#Future of Data Engineering
Explore tagged Tumblr posts
Text
Future of Data Engineering: Know the Great Influence & Its Outline
For more latest news updates, visit https://businesshonor.com/usa
Data engineering has changed since its beginning, when its storage and recovery were basic and lengthy, to the present, where it uses new technologies in huge volume processing and analytics. At first, its management, which focused on organizing and storing, was identical to data engineering. The field grew to include warehousing concepts and actual processing as a result of the notable increase in its volume, diversity, and velocity. These days, this kind of engineering helps businesses use big data to obtain valuable insights that guide their goals and activities. AI and automation for its pipeline optimization will continue to advance. Its skills will begin to be impacted by quantum computing. In the future of data engineering, the growth of IoT and edge computing will necessitate dispersed, real-time data processing. Global management techniques are expected to be impacted by strict privacy and power restrictions.
#future of data engineering#businesshonor#latest magazine#latest news today#data management#datascience#data analytics#digitaltransformation#technologynews
0 notes
Text
The Importance of Data Engineering in Today’s Data-Driven World

In today’s fast-paced, technology-driven world, data has emerged as a critical asset for businesses across all sectors. It serves as the foundation for strategic decisions, drives innovation, and shapes competitive advantage. However, extracting meaningful insights from data requires more than just access to information; it necessitates well-designed systems and processes for efficient data management and analysis. This is where data engineering steps in. A vital aspect of data science and analytics, data engineering is responsible for building, optimizing, and maintaining the systems that collect, store, and process data, ensuring it is accessible and actionable for organizations.
Let's explore how Data Engineering is important in today's world:
1. What is Data Engineering
2. Why is Data Engineering Important
3. Key Components of Data Engineering
4. Trends in Data Engineering
5. The Future of Data Engineering
Let’s examine each one in detail below.
What is Data Engineering?
Data engineering involves creating systems that help collect, store, and process data effectively.It involves creating data pipelines that transport data from its source to storage and analysis systems, implementing ETL processes (Extract, Transform, Load), and maintaining data management systems to ensure data is accessible and secure. It enables organizations to make better use of their data resources for data-driven decision-making.
Why is Data Engineering Important?
Supports Data-Driven Decision-Making: In a competitive world, decisions need to be based on facts and insights. Data engineering ensures that clean, reliable, and up-to-date data is available to decision-makers. From forecasting market trends to optimizing operations, data engineering helps businesses stay ahead.
Manages Big Data Effectively: Big data engineering focuses on handling large and complex datasets, making it possible to process and analyze them efficiently. Industries like finance, healthcare, and e-commerce rely heavily on big data solutions to deliver better results.
Enables Modern Technologies: Technologies like machine learning, artificial intelligence, and predictive analytics depend on well-prepared data. Without a solid modern data infrastructure, these advanced technologies cannot function effectively. Data engineering ensures these systems have the data they need to perform accurately.
Key Components of Data Engineering:
Data Pipelines: Data pipelines move data automatically between systems.They take data from one source, change it into a useful format, and then store it or prepare it for analysis.
ETL Processes: ETL (Extract, Transform, Load) processes are crucial in preparing raw data for analysis. They clean, organize, and format data, ensuring it is ready for use.
Data Management Systems:
These systems keep data organized and make it easy to access. Examples of these systems are databases, data warehouses, and data lakes.
Data Engineering Tools: From tools like Apache Kafka for real-time data streaming to cloud platforms like AWS and Azure, data engineering tools are essential for managing large-scale data workflows.
Trends in Data Engineering:
The field of data engineering is changing quickly, and many trends are shaping its future:
Cloud-Based Infrastructure: More businesses are moving to the cloud for scalable and flexible data storage.
Real-Time Data Processing: The need for instant insights is driving the adoption of real-time data systems.
Automation in ETL: Automating repetitive ETL tasks is becoming a standard practice to improve efficiency.
Focus on Data Security: With increasing concerns about data privacy, data engineering emphasizes building secure systems.
Sustainability: Energy-efficient systems are gaining popularity as companies look for greener solutions.
The Future of Data Engineering:
The future of data engineering looks bright. As data grows in size and complexity, more skilled data engineers will be needed.Innovations in artificial intelligence and machine learning will further integrate with data engineering, making it a critical part of technological progress. Additionally, advancements in data engineering tools and methods will continue to simplify and enhance workflows.
Conclusion:
Data engineering is the backbone of contemporary data management and analytics. It provides the essential infrastructure and frameworks that allow organizations to efficiently process and manage large volumes of data. By focusing on data quality, scalability, and system performance, data engineers ensure that businesses can unlock the full potential of their data, empowering them to make informed decisions and drive innovation in an increasingly data-driven world.
Tudip Technologies has been a pioneering force in the tech industry for over a decade, specializing in AI-driven solutions. Our innovative solutions leverage GenAI capabilities to enhance real-time decision-making, identify opportunities, and minimize costs through seamless processes and maintenance.
If you're interested in learning more about the Data Engineering related courses offered by Tudip Learning please visit: https://tudiplearning.com/course/essentials-of-data-engineering/.
#Data engineering trends#Importance of data engineering#Data-driven decision-making#Big data engineering#Modern data infrastructure#Data pipelines#ETL processes#Data engineering tools#Future of data engineering#Data Engineering
1 note
·
View note
Text
Data Engineering Services Explained: What Lies Ahead for the Industry
In an era where data shapes every aspect of business decision-making, organizations are turning to data engineering to harness its full potential. As data volumes and complexities escalate, the demand for specialized data engineering services has surged. This article delves into the core components of data engineering services and offers insights into the evolving future of this critical field.
What are Data Engineering Services?
Data engineering involves the design, construction, and maintenance of systems and infrastructure that allow for the collection, storage, processing, and analysis of data. Data engineering services encompass a variety of tasks and functions that ensure data is accessible, reliable, and usable for data scientists, analysts, and business stakeholders. Key components of data engineering services include:
1. Data Architecture
Data engineers are responsible for designing data architectures that define how data is collected, stored, and accessed. This includes selecting appropriate databases, data lakes, and data warehouses to optimize performance and scalability.
2. Data Integration
Data often comes from multiple sources, including transactional systems, external APIs, and sensor data. Data engineering services involve creating ETL (Extract, Transform, Load) processes that integrate data from these various sources into a unified format.
3. Data Quality and Governance
Ensuring data quality is critical for accurate analysis. Data engineers implement data validation and cleansing processes to identify and rectify errors. They also establish governance frameworks to maintain data integrity and compliance with regulations.
4. Data Pipeline Development
Data pipelines automate the flow of data from its source to storage and processing systems. Data engineering services focus on building efficient pipelines that can handle large volumes of data while ensuring minimal latency.
5. Performance Optimization
As organizations scale, performance becomes a crucial consideration. Data engineers optimize databases and pipelines for speed and efficiency, enabling faster data retrieval and processing.
6. Collaboration with Data Teams
Data engineers work closely with data scientists, analysts, and other stakeholders to understand their data needs. This collaboration ensures that the data infrastructure supports analytical initiatives effectively.
The Future of Data Engineering
As the field of data engineering evolves, several trends are shaping its future:
1. Increased Automation
Automation is set to revolutionize data engineering. Tools and platforms are emerging that automate repetitive tasks such as data cleansing, pipeline management, and monitoring. This will allow data engineers to focus on more strategic initiatives rather than manual processes.
2. Real-time Data Processing
With the rise of IoT devices and streaming applications, the demand for real-time data processing is growing. Future data engineering services will increasingly incorporate technologies like Apache Kafka and Apache Flink to facilitate real-time data ingestion and analytics.
3. Cloud-based Solutions
Cloud computing is becoming the norm for data storage and processing. Data engineering services will continue to leverage cloud platforms like AWS, Google Cloud, and Azure, offering greater scalability, flexibility, and cost-effectiveness.
4. DataOps
DataOps is an emerging discipline that applies agile methodologies to data management. It emphasizes collaboration, continuous integration, and automation in data pipelines. As organizations adopt DataOps, the role of data engineers will shift toward ensuring seamless collaboration across data teams.
5. Focus on Data Security and Privacy
With growing concerns about data security and privacy, data engineers will play a vital role in implementing security measures and ensuring compliance with regulations like GDPR and CCPA. Future services will prioritize data protection as a foundational element of data architecture.
6. Integration of AI and Machine Learning
Data engineering will increasingly intersect with artificial intelligence and machine learning. Data engineers will need to build infrastructures that support machine learning models, ensuring they have access to clean, structured data for training and inference.
Conclusion
Data engineering services are essential for organizations seeking to harness the power of data. As technology continues to advance, the future of data engineering promises to be dynamic and transformative. With a focus on automation, real-time processing, cloud solutions, and security, data engineers will be at the forefront of driving data strategy and innovation. Embracing these trends will enable organizations to make informed decisions, optimize operations, and ultimately gain a competitive edge in their respective industries.
#Data Engineering Services#Data Security#Data Privacy#Future of Data Engineering#Data Architecture#Data Governance
0 notes
Text
LOOK FOR PORNOGRAPHY FOR MASTRUBATION
LOOK FOR PORNOGRAPHY TO MASTRUBATE TO
#search#searches#existing search requests#future search requests#search requests#LOOK FOR PORNOGRAPHY FOR MASTRUBATION#LOOK FOR PORNOGRAPHY TO MASTRUBATE TO#ibm#international business machines#IOTA BETA MU#ibm pc#ibm 7094#ibmx#IBM DEEPBLUE#IBM DEEP BLUE#taylor swift#pi day#martin luther king jr#melanie martinez#michelle obama#caprica#tim kaine#search engines#microprocessors#solid state battery#solid state drive#hard drive#hard disk drive#hard disk data recovery#💾
25 notes
·
View notes
Text
Cyborg receives space data
Pixel art for today based on videogame Metal Stoker for game console PC Engine. It is something like science fiction cybernetical action. It is even has a intro with a cyborg. Such. Count it as a second Quake. According to game screens, it is flying-shooting videogame about a future.
And this is drawing about the same theme. About theme with cyborg intro. So, I draw my own cyborg. Cyborg makes a connection with his main computer using wires. And he was impressed with data, that he received. Into his mind, it was a flow of data, a lot of running process. From a different electronic calculations machine, all the data goes by network protocol into main computer. And with wires cyborg just establish connection with his main computer. To collect data. And analyze them.
Amazing data! – says cyborg. Cyborg speaks – Fascinating!
What, it is interesting data. So interesting how planets moves. Which, it is interesting asteroid. It has interesting components. And what a data goes from a second planet near the sun. Red dwarf. Wow! It is very interesting!
Dima Link is making retro videogames, apps, a little of music, write stories, and some retro more.
WEBSITE: http://www.dimalink.tv-games.ru/home_eng.html ITCHIO: https://dimalink.itch.io/ GAMEJOLT: https://gamejolt.com/@DimaLink/games
BLOGGER: https://dimalinkeng.blogspot.com/
#8 bit#16 bit#pc engine#megadrive#snes#retro game#pixel art#pixels#pixel aesthetic#80s#cyborg#sci fi#quake 2#lots of data#cyber#future#space#digital art#planets#asteroids#red dwarf#wires#computers#machines#network protocl#programmer#ms dos
9 notes
·
View notes
Text
Protecting Your AI Investment: Why Cooling Strategy Matters More Than Ever
New Post has been published on https://thedigitalinsider.com/protecting-your-ai-investment-why-cooling-strategy-matters-more-than-ever/
Protecting Your AI Investment: Why Cooling Strategy Matters More Than Ever
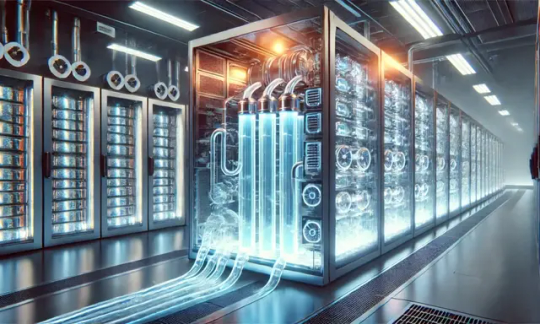
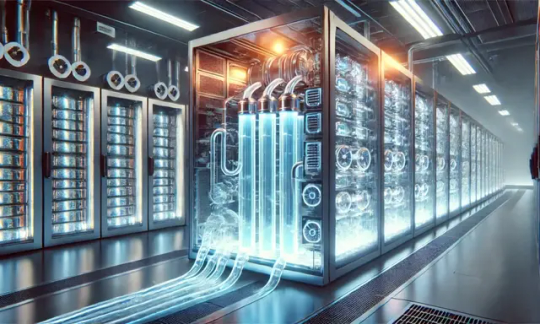
Data center operators are gambling millions on outdated cooling technology. The conversation around data center cooling isn’t just changing—it’s being completely redefined by the economics of AI. The stakes have never been higher.
The rapid advancement of AI has transformed data center economics in ways few predicted. When a single rack of AI servers costs around $3 million—as much as a luxury home—the risk calculation fundamentally changes. As Andreessen Horowitz co-founder Ben Horowitz recently cautioned, data centers financing these massive hardware investments “could get upside down very fast” if they don’t carefully manage their infrastructure strategy.
This new reality demands a fundamental rethinking of cooling approaches. While traditional metrics like PUE and operating costs are still important, they are secondary to protecting these multi-million-dollar hardware investments. The real question data center operators should be asking is: How do we best protect our AI infrastructure investment?
The Hidden Risks of Traditional Cooling
The industry’s historic reliance on single-phase, water-based cooling solutions carries increasingly unacceptable risks in the AI era. While it has served data centers well for years, the thermal demands of AI workloads have pushed this technology beyond its practical limits. The reason is simple physics: single-phase systems require higher flow rates to manage today’s thermal loads, increasing the risk of leaks and catastrophic failures.
This isn’t a hypothetical risk. A single water leak can instantly destroy millions in AI hardware—hardware that often has months-long replacement lead times in today’s supply-constrained market. The cost of even a single catastrophic failure can exceed a data center’s cooling infrastructure budget for an entire year. Yet many operators continue to rely on these systems, effectively gambling their AI investment on aging technology.
At Data Center World 2024, Dr. Mohammad Tradat, NVIDIA’s Manager of Data Center Mechanical Engineering, asked, “How long will single-phase cooling live? It’ll be phased out very soon…and then the need will be for two-phase, refrigerant-based cooling.” This isn’t just a growing opinion—it’s becoming an industry consensus backed by physics and financial reality.
A New Approach to Investment Protection
Two-phase cooling technology, which uses dielectric refrigerants instead of water, fundamentally changes this risk equation. The cost of implementing a two-phase cooling system—typically around $200,000 per rack—should be viewed as insurance for protecting a $5 million AI hardware investment. To put this in perspective, that’s a 4% premium to protect your asset—considerably lower than insurance rates for other multi-million dollar business investments. The business case becomes even clearer when you factor in the potential costs of AI training disruption and idle infrastructure during unplanned downtime.
For data center operators and financial stakeholders, the decision to invest in two-phase cooling should be evaluated through the lens of risk management and investment protection. The relevant metrics should include not just operating costs or energy efficiency but also the total value of hardware being protected, the cost of potential failure scenarios, the future-proofing value for next-generation hardware and the risk-adjusted return on cooling investment.
As AI continues to drive up the density and value of data center infrastructure, the industry must evolve its approach to cooling strategy. The question isn’t whether to move to two-phase cooling but when and how to transition while minimizing risk to existing operations and investments.
Smart operators are already making this shift, while others risk learning an expensive lesson. In an era where a single rack costs more than many data centers’ annual operating budgets, gambling on outdated cooling technology isn’t just risky – it’s potentially catastrophic. The time to act is now—before that risk becomes a reality.
#000#2024#Accelsius#aging#ai#AI Infrastructure#ai training#approach#budgets#Business#cooling#data#Data Center#Data Centers#disruption#Economics#efficiency#energy#energy efficiency#engineering#factor#financial#Fundamental#Future#gambling#Hardware#how#how to#Industry#Infrastructure
2 notes
·
View notes
Text
Your Guide to B.Tech in Computer Science & Engineering Colleges
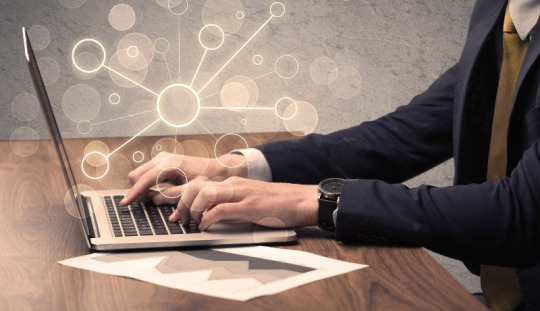
In today's technology-driven world, pursuing a B.Tech in Computer Science and Engineering (CSE) has become a popular choice among students aspiring for a bright future. The demand for skilled professionals in areas like Artificial Intelligence, Machine Learning, Data Science, and Cloud Computing has made computer science engineering colleges crucial in shaping tomorrow's innovators. Saraswati College of Engineering (SCOE), a leader in engineering education, provides students with a perfect platform to build a successful career in this evolving field.
Whether you're passionate about coding, software development, or the latest advancements in AI, pursuing a B.Tech in Computer Science and Engineering at SCOE can open doors to endless opportunities.
Why Choose B.Tech in Computer Science and Engineering?
Choosing a B.Tech in Computer Science and Engineering isn't just about learning to code; it's about mastering problem-solving, logical thinking, and the ability to work with cutting-edge technologies. The course offers a robust foundation that combines theoretical knowledge with practical skills, enabling students to excel in the tech industry.
At SCOE, the computer science engineering courses are designed to meet industry standards and keep up with the rapidly evolving tech landscape. With its AICTE Approved, NAAC Accredited With Grade-"A+" credentials, the college provides quality education in a nurturing environment. SCOE's curriculum goes beyond textbooks, focusing on hands-on learning through projects, labs, workshops, and internships. This approach ensures that students graduate not only with a degree but with the skills needed to thrive in their careers.
The Role of Computer Science Engineering Colleges in Career Development
The role of computer science engineering colleges like SCOE is not limited to classroom teaching. These institutions play a crucial role in shaping students' futures by providing the necessary infrastructure, faculty expertise, and placement opportunities. SCOE, established in 2004, is recognized as one of the top engineering colleges in Navi Mumbai. It boasts a strong placement record, with companies like Goldman Sachs, Cisco, and Microsoft offering lucrative job opportunities to its graduates.
The computer science engineering courses at SCOE are structured to provide a blend of technical and soft skills. From the basics of computer programming to advanced topics like Artificial Intelligence and Data Science, students at SCOE are trained to be industry-ready. The faculty at SCOE comprises experienced professionals who not only impart theoretical knowledge but also mentor students for real-world challenges.
Highlights of the B.Tech in Computer Science and Engineering Program at SCOE
Comprehensive Curriculum: The B.Tech in Computer Science and Engineering program at SCOE covers all major areas, including programming languages, algorithms, data structures, computer networks, operating systems, AI, and Machine Learning. This ensures that students receive a well-rounded education, preparing them for various roles in the tech industry.
Industry-Relevant Learning: SCOE’s focus is on creating professionals who can immediately contribute to the tech industry. The college regularly collaborates with industry leaders to update its curriculum, ensuring students learn the latest technologies and trends in computer science engineering.
State-of-the-Art Infrastructure: SCOE is equipped with modern laboratories, computer centers, and research facilities, providing students with the tools they need to gain practical experience. The institution’s infrastructure fosters innovation, helping students work on cutting-edge projects and ideas during their B.Tech in Computer Science and Engineering.
Practical Exposure: One of the key benefits of studying at SCOE is the emphasis on practical learning. Students participate in hands-on projects, internships, and industry visits, giving them real-world exposure to how technology is applied in various sectors.
Placement Support: SCOE has a dedicated placement cell that works tirelessly to ensure students secure internships and job offers from top companies. The B.Tech in Computer Science and Engineering program boasts a strong placement record, with top tech companies visiting the campus every year. The highest on-campus placement offer for the academic year 2022-23 was an impressive 22 LPA from Goldman Sachs, reflecting the college’s commitment to student success.
Personal Growth: Beyond academics, SCOE encourages students to participate in extracurricular activities, coding competitions, and tech fests. These activities enhance their learning experience, promote teamwork, and help students build a well-rounded personality that is essential in today’s competitive job market.
What Makes SCOE Stand Out?
With so many computer science engineering colleges to choose from, why should you consider SCOE for your B.Tech in Computer Science and Engineering? Here are a few factors that make SCOE a top choice for students:
Experienced Faculty: SCOE prides itself on having a team of highly qualified and experienced faculty members. The faculty’s approach to teaching is both theoretical and practical, ensuring students are equipped to tackle real-world challenges.
Strong Industry Connections: The college maintains strong relationships with leading tech companies, ensuring that students have access to internship opportunities and campus recruitment drives. This gives SCOE graduates a competitive edge in the job market.
Holistic Development: SCOE believes in the holistic development of students. In addition to academic learning, the college offers opportunities for personal growth through various student clubs, sports activities, and cultural events.
Supportive Learning Environment: SCOE provides a nurturing environment where students can focus on their academic and personal growth. The campus is equipped with modern facilities, including spacious classrooms, labs, a library, and a recreation center.
Career Opportunities After B.Tech in Computer Science and Engineering from SCOE
Graduates with a B.Tech in Computer Science and Engineering from SCOE are well-prepared to take on various roles in the tech industry. Some of the most common career paths for CSE graduates include:
Software Engineer: Developing software applications, web development, and mobile app development are some of the key responsibilities of software engineers. This role requires strong programming skills and a deep understanding of software design.
Data Scientist: With the rise of big data, data scientists are in high demand. CSE graduates with knowledge of data science can work on data analysis, machine learning models, and predictive analytics.
AI Engineer: Artificial Intelligence is revolutionizing various industries, and AI engineers are at the forefront of this change. SCOE’s curriculum includes AI and Machine Learning, preparing students for roles in this cutting-edge field.
System Administrator: Maintaining and managing computer systems and networks is a crucial role in any organization. CSE graduates can work as system administrators, ensuring the smooth functioning of IT infrastructure.
Cybersecurity Specialist: With the growing threat of cyberattacks, cybersecurity specialists are essential in protecting an organization’s digital assets. CSE graduates can pursue careers in cybersecurity, safeguarding sensitive information from hackers.
Conclusion: Why B.Tech in Computer Science and Engineering at SCOE is the Right Choice
Choosing the right college is crucial for a successful career in B.Tech in Computer Science and Engineering. Saraswati College of Engineering (SCOE) stands out as one of the best computer science engineering colleges in Navi Mumbai. With its industry-aligned curriculum, state-of-the-art infrastructure, and excellent placement record, SCOE offers students the perfect environment to build a successful career in computer science.
Whether you're interested in AI, data science, software development, or any other field in computer science, SCOE provides the knowledge, skills, and opportunities you need to succeed. With a strong focus on hands-on learning and personal growth, SCOE ensures that students graduate not only as engineers but as professionals ready to take on the challenges of the tech world.
If you're ready to embark on an exciting journey in the world of technology, consider pursuing your B.Tech in Computer Science and Engineering at SCOE—a college where your future takes shape.
#In today's technology-driven world#pursuing a B.Tech in Computer Science and Engineering (CSE) has become a popular choice among students aspiring for a bright future. The de#Machine Learning#Data Science#and Cloud Computing has made computer science engineering colleges crucial in shaping tomorrow's innovators. Saraswati College of Engineeri#a leader in engineering education#provides students with a perfect platform to build a successful career in this evolving field.#Whether you're passionate about coding#software development#or the latest advancements in AI#pursuing a B.Tech in Computer Science and Engineering at SCOE can open doors to endless opportunities.#Why Choose B.Tech in Computer Science and Engineering?#Choosing a B.Tech in Computer Science and Engineering isn't just about learning to code; it's about mastering problem-solving#logical thinking#and the ability to work with cutting-edge technologies. The course offers a robust foundation that combines theoretical knowledge with prac#enabling students to excel in the tech industry.#At SCOE#the computer science engineering courses are designed to meet industry standards and keep up with the rapidly evolving tech landscape. With#NAAC Accredited With Grade-“A+” credentials#the college provides quality education in a nurturing environment. SCOE's curriculum goes beyond textbooks#focusing on hands-on learning through projects#labs#workshops#and internships. This approach ensures that students graduate not only with a degree but with the skills needed to thrive in their careers.#The Role of Computer Science Engineering Colleges in Career Development#The role of computer science engineering colleges like SCOE is not limited to classroom teaching. These institutions play a crucial role in#faculty expertise#and placement opportunities. SCOE#established in 2004#is recognized as one of the top engineering colleges in Navi Mumbai. It boasts a strong placement record
2 notes
·
View notes
Text
y'all the data scientist job market is so bleak. like 75% of companies are just looking for people to write chat bots 😭
#i'm gonna learn how so i can get a fucking job but idk. i might need to consider a career change in the near future lmao#i'm considering a pivot to software engineering. i'll build a fucking website or something. idk#that was my first Serious Career Plan™ at age 16 so i'm gonna laugh so hard if i end up being a software engineer after all#i've applied to a few jobs that are kinda in between data science and software engineering so we'll see about that lol#m.txt
2 notes
·
View notes
Text
The Impact of AI on Everyday Life: A New Normal
The impact of AI on everyday life has become a focal point for discussions among tech enthusiasts, policymakers, and the general public alike. This transformative force is reshaping the way we live, work, and interact with the world around us, making its influence felt across various domains of our daily existence. Revolutionizing Workplaces One of the most significant arenas where the impact…
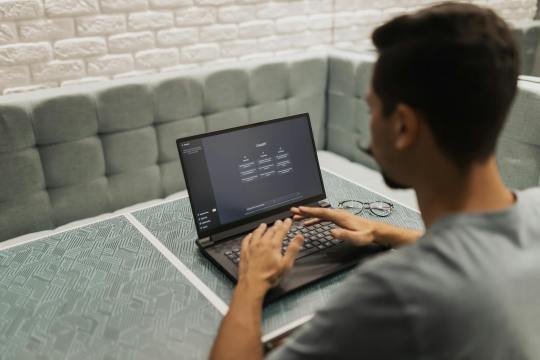
View On WordPress
#adaptive learning#AI accessibility#AI adaptation#AI advancements#AI algorithms#AI applications#AI automation#AI benefits#AI capability#AI challenges#AI collaboration#AI convenience#AI data analysis#AI debate#AI decision-making#AI design#AI diagnostics#AI discussion#AI education#AI efficiency#AI engineering#AI enhancement#AI environment#AI ethics#AI experience#AI future#AI governance#AI healthcare#AI impact#AI implications
1 note
·
View note
Text

Unlock Your Future: Why AI Skills Are Hot in the Job Market! Boost your career! Discover how adding AI skills like machine learning and data science expertise significantly improves your chances in today's competitive job market.
Read more
#AI skills#job market#artificial intelligence#machine learning#tech careers#AI jobs#data science#AI education#prompt engineering#future of work
0 notes
Text
The Future of Digital Marketing: Trends to Watch in 2025
Introduction The digital marketing landscape is evolving faster than ever. With advancements in artificial intelligence, changing consumer behaviors, and new regulations shaping the industry, businesses must stay ahead of the curve. To remain competitive, marketers need to adapt to the latest trends that will define digital marketing in 2025. In this article, we will explore the key digital…
#AI in digital marketing#AI-powered content creation tools#Best marketing automation tools 2025#Best video marketing platforms#Brand awareness through influencer marketing#content-marketing#Data privacy in digital advertising#Digital Marketing#Digital marketing trends 2025#Future of digital marketing#Future of paid advertising#How AI is changing digital marketing#How to optimize for Google search in 2025#Influencer marketing strategies#Interactive ads for higher conversions#Interactive content marketing#marketing#Marketing automation and AI#Micro-influencers vs macro-influencers#Omnichannel digital marketing strategy#Privacy-first marketing#Search engine optimization strategies#SEO#SEO trends 2025#Short-form video marketing#Social media engagement tactics#Social media marketing trends#social-media#The impact of AI on consumer behavior#Video marketing trends
0 notes
Text
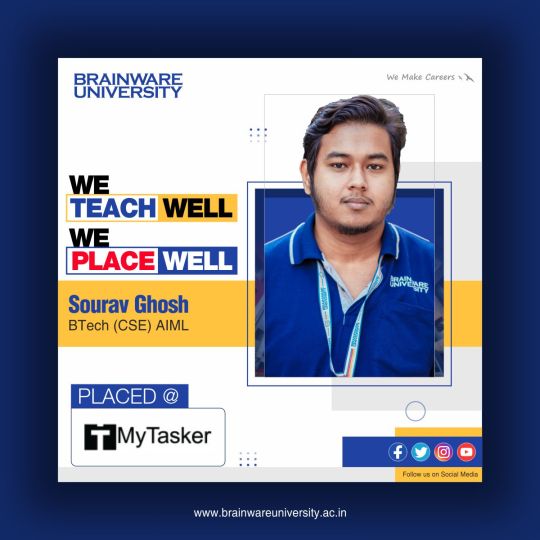
BTech CSE: Your Gateway to High-Demand Tech Careers
Apply now for admission and avail the Early Bird Offer
In the digital age, a BTech in Computer Science & Engineering (CSE) is one of the most sought-after degrees, offering unmatched career opportunities across industries. From software development to artificial intelligence, the possibilities are endless for CSE graduates.
Top Job Opportunities for BTech CSE Graduates
Software Developer: Design and develop innovative applications and systems.
Data Scientist: Analyze big data to drive business decisions.
Cybersecurity Analyst: Safeguard organizations from digital threats.
AI/ML Engineer: Lead the way in artificial intelligence and machine learning.
Cloud Architect: Build and maintain cloud-based infrastructure for global organizations.
Why Choose Brainware University for BTech CSE?
Brainware University provides a cutting-edge curriculum, hands-on training, and access to industry-leading tools. Our dedicated placement cell ensures you’re job-ready, connecting you with top recruiters in tech.
👉 Early Bird Offer: Don’t wait! Enroll now and take the first step toward a high-paying, future-ready career in CSE.
Your journey to becoming a tech leader starts here!
#n the digital age#a BTech in Computer Science & Engineering (CSE) is one of the most sought-after degrees#offering unmatched career opportunities across industries. From software development to artificial intelligence#the possibilities are endless for CSE graduates.#Top Job Opportunities for BTech CSE Graduates#Software Developer: Design and develop innovative applications and systems.#Data Scientist: Analyze big data to drive business decisions.#Cybersecurity Analyst: Safeguard organizations from digital threats.#AI/ML Engineer: Lead the way in artificial intelligence and machine learning.#Cloud Architect: Build and maintain cloud-based infrastructure for global organizations.#Why Choose Brainware University for BTech CSE?#Brainware University provides a cutting-edge curriculum#hands-on training#and access to industry-leading tools. Our dedicated placement cell ensures you’re job-ready#connecting you with top recruiters in tech.#👉 Early Bird Offer: Don’t wait! Enroll now and take the first step toward a high-paying#future-ready career in CSE.#Your journey to becoming a tech leader starts here!#BTechCSE#BrainwareUniversity#TechCareers#SoftwareEngineering#AIJobs#EarlyBirdOffer#DataScience#FutureOfTech#Placements
1 note
·
View note
Text
Data Science and Engineering Driving industry Innovations
The integration of data science and engineering is revolutionizing industries, enabling smarter decision-making, process optimization, and predictive capabilities. At M.Kumaraswamy College of Engineering (MKCE), students are equipped to harness data science to solve complex challenges and drive innovation. By combining theoretical knowledge with practical applications, MKCE prepares students to optimize processes in manufacturing, healthcare, transportation, energy, and urban planning. The curriculum includes courses on machine learning, big data analytics, and programming, alongside hands-on projects and internships. MKCE’s focus on industry collaborations ensures students stay ahead of emerging trends like AI, IoT, and digital twins. This interdisciplinary approach empowers students to lead in data-driven industries and shape the future of engineering.
To Know More : https://mkce.ac.in/blog/data-science-and-engineering-driving-innovation-across-industries/
#best engineering college#mkce college#private college#engineering college#top 10 colleges in tn#libary#best engineering college in karur#engineering college in karur#mkce.ac.in#mkce#Data Science#Engineering Innovation#Predictive Maintenance#Process Optimization#Machine Learning#Big Data Analytics#Smart Manufacturing#Healthcare Engineering#Smart Cities#mkce placement#cse future#ece full form in engineering#ece job roles#mkce fees payment#cse vs mechanical engineering
0 notes
Text
Will AI and Machine Learning Take Over Civil Engineering Degree?
If you’ve been following the latest trends in civil engineering degree, you might have noticed that Artificial Intelligence (AI) and Machine Learning (ML) are making quite a splash. But what does this mean for traditional civil engineering degrees? Will AI and ML render these programs obsolete, or will they enhance the educational landscape? The Changing Face of Civil Engineering Degree Civil…

View On WordPress
#AI applications in engineering#AI education#AI in civil engineering#automation in construction#civil engineering careers#civil engineering degrees#data analysis in civil engineering#degree#engineering job market#engineering technology#future of civil engineering#Generative Design#infrastructure development#Machine Learning in engineering#Predictive Maintenance#skills for engineers
0 notes
Text
If anyone wants to know why every tech company in the world right now is clamoring for AI like drowned rats scrabbling to board a ship, I decided to make a post to explain what's happening.
(Disclaimer to start: I'm a software engineer who's been employed full time since 2018. I am not a historian nor an overconfident Youtube essayist, so this post is my working knowledge of what I see around me and the logical bridges between pieces.)
Okay anyway. The explanation starts further back than what's going on now. I'm gonna start with the year 2000. The Dot Com Bubble just spectacularly burst. The model of "we get the users first, we learn how to profit off them later" went out in a no-money-having bang (remember this, it will be relevant later). A lot of money was lost. A lot of people ended up out of a job. A lot of startup companies went under. Investors left with a sour taste in their mouth and, in general, investment in the internet stayed pretty cooled for that decade. This was, in my opinion, very good for the internet as it was an era not suffocating under the grip of mega-corporation oligarchs and was, instead, filled with Club Penguin and I Can Haz Cheezburger websites.
Then around the 2010-2012 years, a few things happened. Interest rates got low, and then lower. Facebook got huge. The iPhone took off. And suddenly there was a huge new potential market of internet users and phone-havers, and the cheap money was available to start backing new tech startup companies trying to hop on this opportunity. Companies like Uber, Netflix, and Amazon either started in this time, or hit their ramp-up in these years by shifting focus to the internet and apps.
Now, every start-up tech company dreaming of being the next big thing has one thing in common: they need to start off by getting themselves massively in debt. Because before you can turn a profit you need to first spend money on employees and spend money on equipment and spend money on data centers and spend money on advertising and spend money on scale and and and
But also, everyone wants to be on the ship for The Next Big Thing that takes off to the moon.
So there is a mutual interest between new tech companies, and venture capitalists who are willing to invest $$$ into said new tech companies. Because if the venture capitalists can identify a prize pig and get in early, that money could come back to them 100-fold or 1,000-fold. In fact it hardly matters if they invest in 10 or 20 total bust projects along the way to find that unicorn.
But also, becoming profitable takes time. And that might mean being in debt for a long long time before that rocket ship takes off to make everyone onboard a gazzilionaire.
But luckily, for tech startup bros and venture capitalists, being in debt in the 2010's was cheap, and it only got cheaper between 2010 and 2020. If people could secure loans for ~3% or 4% annual interest, well then a $100,000 loan only really costs $3,000 of interest a year to keep afloat. And if inflation is higher than that or at least similar, you're still beating the system.
So from 2010 through early 2022, times were good for tech companies. Startups could take off with massive growth, showing massive potential for something, and venture capitalists would throw infinite money at them in the hopes of pegging just one winner who will take off. And supporting the struggling investments or the long-haulers remained pretty cheap to keep funding.
You hear constantly about "Such and such app has 10-bazillion users gained over the last 10 years and has never once been profitable", yet the thing keeps chugging along because the investors backing it aren't stressed about the immediate future, and are still banking on that "eventually" when it learns how to really monetize its users and turn that profit.
The pandemic in 2020 took a magnifying-glass-in-the-sun effect to this, as EVERYTHING was forcibly turned online which pumped a ton of money and workers into tech investment. Simultaneously, money got really REALLY cheap, bottoming out with historic lows for interest rates.
Then the tide changed with the massive inflation that struck late 2021. Because this all-gas no-brakes state of things was also contributing to off-the-rails inflation (along with your standard-fare greedflation and price gouging, given the extremely convenient excuses of pandemic hardships and supply chain issues). The federal reserve whipped out interest rate hikes to try to curb this huge inflation, which is like a fire extinguisher dousing and suffocating your really-cool, actively-on-fire party where everyone else is burning but you're in the pool. And then they did this more, and then more. And the financial climate followed suit. And suddenly money was not cheap anymore, and new loans became expensive, because loans that used to compound at 2% a year are now compounding at 7 or 8% which, in the language of compounding, is a HUGE difference. A $100,000 loan at a 2% interest rate, if not repaid a single cent in 10 years, accrues to $121,899. A $100,000 loan at an 8% interest rate, if not repaid a single cent in 10 years, more than doubles to $215,892.
Now it is scary and risky to throw money at "could eventually be profitable" tech companies. Now investors are watching companies burn through their current funding and, when the companies come back asking for more, investors are tightening their coin purses instead. The bill is coming due. The free money is drying up and companies are under compounding pressure to produce a profit for their waiting investors who are now done waiting.
You get enshittification. You get quality going down and price going up. You get "now that you're a captive audience here, we're forcing ads or we're forcing subscriptions on you." Don't get me wrong, the plan was ALWAYS to monetize the users. It's just that it's come earlier than expected, with way more feet-to-the-fire than these companies were expecting. ESPECIALLY with Wall Street as the other factor in funding (public) companies, where Wall Street exhibits roughly the same temperament as a baby screaming crying upset that it's soiled its own diaper (maybe that's too mean a comparison to babies), and now companies are being put through the wringer for anything LESS than infinite growth that Wall Street demands of them.
Internal to the tech industry, you get MASSIVE wide-spread layoffs. You get an industry that used to be easy to land multiple job offers shriveling up and leaving recent graduates in a desperately awful situation where no company is hiring and the market is flooded with laid-off workers trying to get back on their feet.
Because those coin-purse-clutching investors DO love virtue-signaling efforts from companies that say "See! We're not being frivolous with your money! We only spend on the essentials." And this is true even for MASSIVE, PROFITABLE companies, because those companies' value is based on the Rich Person Feeling Graph (their stock) rather than the literal profit money. A company making a genuine gazillion dollars a year still tears through layoffs and freezes hiring and removes the free batteries from the printer room (totally not speaking from experience, surely) because the investors LOVE when you cut costs and take away employee perks. The "beer on tap, ping pong table in the common area" era of tech is drying up. And we're still unionless.
Never mind that last part.
And then in early 2023, AI (more specifically, Chat-GPT which is OpenAI's Large Language Model creation) tears its way into the tech scene with a meteor's amount of momentum. Here's Microsoft's prize pig, which it invested heavily in and is galivanting around the pig-show with, to the desperate jealousy and rapture of every other tech company and investor wishing it had that pig. And for the first time since the interest rate hikes, investors have dollar signs in their eyes, both venture capital and Wall Street alike. They're willing to restart the hose of money (even with the new risk) because this feels big enough for them to take the risk.
Now all these companies, who were in varying stages of sweating as their bill came due, or wringing their hands as their stock prices tanked, see a single glorious gold-plated rocket up out of here, the likes of which haven't been seen since the free money days. It's their ticket to buy time, and buy investors, and say "see THIS is what will wring money forth, finally, we promise, just let us show you."
To be clear, AI is NOT profitable yet. It's a money-sink. Perhaps a money-black-hole. But everyone in the space is so wowed by it that there is a wide-spread and powerful conviction that it will become profitable and earn its keep. (Let's be real, half of that profit "potential" is the promise of automating away jobs of pesky employees who peskily cost money.) It's a tech-space industrial revolution that will automate away skilled jobs, and getting in on the ground floor is the absolute best thing you can do to get your pie slice's worth.
It's the thing that will win investors back. It's the thing that will get the investment money coming in again (or, get it second-hand if the company can be the PROVIDER of something needed for AI, which other companies with venture-back will pay handsomely for). It's the thing companies are terrified of missing out on, lest it leave them utterly irrelevant in a future where not having AI-integration is like not having a mobile phone app for your company or not having a website.
So I guess to reiterate on my earlier point:
Drowned rats. Swimming to the one ship in sight.
36K notes
·
View notes
Text
Scientists use generative AI to answer complex questions in physics
New Post has been published on https://thedigitalinsider.com/scientists-use-generative-ai-to-answer-complex-questions-in-physics/
Scientists use generative AI to answer complex questions in physics
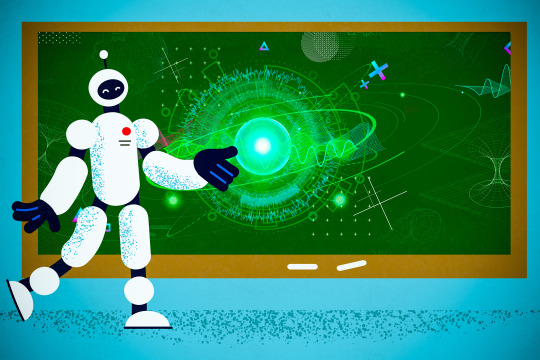
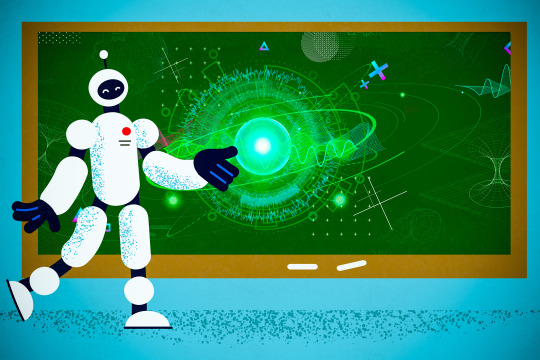
When water freezes, it transitions from a liquid phase to a solid phase, resulting in a drastic change in properties like density and volume. Phase transitions in water are so common most of us probably don’t even think about them, but phase transitions in novel materials or complex physical systems are an important area of study.
To fully understand these systems, scientists must be able to recognize phases and detect the transitions between. But how to quantify phase changes in an unknown system is often unclear, especially when data are scarce.
Researchers from MIT and the University of Basel in Switzerland applied generative artificial intelligence models to this problem, developing a new machine-learning framework that can automatically map out phase diagrams for novel physical systems.
Their physics-informed machine-learning approach is more efficient than laborious, manual techniques which rely on theoretical expertise. Importantly, because their approach leverages generative models, it does not require huge, labeled training datasets used in other machine-learning techniques.
Such a framework could help scientists investigate the thermodynamic properties of novel materials or detect entanglement in quantum systems, for instance. Ultimately, this technique could make it possible for scientists to discover unknown phases of matter autonomously.
“If you have a new system with fully unknown properties, how would you choose which observable quantity to study? The hope, at least with data-driven tools, is that you could scan large new systems in an automated way, and it will point you to important changes in the system. This might be a tool in the pipeline of automated scientific discovery of new, exotic properties of phases,” says Frank Schäfer, a postdoc in the Julia Lab in the Computer Science and Artificial Intelligence Laboratory (CSAIL) and co-author of a paper on this approach.
Joining Schäfer on the paper are first author Julian Arnold, a graduate student at the University of Basel; Alan Edelman, applied mathematics professor in the Department of Mathematics and leader of the Julia Lab; and senior author Christoph Bruder, professor in the Department of Physics at the University of Basel. The research is published today in Physical Review Letters.
Detecting phase transitions using AI
While water transitioning to ice might be among the most obvious examples of a phase change, more exotic phase changes, like when a material transitions from being a normal conductor to a superconductor, are of keen interest to scientists.
These transitions can be detected by identifying an “order parameter,” a quantity that is important and expected to change. For instance, water freezes and transitions to a solid phase (ice) when its temperature drops below 0 degrees Celsius. In this case, an appropriate order parameter could be defined in terms of the proportion of water molecules that are part of the crystalline lattice versus those that remain in a disordered state.
In the past, researchers have relied on physics expertise to build phase diagrams manually, drawing on theoretical understanding to know which order parameters are important. Not only is this tedious for complex systems, and perhaps impossible for unknown systems with new behaviors, but it also introduces human bias into the solution.
More recently, researchers have begun using machine learning to build discriminative classifiers that can solve this task by learning to classify a measurement statistic as coming from a particular phase of the physical system, the same way such models classify an image as a cat or dog.
The MIT researchers demonstrated how generative models can be used to solve this classification task much more efficiently, and in a physics-informed manner.
The Julia Programming Language, a popular language for scientific computing that is also used in MIT’s introductory linear algebra classes, offers many tools that make it invaluable for constructing such generative models, Schäfer adds.
Generative models, like those that underlie ChatGPT and Dall-E, typically work by estimating the probability distribution of some data, which they use to generate new data points that fit the distribution (such as new cat images that are similar to existing cat images).
However, when simulations of a physical system using tried-and-true scientific techniques are available, researchers get a model of its probability distribution for free. This distribution describes the measurement statistics of the physical system.
A more knowledgeable model
The MIT team’s insight is that this probability distribution also defines a generative model upon which a classifier can be constructed. They plug the generative model into standard statistical formulas to directly construct a classifier instead of learning it from samples, as was done with discriminative approaches.
“This is a really nice way of incorporating something you know about your physical system deep inside your machine-learning scheme. It goes far beyond just performing feature engineering on your data samples or simple inductive biases,” Schäfer says.
This generative classifier can determine what phase the system is in given some parameter, like temperature or pressure. And because the researchers directly approximate the probability distributions underlying measurements from the physical system, the classifier has system knowledge.
This enables their method to perform better than other machine-learning techniques. And because it can work automatically without the need for extensive training, their approach significantly enhances the computational efficiency of identifying phase transitions.
At the end of the day, similar to how one might ask ChatGPT to solve a math problem, the researchers can ask the generative classifier questions like “does this sample belong to phase I or phase II?” or “was this sample generated at high temperature or low temperature?”
Scientists could also use this approach to solve different binary classification tasks in physical systems, possibly to detect entanglement in quantum systems (Is the state entangled or not?) or determine whether theory A or B is best suited to solve a particular problem. They could also use this approach to better understand and improve large language models like ChatGPT by identifying how certain parameters should be tuned so the chatbot gives the best outputs.
In the future, the researchers also want to study theoretical guarantees regarding how many measurements they would need to effectively detect phase transitions and estimate the amount of computation that would require.
This work was funded, in part, by the Swiss National Science Foundation, the MIT-Switzerland Lockheed Martin Seed Fund, and MIT International Science and Technology Initiatives.
#ai#approach#artificial#Artificial Intelligence#Bias#binary#change#chatbot#chatGPT#classes#computation#computer#Computer modeling#Computer Science#Computer Science and Artificial Intelligence Laboratory (CSAIL)#Computer science and technology#computing#crystalline#dall-e#data#data-driven#datasets#dog#efficiency#Electrical Engineering&Computer Science (eecs)#engineering#Foundation#framework#Future#generative
2 notes
·
View notes