#Customer Data Analysis
Explore tagged Tumblr posts
Text
3 Ways to Boost Sales with Artificial Intelligence
Delivering Hyper-Targeted Ecommerce Experiences The era of generic, one-size-fits-all ecommerce experiences is fading fast. In its place, we’re witnessing the rise of hyper-personalization, driven by the power of Artificial Intelligence (AI). Gone are the days of basic product recommendations. Today, AI algorithms can analyze vast amounts of customer data – browsing history, purchase history,…
#AI#AI-driven content personalization#Artificial Intelligence#Customer data analysis#Customer needs anticipation#Dynamic content adjustments#Ecommerce experiences#Inventory optimization#Personalized marketing messages#Predictive Analytics#Product recommendations
0 notes
Text
AI and Conversion Rate Optimization: Driving Sales in the Digital Landscape
In the rapidly evolving digital landscape, businesses strive to optimize their conversion rates and drive sales. With the advent of artificial intelligence (AI), marketers now have a powerful ally in their pursuit of effective conversion rate optimization (CRO) strategies. AI-powered tools and techniques have transformed the way businesses analyze customer behavior, personalize user experiences,…
View On WordPress
#AI#AI in digital marketing#AI-Powered Chatbots#Customer Data Analysis#Digital Marketing#Predictive Analytics#sales
0 notes
Text
This is a shameless pitch for my field of work but if you like biology and you like coding...consider bioinformatics as a career 👀 Especially if you live in the US, as it's well-known for its bionformatics scene.
#musings#bioinformatics#stem#computer science#python#biology#i was just thinking about how not a lot of people know that a career like bioinformatics exists#hence my little post#most people i tell my job too just look at me confused like they didnt realize you could mix these fields#and a lot of people studying biology forget about how important it is to have a quantitative skill like math or computer science or physics#simply because the programs dont teach those skills#to do any sort of custom data analysis its important to have quantitative skills#and if you're passionate about genomics especially...and dna and the genome...then this may be the field for you!#good money especially in the states#of course a graduate degree is needed#masters minimum phd preferred#i have a masters
57 notes
·
View notes
Text
What Are the Qualifications for a Data Scientist?
In today's data-driven world, the role of a data scientist has become one of the most coveted career paths. With businesses relying on data for decision-making, understanding customer behavior, and improving products, the demand for skilled professionals who can analyze, interpret, and extract value from data is at an all-time high. If you're wondering what qualifications are needed to become a successful data scientist, how DataCouncil can help you get there, and why a data science course in Pune is a great option, this blog has the answers.
The Key Qualifications for a Data Scientist
To succeed as a data scientist, a mix of technical skills, education, and hands-on experience is essential. Here are the core qualifications required:
1. Educational Background
A strong foundation in mathematics, statistics, or computer science is typically expected. Most data scientists hold at least a bachelor’s degree in one of these fields, with many pursuing higher education such as a master's or a Ph.D. A data science course in Pune with DataCouncil can bridge this gap, offering the academic and practical knowledge required for a strong start in the industry.
2. Proficiency in Programming Languages
Programming is at the heart of data science. You need to be comfortable with languages like Python, R, and SQL, which are widely used for data analysis, machine learning, and database management. A comprehensive data science course in Pune will teach these programming skills from scratch, ensuring you become proficient in coding for data science tasks.
3. Understanding of Machine Learning
Data scientists must have a solid grasp of machine learning techniques and algorithms such as regression, clustering, and decision trees. By enrolling in a DataCouncil course, you'll learn how to implement machine learning models to analyze data and make predictions, an essential qualification for landing a data science job.
4. Data Wrangling Skills
Raw data is often messy and unstructured, and a good data scientist needs to be adept at cleaning and processing data before it can be analyzed. DataCouncil's data science course in Pune includes practical training in tools like Pandas and Numpy for effective data wrangling, helping you develop a strong skill set in this critical area.
5. Statistical Knowledge
Statistical analysis forms the backbone of data science. Knowledge of probability, hypothesis testing, and statistical modeling allows data scientists to draw meaningful insights from data. A structured data science course in Pune offers the theoretical and practical aspects of statistics required to excel.
6. Communication and Data Visualization Skills
Being able to explain your findings in a clear and concise manner is crucial. Data scientists often need to communicate with non-technical stakeholders, making tools like Tableau, Power BI, and Matplotlib essential for creating insightful visualizations. DataCouncil’s data science course in Pune includes modules on data visualization, which can help you present data in a way that’s easy to understand.
7. Domain Knowledge
Apart from technical skills, understanding the industry you work in is a major asset. Whether it’s healthcare, finance, or e-commerce, knowing how data applies within your industry will set you apart from the competition. DataCouncil's data science course in Pune is designed to offer case studies from multiple industries, helping students gain domain-specific insights.
Why Choose DataCouncil for a Data Science Course in Pune?
If you're looking to build a successful career as a data scientist, enrolling in a data science course in Pune with DataCouncil can be your first step toward reaching your goals. Here’s why DataCouncil is the ideal choice:
Comprehensive Curriculum: The course covers everything from the basics of data science to advanced machine learning techniques.
Hands-On Projects: You'll work on real-world projects that mimic the challenges faced by data scientists in various industries.
Experienced Faculty: Learn from industry professionals who have years of experience in data science and analytics.
100% Placement Support: DataCouncil provides job assistance to help you land a data science job in Pune or anywhere else, making it a great investment in your future.
Flexible Learning Options: With both weekday and weekend batches, DataCouncil ensures that you can learn at your own pace without compromising your current commitments.
Conclusion
Becoming a data scientist requires a combination of technical expertise, analytical skills, and industry knowledge. By enrolling in a data science course in Pune with DataCouncil, you can gain all the qualifications you need to thrive in this exciting field. Whether you're a fresher looking to start your career or a professional wanting to upskill, this course will equip you with the knowledge, skills, and practical experience to succeed as a data scientist.
Explore DataCouncil’s offerings today and take the first step toward unlocking a rewarding career in data science! Looking for the best data science course in Pune? DataCouncil offers comprehensive data science classes in Pune, designed to equip you with the skills to excel in this booming field. Our data science course in Pune covers everything from data analysis to machine learning, with competitive data science course fees in Pune. We provide job-oriented programs, making us the best institute for data science in Pune with placement support. Explore online data science training in Pune and take your career to new heights!
#In today's data-driven world#the role of a data scientist has become one of the most coveted career paths. With businesses relying on data for decision-making#understanding customer behavior#and improving products#the demand for skilled professionals who can analyze#interpret#and extract value from data is at an all-time high. If you're wondering what qualifications are needed to become a successful data scientis#how DataCouncil can help you get there#and why a data science course in Pune is a great option#this blog has the answers.#The Key Qualifications for a Data Scientist#To succeed as a data scientist#a mix of technical skills#education#and hands-on experience is essential. Here are the core qualifications required:#1. Educational Background#A strong foundation in mathematics#statistics#or computer science is typically expected. Most data scientists hold at least a bachelor’s degree in one of these fields#with many pursuing higher education such as a master's or a Ph.D. A data science course in Pune with DataCouncil can bridge this gap#offering the academic and practical knowledge required for a strong start in the industry.#2. Proficiency in Programming Languages#Programming is at the heart of data science. You need to be comfortable with languages like Python#R#and SQL#which are widely used for data analysis#machine learning#and database management. A comprehensive data science course in Pune will teach these programming skills from scratch#ensuring you become proficient in coding for data science tasks.#3. Understanding of Machine Learning
3 notes
·
View notes
Text
Beyond Chain-of-Thought: How Thought Preference Optimization is Advancing LLMs
New Post has been published on https://thedigitalinsider.com/beyond-chain-of-thought-how-thought-preference-optimization-is-advancing-llms/
Beyond Chain-of-Thought: How Thought Preference Optimization is Advancing LLMs
A groundbreaking new technique, developed by a team of researchers from Meta, UC Berkeley, and NYU, promises to enhance how AI systems approach general tasks. Known as “Thought Preference Optimization” (TPO), this method aims to make large language models (LLMs) more thoughtful and deliberate in their responses.
The collaborative effort behind TPO brings together expertise from some of the leading institutions in AI research.
The Mechanics of Thought Preference Optimization
At its core, TPO works by encouraging AI models to generate “thought steps” before producing a final answer. This process mimics human cognitive processes, where we often think through a problem or question before articulating our response.
The technique involves several key steps:
The model is prompted to generate thought steps before answering a query.
Multiple outputs are created, each with its own set of thought steps and final answer.
An evaluator model assesses only the final answers, not the thought steps themselves.
The model is then trained through preference optimization based on these evaluations.
This approach differs significantly from previous techniques, such as Chain-of-Thought (CoT) prompting. While CoT has been primarily used for math and logic tasks, TPO is designed to have broader utility across various types of queries and instructions. Furthermore, TPO doesn’t require explicit supervision of the thought process, allowing the model to develop its own effective thinking strategies.
Another key difference is that TPO overcomes the challenge of limited training data containing human thought processes. By focusing the evaluation on the final output rather than the intermediate steps, TPO allows for more flexible and diverse thinking patterns to emerge.
Experimental Setup and Results
To test the effectiveness of TPO, the researchers conducted experiments using two prominent benchmarks in the field of AI language models: AlpacaEval and Arena-Hard. These benchmarks are designed to evaluate the general instruction-following capabilities of AI models across a wide range of tasks.
The experiments used Llama-3-8B-Instruct as a seed model, with different judge models employed for evaluation. This setup allowed the researchers to compare the performance of TPO against baseline models and assess its impact on various types of tasks.
The results of these experiments were promising, showing improvements in several categories:
Reasoning and problem-solving: As expected, TPO showed gains in tasks requiring logical thinking and analysis.
General knowledge: Interestingly, the technique also improved performance on queries related to broad, factual information.
Marketing: Perhaps surprisingly, TPO demonstrated enhanced capabilities in tasks related to marketing and sales.
Creative tasks: The researchers noted potential benefits in areas such as creative writing, suggesting that “thinking” can aid in planning and structuring creative outputs.
These improvements were not limited to traditionally reasoning-heavy tasks, indicating that TPO has the potential to enhance AI performance across a broad spectrum of applications. The win rates on AlpacaEval and Arena-Hard benchmarks showed significant improvements over baseline models, with TPO achieving competitive results even when compared to much larger language models.
However, it’s important to note that the current implementation of TPO showed some limitations, particularly in mathematical tasks. The researchers observed that performance on math problems actually declined compared to the baseline model, suggesting that further refinement may be necessary to address specific domains.
Implications for AI Development
The success of TPO in improving performance across various categories opens up exciting possibilities for AI applications. Beyond traditional reasoning and problem-solving tasks, this technique could enhance AI capabilities in creative writing, language translation, and content generation. By allowing AI to “think” through complex processes before generating output, we could see more nuanced and context-aware results in these fields.
In customer service, TPO could lead to more thoughtful and comprehensive responses from chatbots and virtual assistants, potentially improving user satisfaction and reducing the need for human intervention. Additionally, in the realm of data analysis, this approach might enable AI to consider multiple perspectives and potential correlations before drawing conclusions from complex datasets, leading to more insightful and reliable analyses.
Despite its promising results, TPO faces several challenges in its current form. The observed decline in math-related tasks suggests that the technique may not be universally beneficial across all domains. This limitation highlights the need for domain-specific refinements to the TPO approach.
Another significant challenge is the potential increase in computational overhead. The process of generating and evaluating multiple thought paths could potentially increase processing time and resource requirements, which may limit TPO’s applicability in scenarios where rapid responses are crucial.
Furthermore, the current study focused on a specific model size, raising questions about how well TPO will scale to larger or smaller language models. There’s also the risk of “overthinking” – excessive “thinking” could lead to convoluted or overly complex responses for simple tasks.
Balancing the depth of thought with the complexity of the task at hand will be a key area for future research and development.
Future Directions
One key area for future research is developing methods to control the length and depth of the AI’s thought processes. This could involve dynamic adjustment, allowing the model to adapt its thinking depth based on the complexity of the task at hand. Researchers might also explore user-defined parameters, enabling users to specify the desired level of thinking for different applications.
Efficiency optimization will be crucial in this area. Developing algorithms to find the sweet spot between thorough consideration and rapid response times could significantly enhance the practical applicability of TPO across various domains and use cases.
As AI models continue to grow in size and capability, exploring how TPO scales with model size will be crucial. Future research directions may include:
Testing TPO on state-of-the-art large language models to assess its impact on more advanced AI systems
Investigating whether larger models require different approaches to thought generation and evaluation
Exploring the potential for TPO to bridge the performance gap between smaller and larger models, potentially making more efficient use of computational resources
This research could lead to more sophisticated AI systems that can handle increasingly complex tasks while maintaining efficiency and accuracy.
The Bottom Line
Thought Preference Optimization represents a significant step forward in enhancing the capabilities of large language models. By encouraging AI systems to “think before they speak,” TPO has demonstrated improvements across a wide range of tasks, potentially revolutionizing how we approach AI development.
As research in this area continues, we can expect to see further refinements to the technique, addressing current limitations and expanding its applications. The future of AI may well involve systems that not only process information but also engage in more human-like cognitive processes, leading to more nuanced, context-aware, and ultimately more useful artificial intelligence.
#ai#AI development#AI models#AI research#AI systems#Algorithms#analyses#Analysis#applications#approach#arena#Art#artificial#Artificial Intelligence#benchmarks#bridge#chain of thought reasoning#challenge#chatbots#collaborative#complexity#comprehensive#content#customer service#data#data analysis#datasets#development#domains#efficiency
3 notes
·
View notes
Text
The Transformative Benefits of Artificial Intelligence
Title: The Transformative Benefits of Artificial Intelligence Artificial Intelligence (AI) has emerged as one of the most revolutionary technologies of the 21st century. It involves creating intelligent machines that can mimic human cognitive functions such as learning, reasoning, problem-solving, and decision-making. As AI continues to advance, its impact is felt across various industries and…
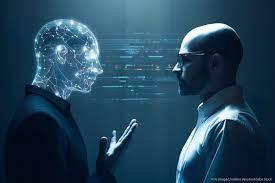
View On WordPress
#Advancements in Education#AI Advantages#AI Benefits#artificial intelligence#Customer Experience#Data Analysis#Data Analytics#Decision-Making#Efficiency and Productivity#Energy Management#Ethical AI Deployment.#Healthcare Transformation#Machine Learning#Personalized Learning#Personalized User Experiences#Robotics in Healthcare#Smart Cities#Smart Technology#Smart Traffic Management#Sustainable Development
2 notes
·
View notes
Text
The need for campaign customer data platforms has been fueled by the ability to gather customer data points from various sources, segment customers according to a wide range of criteria, and provide real-time insights to carry out customized marketing.
#Customer Data Platform Market#Customer Data Platform Market size#Customer Data Platform Market growth#Customer Data Platform Market share#Customer Data Platform Market analysis#Customer Data Platform Market demand
0 notes
Text
Wawasan Pelanggan Berbasis AI untuk Pemasaran yang Tepat Sasaran
Di era digital yang semakin kompleks, memahami pelanggan menjadi kunci keberhasilan pemasaran. Perusahaan yang dapat mengidentifikasi kebutuhan, preferensi, dan perilaku pelanggan memiliki keunggulan kompetitif yang signifikan. Namun, dengan jumlah data yang terus bertambah, menganalisis informasi secara manual menjadi tantangan besar. Di sinilah kecerdasan buatan (AI) memainkan peran penting. AI…
#AI in small businesses#AI marketing#AI-driven campaigns#customer insights#customer loyalty#data ethics#future of AI marketing#hyper-personalization#machine learning tools#marketing automation#omnichannel marketing#personalized marketing#predictive analytics#ROI improvement#sentiment analysis
0 notes
Text
Academic writing services in Dubai:-
Looking for expert review writing in Dubai? At Writers.ae, we offer top-notch data collection & analysis services to provide you with high-quality content. Our talented content writers in Dubai ensure that your requirements are met with precision and professionalism. Trust Writers.ae for all your content writing needs. https://www.writers.ae/Research_Paper_Writing_Services_in_UAE_Dubai_Abu_Dhabi_UK_Australia_HongKong_India_Singapore.aspx
#Ghost writers in Dubai#Content writers near me#Customer handbook writers Dubai#Data collection & analysis services in Dubai#Paramedic report writing#Thesis writer in Dubai
0 notes
Text
AI’s Honeymoon Phase Is Over, So What Comes Next?
New Post has been published on https://thedigitalinsider.com/ais-honeymoon-phase-is-over-so-what-comes-next/
AI’s Honeymoon Phase Is Over, So What Comes Next?


Countless discussions about AI’s transformative potential have taken place over the past two years since ChatGPT’s initial release generated so much excitement. Corporate leaders have been eager to use the technology to reduce operational expenses. Perhaps surprising, though, is that for many leaders, the key metric used to evaluate the success of an AI tool is not the lifetime return on investment (ROI). It’s the speed to ROI.
Amid shrinking risk tolerance and increased revenue pressure, leaders expect investments to drive changes and pay off quickly. At the same time, the hype around AI is dying down, making way for more pragmatic conversations around the return on AI investments.
The Next Phase: Getting Real About Where AI Works
Success in today’s market—where subscriptions are king—relies on how well you keep customers, not how well you acquire them. In most sectors, the market is oversaturated, and many organizations offer similar services of near-identical quality. Add in a decline in customer loyalty, rising expectations and an increased willingness to switch brands, and organizations find themselves with no room for error to keep up with fierce competition. Customer experience (CX) is the factor that determines whether subscription-based organizations thrive or fall short.
In this environment, organizations can compete best by leaning into incremental improvements rather than away from spending. Each and every choice the organization makes must be oriented toward specific, customer-centric goals — even if it costs a bit more at the start. That extends to AI implementation. Organizations have been asking how AI can recoup its cost by using it as a replacement for existing resources. Now, they need to ask how AI can create value for the organization by improving how they work with customers.
The answer is straightforward enough. AI has numerous potential applications that improve CX both directly and indirectly. AI-powered tools can enhance personalization by using customer behavior data to ensure the users see the right message or promotion at the right time. The same data can help guide product development, highlighting gaps in the market that the organization might capitalize on to better serve customers’ needs. They can also make organizations more proactive, helping them anticipate disruptions, activate contingency plans and communicate necessary information to users.
However, this work happens primarily behind the scenes, and it cannot happen overnight.
Want AI at Its Best? Start With ‘Invisible’ Applications
The only way to know for certain whether a back- or front-end use case will yield the results you’re after is to leverage AI’s more discreet, behind-the-scenes capabilities first.
Behind the headlines about instant transformation is AI’s core capability: analysis. Large language models (LLMs) like ChatGPT turned heads for their apparent flexibility, but they perform only one task no matter where they operate. They summarize information. It’s on organizations to make the right information available, and that takes time. Those are two facts that have often been lost in the conversation, and they represent an end to the “quick fix” reputation AI has come to enjoy.
The next era will be defined by the invisible improvements facilitated by AI as organizations build up their technical foundations. Organizations can start with LLMs that help:
Integrate existing databases and break down silos to provide end-to-end visibility – and the context that comes with it.
Implement real-time data collection tools to ensure insights are up to date and reflect the most recent trends, patterns and disruptions.
Expedite reconciliation and management to ensure accuracy and free up workers to focus on higher-level tasks that require a human touch.
Organizational change is the first step to effective implementation and extends to both systems and staff. At this point, leaders should also consider the ways AI deployments might affect staff and work to get ahead of potential obstacles. Developing upskilling and reskilling programs will help ensure staff is ready to work effectively alongside the new technologies. AI itself can help in these efforts—another of its invisible applications. For example, it can highlight individual knowledge gaps based on utilization data. This kind of information can guide training programs to make sure workers have everything they need to thrive.
Once organizations have integrated, accurate and up-to-date records and a staff that understands how and when to use AI, they can add another layer of “invisible” tools. The next wave of solutions should focus on analytics that help cultivate a deep understanding of how the business runs, what customers want and obstacles getting in the way. These solutions build on one another, with each step revealing a new level of insight.
More specifically, descriptive analytics use historical data to identify historical patterns; they tell organizations what happened. Diagnostic analytics use additional data to contextualize what happened, identify causes and highlight the effects of incidents and changes; they tell organizations why things happened the way they did. Predictive analytics use insights from past events to model the impacts of proposed changes and keep tabs on trends; they show organizations what might happen. Prescriptive analytics use all of these outputs to make informed decisions; they tell organizations what to do next.
Though analytics solutions like these may tap into AI’s more advanced capabilities, it’s worth noting that—at first—nearly all these processes happen behind the scenes. Eventually, predictive and prescriptive algorithms may make their way into consumer-facing solutions, but that can only happen once this critical, internal foundation is laid.
As AI’s honeymoon ends, so too will its reputation as a magic fix—but shedding this perception is critical to realizing the technology’s full potential. Leaders who want to make headlines tomorrow with innovative AI applications must first complete this foundational work, which may be a hard pill to swallow amid pressure for faster and faster returns. However, moving toward more holistic, incremental and long-term assessments of AI’s value will enable organizations to expedite returns. This approach gives leaders the tools and time to develop a clear picture of what needs to be fixed, insight into the small changes that will have the biggest impacts and the ability to develop sound strategies that yield returns today without damaging profitability tomorrow.
Pragmatism from End-to-End
Though flashy use cases may entice customers at first glance, and cost-cutting opportunities might catch the eye of corporate leaders, neither is likely to define AI’s impact in the long run. Instead, the technology will become synonymous with behind-the-scenes work that drives tangible improvement at scale.
The end of the honeymoon phase marks the beginning of a more mature relationship with AI, one that requires careful consideration of how it can genuinely enhance customer experiences and drive profitability. Ultimately, the key is to view AI not as a quick fix but as a strategic partner in the pursuit of customer loyalty, satisfying experiences and simple solutions in today’s increasingly complex operations.
In the coming months and years, the organizations that excel will be those that dig deeper, commit to change and recognize AI’s potential as both a short- and long-term investment.
#ADD#ai#AI-powered#Algorithms#Analysis#Analytics#applications#approach#Behavior#behavior data#brands#Business#change#chatGPT#competition#CSG#customer experience#customer experiences#customer loyalty#cutting#data#data collection#databases#development#effects#Environment#Events#excel#eye#factor
0 notes
Text
Predictive Customer Analytics: A Complete Guide

Stabilizing your business in today’s volatile market condition is a feat in itself. Predictive customer analytics helps you thrive by solving a major problem: changing customer preferences.
Every business desires sales and growth. With the rapidly fluctuating economy and booming technology landscape, businesses have to be like water for sustainable growth. The key to achieving that is predicting what the customer wants next and adapting to changing demand. Predictive analytics is an emerging solution for solving these problems and gaining a competitive edge.
This article is a deep dive into predictive customer analytics and how you can leverage it to stabilize your business in the current volatile market scenario.
Understanding Predictive Customer Analytics
Predictive Customer Analytics is a data-driven approach that leverages historical data and machine learning techniques to forecast future customer behavior. What separates this from traditional business intelligence is that it not only analyses past trends but goes a step further by actively predicting future outcomes.
In essence, predictive analytics empowers businesses to move beyond reactive responses and embrace a proactive, customer-centric approach.
The Nuts and Bolts
Let’s explore how predictive customer analytics works. It follows a systematic approach involving multiple key steps:
1: Data Collection
Predictive models depend on a robust foundation of data from various sources. This includes CRM, POS, website analytics, social media, user feedback, and other discrete sources.
2: Data Cleaning
The collected data often requires significant preprocessing. Data engineers clean it by identifying and correcting inconsistencies and removing duplicates. They convert it to suitable formats for analysis and create new variables from existing data to improve model accuracy.
3: Model Training
Data scientists employ various statistical and machine learning algorithms, including:
Regression analysis: Predicting continuous outcomes (e.g., CLTV).
Classification algorithms: Predicting categorical outcomes (e.g., churn or fraud).
Clustering algorithms: Grouping customers into segments.
Time series analysis: Forecasting future trends based on historical data.
4: Evaluation and Validation
The trained model is rigorously tested to assess its reliability. Common evaluation metrics include accuracy, recall, precision, and F1 score. Techniques like cross-validation are used to ensure the model’s ability to generalize well to new, unseen data. This helps prevent overfitting, where the model performs well on the training data but poorly on real-world data.
5: Deployment
Once validated, the model is deployed into a production environment to make real-time predictions. The model is monitored and regularly retrained with updated data for peak performance.
Benefits of Predictive Analytics In Customer Experience
Predictive customer analytics empowers businesses to make smarter decisions based on pure data instead of gut feelings. Currently, over 40% of organizations are leveraging data analytics for decision-making and reaping the benefits. Let’s explore some real-world use cases of how you can use predictive analytics to drive growth.
1: Personalized Customer Journeys
You can create highly personalized customer journeys by analyzing customer data to understand individual customer preferences and behaviors. For example, a retail company can recommend the exact product a customer wants next based on their browsing history and past purchases.
2: Enhanced Customer Retention
Predictive customer analytics can effectively identify customers at high risk of churn. By analyzing factors like purchase frequency, customer service interactions, and social media sentiment, businesses can proactively reach out to at-risk customers with targeted retention offers.
3: Improved Customer Service
You can anticipate customer needs and proactively address potential issues. Chatbots powered by PCA can provide instant, personalized support to customers by understanding their queries and offering relevant solutions.
4: Better Customer Engagement
Predictive analytics helps to increase customer engagement by personalizing your messaging. For example, you can know the best time and channel for messaging to optimize your marketing efforts.
5: Enhanced Customer Loyalty
Predictive analytics helps you provide exceptional customer service, which in turn helps increase customer lifetime value. You can foster long-term relationships by being proactive with your support and personalizing offers.
Top 15 Use Cases of Predictive Customer Analytics
Now that you know what predictive customer analytics is and how it works, let’s explore 15 use cases for your business.
1: Churn Prediction
AI-driven predictive analytics can tell you who’s about to leave before they start packing their bags. It predicts customer churn based on behaviors like canceling subscriptions or brand switching. This empowers you to implement proactive retention strategies like offering incentives and providing personalized support.
2: CLV Prediction
Knowing which customer will reel in the maximum profits is critical for optimizing marketing resources and efforts. Predictive analytics help identify customers with the highest potential lifetime value so you can allocate resources effectively and tailor customer experiences.
3: Customer Segmentation
You can group customers with similar characteristics and behaviors. This step is highly beneficial for developing and executing targeted marketing campaigns for each segment. Your marketing team can send personalized messages and offers based on segment preferences.
4: Hyperpersonalization
AI recommends the most relevant products or services by predicting what the customer wants next. You can extract a plethora of unforeseen opportunities to upsell and cross-sell products to your customers. AI will tell you everything, from the best time to the marketing channel for the same.
5: Fraud Detection
AI monitors websites and applications in real time to detect credit card fraud, phishing, account takeover, insurance claims, and more. It sends instant alerts, allowing your team to take proactive steps to safeguard your business and customers. Minimize financial loss due to fraudulent activities.
Read full article here: Use Cases of Predictive Customer Analytics
#data analytics#business intelligence and analytics software#ai data analytics tool#ai for business#ai data analysis#Predictive Customer Analytics
0 notes
Text
#R Programming Assignment Help#R Programming Homework Help#Expert Help with R Programming Assignments#Online R Programming Homework Solutions#Custom R Programming Assignment Assistance#R Programming Data Analysis Help#Professional R Programming Tutors Online#Help with R Programming Projects#Affordable R Programming Assignment Support#R Programming Statistical Analysis Help#R Coding Assignment Help#Debugging R Programming Homework#Advanced R Programming Solutions#Machine Learning with R Assignment Help#R Programming Assistance for Students
0 notes
Text
The Role of AI in Shaping Modern Business Practices
Artificial Intelligence (AI) is at the forefront of technological advancements impacting businesses today. Its ability to analyze vast amounts of data quickly and accurately has made it an invaluable tool for organizations looking to enhance their operations.
AI-driven solutions are revolutionizing customer service through chatbots and virtual assistants that provide instant support to customers around the clock. This not only improves customer satisfaction but also reduces operational costs by minimizing the need for extensive human intervention.
In addition, AI enhances decision-making processes by providing predictive analytics that help businesses anticipate market trends and consumer behavior. With these insights, companies can develop strategies that align with customer needs and preferences.
Moreover, AI streamlines operational efficiency by optimizing supply chain management and automating routine tasks. This allows businesses to allocate resources more effectively and respond swiftly to changes in demand.
As AI technology continues to evolve, its integration into business practices will become even more profound, driving innovation and growth across various industries.
#artificial intelligence#ai#customer service#predictive analytics#business innovation#data analysis#chatbots#virtual assistants#market trends#decision making#ai solutions#industry insights#customer experience#technology
0 notes
Text
Google Forms क्या है और इसका कैसे प्रयोग करे [Step by Step]
Google Forms एक उपयोगी और आसान टूल है जो गूगल द्वारा प्रदान किया गया है, जिससे हम ऑनलाइन सर्वे, क्विज़, फीडबैक, रजिस्ट्रेशन फॉर्म्स आदि बना सकते हैं। इस टूल की मदद से हम आसानी से किसी भी प्रकार का डेटा इकट्ठा कर सकते हैं और उसे विश्लेषित (analyze) कर सकते हैं। Google Forms का सबसे बड़ा फायदा यह है कि यह मुफ़्त और पूरी तरह से कस्टमाइज़ेबल है, जिससे छोटे-बड़े सभी प्रकार के बिजनेस और व्यक्तिगत…
#benefits of Google Forms#create Google Forms#Google Forms automation#Google Forms customization#Google Forms data analysis#Google Forms examples#Google Forms features#Google Forms for business#Google Forms for feedback#Google Forms for surveys#Google Forms guide#Google Forms integration#Google Forms mobile friendly#Google Forms quiz#Google Forms security#Google Forms templates#Google Forms tips#Google Forms tutorial#how to use Google Forms#online forms with Google
0 notes
Text
Jayson Murphy IT service
Website: http://jaysonmurphyitservicer.com/
Address: 609 New York Ave, Brooklyn, NY 11203, USA
Phone: 917-577-3337
Jayson Murphy IT Service is a comprehensive provider of managed IT solutions tailored to meet the unique needs of businesses. With a focus on enhancing operational efficiency and ensuring robust cybersecurity, we offer a range of services including network management, cloud solutions, data backup, and IT consulting. Our team of experienced professionals is dedicated to delivering reliable support and innovative technology strategies that empower organizations to thrive in a digital landscape. At Jayson Murphy IT Service, we prioritize customer satisfaction and work closely with our clients to develop customized solutions that drive growth and success.
Business Email: [email protected]
Facebook: https://facebook.com/abdulmanufacturerlimited
Twitter: https://twitter.com/abdulmanufacturerlimited
Instagram: https://instagram.com/abdulmanufacturerlimited
TikTok: https://tiktok.com/@abdulmanufacturerl
#IT services#Managed IT services#IT support#IT consulting#Technology solutions#IT staff training#Cybersecurity training#Software training workshops#Technology bootcamps#IT certification programs#Custom software development#E-commerce solutions#CRM implementation#Workflow automation solutions#Digital transformation services#IT performance monitoring#Network monitoring tools#Application performance analysis#IT health checks#Data analytics services#Brooklyn NY IT services#IT support in Brooklyn NY#Managed IT services Brooklyn NY#Cybersecurity Brooklyn NY#IT consulting Brooklyn NY#Remote work solutions#Team collaboration tools#Video conferencing solutions#Unified communication systems#Project management software
1 note
·
View note