#Intelligent architecture building
Explore tagged Tumblr posts
Text
The Future of Architecture: What You Need to Know
Architecture is a constantly evolving field, and the future of architecture is full of possibilities. Here are some of the trends that are expected to shape the future of architecture:
Sustainable architecture: As the world becomes increasingly aware of the environmental impact of buildings, sustainable architecture is becoming increasingly important. Sustainable buildings are designed to use less energy and resources, and they often use renewable energy sources.
Intelligent architecture: Intelligent architecture uses technology to create buildings that are more efficient and user-friendly. These buildings may use sensors to monitor energy usage and adjust accordingly, or they may use artificial intelligence to provide occupants with personalized services.
Adaptive architecture: Adaptive architecture is designed to respond to changes in the environment. These buildings may be able to adjust their shape or orientation to track the sun, or they may be able to collect rainwater or solar energy.
3D printing: 3D printing is already being used to create architectural models and prototypes, and it is expected to play an increasingly important role in the construction of buildings in the future. 3D printing can be used to create complex shapes and structures that would be difficult or impossible to build using traditional methods.
Virtual reality: Virtual reality (VR) is being used in architecture to allow clients to experience a building before it is even built. VR can be used to provide clients with a 360-degree view of a building, and it can also be used to simulate how light and sound will behave in a building.
These are just a few of the trends that are expected to shape the future of architecture. As technology continues to evolve, so too will the field of architecture. Architects who are able to embrace new technologies and trends will be well-positioned for success in the years to come.
The College of Architecture, MLSU is one of the leading colleges of architecture in India. The college offers a variety of undergraduate and postgraduate programs in architecture. The college's curriculum is designed to prepare students for the future of architecture by teaching them about sustainable architecture, intelligent architecture, adaptive architecture, 3D printing, and virtual reality. The college also has a strong research program that is focused on developing new technologies and techniques for architecture.
If you are interested in a career in architecture, the College of Architecture, MLSU is a great option. The college's strong curriculum and research program will prepare you for the future of architecture and give you the skills you need to succeed in this rapidly evolving field.
#sustainablearchitecture#Sustainable architecture building#intelligentarchitecture#Intelligent architecture building#adaptivearchitecture#3dprintingarchitecture#architecturecollege#mlsu#architecturecareer
0 notes
Text
Created by DALL•E 3
#photography#art#fashion#beauty#anime#nature#architecture#artificial intelligence#landscape#interiors#design#graphic design#buildings#furniture#product design#home design#loft#bathroom#minimalism#furniture design
2K notes
·
View notes
Note
Okay so I've been wanting to tell you that you're literally my favourite twst artist 😭🩷
So my question is, how do you manage to come up with these funny comics? CUZ I LOVE THEM SO MUCH
(P.s: Lovin' the art style ✨)
oh geeze, thanks! 💚💚💚 I'm really glad people enjoy my stupid sense of humor; mostly I just draw things to make myself laugh, and if it makes other people laugh too, then bonus points! usually it's just one joke or mental image that gets stuck in my head (every time I saw Fellow spin his cane, all I could think about was him go-go-gadgeting away on it...) and in my quest to justify it, it picks up other jokes and bits along the way and usually doesn't even end up as the main focus anymore. entire narrative arcs have spun out just so I could use a single bad pun in a throwaway line. this is a terrible way to explain it but I'm not sure how else to put it into words!
and sometimes it's just "weird things my sister has said that I make fun of her for"
#twisted wonderland#reposting old art but i will never not make fun of her for her incredibly pretentious opinions about the local mall's architectural design#yuu listening to malleus be weird about buildings is supposed to be relateable right#that said this is also the only place i get to talk about twst so it is also just...a lot of my thoughts spilling out everywhere#(sorry other fandoms)#(i have spaces where i can debate the various merits of himeno/rita versus morfonia/rita)#(the correct answer is that rita has two hands but i digress)#but where else can i talk at length about these anime disney dipshits who have flawless eyeliner and zero emotional intelligence#me at the top of my lungs: you don't understand that's his DAD#the group dm: w...what are you talking about#there's gonna be a few posts today sorry#gotta get some stuff out before the update tonight/tomorrow morning/whatever happens and becomes all i think about for the next week
2K notes
·
View notes
Text
Translating MIT research into real-world results
New Post has been published on https://thedigitalinsider.com/translating-mit-research-into-real-world-results/
Translating MIT research into real-world results


Inventive solutions to some of the world’s most critical problems are being discovered in labs, classrooms, and centers across MIT every day. Many of these solutions move from the lab to the commercial world with the help of over 85 Institute resources that comprise MIT’s robust innovation and entrepreneurship (I&E) ecosystem. The Abdul Latif Jameel Water and Food Systems Lab (J-WAFS) draws on MIT’s wealth of I&E knowledge and experience to help researchers commercialize their breakthrough technologies through the J-WAFS Solutions grant program. By collaborating with I&E programs on campus, J-WAFS prepares MIT researchers for the commercial world, where their novel innovations aim to improve productivity, accessibility, and sustainability of water and food systems, creating economic, environmental, and societal benefits along the way.
The J-WAFS Solutions program launched in 2015 with support from Community Jameel, an international organization that advances science and learning for communities to thrive. Since 2015, J-WAFS Solutions has supported 19 projects with one-year grants of up to $150,000, with some projects receiving renewal grants for a second year of support. Solutions projects all address challenges related to water or food. Modeled after the esteemed grant program of MIT’s Deshpande Center for Technological Innovation, and initially administered by Deshpande Center staff, the J-WAFS Solutions program follows a similar approach by supporting projects that have already completed the basic research and proof-of-concept phases. With technologies that are one to three years away from commercialization, grantees work on identifying their potential markets and learn to focus on how their technology can meet the needs of future customers.
“Ingenuity thrives at MIT, driving inventions that can be translated into real-world applications for widespread adoption, implantation, and use,” says J-WAFS Director Professor John H. Lienhard V. “But successful commercialization of MIT technology requires engineers to focus on many challenges beyond making the technology work. MIT’s I&E network offers a variety of programs that help researchers develop technology readiness, investigate markets, conduct customer discovery, and initiate product design and development,” Lienhard adds. “With this strong I&E framework, many J-WAFS Solutions teams have established startup companies by the completion of the grant. J-WAFS-supported technologies have had powerful, positive effects on human welfare. Together, the J-WAFS Solutions program and MIT’s I&E ecosystem demonstrate how academic research can evolve into business innovations that make a better world,” Lienhard says.
Creating I&E collaborations
In addition to support for furthering research, J-WAFS Solutions grants allow faculty, students, postdocs, and research staff to learn the fundamentals of how to transform their work into commercial products and companies. As part of the grant requirements, researchers must interact with mentors through MIT Venture Mentoring Service (VMS). VMS connects MIT entrepreneurs with teams of carefully selected professionals who provide free and confidential mentorship, guidance, and other services to help advance ideas into for-profit, for-benefit, or nonprofit ventures. Since 2000, VMS has mentored over 4,600 MIT entrepreneurs across all industries, through a dynamic and accomplished group of nearly 200 mentors who volunteer their time so that others may succeed. The mentors provide impartial and unbiased advice to members of the MIT community, including MIT alumni in the Boston area. J-WAFS Solutions teams have been guided by 21 mentors from numerous companies and nonprofits. Mentors often attend project events and progress meetings throughout the grant period.
“Working with VMS has provided me and my organization with a valuable sounding board for a range of topics, big and small,” says Eric Verploegen PhD ’08, former research engineer in MIT’s D-Lab and founder of J-WAFS spinout CoolVeg. Along with professors Leon Glicksman and Daniel Frey, Verploegen received a J-WAFS Solutions grant in 2021 to commercialize cold-storage chambers that use evaporative cooling to help farmers preserve fruits and vegetables in rural off-grid communities. Verploegen started CoolVeg in 2022 to increase access and adoption of open-source, evaporative cooling technologies through collaborations with businesses, research institutions, nongovernmental organizations, and government agencies. “Working as a solo founder at my nonprofit venture, it is always great to have avenues to get feedback on communications approaches, overall strategy, and operational issues that my mentors have experience with,” Verploegen says. Three years after the initial Solutions grant, one of the VMS mentors assigned to the evaporative cooling team still acts as a mentor to Verploegen today.
Another Solutions grant requirement is for teams to participate in the Spark program — a free, three-week course that provides an entry point for researchers to explore the potential value of their innovation. Spark is part of the National Science Foundation’s (NSF) Innovation Corps (I-Corps), which is an “immersive, entrepreneurial training program that facilitates the transformation of invention to impact.” In 2018, MIT received an award from the NSF, establishing the New England Regional Innovation Corps Node (NE I-Corps) to deliver I-Corps training to participants across New England. Trainings are open to researchers, engineers, scientists, and others who want to engage in a customer discovery process for their technology. Offered regularly throughout the year, the Spark course helps participants identify markets and explore customer needs in order to understand how their technologies can be positioned competitively in their target markets. They learn to assess barriers to adoption, as well as potential regulatory issues or other challenges to commercialization. NE-I-Corps reports that since its start, over 1,200 researchers from MIT have completed the program and have gone on to launch 175 ventures, raising over $3.3 billion in funding from grants and investors, and creating over 1,800 jobs.
Constantinos Katsimpouras, a research scientist in the Department of Chemical Engineering, went through the NE I-Corps Spark program to better understand the customer base for a technology he developed with professors Gregory Stephanopoulos and Anthony Sinskey. The group received a J-WAFS Solutions grant in 2021 for their microbial platform that converts food waste from the dairy industry into valuable products. “As a scientist with no prior experience in entrepreneurship, the program introduced me to important concepts and tools for conducting customer interviews and adopting a new mindset,” notes Katsimpouras. “Most importantly, it encouraged me to get out of the building and engage in interviews with potential customers and stakeholders, providing me with invaluable insights and a deeper understanding of my industry,” he adds. These interviews also helped connect the team with companies willing to provide resources to test and improve their technology — a critical step to the scale-up of any lab invention.
In the case of Professor Cem Tasan’s research group in the Department of Materials Science and Engineering, the I-Corps program led them to the J-WAFS Solutions grant, instead of the other way around. Tasan is currently working with postdoc Onur Guvenc on a J-WAFS Solutions project to manufacture formable sheet metal by consolidating steel scrap without melting, thereby reducing water use compared to traditional steel processing. Before applying for the Solutions grant, Guvenc took part in NE I-Corps. Like Katsimpouras, Guvenc benefited from the interaction with industry. “This program required me to step out of the lab and engage with potential customers, allowing me to learn about their immediate challenges and test my initial assumptions about the market,” Guvenc recalls. “My interviews with industry professionals also made me aware of the connection between water consumption and steelmaking processes, which ultimately led to the J-WAFS 2023 Solutions Grant,” says Guvenc.
After completing the Spark program, participants may be eligible to apply for the Fusion program, which provides microgrants of up to $1,500 to conduct further customer discovery. The Fusion program is self-paced, requiring teams to conduct 12 additional customer interviews and craft a final presentation summarizing their key learnings. Professor Patrick Doyle’s J-WAFS Solutions team completed the Spark and Fusion programs at MIT. Most recently, their team was accepted to join the NSF I-Corps National program with a $50,000 award. The intensive program requires teams to complete an additional 100 customer discovery interviews over seven weeks. Located in the Department of Chemical Engineering, the Doyle lab is working on a sustainable microparticle hydrogel system to rapidly remove micropollutants from water. The team’s focus has expanded to higher value purifications in amino acid and biopharmaceutical manufacturing applications. Devashish Gokhale PhD ’24 worked with Doyle on much of the underlying science.
“Our platform technology could potentially be used for selective separations in very diverse market segments, ranging from individual consumers to large industries and government bodies with varied use-cases,” Gokhale explains. He goes on to say, “The I-Corps Spark program added significant value by providing me with an effective framework to approach this problem … I was assigned a mentor who provided critical feedback, teaching me how to formulate effective questions and identify promising opportunities.” Gokhale says that by the end of Spark, the team was able to identify the best target markets for their products. He also says that the program provided valuable seminars on topics like intellectual property, which was helpful in subsequent discussions the team had with MIT’s Technology Licensing Office.
Another member of Doyle’s team, Arjav Shah, a recent PhD from MIT’s Department of Chemical Engineering and a current MBA candidate at the MIT Sloan School of Management, is spearheading the team’s commercialization plans. Shah attended Fusion last fall and hopes to lead efforts to incorporate a startup company called hydroGel. “I admire the hypothesis-driven approach of the I-Corps program,” says Shah. “It has enabled us to identify our customers’ biggest pain points, which will hopefully lead us to finding a product-market fit.” He adds “based on our learnings from the program, we have been able to pivot to impact-driven, higher-value applications in the food processing and biopharmaceutical industries.” Postdoc Luca Mazzaferro will lead the technical team at hydroGel alongside Shah.
In a different project, Qinmin Zheng, a postdoc in the Department of Civil and Environmental Engineering, is working with Professor Andrew Whittle and Lecturer Fábio Duarte. Zheng plans to take the Fusion course this fall to advance their J-WAFS Solutions project that aims to commercialize a novel sensor to quantify the relative abundance of major algal species and provide early detection of harmful algal blooms. After completing Spark, Zheng says he’s “excited to participate in the Fusion program, and potentially the National I-Corps program, to further explore market opportunities and minimize risks in our future product development.”
Economic and societal benefits
Commercializing technologies developed at MIT is one of the ways J-WAFS helps ensure that MIT research advances will have real-world impacts in water and food systems. Since its inception, the J-WAFS Solutions program has awarded 28 grants (including renewals), which have supported 19 projects that address a wide range of global water and food challenges. The program has distributed over $4 million to 24 professors, 11 research staff, 15 postdocs, and 30 students across MIT. Nearly half of all J-WAFS Solutions projects have resulted in spinout companies or commercialized products, including eight companies to date plus two open-source technologies.
Nona Technologies is an example of a J-WAFS spinout that is helping the world by developing new approaches to produce freshwater for drinking. Desalination — the process of removing salts from seawater — typically requires a large-scale technology called reverse osmosis. But Nona created a desalination device that can work in remote off-grid locations. By separating salt and bacteria from water using electric current through a process called ion concentration polarization (ICP), their technology also reduces overall energy consumption. The novel method was developed by Jongyoon Han, professor of electrical engineering and biological engineering, and research scientist Junghyo Yoon. Along with Bruce Crawford, a Sloan MBA alum, Han and Yoon created Nona Technologies to bring their lightweight, energy-efficient desalination technology to the market.
“My feeling early on was that once you have technology, commercialization will take care of itself,” admits Crawford. The team completed both the Spark and Fusion programs and quickly realized that much more work would be required. “Even in our first 24 interviews, we learned that the two first markets we envisioned would not be viable in the near term, and we also got our first hints at the beachhead we ultimately selected,” says Crawford. Nona Technologies has since won MIT’s $100K Entrepreneurship Competition, received media attention from outlets like Newsweek and Fortune, and hired a team that continues to further the technology for deployment in resource-limited areas where clean drinking water may be scarce.
Food-borne diseases sicken millions of people worldwide each year, but J-WAFS researchers are addressing this issue by integrating molecular engineering, nanotechnology, and artificial intelligence to revolutionize food pathogen testing. Professors Tim Swager and Alexander Klibanov, of the Department of Chemistry, were awarded one of the first J-WAFS Solutions grants for their sensor that targets food safety pathogens. The sensor uses specialized droplets that behave like a dynamic lens, changing in the presence of target bacteria in order to detect dangerous bacterial contamination in food. In 2018, Swager launched Xibus Systems Inc. to bring the sensor to market and advance food safety for greater public health, sustainability, and economic security.
“Our involvement with the J-WAFS Solutions Program has been vital,” says Swager. “It has provided us with a bridge between the academic world and the business world and allowed us to perform more detailed work to create a usable application,” he adds. In 2022, Xibus developed a product called XiSafe, which enables the detection of contaminants like salmonella and listeria faster and with higher sensitivity than other food testing products. The innovation could save food processors billions of dollars worldwide and prevent thousands of food-borne fatalities annually.
J-WAFS Solutions companies have raised nearly $66 million in venture capital and other funding. Just this past June, J-WAFS spinout SiTration announced that it raised an $11.8 million seed round. Jeffrey Grossman, a professor in MIT’s Department of Materials Science and Engineering, was another early J-WAFS Solutions grantee for his work on low-cost energy-efficient filters for desalination. The project enabled the development of nanoporous membranes and resulted in two spinout companies, Via Separations and SiTration. SiTration was co-founded by Brendan Smith PhD ’18, who was a part of the original J-WAFS team. Smith is CEO of the company and has overseen the advancement of the membrane technology, which has gone on to reduce cost and resource consumption in industrial wastewater treatment, advanced manufacturing, and resource extraction of materials such as lithium, cobalt, and nickel from recycled electric vehicle batteries. The company also recently announced that it is working with the mining company Rio Tinto to handle harmful wastewater generated at mines.
But it’s not just J-WAFS spinout companies that are producing real-world results. Products like the ECC Vial — a portable, low-cost method for E. coli detection in water — have been brought to the market and helped thousands of people. The test kit was developed by MIT D-Lab Lecturer Susan Murcott and Professor Jeffrey Ravel of the MIT History Section. The duo received a J-WAFS Solutions grant in 2018 to promote safely managed drinking water and improved public health in Nepal, where it is difficult to identify which wells are contaminated by E. coli. By the end of their grant period, the team had manufactured approximately 3,200 units, of which 2,350 were distributed — enough to help 12,000 people in Nepal. The researchers also trained local Nepalese on best manufacturing practices.
“It’s very important, in my life experience, to follow your dream and to serve others,” says Murcott. Economic success is important to the health of any venture, whether it’s a company or a product, but equally important is the social impact — a philosophy that J-WAFS research strives to uphold. “Do something because it’s worth doing and because it changes people’s lives and saves lives,” Murcott adds.
As J-WAFS prepares to celebrate its 10th anniversary this year, we look forward to continued collaboration with MIT’s many I&E programs to advance knowledge and develop solutions that will have tangible effects on the world’s water and food systems.
Learn more about the J-WAFS Solutions program and about innovation and entrepreneurship at MIT.
#000#2022#2023#Accessibility#adoption#Advice#agriculture#amp#anniversary#applications#approach#architecture#artificial#Artificial Intelligence#attention#Bacteria#batteries#billion#Biological engineering#Biology#board#bridge#Building#Business#CEO#chemical#Chemical engineering#chemistry#Civil and environmental engineering#climate change
3 notes
·
View notes
Text
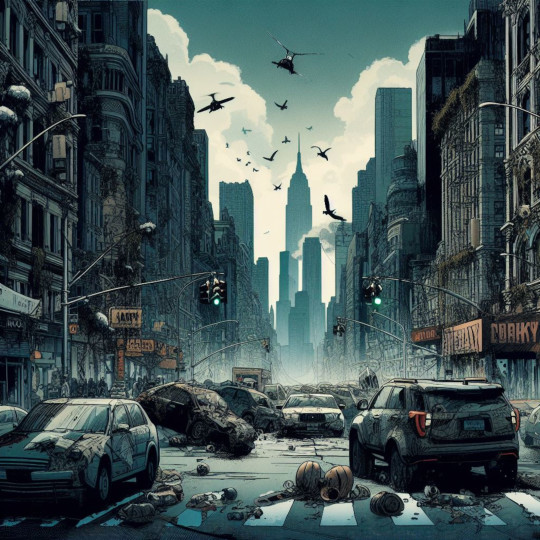



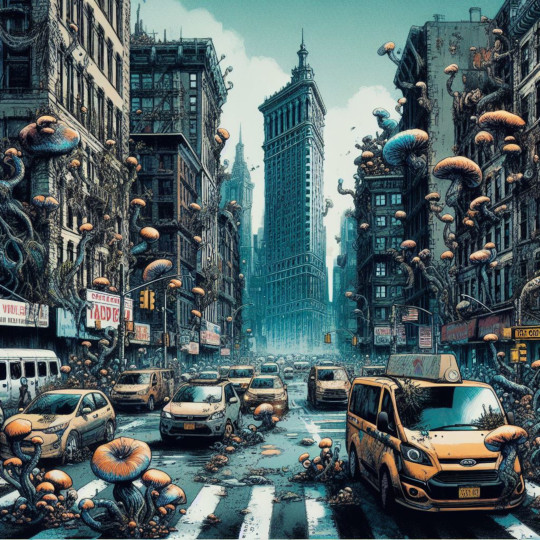
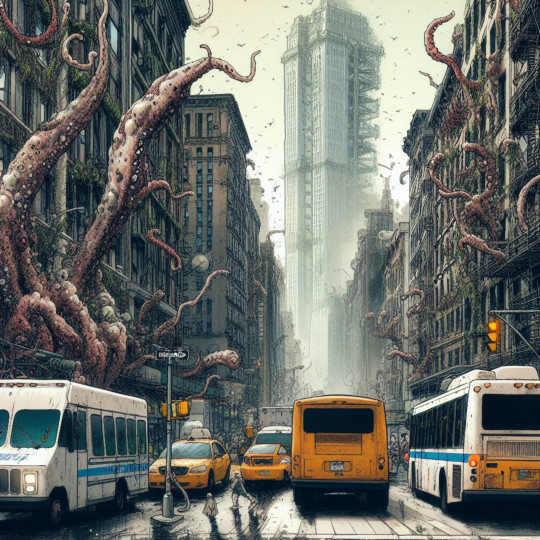
New York AD
#art#ai#ai artwork#digital art#ai art#city#urban#architecture#buildings#ai generated#ai image#artificial intelligence#chatgpt#technology#new york#nyc#new york city#brooklyn#manhattan#apocalypse#post apocalyptic#graphic novel#comic art
16 notes
·
View notes
Photo







‘When Life imitates Art’
@ Marcus Byrne
#art#design#architecture#surreal#graffiti#life#marcus byrne#conceptual#building#AI#artificial intelligence#aabndoned#abandoned places#abandoned building#decay#streetart
39 notes
·
View notes
Text
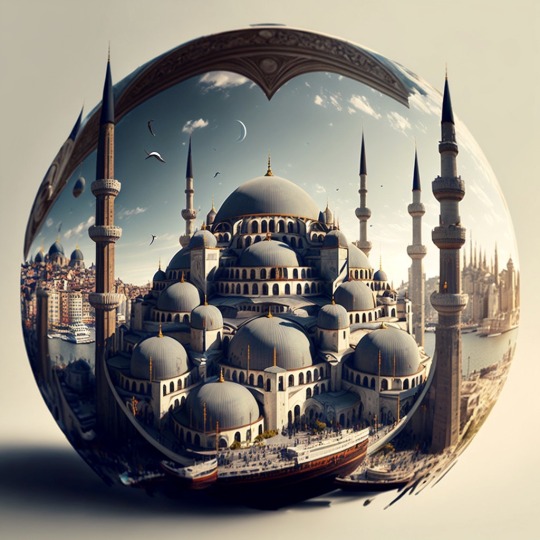
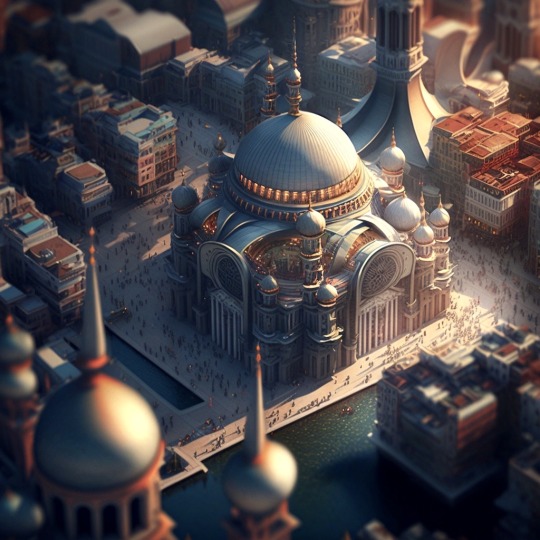
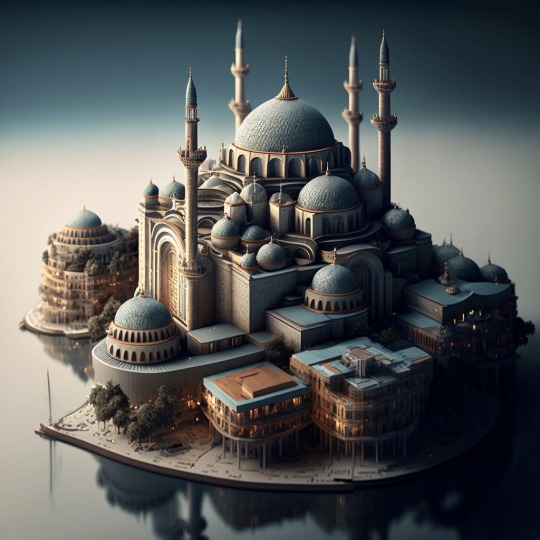
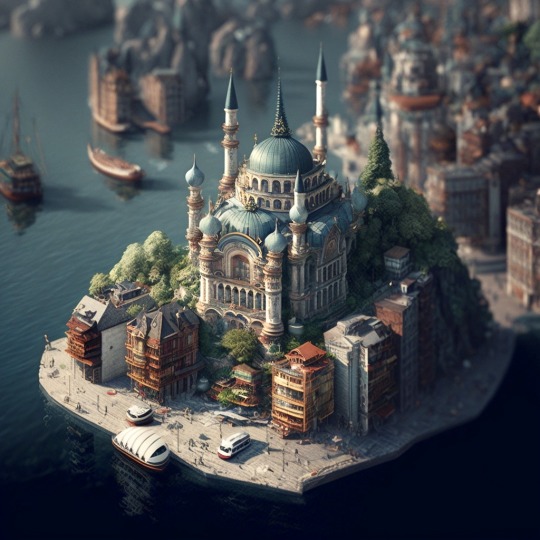
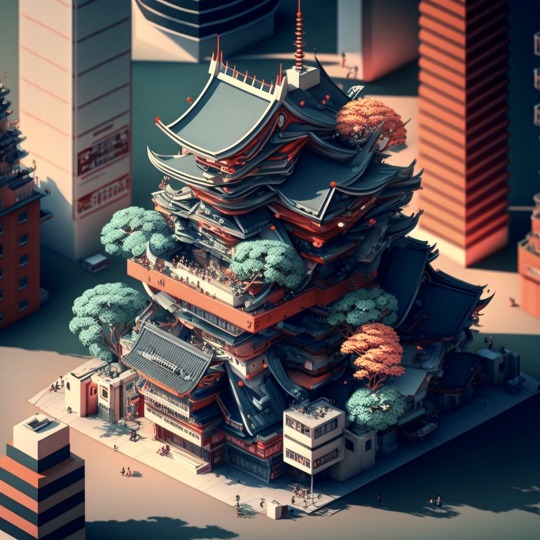
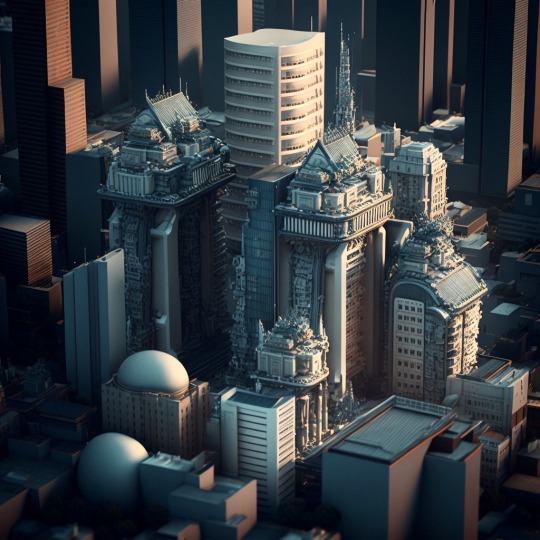
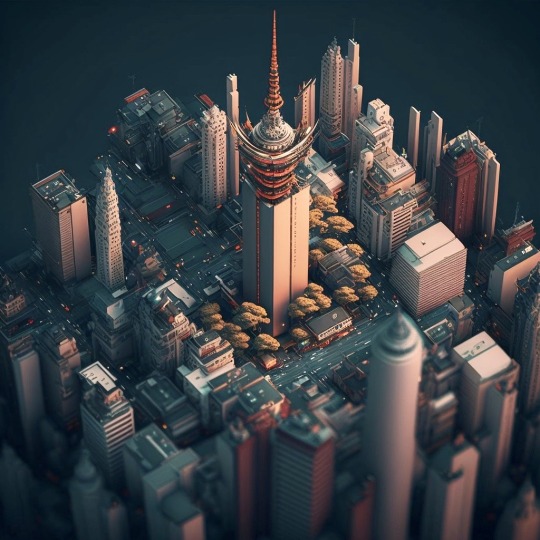
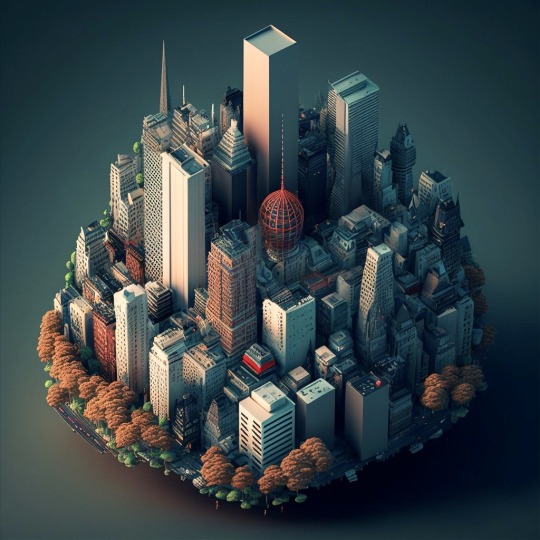
3D Cities: Istanbul & Tokyo
#art#digital art#3d#city#buildings#house#architecture#cityscape#ai#ai art#ai generated#ai art gallery#ai art community#artificial intelligence#mightyoakai#midjourney#midjourney ai#midjourney art#istanbul#tokyo
12 notes
·
View notes
Text
New Construction study I, 10
Follow me, — says Visual Ratatosk
#style rétro#modèles de diffusion#intelligence artificielle#generativ#generative Kunst#KI-Kunst#kollektive Erinnerungen#Vintage-Stil#Retro-Stil#Diffusionsmodelle#künstliche Intelligenz#generativo#arte generativo#arte de IA#Architecture#Arquitetura#Architektur#建築#アーキテクチャ#buildings#bâtiments#Gebäude#edifícios#建物#AI#generative#generative art#ai art#Midjourney#neurophotography
3 notes
·
View notes
Text
youtube
#john hardy#bamboo#building with bamboo#designing with bamboo#growing bamboo#why grow bamboo#cultivating bamboo#bamboo cultivation#why bamboo#why build with bamboo#why use bamboo#permaculture#regenerative agriculture#organic farming#architecture#alternative architecture#free architecture#intelligent architecture#free thinkers#innovators#artist builders#the art of architecture
2 notes
·
View notes
Photo

This Artist Tested The Abilities Of A.I. To Create Architectural Paintings In Different Styles
The artist and blogger used the Midjourney neural network, an artificial intelligence-based program that entered open beta testing in mid-July, to see how familiar Russian buildings would look in different styles.
Artist: Alexander Dobrokotov
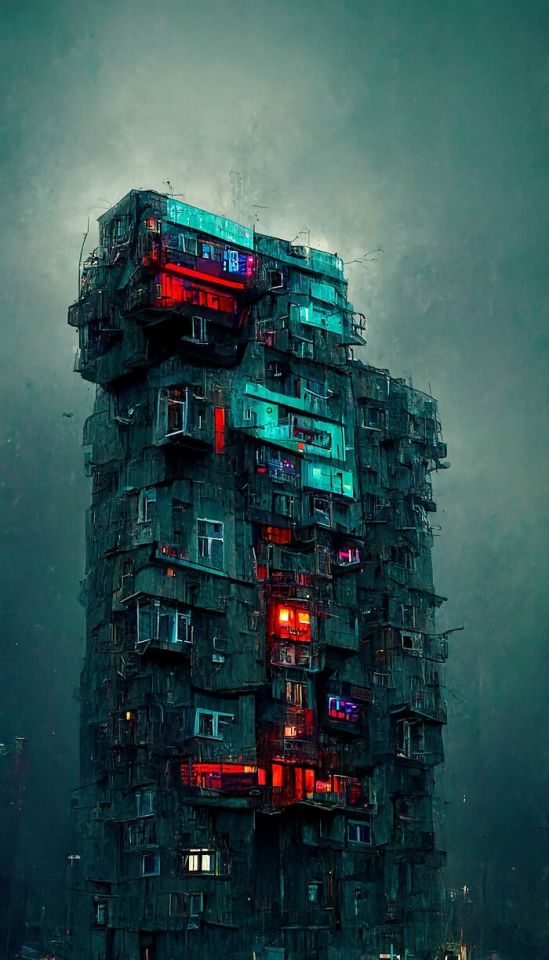

#alexander dobrokotov#artist#art#artificial intelligence#architectural paintings#blogger#midjourney neural network#beta testing#russian buildings
3 notes
·
View notes
Text
#surrealism#surreal#weird#aesthetic#creepy#unsettling#ai artwork#ai image#ai#artificial lemon flavor#artificiallemonflavor#artificial intelligence#ai generated#generative ai#portrait#house#face#head#building#architecture#ai art#ai art gallery#ai artist#weird houses#weirdcore#mine
1 note
·
View note
Text
Smart Çelik Yapı Hakkında
Anahtar Kavramlar Analizi
Çelik Ev (Steel House): Çelik evler, uzun ömürlü ve sağlıklı yapılar olarak tanımlanır. Yangına ve neme dayanıklıdır ve 150 yıl ömre sahiptir. Galvaniz çelikten yapılır.
Tiny House: Küçük, taşınabilir evlerdir. Genellikle hızlı, kaliteli ve uzun ömürlü çözümler sunar.
Modüler Ev (Modular Home): Modüler evler, fabrikada üretilen ve yerinde monte edilen yapılardır. Taşınabilirlikleri ve modüler yapıları ile bilinir.
Prefabrik Ev (Prefabricated House): Fabrikada üretilen ve yerinde monte edilen evlerdir. Hızlı bir şekilde kurulabilir ve maliyet etkin bir çözüm sunar.
Ankara Çelik Evleri (Ankara Steel Homes): Ankara'da çelik ev ve villa modellerinin yapım aşamaları, üretim süreleri ve montaj zamanları hakkında bilgilerin bulunduğu yapılar.
Çelik Yapılar (Steel Structures): Galvaniz çelikten yapılan, yangına ve neme karşı dayanıklı uzun ömürlü yapılardır.
Özet
Bu belgede, çelik evlerin ve diğer modüler yapıların özellikleri ve avantajları ele alınmaktadır. Çelik evlerin yangın ve neme dayanıklı olduğu, 150 yıl ömre sahip olduğu ve galvaniz çelikten yapıldığı vurgulanmaktadır. Tiny House ve modüler yapılar gibi taşınabilir çözümler de hızlı, kaliteli ve uzun ömürlü olarak tanımlanmaktadır. Ayrıca, prefabrik evlerin fabrikada üretilip yerinde monte edilmesiyle hızlı ve maliyet etkin bir çözüm sunduğu belirtilmektedir. Belgede, özellikle Ankara'da çelik ev yapım süreçleri ve montaj süreçlerine dikkat çekilmektedir. Bu anahtar kavramlar, belgede belirtilen yapı türlerinin dayanıklılığı, taşınabilirliği ve ekonomikliği hakkında bilgi verir.
#çelikev#çelikvilla#prefabrik#steelconstruction#ankaraprefabrik#prefabriced#architecture#artificial intelligence#buildings#design
0 notes
Text
Created by DALL•E 3
#photography#art#fashion#beauty#anime#nature#architecture#artificial intelligence#landscape#interiors#design#graphic design#buildings#furniture#product design#home design#loft#bathroom#minimalism#furniture design
891 notes
·
View notes
Text

WBSV
WorldBridge Sport Village is a remarkable mixed-use development located in the rapidly growing area of Chroy Changvar, just 20 minutes away from Phnom Penh's Central Business District. It is a pioneering Sport Village that offers a unique opportunity to blend work and play in a health-conscious environment inspired by international-level sports villages, akin to Olympic athlete villages. It will be the first-ever Sport village that offers you a one-of-a-kind opportunity to experience both work and play in the distinctively healthy atmosphere of an international-level sports village. WorldBridge Sport Village similarly offers a range of landed home living. Properties such as villas, Townhouses, Row Houses, and Shophouses can be found that more than accommodate any family size looking to live in the next big neighborhood in the fastest-growing area of Chroy Changvar.
•Project: WORLD BRIDGE SPORT VILLAGE •Developer: OXLEY-WORLDBRIDGE (CAMBODIA) CO., LTD. •Subsidiary: WB SPORT VILLAGE CO., LTD. •Architectural Manager: Sonetra KETH •Location: Phnom Penh, Cambodia




The condo units offer up to 3-bedroom selections across 12 high-rise blocks with spacious interiors and breathtaking views.
<script async src="https://pagead2.googlesyndication.com/pagead/js/adsbygoogle.js?client=ca-pub-9430617320114361" crossorigin="anonymous"></script>
#Sonetra Keth#Architectural Manager#Architectural Design Manager#BIM Director#BIM Manager#BIM Coordinator#Project Manager#RMIT University Vietnam#Institute of Technology of Cambodia#Real Estate Development#Construction Industry#Building Information Modelling#BIM#AI#Artificial Intelligence#Technology#VDC#Virtual Design#IoT#Machine Learning#C4R#Collaboration for Revit#Cloud Computing and Collaboration Platforms#NETRA#netra#នេត្រា#កេត សុនេត្រា#<meta name=“google-adsense-account” content=“ca-pub-9430617320114361”>#crossorigin=“anonymous”></script>#<script async src=“https://pagead2.googlesyndication.com/pagead/js/adsbygoogle.js?client=ca-pub-9430617320114361”
0 notes
Text
Can AI World Models Really Understand Physical Laws?
New Post has been published on https://thedigitalinsider.com/can-ai-world-models-really-understand-physical-laws/
Can AI World Models Really Understand Physical Laws?
The great hope for vision-language AI models is that they will one day become capable of greater autonomy and versatility, incorporating principles of physical laws in much the same way that we develop an innate understanding of these principles through early experience.
For instance, children’s ball games tend to develop an understanding of motion kinetics, and of the effect of weight and surface texture on trajectory. Likewise, interactions with common scenarios such as baths, spilled drinks, the ocean, swimming pools and other diverse liquid bodies will instill in us a versatile and scalable comprehension of the ways that liquid behaves under gravity.
Even the postulates of less common phenomena – such as combustion, explosions and architectural weight distribution under pressure – are unconsciously absorbed through exposure to TV programs and movies, or social media videos.
By the time we study the principles behind these systems, at an academic level, we are merely ‘retrofitting’ our intuitive (but uninformed) mental models of them.
Masters of One
Currently, most AI models are, by contrast, more ‘specialized’, and many of them are either fine-tuned or trained from scratch on image or video datasets that are quite specific to certain use cases, rather than designed to develop such a general understanding of governing laws.
Others can present the appearance of an understanding of physical laws; but they may actually be reproducing samples from their training data, rather than really understanding the basics of areas such as motion physics in a way that can produce truly novel (and scientifically plausible) depictions from users’ prompts.
At this delicate moment in the productization and commercialization of generative AI systems, it is left to us, and to investors’ scrutiny, to distinguish the crafted marketing of new AI models from the reality of their limitations.
One of November’s most interesting papers, led by Bytedance Research, tackled this issue, exploring the gap between the apparent and real capabilities of ‘all-purpose’ generative models such as Sora.
The work concluded that at the current state of the art, generated output from models of this type are more likely to be aping examples from their training data than actually demonstrating full understanding of the underlying physical constraints that operate in the real world.
The paper states*:
‘[These] models can be easily biased by “deceptive” examples from the training set, leading them to generalize in a “case-based” manner under certain conditions. This phenomenon, also observed in large language models, describes a model’s tendency to reference similar training cases when solving new tasks.
‘For instance, consider a video model trained on data of a high-speed ball moving in uniform linear motion. If data augmentation is performed by horizontally flipping the videos, thereby introducing reverse-direction motion, the model may generate a scenario where a low-speed ball reverses direction after the initial frames, even though this behavior is not physically correct.’
We’ll take a closer look at the paper – titled Evaluating World Models with LLM for Decision Making – shortly. But first, let’s look at the background for these apparent limitations.
Remembrance of Things Past
Without generalization, a trained AI model is little more than an expensive spreadsheet of references to sections of its training data: find the appropriate search term, and you can summon up an instance of that data.
In that scenario, the model is effectively acting as a ‘neural search engine’, since it cannot produce abstract or ‘creative’ interpretations of the desired output, but instead replicates some minor variation of data that it saw during the training process.
This is known as memorization – a controversial problem that arises because truly ductile and interpretive AI models tend to lack detail, while truly detailed models tend to lack originality and flexibility.
The capacity for models affected by memorization to reproduce training data is a potential legal hurdle, in cases where the model’s creators did not have unencumbered rights to use that data; and where benefits from that data can be demonstrated through a growing number of extraction methods.
Because of memorization, traces of non-authorized data can persist, daisy-chained, through multiple training systems, like an indelible and unintended watermark – even in projects where the machine learning practitioner has taken care to ensure that ‘safe’ data is used.
World Models
However, the central usage issue with memorization is that it tends to convey the illusion of intelligence, or suggest that the AI model has generalized fundamental laws or domains, where in fact it is the high volume of memorized data that furnishes this illusion (i.e., the model has so many potential data examples to choose from that it is difficult for a human to tell whether it is regurgitating learned content or whether it has a truly abstracted understanding of the concepts involved in the generation).
This issue has ramifications for the growing interest in world models – the prospect of highly diverse and expensively-trained AI systems that incorporate multiple known laws, and are richly explorable.
World models are of particular interest in the generative image and video space. In 2023 RunwayML began a research initiative into the development and feasibility of such models; DeepMind recently hired one of the originators of the acclaimed Sora generative video to work on a model of this kind; and startups such as Higgsfield are investing significantly in world models for image and video synthesis.
Hard Combinations
One of the promises of new developments in generative video AI systems is the prospect that they can learn fundamental physical laws, such as motion, human kinematics (such as gait characteristics), fluid dynamics, and other known physical phenomena which are, at the very least, visually familiar to humans.
If generative AI could achieve this milestone, it could become capable of producing hyper-realistic visual effects that depict explosions, floods, and plausible collision events across multiple types of object.
If, on the other hand, the AI system has simply been trained on thousands (or hundreds of thousands) of videos depicting such events, it could be capable of reproducing the training data quite convincingly when it was trained on a similar data point to the user’s target query; yet fail if the query combines too many concepts that are, in such a combination, not represented at all in the data.
Further, these limitations would not be immediately apparent, until one pushed the system with challenging combinations of this kind.
This means that a new generative system may be capable of generating viral video content that, while impressive, can create a false impression of the system’s capabilities and depth of understanding, because the task it represents is not a real challenge for the system.
For instance, a relatively common and well-diffused event, such as ‘a building is demolished’, might be present in multiple videos in a dataset used to train a model that is supposed to have some understanding of physics. Therefore the model could presumably generalize this concept well, and even produce genuinely novel output within the parameters learned from abundant videos.
This is an in-distribution example, where the dataset contains many useful examples for the AI system to learn from.
However, if one was to request a more bizarre or specious example, such as ‘The Eiffel Tower is blown up by alien invaders’, the model would be required to combine diverse domains such as ‘metallurgical properties’, ‘characteristics of explosions’, ‘gravity’, ‘wind resistance’ – and ‘alien spacecraft’.
This is an out-of-distribution (OOD) example, which combines so many entangled concepts that the system will likely either fail to generate a convincing example, or will default to the nearest semantic example that it was trained on – even if that example does not adhere to the user’s prompt.
Excepting that the model’s source dataset contained Hollywood-style CGI-based VFX depicting the same or a similar event, such a depiction would absolutely require that it achieve a well-generalized and ductile understanding of physical laws.
Physical Restraints
The new paper – a collaboration between Bytedance, Tsinghua University and Technion – suggests not only that models such as Sora do not really internalize deterministic physical laws in this way, but that scaling up the data (a common approach over the last 18 months) appears, in most cases, to produce no real improvement in this regard.
The paper explores not only the limits of extrapolation of specific physical laws – such as the behavior of objects in motion when they collide, or when their path is obstructed – but also a model’s capacity for combinatorial generalization – instances where the representations of two different physical principles are merged into a single generative output.
A video summary of the new paper. Source: https://x.com/bingyikang/status/1853635009611219019
The three physical laws selected for study by the researchers were parabolic motion; uniform linear motion; and perfectly elastic collision.
As can be seen in the video above, the findings indicate that models such as Sora do not really internalize physical laws, but tend to reproduce training data.
Further, the authors found that facets such as color and shape become so entangled at inference time that a generated ball would likely turn into a square, apparently because a similar motion in a dataset example featured a square and not a ball (see example in video embedded above).
The paper, which has notably engaged the research sector on social media, concludes:
‘Our study suggests that scaling alone is insufficient for video generation models to uncover fundamental physical laws, despite its role in Sora’s broader success…
‘…[Findings] indicate that scaling alone cannot address the OOD problem, although it does enhance performance in other scenarios.
‘Our in-depth analysis suggests that video model generalization relies more on referencing similar training examples rather than learning universal rules. We observed a prioritization order of color > size > velocity > shape in this “case-based” behavior.
‘[Our] study suggests that naively scaling is insufficient for video generation models to discover fundamental physical laws.’
Asked whether the research team had found a solution to the issue, one of the paper’s authors commented:
‘Unfortunately, we have not. Actually, this is probably the mission of the whole AI community.’
Method and Data
The researchers used a Variational Autoencoder (VAE) and DiT architectures to generate video samples. In this setup, the compressed latent representations produced by the VAE work in tandem with DiT’s modeling of the denoising process.
Videos were trained over the Stable Diffusion V1.5-VAE. The schema was left fundamentally unchanged, with only end-of-process architectural enhancements:
‘[We retain] the majority of the original 2D convolution, group normalization, and attention mechanisms on the spatial dimensions.
‘To inflate this structure into a spatial-temporal auto-encoder, we convert the final few 2D downsample blocks of the encoder and the initial few 2D upsample blocks of the decoder into 3D ones, and employ multiple extra 1D layers to enhance temporal modeling.’
In order to enable video modeling, the modified VAE was jointly trained with HQ image and video data, with the 2D Generative Adversarial Network (GAN) component native to the SD1.5 architecture augmented for 3D.
The image dataset used was Stable Diffusion’s original source, LAION-Aesthetics, with filtering, in addition to DataComp. For video data, a subset was curated from the Vimeo-90K, Panda-70m and HDVG datasets.
The data was trained for one million steps, with random resized crop and random horizontal flip applied as data augmentation processes.
Flipping Out
As noted above, the random horizontal flip data augmentation process can be a liability in training a system designed to produce authentic motion. This is because output from the trained model may consider both directions of an object, and cause random reversals as it attempts to negotiate this conflicting data (see embedded video above).
On the other hand, if one turns horizontal flipping off, the model is then more likely to produce output that adheres to only one direction learned from the training data.
So there is no easy solution to the issue, except that the system truly assimilates the entirety of possibilities of movement from both the native and flipped version – a facility that children develop easily, but which is more of a challenge, apparently, for AI models.
Tests
For the first set of experiments, the researchers formulated a 2D simulator to produce videos of object movement and collisions that accord with the laws of classical mechanics, which furnished a high volume and controlled dataset that excluded the ambiguities of real-world videos, for the evaluation of the models. The Box2D physics game engine was used to create these videos.
The three fundamental scenarios listed above were the focus of the tests: uniform linear motion, perfectly elastic collisions, and parabolic motion.
Datasets of increasing size (ranging from 30,000 to three million videos) were used to train models of different size and complexity (DiT-S to DiT-L), with the first three frames of each video used for conditioning.
Details of the varying models trained in the first set of experiments. Source: https://arxiv.org/pdf/2411.02385
The researchers found that the in-distribution (ID) results scaled well with increasing amounts of data, while the OOD generations did not improve, indicating shortcomings in generalization.
Results for the first round of tests.
The authors note:
‘These findings suggest the inability of scaling to perform reasoning in OOD scenarios.’
Next, the researchers tested and trained systems designed to exhibit a proficiency for combinatorial generalization, wherein two contrasting movements are combined to (hopefully) produce a cohesive movement that is faithful to the physical law behind each of the separate movements.
For this phase of the tests, the authors used the PHYRE simulator, creating a 2D environment which depicts multiple and diversely-shaped objects in free-fall, colliding with each other in a variety of complex interactions.
Evaluation metrics for this second test were Fréchet Video Distance (FVD); Structural Similarity Index (SSIM); Peak Signal-to-Noise Ratio (PSNR); Learned Perceptual Similarity Metrics (LPIPS); and a human study (denoted as ‘abnormal’ in results).
Three scales of training datasets were created, at 100,000 videos, 0.6 million videos, and 3-6 million videos. DiT-B and DiT-XL models were used, due to the increased complexity of the videos, with the first frame used for conditioning.
The models were trained for one million steps at 256×256 resolution, with 32 frames per video.
Results for the second round of tests.
The outcome of this test suggests that merely increasing data volume is an inadequate approach:
The paper states:
‘These results suggest that both model capacity and coverage of the combination space are crucial for combinatorial generalization. This insight implies that scaling laws for video generation should focus on increasing combination diversity, rather than merely scaling up data volume.’
Finally, the researchers conducted further tests to attempt to determine whether a video generation models can truly assimilate physical laws, or whether it simply memorizes and reproduces training data at inference time.
Here they examined the concept of ‘case-based’ generalization, where models tend to mimic specific training examples when confronting novel situations, as well as examining examples of uniform motion – specifically, how the direction of motion in training data influences the trained model’s predictions.
Two sets of training data, for uniform motion and collision, were curated, each consisting of uniform motion videos depicting velocities between 2.5 to 4 units, with the first three frames used as conditioning. Latent values such as velocity were omitted, and, after training, testing was performed on both seen and unseen scenarios.
Below we see results for the test for uniform motion generation:
Results for tests for uniform motion generation, where the ‘velocity’ variable is omitted during training.
The authors state:
‘[With] a large gap in the training set, the model tends to generate videos where the velocity is either high or low to resemble training data when initial frames show middle-range velocities.’
For the collision tests, far more variables are involved, and the model is required to learn a two-dimensional non-linear function.
Collision: results for the third and final round of tests.
The authors observe that the presence of ‘deceptive’ examples, such as reversed motion (i.e., a ball that bounces off a surface and reverses its course), can mislead the model and cause it to generate physically incorrect predictions.
Conclusion
If a non-AI algorithm (i.e., a ‘baked’, procedural method) contains mathematical rules for the behavior of physical phenomena such as fluids, or objects under gravity, or under pressure, there are a set of unchanging constants available for accurate rendering.
However, the new paper’s findings indicate that no such equivalent relationship or intrinsic understanding of classical physical laws is developed during the training of generative models, and that increasing amounts of data do not resolve the problem, but rather obscure it –because a greater number of training videos are available for the system to imitate at inference time.
* My conversion of the authors’ inline citations to hyperlinks.
First published Tuesday, November 26, 2024
#000#2023#2024#3d#ai#ai model#AI models#AI systems#AI video creation#algorithm#alien#Analysis#approach#architecture#Art#Artificial Intelligence#attention#background#Behavior#Building#Bytedance#challenge#Children#classical#Collaboration#collisions#Color#Combinatorial#Community#complexity
0 notes