#Enterprise GenAI Framework
Explore tagged Tumblr posts
Text
Know about Needle: Enterprise Generative AI Framework
0 notes
Text

💡 Unlock the Power of AI! 💡
Join our Interactive Masterclass and explore: ✨ Building GenAI Products from Scratch. Gain hands-on experience with frameworks, tools, and strategies to create enterprise-ready AI solutions.
📅 Date: 25th January 2025 🕒 Time: 12:00 PM - 3:00 PM 📍 Location: 8Q5P+QPH Sona Sujan Appartment, ProLEAP Academy, #004, First Ln Ext, Gandhi Nagar, Brahmapur, Odisha 760001
🔥 What’s in it for you? ✅ Learn from industry veterans ✅ Get insights into GenAI ✅ Interactive Q&A and quiz session
🔗 Seats are limited! For Registration: Scan QR/(link in bio)
#GenAI#TechMasterclass#Innovation#EnterpriseTech#LearnAndLead
0 notes
Text
Strategies for Measuring ROI with Generative AI in Enterprises

In today’s rapidly evolving technological landscape, Generative AI (GenAI) has emerged as a game-changer for businesses across industries. From content creation and customer support to product design and data analysis, GenAI has shown immense potential to drive efficiency, innovation, and profitability. However, like any transformative technology, enterprises must ensure that their investments in GenAI yield measurable returns. Understanding how to effectively measure Generative AI ROI (Return on Investment) is essential to justify adoption, scale successful initiatives, and refine AI strategies over time. This article explores strategies for measuring the ROI of Generative AI in enterprises, drawing on real-world case studies, insights from the GenAI maturity model, and the latest GenAI solutions and training programs.
1. Defining Clear ROI Metrics for GenAI Projects
Before diving into GenAI use cases, it's crucial for enterprises to define clear and measurable ROI metrics. Unlike traditional software or automation tools, the value of Generative AI can be multifaceted, encompassing both tangible and intangible outcomes. Key metrics for evaluating GenAI ROI might include:
Cost Savings: How much has the use of GenAI reduced operational costs? For example, using AI to automate customer support or content generation can reduce the need for human labor, lowering operational expenses.
Productivity Gains: Has GenAI improved productivity? Automation of repetitive tasks, enhanced data processing, or accelerated product development timelines can result in more output with fewer resources.
Revenue Growth: Does GenAI contribute to increasing revenue? AI-driven personalization, predictive analytics, and optimized marketing campaigns can result in higher conversion rates and customer retention, driving sales.
Customer Satisfaction: How does the use of GenAI impact customer experience? AI-powered solutions like chatbots or personalized recommendations can lead to enhanced customer satisfaction, indirectly boosting retention and loyalty.
2. Leveraging GenAI Case Studies for Benchmarking
One of the most effective ways to measure ROI is by studying how other enterprises have implemented Generative AI. GenAI case studies offer valuable lessons and benchmarks, showcasing how companies across industries have achieved success and quantified their returns. For instance:
Content Creation in Media & Entertainment: Companies like OpenAI and Copy.ai have empowered marketing teams to generate personalized, high-quality content at scale. These businesses have reported significant cost reductions in content production, while also improving content relevance and engagement metrics. A key takeaway is that time saved on content creation directly correlates with revenue growth from improved digital marketing strategies.
Customer Support Automation: In the financial services industry, companies have used AI-powered chatbots to handle routine inquiries. This not only cuts down on operational costs but also allows human agents to focus on more complex queries. Enterprises that integrated chatbots reported faster response times and better overall customer satisfaction scores, which contributed to increased customer loyalty and reduced churn.
By examining similar use cases, companies can develop a clearer understanding of the potential ROI of their own GenAI projects, set more realistic expectations, and identify the metrics that matter most for their specific needs.
3. Adopting the GenAI Maturity Model
The GenAI maturity model provides a framework to assess where an organization stands in its journey of adopting Generative AI technologies. By understanding their current maturity level, businesses can tailor their ROI measurement strategies to suit their stage of GenAI adoption.
Stage 1 – Exploration: At this initial stage, organizations are experimenting with AI tools and technologies. ROI measurement here is often qualitative, focusing on the potential of GenAI solutions and exploring early use cases. The ROI is more about validating the feasibility of AI initiatives rather than immediate financial returns.
Stage 2 – Expansion: Once GenAI tools are deployed on a larger scale, businesses start seeing more tangible benefits. Metrics such as reduced time to market, lower operational costs, and improved efficiency become more measurable.
Stage 3 – Optimization: At this stage, enterprises optimize their AI models, fine-tuning for performance and scalability. ROI measurements here are more sophisticated, including advanced KPIs like customer lifetime value (CLV), cross-sell and up-sell success, and market share gains.
Stage 4 – Transformation: Organizations at this maturity stage have fully integrated GenAI into their business operations. ROI is now reflected in strategic outcomes such as competitive advantage, accelerated innovation, and deep data-driven decision-making.
Using the GenAI maturity model helps businesses understand their current position and define ROI benchmarks that align with their adoption trajectory.
4. Utilizing GenAI Insights to Guide Investment Decisions
The ability to measure and act on GenAI insights is key to understanding the true ROI of these technologies. Generative AI can provide valuable insights through data-driven predictions, patterns, and trends that businesses can leverage to refine their strategies. For instance, by analyzing customer behavior and preferences, companies can optimize product offerings, marketing campaigns, and sales processes.
Predictive Analytics: With advanced predictive models, GenAI can help businesses forecast demand, manage inventory, and personalize offerings, leading to improved business outcomes and cost efficiencies.
Customer Insights: Understanding the nuances of customer preferences and behavior allows businesses to tailor their services or products more effectively, improving customer retention and lifetime value.
By leveraging these insights, enterprises can make more informed decisions about where to invest in GenAI and track the direct impact on their ROI.
5. Investing in GenAI Training Programs for Long-Term Success
An often overlooked aspect of measuring ROI is the readiness and capability of the workforce to leverage GenAI effectively. To maximize ROI, businesses should invest in GenAI training programs to upskill their employees. These programs help employees understand the technology, integrate it into daily workflows, and use it to its full potential.
The more proficient the team becomes at using GenAI tools, the more likely the organization will see the benefits in terms of productivity gains, improved problem-solving, and innovation. This investment in human capital, though indirect, can lead to significant long-term ROI.
Conclusion
As Generative AI continues to reshape industries, measuring its ROI becomes a crucial task for enterprises looking to stay competitive. By defining clear ROI metrics, learning from GenAI case studies, leveraging the GenAI maturity model, extracting actionable insights, and investing in training, businesses can ensure they are making informed decisions that drive value from their GenAI initiatives. In the end, Generative AI is not just about the technology itself, but about how organizations leverage it to enhance efficiency, foster innovation, and create a sustainable competitive edge.
Find more info:-
Managing AI expectations with the board
GenAI Case Studies
GenAI Insights
GenAI Solutions
0 notes
Text
Agentic RAG On Dell & NVIDIA Changes AI-Driven Data Access

Agentic RAG Changes AI Data Access with Dell & NVIDIA
The secret to successfully implementing and utilizing AI in today’s corporate environment is comprehending the use cases within the company and determining the most effective and frequently quickest AI-ready strategies that produce outcomes fast. There is also a great need for high-quality data and effective retrieval techniques like RAG retrieval augmented generation. The value of AI for businesses is further accelerated at SC24 by fresh innovation at the Dell AI Factory with NVIDIA, which also gets them ready for the future.
AI Applications Place New Demands
GenAI applications are growing quickly and proliferating throughout the company as businesses gain confidence in the results of applying AI to their departmental use cases. The pressure on the AI infrastructure increases as the use of larger, foundational LLMs increases and as more use cases with multi-modal outcomes are chosen.
RAG’s capacity to facilitate richer decision-making based on an organization’s own data while lowering hallucinations has also led to a notable increase in interest. RAG is particularly helpful for digital assistants and chatbots with contextual data, and it can be easily expanded throughout the company to knowledge workers. However, RAG’s potential might still be limited by inadequate data, a lack of multiple sourcing, and confusing prompts, particularly for large data-driven businesses.
It will be crucial to provide IT managers with a growth strategy, support for new workloads at scale, a consistent approach to AI infrastructure, and innovative methods for turning massive data sets into useful information.
Raising the AI Performance bar
The performance for AI applications is provided by the Dell AI Factory with NVIDIA, giving clients a simplified way to deploy AI using a scalable, consistent, and outcome-focused methodology. Dell is now unveiling new NVIDIA accelerated compute platforms that have been added to Dell AI Factory with NVIDIA. These platforms offer acceleration across a wide range of enterprise applications, further efficiency for inferencing, and performance for developing AI applications.
The NVIDIA HGX H200 and NVIDIA H100 NVL platforms, which are supercharging data centers, offer state-of-the-art technology with enormous processing power and enhanced energy efficiency for genAI and HPC applications. Customers who have already implemented the Dell AI Factory with NVIDIA may quickly grow their footprint with the same excellent foundations, direction, and support to expedite their AI projects with these additions for PowerEdge XE9680 and rack servers. By the end of the year, these combinations with NVIDIA HGX H200 and H100 NVL should be available.
Deliver Informed Decisions, Faster
RAG already provides enterprises with genuine intelligence and increases productivity. Expanding RAG’s reach throughout the company, however, may make deployment more difficult and affect quick response times. In order to provide a variety of outputs, or multi-modal outcomes, large, data-driven companies, such as healthcare and financial institutions, also require access to many data kinds.
Innovative approaches to managing these enormous data collections are provided by agentic RAG. Within the RAG framework, it automates analysis, processing, and reasoning through the use of AI agents. With this method, users may easily combine structured and unstructured data, providing trustworthy, contextually relevant insights in real time.
Organizations in a variety of industries can gain from a substantial advancement in AI-driven information retrieval and processing with Agentic RAG on the Dell AI Factory with NVIDIA. Using the healthcare industry as an example, the agentic RAG design demonstrates how businesses can overcome the difficulties posed by fragmented data (accessing both structured and unstructured data, including imaging files and medical notes, while adhering to HIPAA and other regulations). The complete solution, which is based on the NVIDIA and Dell AI Factory platforms, has the following features:
PowerEdge servers from Dell that use NVIDIA L40S GPUs
Storage from Dell PowerScale
Spectrum-X Ethernet networking from NVIDIA
Platform for NVIDIA AI Enterprise software
Together with the NVIDIA Llama-3.1-8b-instruct LLM NIM microservice, NVIDIA NeMo embeds and reranks NVIDIA NIM microservices.
The recently revealed NVIDIA Enterprise Reference Architecture for NVIDIA L40S GPUs serves as the foundation for the solution, which allows businesses constructing AI factories to power the upcoming generation of generative AI solutions cut down on complexity, time, and expense.
A thorough beginning strategy for enterprises to modify and implement their own Agentic RAG and raise the standard of value delivery is provided by the full integration of these components.
Readying for the Next Era of AI
As employees, developers, and companies start to use AI to generate value, new applications and uses for the technology are released on a daily basis. It can be intimidating to be ready for a large-scale adoption, but any company can change its operations with the correct strategy, partner, and vision.
The Dell AI factory with NVIDIA offers a scalable architecture that can adapt to an organization’s changing needs, from state-of-the-art AI operations to enormous data set ingestion and high-quality results.
The first and only end-to-end enterprise AI solution in the industry, the Dell AI Factory with NVIDIA, aims to accelerate the adoption of AI by providing integrated Dell and NVIDIA capabilities to speed up your AI-powered use cases, integrate your data and workflows, and let you create your own AI journey for scalable, repeatable results.
What is Agentic Rag?
An AI framework called Agentic RAG employs intelligent agents to do tasks beyond creating and retrieving information. It is a development of the classic Retrieval-Augmented Generation (RAG) method, which blends generative and retrieval-based models.
Agentic RAG uses AI agents to:
Data analysis: Based on real-time input, agentic RAG systems are able to evaluate data, improve replies, and make necessary adjustments.
Make choices: Agentic RAG systems are capable of making choices on their own.
Dividing complicated tasks into smaller ones and allocating distinct agents to each component is possible with agentic RAG systems.
Employ external tools: To complete tasks, agentic RAG systems can make use of any tool or API.
Recall what has transpired: Because agentic RAG systems contain memory, like as chat history, they are aware of past events and know what to do next.
For managing intricate questions and adjusting to changing information environments, agentic RAG is helpful. Applications for it are numerous and include:
Management of knowledge
Large businesses can benefit from agentic RAG systems’ ability to generate summaries, optimize searches, and obtain pertinent data.
Research
Researchers can generate analyses, synthesize findings, and access pertinent material with the use of agentic RAG systems.
Read more on govindhtech.com
#AgenticRAG#NVIDIAChanges#dell#AIDriven#ai#DataAccess#RAGretrievalaugmentedgeneration#DellAIFactory#NVIDIAHGXH200#PowerEdgeXE9680#NVIDIAL40SGPU#DellPowerScale#generativeAI#RetrievalAugmentedGeneration#rag#technology#technews#news#govindhtech
0 notes
Text
Kein DLP: Datenabfluss durch generative KIs

Mehr als ein Drittel der Daten die in generative KI-Apps eingegeben werden, sind sensible Geschäftsinformationen. Der Einsatz von generativer KI hat sich in den letzten 12 Monaten mehr als verdreifacht. Hier fehlen Data Loss Prevention (DLP)-Lösungen. Aus der neuen Studie geht hervor, dass mehr als ein Drittel der sensiblen Daten, die mit generativen KI-Tools ausgetauscht werden, regulierte Daten sind – Daten, zu deren Schutz Unternehmen gesetzlich verpflichtet sind. Dies stellt für Unternehmen ein potenzielles Risiko für kostspielige Datenschutzverletzungen dar. KI-Apps nur teilweise blockiert Die neue Studie von Netskope Threat Labs zeigt, dass drei Viertel der befragten Unternehmen mindestens eine genAI-App vollständig blockieren. Dies spiegelt den Wunsch der Technologieverantwortlichen in Unternehmen wider, das Risiko der Verbreitung sensibler Daten zu begrenzen. Da jedoch weniger als die Hälfte der Unternehmen datenbezogene Kontrollen anwenden, um zu verhindern, dass sensible Informationen weitergegeben werden, sind die meisten Unternehmen im Rückstand bei der Einführung fortschrittlicher Data Loss Prevention (DLP)-Lösungen. Diese sind für die sichere Nutzung von genAI jedoch erforderlich. Keine vorhandene Data Loss Prevention (DLP)-Lösungen Anhand globaler Datensätze fanden die Forscher heraus, dass 96 % der Unternehmen inzwischen genAI einsetzen – eine Zahl, die sich in den letzten 12 Monaten verdreifacht hat. Im Durchschnitt nutzen Unternehmen jetzt fast zehn genAI-Apps, im letzten Jahr waren es noch drei. Die Top 1 % der Unternehmen, die genAI einsetzen, nutzen jetzt durchschnittlich 80 Apps, eine deutliche Steigerung von zuvor 14. Mit der zunehmenden Nutzung haben Unternehmen einen Anstieg bei der Freigabe von firmeneigenem Quellcode innerhalb von genAI-Apps erlebt, der 46 % aller dokumentierten Verstöße gegen die Datenrichtlinie ausmacht. Diese sich verändernde Dynamik erschwert die Risikokontrolle in Unternehmen und macht stärkere DLP-Maßnahmen erforderlich. Es gibt positive Anzeichen für ein proaktives Risikomanagement bei verschiedenen Sicherheits- und Datenverlustkontrollen, die Unternehmen anwenden: Beispielsweise implementieren 65 % der Unternehmen jetzt ein Nutzer-Coaching in Echtzeit, um die Anwenderinteraktionen mit genAI-Apps zu steuern. Laut der Studie spielt ein effektives Nutzer-Coaching eine entscheidende Rolle bei der Minderung von Datenrisiken, da 57 % der Nutzer ihre Handlungen nach dem Erhalt von Warnungen ändern. Wichtige Erkenntnisse der Studie Der Cloud- und Threat Report von Netskope „AI Apps in the Enterprise“ zeigt außerdem: - ChatGPT bleibt die beliebteste App – sie wird von mehr als 80 % der Unternehmen genutzt - Microsoft Copilot verzeichnete mit 57 % den stärksten Anstieg der Nutzung seit seiner Einführung im Januar 2024 - 19 % der Unternehmen haben ein generelles Verbot von GitHub CoPilot erhoben Wichtige Erkenntnisse für Unternehmen Netskope empfiehlt Unternehmen, ihre Risiko-Frameworks zu überprüfen, anzupassen und speziell auf AI oder genAI zuzuschneiden, indem sie Ansätze wie das NIST AI Risk Management Framework nutzen. Taktische Schritte zur Bewältigung von Risiken durch genAI: - Kennen Sie Ihren aktuellen Stand: Beginnen Sie damit, die aktuelle Nutzung von KI und maschinellem Lernen, Datenpipelines und genAI-Anwendungen zu bewerten. Identifizieren Sie Schwachstellen und Lücken in den Sicherheitskontrollen. - Kernkontrollen implementieren: Legen Sie grundlegende Sicherheitsmaßnahmen fest, wie z. B. Zugriffskontrollen, Authentifizierungsmechanismen und Verschlüsselungen. - Erweiterte Kontrollmaßnahmen planen: Entwickeln Sie über die Grundlagen hinaus eine Roadmap für erweiterte Sicherheitskontrollen. Ziehen Sie Bedrohungsmodellierung, Anomalieerkennung, kontinuierliche Überwachung und Verhaltenserkennung in Betracht, um verdächtige Datenbewegungen in Cloud-Umgebungen und genAI-Apps zu identifizieren, die von normalen Verhaltensmustern abweichen. - Messen, Starten, Überarbeiten, Wiederholen: Bewerten Sie regelmäßig die Wirksamkeit Ihrer Sicherheitsmaßnahmen. Passen Sie sie an und verfeinern Sie diese auf der Grundlage von Erfahrungen aus der Praxis und neu auftretenden Bedrohungen. Passende Artikel zum Thema Lesen Sie den ganzen Artikel
0 notes
Text
GAI Insights Launches Inaugural Generative AI Conference in Boston
GAI Insights is excited to announce the launch of its inaugural Generative AI Conference, set to take place on October 7-8, 2024, at the InterContinental Hotel in Boston, MA. This landmark event, dubbed "Generative AI World 2024," promises to be a pivotal gathering for leaders and innovators in the AI industry.

The conference will feature a series of insightful sessions, including AI case studies, AI investment trends, and AI technology trends. Attendees will have the opportunity to explore the latest advancements in AI, learn from industry experts, and network with peers who are at the forefront of AI innovation.
Key highlights of the conference include:
AI Innovation Summit: Explore groundbreaking AI technologies and their applications.
AI Strategy Conference: Discover effective strategies for integrating AI into your business.
Enterprise OpenAI: Understand the implications of OpenAI's developments for enterprises.
GenAI in Insurance: Learn how generative AI is transforming the insurance sector.
GenAI in Financial Services: Gain insights into the adoption of AI in financial services.
GenAI in Life Sciences: Explore AI's impact on the life sciences industry.
The event will also feature renowned speakers such as John Sviokla and Paul Baier, who will share their expertise on AI leadership and the latest trends in the artificial intelligence industry. Attendees can look forward to sessions on how to prioritize GenAI projects, what is the best LLM (Large Language Model), and the WINS framework for AI strategy.

In addition to the educational sessions, the conference will provide a platform for companies to showcase their AI solutions and innovations. The Executive AI Summit will focus on AI business insights and the Corporate Buyers Guide to LLMs, offering valuable information for corporate leaders and decision-makers.
The Generative AI Conference is designed to foster dialogue and collaboration, with sessions like "Design with Dialogue" encouraging interactive discussions. Attendees will leave with actionable insights and a deeper understanding of how to leverage AI for business success.
Don't miss this premier event in the AI industry. Join us in Boston for Generative AI World 2024 and be part of the future of AI innovation. For more information and to register, visit the official conference website. https://www.generativeaiworld2024.com/
0 notes
Text
Enterprise GenAI Governance Framework Offers Deep, Robust Expertise for Responsible GenAI Implementation http://dlvr.it/T8jG1V
0 notes
Text
Enterprise GenAI Governance Framework Offers Deep, Robust Expertise for Responsible GenAI Implementation
http://securitytc.com/T8jG3z
0 notes
Photo

1,000+ business leaders collaborate to create Enterprise GenAI Governance Framework
0 notes
Text
Narrowing the confidence gap for wider AI adoption - AI News
New Post has been published on https://thedigitalinsider.com/narrowing-the-confidence-gap-for-wider-ai-adoption-ai-news/
Narrowing the confidence gap for wider AI adoption - AI News
.pp-multiple-authors-boxes-wrapper display:none; img width:100%;
Artificial intelligence entered the market with a splash, driving massive buzz and adoption. But now the pace is faltering.
Business leaders still talk the talk about embracing AI, because they want the benefits – McKinsey estimates that GenAI could save companies up to $2.6 trillion across a range of operations. However, they aren’t walking the walk. According to one survey of senior analytics and IT leaders, only 20% of GenAI applications are currently in production.
Why the wide gap between interest and reality?
The answer is multifaceted. Concerns around security and data privacy, compliance risks, and data management are high-profile, but there’s also anxiety about AI’s lack of transparency and worries about ROI, costs, and skill gaps. In this article, we’ll examine the barriers to AI adoption, and share some measures that business leaders can take to overcome them.
Get a handle on data
“High-quality data is the cornerstone of accurate and reliable AI models, which in turn drive better decision-making and outcomes,” said Rob Johnson, VP and Global Head of Solutions Engineering at SolarWinds, adding, “Trustworthy data builds confidence in AI among IT professionals, accelerating the broader adoption and integration of AI technologies.”
Today, only 43% of IT professionals say they’re confident about their ability to meet AI’s data demands. Given that data is so vital for AI success, it’s not surprising that data challenges are an oft-cited factor in slow AI adoption.
The best way to overcome this hurdle is to go back to data basics. Organisations need to build a strong data governance strategy from the ground up, with rigorous controls that enforce data quality and integrity.
Take ethics and governance seriously
With regulations mushrooming, compliance is already a headache for many organisations. AI only adds new areas of risk, more regulations, and increased ethical governance issues for business leaders to worry about, to the extent that security and compliance risk was the most-cited concern in Cloudera’s State of Enterprise AI and Modern Data Architecture report.
While the rise in AI regulations might seem alarming at first, executives should embrace the support that these frameworks offer, as they can give organisations a structure around which to build their own risk controls and ethical guardrails.
Developing compliance policies, appointing teams for AI governance, and ensuring that humans retain authority over AI-powered decisions are all important steps in creating a comprehensive system of AI ethics and governance.
Reinforce control over security and privacy
Security and data privacy concerns loom large for every business, and with good reason. Cisco’s 2024 Data Privacy Benchmark Study revealed that 48% of employees admit to entering non-public company information into GenAI tools (and an unknown number have done so and won’t admit it), leading 27% of organisations to ban the use of such tools.
The best way to reduce the risks is to limit access to sensitive data. This involves doubling down on access controls and privilege creep, and keeping data away from publicly-hosted LLMs. Avi Perez, CTO of Pyramid Analytics, explained that his business intelligence software’s AI infrastructure was deliberately built to keep data away from the LLM, sharing only metadata that describes the problem and interfacing with the LLM as the best way for locally-hosted engines to run analysis.”There’s a huge set of issues there. It’s not just about privacy, it’s also about misleading results. So in that framework, data privacy and the issues associated with it are tremendous, in my opinion. They’re a showstopper,” Perez said. With Pyramid’s setup, however, “the LLM generates the recipe, but it does it without ever getting [its] hands on the data, and without doing mathematical operations. […] That eliminates something like 95% of the problem, in terms of data privacy risks.”
Boost transparency and explainability
Another serious obstacle to AI adoption is a lack of trust in its results. The infamous story of Amazon’s AI-powered hiring tool which discriminated against women has become a cautionary tale that scares many people away from AI. The best way to combat this fear is to increase explainability and transparency.
“AI transparency is about clearly explaining the reasoning behind the output, making the decision-making process accessible and comprehensible,” said Adnan Masood, chief AI architect at UST and a Microsoft regional director. “At the end of the day, it’s about eliminating the black box mystery of AI and providing insight into the how and why of AI decision-making.”Unfortunately, many executives overlook the importance of transparency. A recent IBM study reported that only 45% of CEOs say they are delivering on capabilities for openness. AI champions need to prioritise the development of rigorous AI governance policies that prevent black boxes arising, and invest in explainability tools like SHapley Additive exPlanations (SHAPs), fairness toolkits like Google’s Fairness Indicators, and automated compliance checks like the Institute of Internal Auditors’ AI Auditing Framework.
Define clear business value
Cost is on the list of AI barriers, as always. The Cloudera survey found that 26% of respondents said AI tools are too expensive, and Gartner included “unclear business value” as a factor in the failure of AI projects. Yet the same Gartner report noted that GenAI had delivered an average revenue increase and cost savings of over 15% among its users, proof that AI can drive financial lift if implemented correctly.
This is why it’s crucial to approach AI like every other business project – identify areas that will deliver fast ROI, define the benefits you expect to see, and set specific KPIs so you can prove value.”While there’s a lot that goes into building out an AI strategy and roadmap, a critical first step is to identify the most valuable and transformative AI use cases on which to focus,” said Michael Robinson, Director of Product Marketing at UiPath.
Set up effective training programs
The skills gap remains a significant roadblock to AI adoption, but it seems that little effort is being made to address the issue. A report from Worklife indicates the initial boom in AI adoption came from early adopters. Now, it’s down to the laggards, who are inherently sceptical and generally less confident about AI – and any new tech.
This makes training crucial. Yet according to Asana’s State of AI at Work study, 82% of participants said their organisations haven’t provided training on using generative AI. There’s no indication that training isn’t working; rather that it isn’t happening as it should.
The clear takeaway is to offer comprehensive training in quality prompting and other relevant skills. Encouragingly, the same research shows that even using AI without training increases people’s skills and confidence. So, it’s a good idea to get started with low- and no-code tools that allow employees who are unskilled in AI to learn on the job.
The barriers to AI adoption are not insurmountable
Although AI adoption has slowed, there’s no indication that it’s in danger in the long term. The many obstacles holding companies back from rolling out AI tools can be overcome without too much trouble. Many of the steps, like reinforcing data quality and ethical governance, should be taken regardless of whether or not AI is under consideration, while other steps taken will pay for themselves in increased revenue and the productivity gains that AI can bring.
#2024#adoption#ai#AI adoption#AI auditing#AI Ethics#AI Infrastructure#AI models#ai news#AI strategy#ai tools#ai transparency#ai use cases#AI-powered#Amazon#Analysis#Analytics#anxiety#applications#approach#architecture#Article#artificial#Artificial Intelligence#Asana#author#benchmark#benchmark study#black box#box
0 notes
Text
Future Trends in Data Consulting: What Businesses Need to Know
Developers of corporate applications involving data analysis have embraced novel technologies like generative artificial intelligence (GenAI) to accelerate reporting. Meanwhile, developing countries have encouraged industries to increase their efforts toward digital transformation and ethical analytics. This post will explain similar future trends in data consulting that businesses need to know.
What is Data Consulting?
Data consulting encompasses helping corporations develop strategies, technologies, and workflows. It enables efficient data sourcing, validation, analysis, and visualization. Consultants across business intelligence and data strategy also improve organizational resilience to governance risks through cybersecurity and legal compliance assistance.
Reputed data management and analytics firms perform executive duties alongside client enterprises’ in-house professionals. Likewise, data engineers and architects lead ecosystem development. Meanwhile, data strategy & consulting experts oversee a company’s policy creation, business alignment, and digital transformation journey.
Finally, data consultants must guide clients on data quality management (DQM) and business process automation (BPA). The former ensures analytical models remain bias-free and provide realistic insights relevant to long-term objectives. The latter accelerates productivity by reducing delays and letting machines complete mundane activities. That is why artificial intelligence (AI) integration attracts stakeholders in the data consulting industry.
Future Trends in Data Consulting
1| Data Unification and Multidisciplinary Collaboration
Data consultants will utilize unified interfaces to harmonize data consumption among several departments in an organization. Doing so will eliminate the need for frequent updates to the central data resources. Moreover, multiple business units can seamlessly exchange their business intelligence, mitigating the risks of silo formation, toxic competitiveness, or data duplication.
Unified data offers more choices to represent organization-level data according to diverse products and services based on global or regional performance metrics. At the same time, leaders can identify macroeconomic and microeconomic threats to business processes without jumping between a dozen programs.
According to established strategic consulting services, cloud computing has facilitated the ease of data unification, modernization, and collaborative data operations. However, cloud adoption might be challenging depending on a company’s reliance on legacy systems. As a result, brands want domain specialists to implement secure data migration methods for cloud-enabled data unification.
2| Impact-Focused Consulting
Carbon emissions, electronic waste generation, and equitable allocation of energy resources among stakeholders have pressurized many mega-corporations to review their environmental impact. Accordingly, ethical and impact investors have applied unique asset evaluation methods to dedicate their resources to sustainable companies.
Data consulting professionals have acknowledged this reality and invested in innovating analytics and tech engineering practices to combat carbon risks. Therefore, global brands seek responsible data consultants to enhance the on-ground effectiveness of their sustainability accounting initiatives.
An impact-focused data consulting partner might leverage reputed frameworks to audit an organization’s compliance concerning environmental, social, and governance (ESG) metrics. It will also tabulate and visualize them to let decision-makers study compliant and non-compliant activities.
Although ecological impact makes more headlines, social and regulatory factors are equally significant. Consequently, stakeholders expect modern data consultants to deliver 360-degree compliance reporting and business improvement recommendations.
3| GenAI Integration
Generative artificial intelligence (GenAI) exhibits text, image, video, and audio synthesis capabilities. Therefore, several industries are curious about how GenAI tools can streamline data operations. This situation hints at a growing tendency among strategy and data consulting experts to deliver GenAI-led data integration and reporting solutions.
Still, optimizing generative AI programs to address business-relevant challenges takes a lot of work. After all, an enterprise must find the best talent, train workers on advanced computing concepts, and invite specialists to modify current data workflows.
GenAI is one of the noteworthy trends in data consulting because it potentially affects the future of human participation in data sourcing, quality assurance, insight extraction, and presentation.
Its integration across business functions is also divisive. Understandably, GenAI enthusiasts believe the new tech tools will reduce employee workload and encourage creative problem-solving instead of conventional intuitive troubleshooting. On the other hand, critics have concerns about the misuse of GenAI or the reliability of the synthesized output.
However, communicating the scope and safety protocols associated with GenAI adoption must enable brands to address stakeholder doubts. Experienced strategy consultants can assist companies in this endeavor.
4| Data Localization
Countries fear that foreign companies will gather citizens’ personally identifiable information (PII) for unwarranted surveillance on behalf of their parent nations' governments. This sentiment overlaps with the rise of protectionism across some of the world’s most influential and populated geopolitical territories.
Data localization is a multi-stakeholder data strategy assures data subjects, regulators, and industry peers that a brand complies with regional data protection laws. For instance, it is crucial for brands wanting to comply with data localization norms to store citizens' data in a data center physically located within the country’s internationally recognized borders.
Policymakers couple it with consumer privacy, investor confidentiality, and cybersecurity standards. As a result, data localization has become a new corporate governance opportunity. However, global organizations will require more investments to execute a data localization strategy, indicating a need for relevant data consulting.
Data localization projects might be expensive trends in the data and strategy consulting world, but they are vital for a future with solid data security. Besides, all sovereign nations consider it integral to national security since unwarranted foreign surveillance can damage citizens’ faith in their government bodies and defense institutions. For example, some nations can utilize PII datasets to interfere with another country’s elections or similar civic processes.
Conclusion
Data consultant helps enterprises, governments, global associations, and non-governmental organizations throughout all strategy creation efforts. Furthermore, they examine regulatory risks to rectify them before it is too late. Like many other industries, the data consulting and strategy industry has undergone tremendous changes with the rise of novel tech tools.
Today, several data consultants have redesigned their deliverables to fulfill the needs of sustainability-focused investors and companies. Simultaneously, unifying data from multiple departments for single dashboard experiences has become more manageable thanks to the cloud.
Nevertheless, complying with data localization norms remains a bittersweet business aspect for corporations. They want to meet stakeholder expectations, but starting from scratch implies expensive infrastructure development. So, collaborating with regional data consulting professionals is strategically more appropriate.
Finally, GenAI, a technological marvel set to reshape human-machine interactions, has an unexplored potential to lead the next data analysis and business intelligence breakthroughs. It can accelerate report generation and contextual information categorization. Unsurprisingly, generative artificial intelligence tools for analytics are the unmissable trends in data consulting, promising a brighter future.
1 note
·
View note
Text
Hewlett Packard Enterprise Collaborates With NVIDIA To Deliver An Enterprise-class, Full-stack GenAI Solution
Recently, Hewlett Packard Enterprise (HPE) announced an expanded strategic partnership with NVIDIA with the goal of creating an all-encompassing enterprise computing solution for generative artificial intelligence (GenAI). The jointly-engineered solution offers pre-configured AI tuning and inferencing capabilities, enabling businesses to deploy production apps seamlessly across a variety of contexts, from the edge to the cloud, and quickly customize foundation models using private data.
As businesses use generative AI models more and more for applications like conversational search, business process automation, and content creation, there is a rising need for effective and deployable GenAI infrastructure. This partnership aims to meet that demand. A comprehensive relationship between HPE and NVIDIA offers full-stack AI solutions that integrate many components, including the recently announced enterprise computing solution for generative AI.
NVIDIA's AI Enterprise software suite, which includes the NVIDIA NeMo framework, is integrated with HPE's Machine Learning Development Environment Software, HPE Ezmeral Software, HPE ProLiant Compute, and HPE Cray Supercomputers for this solution. By offering a complete, off-the-shelf solution, this integration seeks to streamline the creation and implementation of GenAI models.
The importance of the partnership in lowering obstacles for companies wishing to transform using AI was stressed by Antonio Neri, President and CEO of HPE. The generative AI era is rapidly approaching, as Jensen Huang, the founder and CEO of NVIDIA, noted. He also discussed how the company's partnership with HPE can increase workplace productivity with tools like semantic search, intelligent chatbots, and accurate assistants.
Read More - https://bit.ly/3R2F375
0 notes
Text
How Can GenAI Help Drive ROI for Enterprises?

As businesses continue to look for innovative ways to increase efficiency and profitability, Generative AI (GenAI) has emerged as a game-changer. Whether you are in finance, healthcare, marketing, or any other sector, integrating Enterprise GenAI solutions into your operations can help unlock new opportunities and optimize existing ones. But how exactly can GenAI drive Return on Investment (ROI) for enterprises? In this article, we will explore the various ways that Enterprise GenAI, powered by platforms like OpenAI, can boost ROI and transform businesses.
1. Unlocking Efficiency with GenAI Solutions
One of the primary ways GenAI can contribute to ROI is through the optimization of business processes. By automating repetitive tasks and generating high-quality content, enterprises can free up valuable human resources to focus on higher-level strategic work.
For example, in marketing, GenAI can be used to automate content creation, from blog posts to social media updates, saving time and reducing costs. Similarly, in customer service, AI-powered chatbots and virtual assistants can handle a large volume of inquiries, improving efficiency and customer satisfaction.
2. Enterprise GenAI for Data-Driven Decision Making
Another significant ROI driver is the ability to leverage Enterprise GenAI for advanced data analysis. With access to vast amounts of data, GenAI tools can help enterprises uncover insights that were previously difficult or time-consuming to extract. By analyzing data from various sources, businesses can make informed decisions that lead to improved performance, reduced risks, and greater profitability.
For instance, in supply chain management, AI can predict demand fluctuations, optimize inventory, and streamline logistics. In financial services, GenAI models can detect fraud patterns, assess investment risks, and provide recommendations for optimized portfolio management.
3. GenAI Case Studies: Real-World Applications of ROI
Understanding the practical applications of GenAI in various industries is key to grasping how it drives ROI. Here are a few GenAI case studies that showcase its potential:
Healthcare: A healthcare provider used an Enterprise GenAI solution to analyze patient data and optimize treatment plans. By identifying patterns in patient responses to treatments, the system provided actionable insights that improved patient outcomes and reduced unnecessary costs. This case study highlights how GenAI can optimize decision-making in highly regulated industries, driving both efficiency and profitability.
Retail: A major retailer integrated GenAI into its marketing operations, using AI to personalize advertisements and promotional offers. As a result, the company saw an increase in customer engagement and higher conversion rates, directly translating to a boost in sales and revenue.
Financial Services: A financial institution utilized GenAI for fraud detection and credit scoring. By automating these processes, they reduced errors and human bias, resulting in better decision-making, improved customer trust, and ultimately, stronger financial performance.
4. GenAI Maturity Model: Assessing Readiness for AI Integration
To maximize ROI from GenAI, enterprises must assess their maturity in adopting AI technologies. The GenAI maturity model offers a framework to evaluate where an organization stands in terms of its GenAI capabilities. It typically involves several stages, including:
Initial: The enterprise is experimenting with GenAI on a small scale or using off-the-shelf solutions.
Developing: The organization has integrated some GenAI tools into specific functions, such as marketing or customer service.
Advanced: GenAI is deeply embedded across multiple departments, and the organization is leveraging its full potential for strategic decision-making.
Transformational: GenAI has become a core part of the business, driving innovation, business model changes, and continuous optimization.
By identifying their stage on the maturity model, businesses can better plan their GenAI adoption strategy, ensuring that they achieve sustainable ROI over time.
5. GenAI Training Programs: Building a Competent Workforce
For enterprises to fully realize the potential of GenAI, it’s critical to invest in GenAI training programs. Educating employees on how to leverage AI tools not only improves the effectiveness of the solutions but also drives ROI through increased employee productivity and engagement.
Training programs can include courses on the ethical use of AI, developing AI-driven applications, and integrating AI into existing workflows. Additionally, by upskilling the workforce, enterprises can minimize resistance to AI adoption and foster a culture of innovation.
6. GenAI Insights: Real-Time Feedback for Continuous Improvement
One of the key advantages of GenAI is its ability to provide continuous insights and feedback. Unlike traditional business intelligence tools, which often require manual updates, GenAI can analyze data in real time, offering insights that can immediately inform decision-making.
For instance, real-time insights from GenAI can help identify underperforming areas in an organization, allowing leadership to take corrective action promptly. This ongoing cycle of analysis and improvement contributes to long-term ROI by continuously optimizing business operations.
7. Enterprise OpenAI: A Powerful Tool for Business Transformation
With solutions like Enterprise OpenAI, businesses can access cutting-edge AI models designed to solve complex problems. OpenAI’s tools offer capabilities such as natural language processing, machine learning, and advanced analytics, all of which can be tailored to the needs of specific industries.
By integrating OpenAI’s offerings into business operations, organizations can accelerate innovation, reduce operational costs, and drive better customer experiences—all of which contribute to higher ROI.
8. GenAI News: Staying Ahead of the Curve
The field of AI is evolving rapidly, and staying updated with GenAI news is essential for businesses aiming to maintain a competitive edge. Keeping up with the latest developments allows enterprises to adopt new technologies early, which can lead to cost savings and revenue generation opportunities.
By monitoring the latest trends and breakthroughs in GenAI, businesses can pivot quickly, adopt new tools, and ensure that they remain at the forefront of technological innovation.
Conclusion: The Path to Maximizing ROI with GenAI
In conclusion, the integration of Enterprise GenAI into business operations offers a wealth of opportunities for driving ROI. From enhancing efficiency to enabling data-driven decision-making and providing real-time insights, the potential benefits are vast. By investing in GenAI solutions, upskilling the workforce, and staying informed about the latest AI advancements, enterprises can harness the full potential of GenAI, creating long-term value and achieving sustainable profitability.
To succeed, businesses must approach GenAI adoption strategically, leveraging the right tools and solutions to meet their unique needs. Whether it's through Enterprise OpenAI, targeted GenAI training programs, or leveraging case studies and maturity models, the road to ROI is clear—GenAI is a powerful tool for transformation.
0 notes
Text
Use Intel Gaudi-3 Accelerators To Increase Your AI Skills
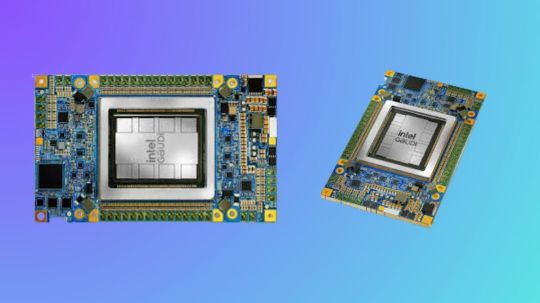
Boost Your Knowledge of AI with Intel Gaudi-3 Accelerators
Large language models (LLMs) and generative artificial intelligence (AI) are two areas in which Intel Gaudi Al accelerators are intended to improve the effectiveness and performance of deep learning workloads. Gaudi processors provide efficient solutions for demanding AI applications including large-scale model training and inference, making them a more affordable option than typical NVIDIA GPUs. Because Intel’s Gaudi architecture is specifically designed to accommodate the increasing computing demands of generative AI applications, businesses looking to implement scalable AI solutions will find it to be a highly competitive option. The main technological characteristics, software integration, and upcoming developments of the Gaudi AI accelerators are all covered in this webinar.
Intel Gaudi Al Accelerators Overview
The very resource-intensive generative AI applications, as LLM training and inference, are the focus of the Gaudi AI accelerator. While Intel Gaudi-3, which is anticipated to be released between 2024 and 2025, promises even more breakthroughs, Gaudi 2, the second-generation CPU, enables a variety of deep learning enhancements.
- Advertisement -
Intel Gaudi 2
The main attributes of Gaudi 2 consist of:
Matrix Multiplication Engine: Hardware specifically designed to process tensors efficiently.
For AI tasks, 24 Tensor Processor Cores offer high throughput.
Larger model and batch sizes are made possible for better performance by the 96 GB of on-board HBM2e memory.
24 on-chip 100 GbE ports offer low latency and high bandwidth communication, making it possible to scale applications over many accelerators.
7nm Process Technology: For deep learning tasks, the 7nm architecture guarantees excellent performance and power efficiency.
These characteristics, particularly the combination of integrated networking and high memory bandwidth, make Gaudi 2 an excellent choice for scalable AI activities like multi-node training of big models. With its specialized on-chip networking, Gaudi’s innovative design does away with the requirement for external network controllers, greatly cutting latency in comparison to competing systems.
Intel Gaudi Pytorch
Software Environment and Stack
With its extensive software package, Intel’s Gaudi platform is designed to interact easily with well-known AI frameworks like PyTorch. There are various essential components that make up this software stack:
Graph Compiler and Runtime: Generates executable graphs that are tailored for the Gaudi hardware using deep learning models.
Kernel Libraries: Reduce the requirement for manual optimizations by using pre-optimized libraries for deep learning operations.
PyTorch Bridge: Requires less code modification to run PyTorch models on Gaudi accelerators.
Complete Docker Support: By using pre-configured Docker images, users may quickly deploy models, which simplifies the environment setup process.
With a GPU migration toolset, Intel also offers comprehensive support for models coming from other platforms, like NVIDIA GPUs. With the use of this tool, model code can be automatically adjusted to work with Gaudi hardware, enabling developers to make the switch without having to completely rebuild their current infrastructure.
Open Platforms for Enterprise AI
Use Cases of Generative AI and Open Platforms for Enterprise AI
The Open Platform for Enterprise AI (OPEA) introduction is one of the webinar’s main highlights. “Enable businesses to develop and implement GenAI solutions powered by an open ecosystem that delivers on security, safety, scalability, cost efficiency, and agility” is the stated mission of OPEA. It is completely open source with open governance, and it was introduced in May 2024 under the Linux Foundation AI and Data umbrella.
It has attracted more than 40 industry partners and has members from system integrators, hardware manufacturers, software developers, and end users on its technical steering committee. With OPEA, businesses can create and implement scalable AI solutions in a variety of fields, ranging from chatbots and question-answering systems to more intricate multimodal models. The platform makes use of Gaudi’s hardware improvements to cut costs while improving performance. Among the important use cases are:
Visual Q&A: This is a model that uses the potent LLaVA model for vision-based reasoning to comprehend and respond to questions based on image input.
Large Language and Vision Assistant, or LLaVA, is a multimodal AI model that combines language and vision to carry out tasks including visual comprehension and reasoning. In essence, it aims to combine the advantages of vision models with LLMs to provide answers to queries pertaining to visual content, such as photographs.
Large language models, such as GPT or others, are the foundation of LLaVA, which expands their functionality by incorporating visual inputs. Typically, it blends the natural language generation and interpretation powers of big language models with image processing techniques (such those from CNNs or Vision Transformers). Compared to solely vision-based models, LLaVA is able to reason about images in addition to describing them thanks to this integration.
Retrieval-Augmented Generation (RAG) or ChatQnA is a cutting-edge architecture that combines a vector database and big language models to improve chatbot capabilities. By ensuring the model obtains and analyzes domain-specific data from the knowledge base and maintains correct and up-to-date responses, this strategy lessens hallucinations.
Microservices can be customized because to OPEA’s modular architecture, which lets users change out databases and models as needed. This adaptability is essential, particularly in quickly changing AI ecosystems where new models and tools are always being developed.
Intel Gaudi Roadmap
According to Intel’s Gaudi roadmap, Gaudi 2 and Intel Gaudi-3 offer notable performance gains. Among the significant developments are:
Doubling AI Compute: In order to handle the increasing complexity of models like LLMs, Intel Gaudi-3 will offer floating-point performance that is 2 times faster for FP8 and 4 times faster for BF16.
Enhanced Memory Bandwidth: Intel Gaudi-3 is equipped with 1.5 times the memory bandwidth of its predecessor, so that speed won’t be compromised when handling larger models.
Increased Network capacity: Intel Gaudi-3’s two times greater networking capacity will help to further eliminate bottlenecks in multi-node training scenarios, which makes it perfect for distributing workloads over big clusters.
Additionally, Gaudi AI IP and Intel’s GPU technology will be combined into a single GPU form factor in Intel’s forthcoming Falcon Shores architecture, which is anticipated to launch in 2025. As part of Intel’s ongoing effort to offer an alternative to conventional GPU-heavy environments, this hybrid architecture is expected to provide an even more potent foundation for deep learning.
Tools for Deployment and Development
Through the Intel Tiber Developer Cloud, which offers cloud-based instances of Gaudi 2 hardware, developers can utilize Gaudi accelerators. Users can test and implement models at large scale using this platform without having to make investments in on-premises infrastructure.
Starting with Gaudi accelerators is as simple as following these steps:
Docker Setup: First, users use pre-built images to build up Docker environments.
Microservices Deployment: End-to-end AI solutions, such chatbots or visual Q&A systems, can be deployed by users using tools like Docker Compose and Kubernetes.
Intel’s inherent support for monitoring tools, such as Prometheus and Grafana, enables users to manage resource utilization and performance throughout their AI pipelines.
In summary
Enterprises seeking an efficient way to scale AI workloads will find a compelling solution in Intel’s Gaudi CPUs, in conjunction with the extensive OPEA framework and software stack. With Gaudi 2’s impressive performance and Intel Gaudi-3‘s upcoming improvements, Intel is establishing itself as a formidable rival in the AI hardware market by offering a reasonably priced substitute for conventional GPU-based architectures. With OPEA’s open and modular design and wide ecosystem support, developers can quickly create and implement AI solutions that are customized to meet their unique requirements.
Read more on govindhtech.com
#IntelGaudi3Accelerators#AISkills#AIapplications#IntelGaudi3#Gaudi2#KernelLibraries#chatbots#LargeLanguage#VisionAssistant#LLaVA#intel#RetrievalAugmentedGeneration#RAG#news#Tools#IntelTiberDeveloperCloud#Prometheus#EnterpriseAI#GenerativeAI#technology#technews#govindhtech
0 notes
Text
Unlocking the Future: Corporate Buyers Guide to Enterprise Intelligence Applications and GAI World 2025

As businesses continue to evolve in this digital age, the demand for robust Enterprise AI solutions is higher than ever. Companies are on the lookout for ways to integrate GenAI into their operations to drive efficiency, innovation, and competitive advantage. With that in mind, we’re excited to introduce our upcoming Corporate Buyers Guide to Enterprise Intelligence Applications (EIA), which will serve as a valuable resource for organizations exploring the potential of Enterprise GenAI.
What to Expect from the Corporate Buyers Guide
Our Corporate Buyers Guide to LLMs is designed to demystify the landscape of Enterprise AI and provide actionable insights into how organizations can leverage GenAI technologies. We’ll explore key areas such as:
Understanding the WINS Framework: A highlight of the guide will be our WINS framework, which helps businesses determine where GenAI can add value. WINS stands for “What’s Important Now, and Scalable,” guiding organizations in aligning their GenAI initiatives with their strategic goals.
Case Studies and Real-World Applications: We’ll share compelling GenAI case studies that illustrate how companies across various industries are successfully implementing Enterprise GenAI solutions. These insights will not only inspire your organization but also provide practical examples of what works and what doesn’t.
The GenAI Maturity Model: Understanding your organization’s position within the GenAI maturity model is crucial. Our guide will help you assess your current capabilities and outline a roadmap for advancement. This way, businesses can confidently navigate their GenAI journey.
Latest Trends and Insights: Stay updated with the latest GenAI news and trends that are shaping the future of Enterprise OpenAI. We’ll cover emerging technologies, innovative solutions, and how they can be integrated into your business model.
Join Us at GAI World 2025
To dive deeper into these topics, we invite you to join us at our annual conference, GAI World 2025, taking place on September 29-30 in Boston. This two-day event will bring together industry leaders, innovators, and practitioners to discuss the latest advancements in Enterprise AI and GenAI solutions.
What You’ll Gain from GAI World 2025
Networking Opportunities: Connect with fellow professionals, potential partners, and thought leaders in the Enterprise AI space. Building relationships at GAI World can open doors for collaboration and growth.
Workshops and Panels: Participate in hands-on workshops and insightful panel discussions featuring experts in the field. Gain practical knowledge on implementing GenAI strategies tailored to your organization’s needs.
Exclusive Insights: Be the first to hear about groundbreaking research, new tools, and technologies that are redefining the landscape of Enterprise GenAI. Our sessions will feature presentations on cutting-edge GenAI insights that can help propel your business forward.
Why Focus on Fractional CAIO?
In today’s fast-paced environment, many organizations are opting for a fractional CAIO (Chief AI Officer) to guide their Enterprise AI strategies. This approach allows businesses to access high-level expertise without the commitment of a full-time executive. At GAI World, you’ll learn how to effectively integrate a fractional CAIO into your strategy, maximizing your investment in GenAI technologies.
Conclusion
As we approach GAI World 2025, we’re thrilled to share our Corporate Buyers Guide to Enterprise Intelligence Applications with you. This guide, combined with the insights and connections you’ll gain at the conference, will empower your organization to harness the full potential of Enterprise GenAI.
0 notes