#opensourceAI
Explore tagged Tumblr posts
Text
Step into the future with Llama 3.1, the latest iteration in open-source large language models by Meta AI. With its high parameter count (405B) and multilingual capabilities, it’s redefining what’s possible in the world of AI.
#Llama3.1#OpenSourceAI#LargeLanguageModel#ArtificialIntelligence#DeepLearning#MachineLearning#NLP#artificial intelligence#open source#machine learning#programming#software engineering#opensource#python
2 notes
·
View notes
Link
DeepSeek, a relatively unknown Chinese AI startup, has emerged as a formidable competitor to U.S. tech giants like OpenAI, Meta, and Google.
1 note
·
View note
Link
#ai#AIDevelopments#AIInnovations#aimodels#ainews#ainewsthisweek#AITools#aiuncovered#aivideo#aiupdates#artificialintelligence#ByteDanceX-Portrait2#ByteDance'sX-Portrait2#DeepLearning#MachineLearning#newaitools#NvidiaNemotron#openai#opensourceai#Replit#ReplitUpdates#RunwayML#TechNews#technologyupdates#TopAINewsThisWeek#WeeklyAINews
0 notes
Text
How Open Source AI Works? Its Advantages And Drawbacks
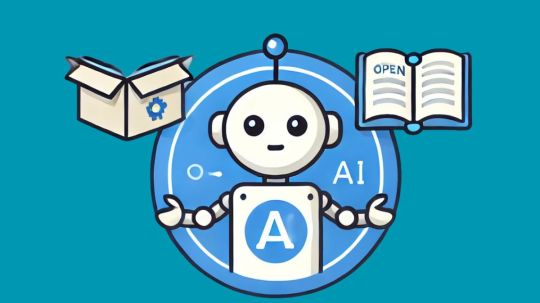
What Is Open-source AI?
Open source AI refers to publicly available AI frameworks, methodologies, and technology. Everyone may view, modify, and share the source code, encouraging innovation and cooperation. Openness has sped AI progress by enabling academics, developers, and companies to build on each other’s work and create powerful AI tools and applications for everyone.
Open Source AI projects include:
Deep learning and neural network frameworks PyTorch and TensorFlow.
Hugging Face Transformers: Language translation and chatbot NLP libraries.
OpenCV: A computer vision toolbox for processing images and videos.
Through openness and community-driven standards, open-source AI increases accessibility to technology while promoting ethical development.
How Open Source AI Works
The way open-source AI operates is by giving anybody unrestricted access to the underlying code of AI tools and frameworks.
Community Contributions
Communities of engineers, academics, and fans create open-source AI projects like TensorFlow or PyTorch. They add functionality, find and solve errors, and contribute code. In order to enhance the program, many people labor individually, while others are from major IT corporations, academic institutions, and research centers.
Access to Source Code
Open Source AI technologies’ source code is made available on websites such as GitHub. All the instructions needed for others to replicate, alter, and comprehend the AI’s operation are included in this code. The code’s usage is governed by open-source licenses (such MIT, Apache, or GPL), which provide rights and restrictions to guarantee equitable and unrestricted distribution.
Building and Customizing AI Models
The code may be downloaded and used “as-is,” or users can alter it to suit their own requirements. Because developers may create bespoke AI models on top of pre-existing frameworks, this flexibility permits experimentation. For example, a researcher may tweak a computer vision model to increase accuracy for medical imaging, or a business could alter an open-source chatbot model to better suit its customer service requirements.
Auditing and Transparency
Because anybody may examine the code for open source AI, possible biases, flaws, and mistakes in AI algorithms can be found and fixed more rapidly. Because it enables peer review and community-driven changes, this openness is particularly crucial for guaranteeing ethical AI activities.
Deployment and Integration
Applications ranging from major business systems to mobile apps may be linked with open-source AI technologies. Many tools are accessible to a broad range of skill levels because they provide documentation and tutorials. Open-source AI frameworks are often supported by cloud services, allowing users to easily expand their models or incorporate them into intricate systems.
Continuous Improvement
Open-source AI technologies allow users to test, improve, update, and fix errors before sharing the findings with the community. Open Source AI democratizes cutting-edge AI technology via cross-sector research and collaboration.
Advantages Of Open-Source AI
Research and Cooperation: Open-source AI promotes international cooperation between organizations, developers, and academics. They lessen effort duplication and speed up AI development by sharing their work.
Transparency and Trust: Open source AI promotes better trust by enabling people to examine and comprehend how algorithms operate. Transparency ensures AI solutions are morally and fairly sound by assisting in the detection of biases or defects.
Startups: Smaller firms, and educational institutions that cannot afford proprietary solutions may employ open-source AI since it is typically free or cheap.
Developers: May customize open-source AI models to meet specific needs, improving flexibility in healthcare and finance. Open Source AI allows students, developers, and data scientists to explore, improve, and participate in projects.
Open-Source AI Security and Privacy issues: Unvetted open source projects may provide security issues. Attackers may take advantage of flaws in popular codebases, particularly if fixes or updates are sluggish.
Quality and Upkeep: Some open-source AI programs have out-of-date models or compatibility problems since they don’t get regular maintenance or upgrades. Projects often depend on unpaid volunteers, which may have an impact on the code’s upkeep and quality.
Complexity: Implementing Open Source AI may be challenging and may call for a high level of experience. Users could have trouble with initial setup or model tweaking in the absence of clear documentation or user assistance.
Ethics and Bias Issues: Training data may introduce biases into even open-source AI, which may have unforeseen repercussions. Users must follow ethical standards and do thorough testing since transparent code does not always translate into equitable results.
Commercial Competition: Open-source initiatives do not have the funds and resources that commercial AI tools possess, which might impede scaling or impede innovation.
Drawbacks
Open source AI is essential to democratizing technology.
Nevertheless, in order to realize its full potential and overcome its drawbacks, it needs constant maintenance, ethical supervision, and active community support.
Read more on Govindhtech.com
#OpensourceAI#Deeplearning#PyTorch#TensorFlow#AImodels#AIprograms#AIdevelopment#AItechnologies#AIframeworks#AItools#News#Technews#Technology#technologynews#Technologytrends#govindhtech
0 notes
Text
Google's AlphaChip and Meta's Llama 3.2 Signal Major Shifts in AI Strategies
Google and Meta update their AI strategies: Google launches AlphaChip for faster chip design and Gemini 1.5 model improvements, while Meta releases Llama 3.2 with powerful LLMs optimized for vision, edge, and mobile.
#thecioconnect#OnlineBusinessMagazine#BusinessMagazine#businessleaders#AIAssistant#MetaAI#AI#Google#Meta#AlphaChip#Llama3#AIModels#TechNews#Gemini#ChipDesign#MachineLearning#AIResearch#AIUpdates#OpenSourceAI#ArtificialIntelligence
0 notes
Text
How to Build a Private LLM 🚀
Want to create a private LLM for your business? Start by selecting a base model—open-source options like GPT-NeoX or LLaMA are popular. Fine-tune it with domain-specific data using transfer learning. Invest in quality datasets and ensure data privacy. You'll need significant compute resources; consider cloud providers offering GPU rentals. Finally, evaluate and continuously improve your model with feedback loops. Secure, customizable, and tailored to your needs—a private LLM can revolutionize your operations!
Read More:
0 notes
Text
instagram
Kyutai has just launched Moshi, an open-source AI assistant that can chat with you in real-time!
Developed in just six months, Moshi uses a special "Audio Language Model" to talk naturally without delays, offering an impressively smooth experience with only 200-240 milliseconds of latency.
Stay tuned for the open-source release soon, and get ready to explore the future of real-time AI conversations!
For more AI related updates, follow @trillionstech.ai
1 note
·
View note
Text
AI Expert Claims Big Tech Using Fear of AI for Market Control
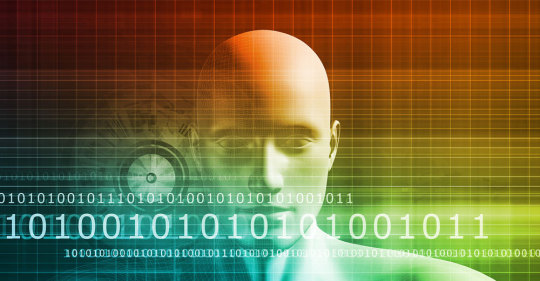
Big Tech leaders are exaggerating the threat AI poses to humanity to solidify their market shares through government regulation, a leading AI figure said Monday. https://jpmellojr.blogspot.com/2023/10/ai-expert-claims-big-tech-using-fear-of.html
0 notes
Text
Meta and Microsoft launch open-source artificial intelligence model "Llama 2"
#Meta#Microsoft#opensource#AI#artificialintelligence#machinelearning#deeplearning#LLAMA2#opensourceAI#opensourceML#opensourceDL#technews#research#innovation#datascience#tech
0 notes
Link
https://bit.ly/3WoRU5R - 🛡️ Defense Unicorns has launched LeapfrogAI, a promising open source project poised to enhance secure Generative AI solutions for highly regulated industries such as defense, intelligence, and commercial enterprises. LeapfrogAI is set to transform the way these sectors operate, optimizing their data advantages while maintaining stringent security protocols. #AI #DefenseUnicorns #LeapfrogAI 🚀 The rapid progress in open-source Generative AI is remarkable. Traditional general-purpose AI models are changing the landscape of business operations. Yet, fine-tuned open-source models backed by mission-specific data are often superior in performance. LeapfrogAI is designed to harness this power, providing a secure and efficient platform for integrating AI capabilities in-house. #OpenSourceAI #Innovation 🎯 With LeapfrogAI, users can deliver new Generative AI capabilities swiftly, ensure security and regulatory compliance, fine-tune models leveraging their data, retain data and model control, deploy AI solutions across various platforms and simplify the use of Generative AI. The Department of the Navy and the United States Space Force are among the early adopters. #GenerativeAI #AIForDefense ⚙️ LeapfrogAI aims to provide AI-as-a-service in resource-constrained environments, bringing sophisticated AI solutions closer to these challenging areas. It bridges the gap between limited resources and the growing AI demand by hosting APIs that offer AI-related services, such as vector databases, Large Language Model (LLM) completions, and creation of embeddings. #AIAsAService 🔐 Hosting your own Large Language Model (LLM) can offer several advantages like data privacy and security, cost-effectiveness, customization and control, and low latency. With LeapfrogAI, you have the flexibility to host your LLM, ensuring you have full control over your data and your AI solutions. #LLM #DataPrivacy 💼 LeapfrogAI provides an API closely aligned with OpenAI's, facilitating a seamless transition for developers familiar with OpenAI's API. Its features include efficient similarity searches via vector databases, fine-tuning models using customer-specific data, and generating embeddings for various tasks. #OpenAI #API 💡 Setting up the Kubernetes Cluster and deploying LeapfrogAI is straightforward, and usage guidelines are provided to help new users get started. LeapfrogAI also allows teams to deploy APIs that mirror OpenAI's spec, enabling secure AI integration without the risk of sensitive data being released to SaaS tools. #Kubernetes #AIDeployment ⚙️ To wrap up, LeapfrogAI is set to be a game-changer in the world of secure Generative AI, offering secure, flexible, and powerful AI solutions for various mission-driven organizations. #LeapfrogAI #AIRevolution GitHub: https://bit.ly/3MhxEP0
#AI#DefenseUnicorns#LeapfrogAI#OpenSourceAI#Innovation#GenerativeAI#AIForDefense#AIAsAService#LLM#DataPrivacy#OpenAI#API#Kubernetes#AIDeployment#AIRevolution
1 note
·
View note
Text
Learn about DeepSeek-R1, DeepSeek AI's open-source model enhancing reasoning through Reinforcement Learning. Explore its unique training, including direct RL and distillation for efficient models. Discover how it achieves emergent chain-of-thought reasoning for complex problem-solving.
#AI#DeepSeekR1#OpenSourceAI#MachineLearning#DeepLearning#ReinforcementLearning#open source#artificial intelligence#software engineering#machine learning#opensource#programming
0 notes
Text
Join #mozilla on their mission to democratize #artificialintelligence!
The tech company known for their human-first approach is taking on big tech giants like #google and #microsoft with their new #opensource AI initiative moz://a. With transparency, accountability, and trust at the core of their mission, Mozilla aims to create a more accessible and democratic AI landscape. By developing free and open-source AI products, they're taking an active role in shaping the future of AI. Read more to know about their first two projects under this initiative.
0 notes
Link
#3DModeling#3DReconstruction#agi#ai#aiforresearchpaper#AIResearch#AIResearchPapers#AITools#Animation#artificialintelligence#Avatars#CameraControl#CamI2V#ComputerVision#DreamVideo-2#Framer#Learning#MachineLearning#manuagi#Mind-BlowingAdvancementsYouWon'tBelieve#opensourceai#ResearchPapers#softwareforresearchers#Top10AIResearchPapersThisWeek#TopAIResearchPapersThisWeek#topaitools#VideoGames
0 notes
Text
IBM Research Data Loader Helps Open-source AI Model Training
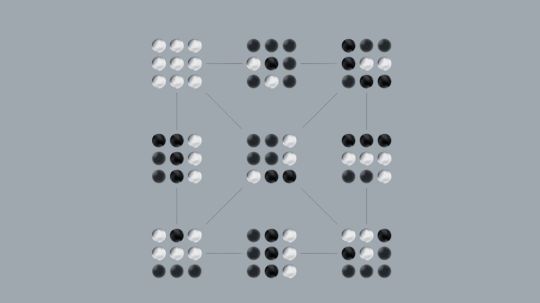
IBM Research data loader improves open-source community’s access to AI models for training.
Training AI models More quickly than ever
IBM showcased new advances in high-throughput AI model training at PyTorch 2024, along with a state-of-the-art data loader, all geared toward empowering the open-source AI community.
IBM Research experts are contributing to the open-source model training framework at this year’s PyTorch Conference. These contributions include major advances in large language model training throughput as well as a data loader that can handle enormous amounts of data with ease.
It must constantly enhance the effectiveness and resilience of the cloud infrastructure supporting LLMs’ training, tuning, and inference to supply their ever-increasing capabilities at a reasonable cost. The open-source PyTorch framework and ecosystem have greatly aided the AI revolution that is about to change its lives. IBM joined the PyTorch Foundation last year and is still bringing new tools and techniques to the AI community because it recognizes that it cannot happen alone.
In addition to IBM’s earlier contributions, these new tools are strengthening PyTorch’s capacity to satisfy the community’s ever-expanding demands, be they related to more cost-effective checkpointing, faster data loading, or more effective use of GPUs.
An exceptional data loader for foundation model training and tuning
Using a high-throughput data loader, PyTorch users can now easily distribute LLM training workloads among computers and even adjust their allocations in-between jobs. In order to prevent work duplication during model training, it also enables developers to save checkpoints more effectively. And all of it is attributable to a group of researchers who were only creating the instruments they required to complete a task.
When you wish to rerun your training run with a new blend of sub-datasets to alter model weights, or when you have all of your raw text data and want to use a different tokenizer or maximum sequence length, the resulting tool is well-suited for LLM training in research contexts. With the help of the data loader, you can tell your dataset what you want to do on the fly rather than having to reconstruct it each time you want to make modifications of this kind.
You can adjust the job even halfway through, for example, by increasing or decreasing the number of GPUs in response to changes in your resource quota. The data loader makes sure that data that has already been viewed won’t be viewed again.
Increasing the throughput of training
Bottlenecks occur because everything goes at the speed of the slowest item when it comes to model training at scale. The efficiency with which the GPU is being used is frequently the bottleneck in AI tasks.
Fully sharded data parallel (FSDP), which uniformly distributes big training datasets across numerous processors to prevent any one machine from becoming overburdened, is one component of this method. It has been demonstrated that this distribution greatly increases the speed and efficiency of model training and tuning while enabling faster AI training with fewer GPUs.
This development progresses concurrently with the data loader since the team discovered ways to use GPUs more effectively while they worked with FSDP and torch.compile to optimize GPU utilization. Consequently, data loaders rather than GPUs became the bottleneck.
Next up
Although FP8 isn’t yet generally accessible for developers to use, Ganti notes that the team is working on projects that will highlight its capabilities. In related work, they’re optimizing model tweaking and training on IBM’s artificial intelligence unit (AIU) with torch.compile.
Triton, Nvidia’s open-source platform for deploying and executing AI, will also be a topic of discussion for Ganti, Wertheimer, and other colleagues. Triton allows programmers to write Python code that is then translated into the native programming language of the hardware Intel or Nvidia, for example, to accelerate computation. Although Triton is currently ten to fifteen percent slower than CUDA, the standard software framework for using Nvidia GPUs, the researchers have just completed the first end-to-end CUDA-free inferencing with Triton. They believe Triton will close this gap and significantly optimize training when this initiative picks up steam.
The starting point of the study
IBM Research’s Davis Wertheimer outlines a few difficulties that may arise during extensive training: It’s possible to use an 80/20 rule to large-scale training. In the published research, algorithmic tradeoffs between GPU memory and compute and communication make up 80% of the work. However, because the pipeline moves at the pace of the narrowest bottleneck, you may expect a very long tail of all these other practical concerns when you really try to build something 80 percent of the time.
The IBM team was running into problems when they constructed their training platform. Wertheimer notes, “As we become more adept at using our GPUs, the data loader is increasingly often the bottleneck.”
Important characteristics of the data loader
Stateful and checkpointable: If your data loader state is saved whenever you save a model, and both the model state and data loader states need to be recovered at the same time whenever you recover from a checkpoint.”
Checkpoint auto-rescaling: During prolonged training sessions, the data loader automatically adapts to workload variations. There are a lot of reasons why you might have to rescale your workload in the middle. Training could easily take weeks or months.”
Effective data streaming: There is no build overhead for shuffling data because the system supports data streaming.
Asynchronous distributed operation: The data loader is non-blocking. The data loader states to be saved and then distributed in a way that requires no communication at all.”
Dynamic data mixing: This feature is helpful for changing training requirements since it allows the data loader to adjust to various data mixing ratios.
Effective global shuffling: As data accumulates, shuffling remains effective since the tool handles memory bottlenecks when working with huge datasets.
Native, modular, and feature-rich PyTorch: The data loader is built to be flexible and scalable, making it ready for future expansion. “What if we have to deal with thirty trillion, fifty trillion, or one hundred trillion tokens next year?” “it needs to build the data loader so it can survive not only today but also tomorrow because the world is changing quickly.”
Actual results
The IBM Research team ran hundreds of small and big workloads over several months to rigorously test their data loader. They saw code numbers that were steady and fluid. Furthermore, the data loader as a whole runs non-blocking and asynchronously.
Read more on govindhtech.com
#IBMResearch#DataLoaderHelp#OpensourceAI#ModelTraining#AImodel#IBM#dataloader#Triton#IBMartificialintelligence#startingpoint#ibm#technology#technews#news#govindhtech
0 notes
Text
youtube
DeepSeek-V3: The AI Revolution Changing Everything You Know! #ArtificialIntelligence, #DeepSeekV3, by Livio Andrea Acerbo (lacer2k) DeepSeek-V3: The AI Revolution Changing Everything You Know! #ArtificialIntelligence, #DeepSeekV3, #AIRevolution, #TechInnovation, #OpenSourceAI, #FutureTech, #DigitalTransformation, #TechNews, #AIForGood, #Greenground The AI world just got rocked by DeepSeek-V3! This revolutionary open-source model with 671 billion parameters is setting new benchmarks for speed, efficiency, and accessibility. Developed at a fraction of the usual cost, DeepSeek-V3 promises to democratize AI innovation like never before. Join us as we dive deep into how this game-changing AI is outperforming giants like OpenAI and Meta, its fascinating quirks, and what it means for the future of technology. From blazing fast performance to its potential to level the playing field, DeepSeek-V3 is more than a model—it’s a movement. Don’t miss out—watch, like, and subscribe for more exciting content about the future of tech! Find all the details in the description or ask your questions in the comments. Let’s shape the future together! Let’s push the boundaries of imagination together! 🚀 and Remember, if you want to stay updated with the latest trends, news, and tech reviews, don’t forget to like and subscribe to GreengroundIT! Follow up on our website https://ift.tt/ltNLGKm or follow up on our social #fyp, #foryou, #love, #explorepage, #trending, #reviews, #news YouTube: https://youtube.com/TikTok: https://ift.tt/ekbDwRV: https://ift.tt/Ap1rV3M Instagram: https://ift.tt/INJmFxX Podcast: https://ift.tt/aF98MfA Medium: https://ift.tt/L25X0xS: https://ift.tt/SWDXM3B Reddit: https://ift.tt/jqw1y2V Rumble: https://ift.tt/PX5Eq9e Soundcloud: https://ift.tt/ap3wBuQ Suno: https://suno.com/ via YouTube https://www.youtube.com/watch?v=va0RzHBG9mA
0 notes
Text
The Colonial Bookstore, political satire, honesty, boris johnson, justin trudeau, education, colonialsm, economic exploitation, oppression #ClimateJustice #RightsOfNature #HumanRights
https://docs.google.com/document/d/e/2PACX-1vTX8K_fI6kgAIOeqR9BFiEdk9How97PTQUO2czQ643QfkwBhlkBuLTldaB61KOsspzBosMWyhApKIrv/pub https://unitedforclimate.blogspot.com/2024/11/we-only-sell-honest-impartial-images.html https://labradorleadstheworld.blogspot.com/2024/11/we-only-sell-honest-impartial-images.html https://muskratfallscivilrightsmovement.blogspot.com/2024/11/we-only-sell-honest-impartial-images.html https://landprotectorshumanrightsmovement.blogspot.com/2024/11/we-only-sell-honest-impartial-images.html The Colonial Bookstore: We only sell honest impartial images #DeepAiImage #PoliticalSatire … ‘The colonial legacies of the UK and Canada create both increased responsibility and challenges for their fossil fuel extraction policies. While these histories have led to ambitious rhetoric and some initiatives, both countries still face criticism for not doing enough to address their historical emissions and support global climate action. Their approaches to fossil fuel extraction are thus shaped by the need to balance domestic economic concerns with growing international pressure to lead on climate action due to their colonial pasts.’ #OpenSourceAI #Perplexity #DeepAI Llewelyn Pritchard 9 November 2024
#Colonialism#PriviligedEducation#WhiteSupremacy#Racism#ArtificialIntelligence#AI#Ethics#Morality#Law#Justice#Education#Standards#ParliamentaryStandards#Constitution#ConstitutionalCorrosion#DefendDemocracy#LawNational#LawInternational#Democracy#DemocraticInstitutions#DemocraticStandards#PublicOffice#StandardsInPublicOffice#CivilServants#Politicians#PrimeMinister#GamingTheSystem#PublicServants#Truth#Accountability
1 note
·
View note