proba` method: Returns the predicted probabilities for classification tasks.
8. Model Evaluation:
- `Evaluator` class: Assesses model performance using various metrics (e.g., accuracy, precision, recall, F1-score, ROC-AUC).
- `cross_validate` method: Performs cross-validation to evaluate the model's performance.
- `confusion_matrix` method: Generates a confusion matrix for classification tasks.
- `classification_report` method: Provides a detailed classification report.
9. Hyperparameter Tuning:
- Tuner class that uses techniques likes Grid Search and Random Search for hyperparameter optimization.
10. Visualization:
- Integration with Matplotlib and Seaborn for generating plots to analyze model performance and data characteristics.
- Visualization support: Enables users to visualize data, model performance, and predictions using plotting functionalities.
- `Visualizer` class: Integrates with Matplotlib and Seaborn to generate plots for model performance analysis and data visualization.
- `plot_confusion_matrix` method: Visualizes the confusion matrix.
- `plot_roc_curve` method: Plots the Receiver Operating Characteristic (ROC) curve.
- `plot_feature_importance` method: Visualizes feature importance for applicable algorithms.
11. Utility Functions:
- Functions for saving and loading trained models.
- Logging functionalities to track the model training and prediction processes.
- `save_model` method: Saves the trained model to a file.
- `load_model` method: Loads a previously trained model from a file.
- `set_logger` method: Configures logging functionality for tracking model training and prediction processes.
12. User-Friendly Interface: Provides a simplified and intuitive interface for users to interact with and apply classic AI algorithms without extensive knowledge or configuration.
13.. Error Handling: Incorporates mechanisms to handle invalid inputs, errors during training, and other potential issues during algorithm usage.
- Custom exception classes for handling specific errors and providing informative error messages to users.
14. Documentation: Comprehensive documentation to guide users on how to use Easylibpal effectively and efficiently
- Comprehensive documentation explaining the usage and functionality of each component.
- Example scripts demonstrating how to use Easylibpal for various AI tasks and datasets.
15. Testing Suite:
- Unit tests for each component to ensure code reliability and maintainability.
- Integration tests to verify the smooth interaction between different components.
IMPLEMENTATION EXAMPLE WITH ADDITIONAL FEATURES:
Here is an example of how the expanded Easylibpal library could be structured and used:
```python
import numpy as np
import pandas as pd
from sklearn.model_selection import train_test_split
from sklearn.preprocessing import StandardScaler
from easylibpal import Easylibpal, DataLoader, Evaluator, Tuner
# Example DataLoader
class DataLoader:
def load_data(self, filepath, file_type='csv'):
if file_type == 'csv':
return pd.read_csv(filepath)
else:
raise ValueError("Unsupported file type provided.")
# Example Evaluator
class Evaluator:
def evaluate(self, model, X_test, y_test):
predictions = model.predict(X_test)
accuracy = np.mean(predictions == y_test)
return {'accuracy': accuracy}
# Example usage of Easylibpal with DataLoader and Evaluator
if __name__ == "__main__":
# Load and prepare the data
data_loader = DataLoader()
data = data_loader.load_data('path/to/your/data.csv')
X = data.iloc[:, :-1]
y = data.iloc[:, -1]
X_train, X_test, y_train, y_test = train_test_split(X, y, test_size=0.2, random_state=42)
# Scale features
scaler = StandardScaler()
X_train_scaled = scaler.fit_transform(X_train)
X_test_scaled = scaler.transform(X_test)
# Initialize Easylibpal with the desired algorithm
model = Easylibpal('Random Forest')
model.fit(X_train_scaled, y_train)
# Evaluate the model
evaluator = Evaluator()
results = evaluator.evaluate(model, X_test_scaled, y_test)
print(f"Model Accuracy: {results['accuracy']}")
# Optional: Use Tuner for hyperparameter optimization
tuner = Tuner(model, param_grid={'n_estimators': [100, 200], 'max_depth': [10, 20, 30]})
best_params = tuner.optimize(X_train_scaled, y_train)
print(f"Best Parameters: {best_params}")
```
This example demonstrates the structured approach to using Easylibpal with enhanced data handling, model evaluation, and optional hyperparameter tuning. The library empowers users to handle real-world datasets, apply various machine learning algorithms, and evaluate their performance with ease, making it an invaluable tool for developers and data scientists aiming to implement AI solutions efficiently.
Easylibpal is dedicated to making the latest AI technology accessible to everyone, regardless of their background or expertise. Our platform simplifies the process of selecting and implementing classic AI algorithms, enabling users across various industries to harness the power of artificial intelligence with ease. By democratizing access to AI, we aim to accelerate innovation and empower users to achieve their goals with confidence. Easylibpal's approach involves a democratization framework that reduces entry barriers, lowers the cost of building AI solutions, and speeds up the adoption of AI in both academic and business settings.
Below are examples showcasing how each main component of the Easylibpal library could be implemented and used in practice to provide a user-friendly interface for utilizing classic AI algorithms.
1. Core Components
Easylibpal Class Example:
```python
class Easylibpal:
def __init__(self, algorithm):
self.algorithm = algorithm
self.model = None
def fit(self, X, y):
# Simplified example: Instantiate and train a model based on the selected algorithm
if self.algorithm == 'Linear Regression':
from sklearn.linear_model import LinearRegression
self.model = LinearRegression()
elif self.algorithm == 'Random Forest':
from sklearn.ensemble import RandomForestClassifier
self.model = RandomForestClassifier()
self.model.fit(X, y)
def predict(self, X):
return self.model.predict(X)
```
2. Data Handling
DataLoader Class Example:
```python
class DataLoader:
def load_data(self, filepath, file_type='csv'):
if file_type == 'csv':
import pandas as pd
return pd.read_csv(filepath)
else:
raise ValueError("Unsupported file type provided.")
```
3. Model Evaluation
Evaluator Class Example:
```python
from sklearn.metrics import accuracy_score, classification_report
class Evaluator:
def evaluate(self, model, X_test, y_test):
predictions = model.predict(X_test)
accuracy = accuracy_score(y_test, predictions)
report = classification_report(y_test, predictions)
return {'accuracy': accuracy, 'report': report}
```
4. Hyperparameter Tuning
Tuner Class Example:
```python
from sklearn.model_selection import GridSearchCV
class Tuner:
def __init__(self, model, param_grid):
self.model = model
self.param_grid = param_grid
def optimize(self, X, y):
grid_search = GridSearchCV(self.model, self.param_grid, cv=5)
grid_search.fit(X, y)
return grid_search.best_params_
```
5. Visualization
Visualizer Class Example:
```python
import matplotlib.pyplot as plt
class Visualizer:
def plot_confusion_matrix(self, cm, classes, normalize=False, title='Confusion matrix'):
plt.imshow(cm, interpolation='nearest', cmap=plt.cm.Blues)
plt.title(title)
plt.colorbar()
tick_marks = np.arange(len(classes))
plt.xticks(tick_marks, classes, rotation=45)
plt.yticks(tick_marks, classes)
plt.ylabel('True label')
plt.xlabel('Predicted label')
plt.show()
```
6. Utility Functions
Save and Load Model Example:
```python
import joblib
def save_model(model, filename):
joblib.dump(model, filename)
def load_model(filename):
return joblib.load(filename)
```
7. Example Usage Script
Using Easylibpal in a Script:
```python
# Assuming Easylibpal and other classes have been imported
data_loader = DataLoader()
data = data_loader.load_data('data.csv')
X = data.drop('Target', axis=1)
y = data['Target']
model = Easylibpal('Random Forest')
model.fit(X, y)
evaluator = Evaluator()
results = evaluator.evaluate(model, X, y)
print("Accuracy:", results['accuracy'])
print("Report:", results['report'])
visualizer = Visualizer()
visualizer.plot_confusion_matrix(results['cm'], classes=['Class1', 'Class2'])
save_model(model, 'trained_model.pkl')
loaded_model = load_model('trained_model.pkl')
```
These examples illustrate the practical implementation and use of the Easylibpal library components, aiming to simplify the application of AI algorithms for users with varying levels of expertise in machine learning.
EASYLIBPAL IMPLEMENTATION:
Step 1: Define the Problem
First, we need to define the problem we want to solve. For this POC, let's assume we want to predict house prices based on various features like the number of bedrooms, square footage, and location.
Step 2: Choose an Appropriate Algorithm
Given our problem, a supervised learning algorithm like linear regression would be suitable. We'll use Scikit-learn, a popular library for machine learning in Python, to implement this algorithm.
Step 3: Prepare Your Data
We'll use Pandas to load and prepare our dataset. This involves cleaning the data, handling missing values, and splitting the dataset into training and testing sets.
Step 4: Implement the Algorithm
Now, we'll use Scikit-learn to implement the linear regression algorithm. We'll train the model on our training data and then test its performance on the testing data.
Step 5: Evaluate the Model
Finally, we'll evaluate the performance of our model using metrics like Mean Squared Error (MSE) and R-squared.
Python Code POC
```python
import numpy as np
import pandas as pd
from sklearn.model_selection import train_test_split
from sklearn.linear_model import LinearRegression
from sklearn.metrics import mean_squared_error, r2_score
# Load the dataset
data = pd.read_csv('house_prices.csv')
# Prepare the data
X = data'bedrooms', 'square_footage', 'location'
y = data['price']
# Split the data into training and testing sets
X_train, X_test, y_train, y_test = train_test_split(X, y, test_size=0.2, random_state=42)
# Create and train the model
model = LinearRegression()
model.fit(X_train, y_train)
# Make predictions
predictions = model.predict(X_test)
# Evaluate the model
mse = mean_squared_error(y_test, predictions)
r2 = r2_score(y_test, predictions)
print(f'Mean Squared Error: {mse}')
print(f'R-squared: {r2}')
```
Below is an implementation, Easylibpal provides a simple interface to instantiate and utilize classic AI algorithms such as Linear Regression, Logistic Regression, SVM, Naive Bayes, and K-NN. Users can easily create an instance of Easylibpal with their desired algorithm, fit the model with training data, and make predictions, all with minimal code and hassle. This demonstrates the power of Easylibpal in simplifying the integration of AI algorithms for various tasks.
```python
# Import necessary libraries
import numpy as np
import pandas as pd
import matplotlib.pyplot as plt
from sklearn.linear_model import LinearRegression
from sklearn.linear_model import LogisticRegression
from sklearn.svm import SVC
from sklearn.naive_bayes import GaussianNB
from sklearn.neighbors import KNeighborsClassifier
class Easylibpal:
def __init__(self, algorithm):
self.algorithm = algorithm
def fit(self, X, y):
if self.algorithm == 'Linear Regression':
self.model = LinearRegression()
elif self.algorithm == 'Logistic Regression':
self.model = LogisticRegression()
elif self.algorithm == 'SVM':
self.model = SVC()
elif self.algorithm == 'Naive Bayes':
self.model = GaussianNB()
elif self.algorithm == 'K-NN':
self.model = KNeighborsClassifier()
else:
raise ValueError("Invalid algorithm specified.")
self.model.fit(X, y)
def predict(self, X):
return self.model.predict(X)
# Example usage:
# Initialize Easylibpal with the desired algorithm
easy_algo = Easylibpal('Linear Regression')
# Generate some sample data
X = np.array([[1], [2], [3], [4]])
y = np.array([2, 4, 6, 8])
# Fit the model
easy_algo.fit(X, y)
# Make predictions
predictions = easy_algo.predict(X)
# Plot the results
plt.scatter(X, y)
plt.plot(X, predictions, color='red')
plt.title('Linear Regression with Easylibpal')
plt.xlabel('X')
plt.ylabel('y')
plt.show()
```
Easylibpal is an innovative Python library designed to simplify the integration and use of classic AI algorithms in a user-friendly manner. It aims to bridge the gap between the complexity of AI libraries and the ease of use, making it accessible for developers and data scientists alike. Easylibpal abstracts the underlying complexity of each algorithm, providing a unified interface that allows users to apply these algorithms with minimal configuration and understanding of the underlying mechanisms.
ENHANCED DATASET HANDLING
Easylibpal should be able to handle datasets more efficiently. This includes loading datasets from various sources (e.g., CSV files, databases), preprocessing data (e.g., normalization, handling missing values), and splitting data into training and testing sets.
```python
import os
from sklearn.model_selection import train_test_split
class Easylibpal:
# Existing code...
def load_dataset(self, filepath):
"""Loads a dataset from a CSV file."""
if not os.path.exists(filepath):
raise FileNotFoundError("Dataset file not found.")
return pd.read_csv(filepath)
def preprocess_data(self, dataset):
"""Preprocesses the dataset."""
# Implement data preprocessing steps here
return dataset
def split_data(self, X, y, test_size=0.2):
"""Splits the dataset into training and testing sets."""
return train_test_split(X, y, test_size=test_size)
```
Additional Algorithms
Easylibpal should support a wider range of algorithms. This includes decision trees, random forests, and gradient boosting machines.
```python
from sklearn.tree import DecisionTreeClassifier
from sklearn.ensemble import RandomForestClassifier
from sklearn.ensemble import GradientBoostingClassifier
class Easylibpal:
# Existing code...
def fit(self, X, y):
# Existing code...
elif self.algorithm == 'Decision Tree':
self.model = DecisionTreeClassifier()
elif self.algorithm == 'Random Forest':
self.model = RandomForestClassifier()
elif self.algorithm == 'Gradient Boosting':
self.model = GradientBoostingClassifier()
# Add more algorithms as needed
```
User-Friendly Features
To make Easylibpal even more user-friendly, consider adding features like:
- Automatic hyperparameter tuning: Implementing a simple interface for hyperparameter tuning using GridSearchCV or RandomizedSearchCV.
- Model evaluation metrics: Providing easy access to common evaluation metrics like accuracy, precision, recall, and F1 score.
- Visualization tools: Adding methods for plotting model performance, confusion matrices, and feature importance.
```python
from sklearn.metrics import accuracy_score, classification_report
from sklearn.model_selection import GridSearchCV
class Easylibpal:
# Existing code...
def evaluate_model(self, X_test, y_test):
"""Evaluates the model using accuracy and classification report."""
y_pred = self.predict(X_test)
print("Accuracy:", accuracy_score(y_test, y_pred))
print(classification_report(y_test, y_pred))
def tune_hyperparameters(self, X, y, param_grid):
"""Tunes the model's hyperparameters using GridSearchCV."""
grid_search = GridSearchCV(self.model, param_grid, cv=5)
grid_search.fit(X, y)
self.model = grid_search.best_estimator_
```
Easylibpal leverages the power of Python and its rich ecosystem of AI and machine learning libraries, such as scikit-learn, to implement the classic algorithms. It provides a high-level API that abstracts the specifics of each algorithm, allowing users to focus on the problem at hand rather than the intricacies of the algorithm.
Python Code Snippets for Easylibpal
Below are Python code snippets demonstrating the use of Easylibpal with classic AI algorithms. Each snippet demonstrates how to use Easylibpal to apply a specific algorithm to a dataset.
# Linear Regression
```python
from Easylibpal import Easylibpal
# Initialize Easylibpal with a dataset
Easylibpal = Easylibpal(dataset='your_dataset.csv')
# Apply Linear Regression
result = Easylibpal.apply_algorithm('linear_regression', target_column='target')
# Print the result
print(result)
```
# Logistic Regression
```python
from Easylibpal import Easylibpal
# Initialize Easylibpal with a dataset
Easylibpal = Easylibpal(dataset='your_dataset.csv')
# Apply Logistic Regression
result = Easylibpal.apply_algorithm('logistic_regression', target_column='target')
# Print the result
print(result)
```
# Support Vector Machines (SVM)
```python
from Easylibpal import Easylibpal
# Initialize Easylibpal with a dataset
Easylibpal = Easylibpal(dataset='your_dataset.csv')
# Apply SVM
result = Easylibpal.apply_algorithm('svm', target_column='target')
# Print the result
print(result)
```
# Naive Bayes
```python
from Easylibpal import Easylibpal
# Initialize Easylibpal with a dataset
Easylibpal = Easylibpal(dataset='your_dataset.csv')
# Apply Naive Bayes
result = Easylibpal.apply_algorithm('naive_bayes', target_column='target')
# Print the result
print(result)
```
# K-Nearest Neighbors (K-NN)
```python
from Easylibpal import Easylibpal
# Initialize Easylibpal with a dataset
Easylibpal = Easylibpal(dataset='your_dataset.csv')
# Apply K-NN
result = Easylibpal.apply_algorithm('knn', target_column='target')
# Print the result
print(result)
```
ABSTRACTION AND ESSENTIAL COMPLEXITY
- Essential Complexity: This refers to the inherent complexity of the problem domain, which cannot be reduced regardless of the programming language or framework used. It includes the logic and algorithm needed to solve the problem. For example, the essential complexity of sorting a list remains the same across different programming languages.
- Accidental Complexity: This is the complexity introduced by the choice of programming language, framework, or libraries. It can be reduced or eliminated through abstraction. For instance, using a high-level API in Python can hide the complexity of lower-level operations, making the code more readable and maintainable.
HOW EASYLIBPAL ABSTRACTS COMPLEXITY
Easylibpal aims to reduce accidental complexity by providing a high-level API that encapsulates the details of each classic AI algorithm. This abstraction allows users to apply these algorithms without needing to understand the underlying mechanisms or the specifics of the algorithm's implementation.
- Simplified Interface: Easylibpal offers a unified interface for applying various algorithms, such as Linear Regression, Logistic Regression, SVM, Naive Bayes, and K-NN. This interface abstracts the complexity of each algorithm, making it easier for users to apply them to their datasets.
- Runtime Fusion: By evaluating sub-expressions and sharing them across multiple terms, Easylibpal can optimize the execution of algorithms. This approach, similar to runtime fusion in abstract algorithms, allows for efficient computation without duplicating work, thereby reducing the computational complexity.
- Focus on Essential Complexity: While Easylibpal abstracts away the accidental complexity; it ensures that the essential complexity of the problem domain remains at the forefront. This means that while the implementation details are hidden, the core logic and algorithmic approach are still accessible and understandable to the user.
To implement Easylibpal, one would need to create a Python class that encapsulates the functionality of each classic AI algorithm. This class would provide methods for loading datasets, preprocessing data, and applying the algorithm with minimal configuration required from the user. The implementation would leverage existing libraries like scikit-learn for the actual algorithmic computations, abstracting away the complexity of these libraries.
Here's a conceptual example of how the Easylibpal class might be structured for applying a Linear Regression algorithm:
```python
class Easylibpal:
def __init__(self, dataset):
self.dataset = dataset
# Load and preprocess the dataset
def apply_linear_regression(self, target_column):
# Abstracted implementation of Linear Regression
# This method would internally use scikit-learn or another library
# to perform the actual computation, abstracting the complexity
pass
# Usage
Easylibpal = Easylibpal(dataset='your_dataset.csv')
result = Easylibpal.apply_linear_regression(target_column='target')
```
This example demonstrates the concept of Easylibpal by abstracting the complexity of applying a Linear Regression algorithm. The actual implementation would need to include the specifics of loading the dataset, preprocessing it, and applying the algorithm using an underlying library like scikit-learn.
Easylibpal abstracts the complexity of classic AI algorithms by providing a simplified interface that hides the intricacies of each algorithm's implementation. This abstraction allows users to apply these algorithms with minimal configuration and understanding of the underlying mechanisms. Here are examples of specific algorithms that Easylibpal abstracts:
To implement Easylibpal, one would need to create a Python class that encapsulates the functionality of each classic AI algorithm. This class would provide methods for loading datasets, preprocessing data, and applying the algorithm with minimal configuration required from the user. The implementation would leverage existing libraries like scikit-learn for the actual algorithmic computations, abstracting away the complexity of these libraries.
Here's a conceptual example of how the Easylibpal class might be structured for applying a Linear Regression algorithm:
```python
class Easylibpal:
def __init__(self, dataset):
self.dataset = dataset
# Load and preprocess the dataset
def apply_linear_regression(self, target_column):
# Abstracted implementation of Linear Regression
# This method would internally use scikit-learn or another library
# to perform the actual computation, abstracting the complexity
pass
# Usage
Easylibpal = Easylibpal(dataset='your_dataset.csv')
result = Easylibpal.apply_linear_regression(target_column='target')
```
This example demonstrates the concept of Easylibpal by abstracting the complexity of applying a Linear Regression algorithm. The actual implementation would need to include the specifics of loading the dataset, preprocessing it, and applying the algorithm using an underlying library like scikit-learn.
Easylibpal abstracts the complexity of feature selection for classic AI algorithms by providing a simplified interface that automates the process of selecting the most relevant features for each algorithm. This abstraction is crucial because feature selection is a critical step in machine learning that can significantly impact the performance of a model. Here's how Easylibpal handles feature selection for the mentioned algorithms:
To implement feature selection in Easylibpal, one could use scikit-learn's `SelectKBest` or `RFE` classes for feature selection based on statistical tests or model coefficients. Here's a conceptual example of how feature selection might be integrated into the Easylibpal class for Linear Regression:
```python
from sklearn.feature_selection import SelectKBest, f_regression
from sklearn.linear_model import LinearRegression
class Easylibpal:
def __init__(self, dataset):
self.dataset = dataset
# Load and preprocess the dataset
def apply_linear_regression(self, target_column):
# Feature selection using SelectKBest
selector = SelectKBest(score_func=f_regression, k=10)
X_new = selector.fit_transform(self.dataset.drop(target_column, axis=1), self.dataset[target_column])
# Train Linear Regression model
model = LinearRegression()
model.fit(X_new, self.dataset[target_column])
# Return the trained model
return model
# Usage
Easylibpal = Easylibpal(dataset='your_dataset.csv')
model = Easylibpal.apply_linear_regression(target_column='target')
```
This example demonstrates how Easylibpal abstracts the complexity of feature selection for Linear Regression by using scikit-learn's `SelectKBest` to select the top 10 features based on their statistical significance in predicting the target variable. The actual implementation would need to adapt this approach for each algorithm, considering the specific characteristics and requirements of each algorithm.
To implement feature selection in Easylibpal, one could use scikit-learn's `SelectKBest`, `RFE`, or other feature selection classes based on the algorithm's requirements. Here's a conceptual example of how feature selection might be integrated into the Easylibpal class for Logistic Regression using RFE:
```python
from sklearn.feature_selection import RFE
from sklearn.linear_model import LogisticRegression
class Easylibpal:
def __init__(self, dataset):
self.dataset = dataset
# Load and preprocess the dataset
def apply_logistic_regression(self, target_column):
# Feature selection using RFE
model = LogisticRegression()
rfe = RFE(model, n_features_to_select=10)
rfe.fit(self.dataset.drop(target_column, axis=1), self.dataset[target_column])
# Train Logistic Regression model
model.fit(self.dataset.drop(target_column, axis=1), self.dataset[target_column])
# Return the trained model
return model
# Usage
Easylibpal = Easylibpal(dataset='your_dataset.csv')
model = Easylibpal.apply_logistic_regression(target_column='target')
```
This example demonstrates how Easylibpal abstracts the complexity of feature selection for Logistic Regression by using scikit-learn's `RFE` to select the top 10 features based on their importance in the model. The actual implementation would need to adapt this approach for each algorithm, considering the specific characteristics and requirements of each algorithm.
EASYLIBPAL HANDLES DIFFERENT TYPES OF DATASETS
Easylibpal handles different types of datasets with varying structures by adopting a flexible and adaptable approach to data preprocessing and transformation. This approach is inspired by the principles of tidy data and the need to ensure data is in a consistent, usable format before applying AI algorithms. Here's how Easylibpal addresses the challenges posed by varying dataset structures:
One Type in Multiple Tables
When datasets contain different variables, the same variables with different names, different file formats, or different conventions for missing values, Easylibpal employs a process similar to tidying data. This involves identifying and standardizing the structure of each dataset, ensuring that each variable is consistently named and formatted across datasets. This process might include renaming columns, converting data types, and handling missing values in a uniform manner. For datasets stored in different file formats, Easylibpal would use appropriate libraries (e.g., pandas for CSV, Excel files, and SQL databases) to load and preprocess the data before applying the algorithms.
Multiple Types in One Table
For datasets that involve values collected at multiple levels or on different types of observational units, Easylibpal applies a normalization process. This involves breaking down the dataset into multiple tables, each representing a distinct type of observational unit. For example, if a dataset contains information about songs and their rankings over time, Easylibpal would separate this into two tables: one for song details and another for rankings. This normalization ensures that each fact is expressed in only one place, reducing inconsistencies and making the data more manageable for analysis.
Data Semantics
Easylibpal ensures that the data is organized in a way that aligns with the principles of data semantics, where every value belongs to a variable and an observation. This organization is crucial for the algorithms to interpret the data correctly. Easylibpal might use functions like `pivot_longer` and `pivot_wider` from the tidyverse or equivalent functions in pandas to reshape the data into a long format, where each row represents a single observation and each column represents a single variable. This format is particularly useful for algorithms that require a consistent structure for input data.
Messy Data
Dealing with messy data, which can include inconsistent data types, missing values, and outliers, is a common challenge in data science. Easylibpal addresses this by implementing robust data cleaning and preprocessing steps. This includes handling missing values (e.g., imputation or deletion), converting data types to ensure consistency, and identifying and removing outliers. These steps are crucial for preparing the data in a format that is suitable for the algorithms, ensuring that the algorithms can effectively learn from the data without being hindered by its inconsistencies.
To implement these principles in Python, Easylibpal would leverage libraries like pandas for data manipulation and preprocessing. Here's a conceptual example of how Easylibpal might handle a dataset with multiple types in one table:
```python
import pandas as pd
# Load the dataset
dataset = pd.read_csv('your_dataset.csv')
# Normalize the dataset by separating it into two tables
song_table = dataset'artist', 'track'.drop_duplicates().reset_index(drop=True)
song_table['song_id'] = range(1, len(song_table) + 1)
ranking_table = dataset'artist', 'track', 'week', 'rank'.drop_duplicates().reset_index(drop=True)
# Now, song_table and ranking_table can be used separately for analysis
```
This example demonstrates how Easylibpal might normalize a dataset with multiple types of observational units into separate tables, ensuring that each type of observational unit is stored in its own table. The actual implementation would need to adapt this approach based on the specific structure and requirements of the dataset being processed.
CLEAN DATA
Easylibpal employs a comprehensive set of data cleaning and preprocessing steps to handle messy data, ensuring that the data is in a suitable format for machine learning algorithms. These steps are crucial for improving the accuracy and reliability of the models, as well as preventing misleading results and conclusions. Here's a detailed look at the specific steps Easylibpal might employ:
1. Remove Irrelevant Data
The first step involves identifying and removing data that is not relevant to the analysis or modeling task at hand. This could include columns or rows that do not contribute to the predictive power of the model or are not necessary for the analysis .
2. Deduplicate Data
Deduplication is the process of removing duplicate entries from the dataset. Duplicates can skew the analysis and lead to incorrect conclusions. Easylibpal would use appropriate methods to identify and remove duplicates, ensuring that each entry in the dataset is unique.
3. Fix Structural Errors
Structural errors in the dataset, such as inconsistent data types, incorrect values, or formatting issues, can significantly impact the performance of machine learning algorithms. Easylibpal would employ data cleaning techniques to correct these errors, ensuring that the data is consistent and correctly formatted.
4. Deal with Missing Data
Handling missing data is a common challenge in data preprocessing. Easylibpal might use techniques such as imputation (filling missing values with statistical estimates like mean, median, or mode) or deletion (removing rows or columns with missing values) to address this issue. The choice of method depends on the nature of the data and the specific requirements of the analysis.
5. Filter Out Data Outliers
Outliers can significantly affect the performance of machine learning models. Easylibpal would use statistical methods to identify and filter out outliers, ensuring that the data is more representative of the population being analyzed.
6. Validate Data
The final step involves validating the cleaned and preprocessed data to ensure its quality and accuracy. This could include checking for consistency, verifying the correctness of the data, and ensuring that the data meets the requirements of the machine learning algorithms. Easylibpal would employ validation techniques to confirm that the data is ready for analysis.
To implement these data cleaning and preprocessing steps in Python, Easylibpal would leverage libraries like pandas and scikit-learn. Here's a conceptual example of how these steps might be integrated into the Easylibpal class:
```python
import pandas as pd
from sklearn.impute import SimpleImputer
from sklearn.preprocessing import StandardScaler
class Easylibpal:
def __init__(self, dataset):
self.dataset = dataset
# Load and preprocess the dataset
def clean_and_preprocess(self):
# Remove irrelevant data
self.dataset = self.dataset.drop(['irrelevant_column'], axis=1)
# Deduplicate data
self.dataset = self.dataset.drop_duplicates()
# Fix structural errors (example: correct data type)
self.dataset['correct_data_type_column'] = self.dataset['correct_data_type_column'].astype(float)
# Deal with missing data (example: imputation)
imputer = SimpleImputer(strategy='mean')
self.dataset['missing_data_column'] = imputer.fit_transform(self.dataset'missing_data_column')
# Filter out data outliers (example: using Z-score)
# This step requires a more detailed implementation based on the specific dataset
# Validate data (example: checking for NaN values)
assert not self.dataset.isnull().values.any(), "Data still contains NaN values"
# Return the cleaned and preprocessed dataset
return self.dataset
# Usage
Easylibpal = Easylibpal(dataset=pd.read_csv('your_dataset.csv'))
cleaned_dataset = Easylibpal.clean_and_preprocess()
```
This example demonstrates a simplified approach to data cleaning and preprocessing within Easylibpal. The actual implementation would need to adapt these steps based on the specific characteristics and requirements of the dataset being processed.
VALUE DATA
Easylibpal determines which data is irrelevant and can be removed through a combination of domain knowledge, data analysis, and automated techniques. The process involves identifying data that does not contribute to the analysis, research, or goals of the project, and removing it to improve the quality, efficiency, and clarity of the data. Here's how Easylibpal might approach this:
Domain Knowledge
Easylibpal leverages domain knowledge to identify data that is not relevant to the specific goals of the analysis or modeling task. This could include data that is out of scope, outdated, duplicated, or erroneous. By understanding the context and objectives of the project, Easylibpal can systematically exclude data that does not add value to the analysis.
Data Analysis
Easylibpal employs data analysis techniques to identify irrelevant data. This involves examining the dataset to understand the relationships between variables, the distribution of data, and the presence of outliers or anomalies. Data that does not have a significant impact on the predictive power of the model or the insights derived from the analysis is considered irrelevant.
Automated Techniques
Easylibpal uses automated tools and methods to remove irrelevant data. This includes filtering techniques to select or exclude certain rows or columns based on criteria or conditions, aggregating data to reduce its complexity, and deduplicating to remove duplicate entries. Tools like Excel, Google Sheets, Tableau, Power BI, OpenRefine, Python, R, Data Linter, Data Cleaner, and Data Wrangler can be employed for these purposes .
Examples of Irrelevant Data
- Personal Identifiable Information (PII): Data such as names, addresses, and phone numbers are irrelevant for most analytical purposes and should be removed to protect privacy and comply with data protection regulations .
- URLs and HTML Tags: These are typically not relevant to the analysis and can be removed to clean up the dataset.
- Boilerplate Text: Excessive blank space or boilerplate text (e.g., in emails) adds noise to the data and can be removed.
- Tracking Codes: These are used for tracking user interactions and do not contribute to the analysis.
To implement these steps in Python, Easylibpal might use pandas for data manipulation and filtering. Here's a conceptual example of how to remove irrelevant data:
```python
import pandas as pd
# Load the dataset
dataset = pd.read_csv('your_dataset.csv')
# Remove irrelevant columns (example: email addresses)
dataset = dataset.drop(['email_address'], axis=1)
# Remove rows with missing values (example: if a column is required for analysis)
dataset = dataset.dropna(subset=['required_column'])
# Deduplicate data
dataset = dataset.drop_duplicates()
# Return the cleaned dataset
cleaned_dataset = dataset
```
This example demonstrates how Easylibpal might remove irrelevant data from a dataset using Python and pandas. The actual implementation would need to adapt these steps based on the specific characteristics and requirements of the dataset being processed.
Detecting Inconsistencies
Easylibpal starts by detecting inconsistencies in the data. This involves identifying discrepancies in data types, missing values, duplicates, and formatting errors. By detecting these inconsistencies, Easylibpal can take targeted actions to address them.
Handling Formatting Errors
Formatting errors, such as inconsistent data types for the same feature, can significantly impact the analysis. Easylibpal uses functions like `astype()` in pandas to convert data types, ensuring uniformity and consistency across the dataset. This step is crucial for preparing the data for analysis, as it ensures that each feature is in the correct format expected by the algorithms.
Handling Missing Values
Missing values are a common issue in datasets. Easylibpal addresses this by consulting with subject matter experts to understand why data might be missing. If the missing data is missing completely at random, Easylibpal might choose to drop it. However, for other cases, Easylibpal might employ imputation techniques to fill in missing values, ensuring that the dataset is complete and ready for analysis.
Handling Duplicates
Duplicate entries can skew the analysis and lead to incorrect conclusions. Easylibpal uses pandas to identify and remove duplicates, ensuring that each entry in the dataset is unique. This step is crucial for maintaining the integrity of the data and ensuring that the analysis is based on distinct observations.
Handling Inconsistent Values
Inconsistent values, such as different representations of the same concept (e.g., "yes" vs. "y" for a binary variable), can also pose challenges. Easylibpal employs data cleaning techniques to standardize these values, ensuring that the data is consistent and can be accurately analyzed.
To implement these steps in Python, Easylibpal would leverage pandas for data manipulation and preprocessing. Here's a conceptual example of how these steps might be integrated into the Easylibpal class:
```python
import pandas as pd
class Easylibpal:
def __init__(self, dataset):
self.dataset = dataset
# Load and preprocess the dataset
def clean_and_preprocess(self):
# Detect inconsistencies (example: check data types)
print(self.dataset.dtypes)
# Handle formatting errors (example: convert data types)
self.dataset['date_column'] = pd.to_datetime(self.dataset['date_column'])
# Handle missing values (example: drop rows with missing values)
self.dataset = self.dataset.dropna(subset=['required_column'])
# Handle duplicates (example: drop duplicates)
self.dataset = self.dataset.drop_duplicates()
# Handle inconsistent values (example: standardize values)
self.dataset['binary_column'] = self.dataset['binary_column'].map({'yes': 1, 'no': 0})
# Return the cleaned and preprocessed dataset
return self.dataset
# Usage
Easylibpal = Easylibpal(dataset=pd.read_csv('your_dataset.csv'))
cleaned_dataset = Easylibpal.clean_and_preprocess()
```
This example demonstrates a simplified approach to handling inconsistent or messy data within Easylibpal. The actual implementation would need to adapt these steps based on the specific characteristics and requirements of the dataset being processed.
Statistical Imputation
Statistical imputation involves replacing missing values with statistical estimates such as the mean, median, or mode of the available data. This method is straightforward and can be effective for numerical data. For categorical data, mode imputation is commonly used. The choice of imputation method depends on the distribution of the data and the nature of the missing values.
Model-Based Imputation
Model-based imputation uses machine learning models to predict missing values. This approach can be more sophisticated and potentially more accurate than statistical imputation, especially for complex datasets. Techniques like K-Nearest Neighbors (KNN) imputation can be used, where the missing values are replaced with the values of the K nearest neighbors in the feature space.
Using SimpleImputer in scikit-learn
The scikit-learn library provides the `SimpleImputer` class, which supports both statistical and model-based imputation. `SimpleImputer` can be used to replace missing values with the mean, median, or most frequent value (mode) of the column. It also supports more advanced imputation methods like KNN imputation.
To implement these imputation techniques in Python, Easylibpal might use the `SimpleImputer` class from scikit-learn. Here's an example of how to use `SimpleImputer` for statistical imputation:
```python
from sklearn.impute import SimpleImputer
import pandas as pd
# Load the dataset
dataset = pd.read_csv('your_dataset.csv')
# Initialize SimpleImputer for numerical columns
num_imputer = SimpleImputer(strategy='mean')
# Fit and transform the numerical columns
dataset'numerical_column1', 'numerical_column2' = num_imputer.fit_transform(dataset'numerical_column1', 'numerical_column2')
# Initialize SimpleImputer for categorical columns
cat_imputer = SimpleImputer(strategy='most_frequent')
# Fit and transform the categorical columns
dataset'categorical_column1', 'categorical_column2' = cat_imputer.fit_transform(dataset'categorical_column1', 'categorical_column2')
# The dataset now has missing values imputed
```
This example demonstrates how to use `SimpleImputer` to fill in missing values in both numerical and categorical columns of a dataset. The actual implementation would need to adapt these steps based on the specific characteristics and requirements of the dataset being processed.
Model-based imputation techniques, such as Multiple Imputation by Chained Equations (MICE), offer powerful ways to handle missing data by using statistical models to predict missing values. However, these techniques come with their own set of limitations and potential drawbacks:
1. Complexity and Computational Cost
Model-based imputation methods can be computationally intensive, especially for large datasets or complex models. This can lead to longer processing times and increased computational resources required for imputation.
2. Overfitting and Convergence Issues
These methods are prone to overfitting, where the imputation model captures noise in the data rather than the underlying pattern. Overfitting can lead to imputed values that are too closely aligned with the observed data, potentially introducing bias into the analysis. Additionally, convergence issues may arise, where the imputation process does not settle on a stable solution.
3. Assumptions About Missing Data
Model-based imputation techniques often assume that the data is missing at random (MAR), which means that the probability of a value being missing is not related to the values of other variables. However, this assumption may not hold true in all cases, leading to biased imputations if the data is missing not at random (MNAR).
4. Need for Suitable Regression Models
For each variable with missing values, a suitable regression model must be chosen. Selecting the wrong model can lead to inaccurate imputations. The choice of model depends on the nature of the data and the relationship between the variable with missing values and other variables.
5. Combining Imputed Datasets
After imputing missing values, there is a challenge in combining the multiple imputed datasets to produce a single, final dataset. This requires careful consideration of how to aggregate the imputed values and can introduce additional complexity and uncertainty into the analysis.
6. Lack of Transparency
The process of model-based imputation can be less transparent than simpler imputation methods, such as mean or median imputation. This can make it harder to justify the imputation process, especially in contexts where the reasons for missing data are important, such as in healthcare research.
Despite these limitations, model-based imputation techniques can be highly effective for handling missing data in datasets where a amusingness is MAR and where the relationships between variables are complex. Careful consideration of the assumptions, the choice of models, and the methods for combining imputed datasets are crucial to mitigate these drawbacks and ensure the validity of the imputation process.
USING EASYLIBPAL FOR AI ALGORITHM INTEGRATION OFFERS SEVERAL SIGNIFICANT BENEFITS, PARTICULARLY IN ENHANCING EVERYDAY LIFE AND REVOLUTIONIZING VARIOUS SECTORS. HERE'S A DETAILED LOOK AT THE ADVANTAGES:
1. Enhanced Communication: AI, through Easylibpal, can significantly improve communication by categorizing messages, prioritizing inboxes, and providing instant customer support through chatbots. This ensures that critical information is not missed and that customer queries are resolved promptly.
2. Creative Endeavors: Beyond mundane tasks, AI can also contribute to creative endeavors. For instance, photo editing applications can use AI algorithms to enhance images, suggesting edits that align with aesthetic preferences. Music composition tools can generate melodies based on user input, inspiring musicians and amateurs alike to explore new artistic horizons. These innovations empower individuals to express themselves creatively with AI as a collaborative partner.
3. Daily Life Enhancement: AI, integrated through Easylibpal, has the potential to enhance daily life exponentially. Smart homes equipped with AI-driven systems can adjust lighting, temperature, and security settings according to user preferences. Autonomous vehicles promise safer and more efficient commuting experiences. Predictive analytics can optimize supply chains, reducing waste and ensuring goods reach users when needed.
4. Paradigm Shift in Technology Interaction: The integration of AI into our daily lives is not just a trend; it's a paradigm shift that's redefining how we interact with technology. By streamlining routine tasks, personalizing experiences, revolutionizing healthcare, enhancing communication, and fueling creativity, AI is opening doors to a more convenient, efficient, and tailored existence.
5. Responsible Benefit Harnessing: As we embrace AI's transformational power, it's essential to approach its integration with a sense of responsibility, ensuring that its benefits are harnessed for the betterment of society as a whole. This approach aligns with the ethical considerations of using AI, emphasizing the importance of using AI in a way that benefits all stakeholders.
In summary, Easylibpal facilitates the integration and use of AI algorithms in a manner that is accessible and beneficial across various domains, from enhancing communication and creative endeavors to revolutionizing daily life and promoting a paradigm shift in technology interaction. This integration not only streamlines the application of AI but also ensures that its benefits are harnessed responsibly for the betterment of society.
USING EASYLIBPAL OVER TRADITIONAL AI LIBRARIES OFFERS SEVERAL BENEFITS, PARTICULARLY IN TERMS OF EASE OF USE, EFFICIENCY, AND THE ABILITY TO APPLY AI ALGORITHMS WITH MINIMAL CONFIGURATION. HERE ARE THE KEY ADVANTAGES:
- Simplified Integration: Easylibpal abstracts the complexity of traditional AI libraries, making it easier for users to integrate classic AI algorithms into their projects. This simplification reduces the learning curve and allows developers and data scientists to focus on their core tasks without getting bogged down by the intricacies of AI implementation.
- User-Friendly Interface: By providing a unified platform for various AI algorithms, Easylibpal offers a user-friendly interface that streamlines the process of selecting and applying algorithms. This interface is designed to be intuitive and accessible, enabling users to experiment with different algorithms with minimal effort.
- Enhanced Productivity: The ability to effortlessly instantiate algorithms, fit models with training data, and make predictions with minimal configuration significantly enhances productivity. This efficiency allows for rapid prototyping and deployment of AI solutions, enabling users to bring their ideas to life more quickly.
- Democratization of AI: Easylibpal democratizes access to classic AI algorithms, making them accessible to a wider range of users, including those with limited programming experience. This democratization empowers users to leverage AI in various domains, fostering innovation and creativity.
- Automation of Repetitive Tasks: By automating the process of applying AI algorithms, Easylibpal helps users save time on repetitive tasks, allowing them to focus on more complex and creative aspects of their projects. This automation is particularly beneficial for users who may not have extensive experience with AI but still wish to incorporate AI capabilities into their work.
- Personalized Learning and Discovery: Easylibpal can be used to enhance personalized learning experiences and discovery mechanisms, similar to the benefits seen in academic libraries. By analyzing user behaviors and preferences, Easylibpal can tailor recommendations and resource suggestions to individual needs, fostering a more engaging and relevant learning journey.
- Data Management and Analysis: Easylibpal aids in managing large datasets efficiently and deriving meaningful insights from data. This capability is crucial in today's data-driven world, where the ability to analyze and interpret large volumes of data can significantly impact research outcomes and decision-making processes.
In summary, Easylibpal offers a simplified, user-friendly approach to applying classic AI algorithms, enhancing productivity, democratizing access to AI, and automating repetitive tasks. These benefits make Easylibpal a valuable tool for developers, data scientists, and users looking to leverage AI in their projects without the complexities associated with traditional AI libraries.
2 notes
·
View notes