#legal GenAI solutions
Explore tagged Tumblr posts
Text
How generative AI framework offers efficiency in legal drafting?
In the legal industry, contract drafting has long been a time-consuming and intricate task. Traditional methods often involve meticulous manual work, which increases the risk of human error and leads to high costs.
How much time do you spend manually renumbering paragraphs or re-entering client comments lost during copying and pasting?
How long does it take to perfect your document?
How much time it takes to refer to different documents and extract accurate information from those documents?
If you answered these questions with — too much or too long, you are not alone. Lawyers spend roughly 40–60% of their time drafting and reviewing contracts. The hassles of traditional methods are evident.
According to a study by the American Bar Association, AI-based document review can improve the speed and accuracy of document review by up to 90%.
This is where generative AI solutions come into play. GenAI-powered automated drafting and review free up substantial time, enabling law professionals to dedicate more attention to their cases.
In this blog, we will explore how generative AI helps with contract drafting, its benefits, and use cases.
The challenges of traditional contract drafting
Time-consuming non-billable hours: Drafting a comprehensive and legally sound contract demands significant time and effort. It often entails multiple rounds of revisions and consultations among legal teams and clients, prolonging the contract lifecycle.
Lack of standardization: Contracts drafted and reviewed manually are often inconsistent in language and clauses. Also, up-to-date versions of contracts are not easily accessible to the lawyers.
Risk of human errors: Manual drafting increases the vulnerability to errors such as omissions, inconsistencies, and inaccuracies. These errors can compromise the legal validity of contracts, potentially leading to disputes and legal liabilities.
High costs: The labor-intensive nature of traditional contract drafting translates into substantial financial expenditures. Law firms and their clients bear costs associated with prolonged drafting cycles, extensive review processes, and the need for specialized legal expertise.
Where traditional methods fail, generative AI solutions for the legal industry help address these issues. Let’s explore how the technology helps in detail.
How legal document automation with generative AI addresses these challenges
Automate repetitive tasks
Automate routine and repetitive tasks such as clause insertion, formatting, and renumbering of paragraphs. This reduces the manual workload on legal professionals, allowing them to focus on more complex aspects of contract drafting and other billable opportunities.
Draft contracts efficiently
NLP algorithms analyze and understand legal language, enabling AI systems to draft contracts that adhere to legal standards and best practices. This ensures the inclusion of all necessary clauses and provisions, reducing the risk of human errors and omissions.
Navigate the future with generative AI: A step-by-step guide for business implementation
Discover the benefits, understand the implementation process, and follow a detailed step-by-step guide to successfully integrate generative AI into your operations.
Download
Template generation and customization
Create and customize contract templates based on specific legal requirements and client needs. This standardization ensures consistency across all contracts.
Real-time collaboration and review
AI facilitates real-time collaboration among legal teams, enabling multiple stakeholders to review and edit contracts simultaneously. This streamlines the review process, reduces delays, and enhances overall document production efficiency for litigation and other legal matters.
Explore the benefits of our robust generative AI framework, Needle! Get a free demo
Predictive analytics and risk management
AI tools use predictive analytics to foresee potential legal risks in the documentation and suggest preventive measures. By analyzing patterns and trends in legal data, AI helps in identifying and mitigating risks early in the drafting process.
Efficiency in legal drafting and research
Generative AI integrates AI legal research capabilities, allowing legal professionals to access comprehensive and up-to-date legal information quickly. This supports informed decision-making and ensures that contracts are aligned with current legal standards.
Contract lifecycle management
Key benefits of contract drafting with AI
Enhanced accuracy and reduced errors: By automating repetitive tasks and leveraging pre-approved clauses, you can minimize the risk of human error.
Cost savings and resource optimization: AI reduces the time and resources needed for contract drafting, freeing up lawyers to focus on higher-value strategic tasks.
Expedited document production: Accelerate the drafting process by significantly reducing the time required to produce comprehensive legal documents. This leads to faster turnaround times and improved client satisfaction.
Improved client relationship: Enhance the overall client experience by providing accurate and timely legal documents. Clients benefit from faster service delivery and the assurance that their legal matters are handled with the utmost precision.
Suggested: 5 tips to implement generative AI in your organization
Real-life use cases of generative AI in the legal industry
Dechert LLP
Dechert LLP is a multinational American law firm with more than 900 lawyers. The company developed DechertMind, a proprietary suite of generative AI tools designed to transform law firm operations. Dechert LLP used their generative AI solutions for:
Automated contract drafting
Enhanced legal research
Improved document review
Client service optimization
Dentons
Dentons is the world’s largest global law firm by number of lawyers and the 6th-largest law firm by revenue. The company launched a client-secure version of ChatGPT to enhance client interactions and legal services. This solution offered them the following benefits:
Secure client communication
Efficient legal support
Legal AI assistant
Enhanced legal research
McGuireWoods
McGuireWoods was established in 1834 and is the largest law firm in the US. They embraced AI innovation through its partnership with Casetext Co-Counsel. The integration of generative AI in legal research ensures prompt resolution of client issues and facilitates the provision of accurate information for client documentation.
Enhanced legal research
Faster contract drafting
Improved operational efficiency
The future of generative AI in the legal industry
Integration with blockchain: Combining AI with blockchain technology can provide greater transparency and security in contract management.
Personalized contract templates: Generative AI will be capable of creating highly customized contract templates tailored to specific client needs and industry standards.
Enhanced predictive analytics: By analyzing vast amounts of data from previous cases and contracts, generative AI solutions will provide more accurate risk assessments and suggest preventive measures, aiding legal professionals in making more informed decisions.
AI-driven negotiation support: AI tools will assist in the negotiation phase by providing real-time suggestions and counterproposals based on historical data and legal precedents.
Increased adoption of generative AI in small and med-size legal firms: While large law firms are already embracing AI, the future will see increased adoption among small and medium-sized firms. As AI technology becomes more accessible and cost-effective, these firms will leverage generative AI to enhance their competitiveness and service offerings.
Efficiency in legal drafting and compliance: AI will play a critical role in ensuring authentication of legal documents. It will also ensure legal compliance by continuously monitoring regulatory changes and updating contract templates accordingly.
Leverage legal document automation to achieve operational excellence
As AI technology advances, it’s impact and adoption in the legal industry will see a significant growth. While many companies are utilizing generative AI for time-consuming task of contract drafting, the technology offers more opportunities. With AI-powered tools, law firms can gain a competitive advantage.
One notable example of such a tool is Needle, a generative AI framework developed by Softweb Solutions. Needle integrates seamlessly into legal workflows, providing robust AI capabilities for efficient contract drafting. To explore more benefits and use cases of our GenAI framework, contact our AI experts.
Originally published at https://www.softwebsolutions.com on July 17, 2024.
#legal GenAI solutions#generative AI solutions#generative AI solutions for the legal industry#generative AI framework
0 notes
Text
[Image IDs: Image #1: Headline reading: The Internet Archive Loses Its Appeal of a Major Copyright Case. Subheading: Hachette v. Internet Archive was brought by book publishers objecting to the archive's digital lending library.
Image #2: Tumblr post reading: This is all so true and that's why I hate GenAI even more, because it feels like relying on copyright laws is the only legal protection we have from it. Tumblr tags reading: #internet archive #fan works #copyright #us politics #informative post #i think one solution is that original creators should receive a mandatory percentage from any profits made by derivative works #profits in this case being those directly linked to sales of the derivative or in providing access to the derivative #that way not-for-profit institutions like public libraries would be protected #but so would smaller creators from corporations stealing and reproducing their works on a larger scale #hmmm perhaps i'd actually still make it illegal for corporations to do so #like we have gotta find a way to separate legal "human" rights from these businesses bc they are literally not people! /End IDs]
i am pro-copyright infringement. anybody who does fanfiction or fanart or anything should be pro-copyright infringement and obviously we are on the Fanart Website. why this is not a more popular stance among people who spend all their time doing transformative works is beyond me
21K notes
·
View notes
Text
Contract Authorization On The Blockchain
Integra Ledger, the legal blockchain pioneer, has launched ‘Integra Ledger Connect’ to help lawyers authenticate and automate contracts, which the company sees as very timely in a new era where genAI can be used for deepfakes and other forms of data manipulation, as well as creating unintentional errors and hallucinations as key documents are reviewed and edited.
Key features include:
‘Guards Against AI Manipulation: Provides a robust shield for critical documents against emerging AI-driven risks, ensuring the authenticity of contracts, invoices, payments, and financial records in an increasingly complex digital landscape.
Eliminate Document Fraud and Uncertainty: Registers and timestamps a permanent blockchain identifier and hash (‘digital fingerprint’) for every document, guaranteeing absolute time- and date-stamped document identity and integrity while reducing administrative costs and errors.
Streamline Cross-Organizational Workflows: Enables peer-to-peer contract automation between organizations even when they are using different software, unlocking efficiencies previously considered unattainable throughout entire ecosystems of clients and suppliers.
Enhance Transaction Trust with Contract Tokenization: Digitally binds ownership information to contract identity, providing public proof of transactions on the Integra Ledger blockchain and increasing transaction integrity, trust, and efficiency
Integrated Financial Solutions: Optionally encodes payment instructions directly into contracts, supporting ACH, credit card, and cryptocurrency transactions while integrating smoothly with existing payment software.
Comprehensive Compliance Protection: Registers digital proof of all contract-related events with an immutable blockchain- registered audit trail, streamlining due diligence and safeguarding against mistakes and disputes.
https://www.artificiallawyer.com/2024/12/05/blockchain-pioneer-integra-targets-contract-authentication/
0 notes
Text
IBM Watsonx.ai Management Tools Release GenAI Possibility
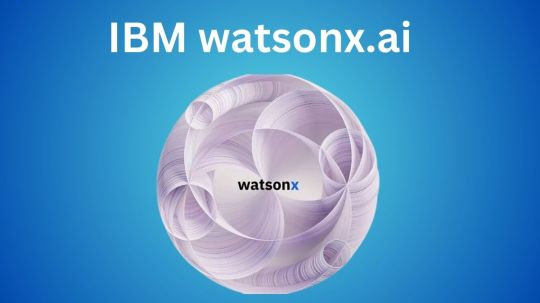
IBM Watsonx.ai Management Tools Unleash GenAI Potential.
US regulators like the FRB, SEC, and OCC require financial services firms to show that their risk governance structure addresses laws, rules, and regulations (LRRs). This monitoring helps maintain a safe and dependable control environment that meets tighter rules and the organization’s risk tolerance.
However, determining the applicability of banking regulations to particular sections of a legislation can be a difficult and subjective process that calls for expert judgment. Based on the bank’s attributes, such as being a Global Systemically Important Bank (GSIB) or providing certain goods and services, banks frequently depend on outside suppliers to evaluate LRRs and generic controls.
Furthermore, LRRs are always changing, as are other industry frameworks like the Control Objectives for Information and Related Technologies (COBIT), Information Technology Infrastructure Library (ITIL), and National Institute of Standards and Technology (NIST).
This ongoing development necessitates constant work to help guarantee that the organization’s control environment is free of holes. Regretfully, it takes a lot of time and frequently causes delays to manually link LRRs to rules, standards, procedures, risk metrics, and controls. This procedure creates a discrepancy between the organization’s capacity to prove compliance with LRRs and regulatory expectations.
For instance, a bank may have a policy requiring the protection of its clients’ personal information, and the standard may call for the encryption of such information. In that scenario, the control would assist in guaranteeing that personal data is encrypted, and the procedure would specify the steps to encrypt it. However, the bank may not be able to prove compliance with the encryption standard, putting them at risk of noncompliance, if the links between LRRs and controls are not updated promptly.
The watsonx Regulatory Compliance Platform reduces manual effort for control owners, compliance, risk and legal teams
Legal and regulatory requirements can be mapped to a risk governance framework using IBM Watsonx, which also automates the identification of regulatory duties. This solution facilitates the verification of compliance with current responsibilities by examining governance documents and controls and connecting them to relevant LRRs. By using this technology, audit, compliance, risk, legal, IT, and business control owners can construct and maintain LRR libraries with a great deal less human labor.
For instance, Watson Discovery can undertake an effect analysis by actively searching the internet for regulatory revisions for a certain group of LRRs. Watson Assistant can be utilized as an interactive Q&A tool to answer questions from external parties, auditors, and regulators regarding the risk and control environment in a conversational fashion. A risk and compliance program is increasingly using large language model (LLM), which need little to no training.
To apply the banks’ different process, risk, and control taxonomies, LLMs stored in Watsonx augment LRR and governance data. A prompt evaluates an obligation using a programmed approach. For instance, every risk category of the company, including strategic, reputational, wholesale, interest rate, and liquidity risks, would be examined to see what applies. The matching categories to internal controls and other pertinent policy and governance datasets are supported by the improved metadata.
When the content is publicly accessible, whether from third parties or is curated by the organization in an obligation’s library, the procedure is uniform and repeatable across regulations. IT and cybersecurity frameworks like NIST, ITIL, COBIT, Cloud Security Alliance Control Matrix, Federal Financial Institutions Examination Council (FFIEC), and others are included in the mapping and coverage capabilities that are not exclusive to LRRs.
The solution may link the pertinent LRRs to the applicable NIST controls, for example, if a bank wishes to guarantee adherence to the NIST cybersecurity framework. This gives the bank a clear and thorough picture of its cybersecurity posture.
IBM Watsonx.ai
How the watsonx Regulatory Compliance Platform accelerates risk management
The platform’s advanced artificial intelligence (AI) modules, IBM Watsonx.ai, watsonx.gov, and watsonx.data, provide a variety of cutting-edge technical features tailored to the particular requirements of the sector. These components, which may be installed on-premises or in any cloud, are based on IBM’s cutting-edge AI technology.
Users can participate in the whole lifecycle management of generative AI (gen AI) solutions within the IBM Watsonx.ai platform, which includes training, validation, tuning, and deployment processes. Watsonx.ai supports a variety of natural and programming language use cases by facilitating the development of expanded language models through the usage of foundation models from IBM and other sources.
The platform includes the cutting-edge Prompt Lab tool, which was created especially to expedite prompt engineering procedures. By using pre-written sample prompts, customers may confidently start their regulatory and compliance projects quickly and save successful prompts as notebook entries or reusable resources.
Interestingly, the prompt engineering parameters, model references, and prompt wording are all carefully formatted as Python code inside notebooks, enabling smooth programmable interaction. Additionally, IBM Watsonx.ai provides the Tuning Studio function, which enables users to iteratively steer foundation models toward outputs that are more in line with their particular needs.
Watsonx.governance‘s comprehensive suite of tools allows customers to quickly construct responsible, transparent, and explainable AI workflows that are suited to both machine learning and generative AI models. When installed, watsonx.governance combines the features of AI factsheets and Watson OpenScale with the Model Risk Governance features of OpenPages into a single service.
Watsonx.governance also expands its governance features to include generative AI assets. This platform enables users to evaluate machine learning models and foundation model prompts, build AI use cases for the methodical tracking of solutions addressing relevant business concerns, and develop processes while precisely monitoring lifecycle activities.
By supporting data from various sources and removing the requirement for migration or cataloging through open formats, IBM Watsonx.data enables scalable analytics and AI initiatives. This method reduces data duplication and extract, transform, and load (ETL) operations while allowing centralized access and sharing. Data preparation for a variety of applications, including retrieval augmented generation (RAG) and other machine learning and generative AI use cases, is made easier by integrated vectorized embedding capabilities.
Without the need for SQL knowledge, a conversational interface driven by Gen AI makes data discovery, augmentation, and visualization easier. Interoperability is ensured by smooth interface with current data stacks, tools, and databases.
All things considered, using Watsonx for regulatory compliance provides a revolutionary method of transparently and responsibly managing risk and AI projects. Organizations may easily handle the intricacies of regulatory requirements by utilizing its full range of capabilities. This makes it easier to guarantee ethical AI practices throughout the whole lifecycle, from data management to model training. IBM Watsonx.ai enables users to confidently evaluate, track, and improve AI workflows, promoting creativity and confidence in AI-driven solutions while easing regulatory compliance.
Read more on Govindhtech.com
#IBM Watsonx.ai#largelanguagemodel#artificialintelligence#Python#Watsonxgovernance#retrievalaugmentedgenerationRAG#machinelearning#News#Technews#technology#technologynews
0 notes
Text
Real Identities Can Be Recovered From Synthetic Datasets
New Post has been published on https://thedigitalinsider.com/real-identities-can-be-recovered-from-synthetic-datasets/
Real Identities Can Be Recovered From Synthetic Datasets
If 2022 marked the moment when generative AI’s disruptive potential first captured wide public attention, 2024 has been the year when questions about the legality of its underlying data have taken center stage for businesses eager to harness its power.
The USA’s fair use doctrine, along with the implicit scholarly license that had long allowed academic and commercial research sectors to explore generative AI, became increasingly untenable as mounting evidence of plagiarism surfaced. Subsequently, the US has, for the moment, disallowed AI-generated content from being copyrighted.
These matters are far from settled, and far from being imminently resolved; in 2023, due in part to growing media and public concern about the legal status of AI-generated output, the US Copyright Office launched a years-long investigation into this aspect of generative AI, publishing the first segment (concerning digital replicas) in July of 2024.
In the meantime, business interests remain frustrated by the possibility that the expensive models they wish to exploit could expose them to legal ramifications when definitive legislation and definitions eventually emerge.
The expensive short-term solution has been to legitimize generative models by training them on data that companies have a right to exploit. Adobe’s text-to-image (and now text-to-video) Firefly architecture is powered primarily by its purchase of the Fotolia stock image dataset in 2014, supplemented by the use of copyright-expired public domain data*. At the same time, incumbent stock photo suppliers such as Getty and Shutterstock have capitalized on the new value of their licensed data, with a growing number of deals to license content or else develop their own IP-compliant GenAI systems.
Synthetic Solutions
Since removing copyrighted data from the trained latent space of an AI model is fraught with problems, mistakes in this area could potentially be very costly for companies experimenting with consumer and business solutions that use machine learning.
An alternative, and much cheaper solution for computer vision systems (and also Large Language Models, or LLMs), is the use of synthetic data, where the dataset is composed of randomly-generated examples of the target domain (such as faces, cats, churches, or even a more generalized dataset).
Sites such as thispersondoesnotexist.com long ago popularized the idea that authentic-looking photos of ‘non-real’ people could be synthesized (in that particular case, through Generative Adversarial Networks, or GANs) without bearing any relation to people that actually exist in the real world.
Therefore, if you train a facial recognition system or a generative system on such abstract and non-real examples, you can in theory obtain a photorealistic standard of productivity for an AI model without needing to consider whether the data is legally usable.
Balancing Act
The problem is that the systems which produce synthetic data are themselves trained on real data. If traces of that data bleed through into the synthetic data, this potentially provides evidence that restricted or otherwise unauthorized material has been exploited for monetary gain.
To avoid this, and in order to produce truly ‘random’ imagery, such models need to ensure that they are well-generalized. Generalization is the measure of a trained AI model’s capability to intrinsically understand high-level concepts (such as ‘face’, ‘man’, or ‘woman’) without resorting to replicating the actual training data.
Unfortunately, it can be difficult for trained systems to produce (or recognize) granular detail unless it trains quite extensively on a dataset. This exposes the system to risk of memorization: a tendency to reproduce, to some extent, examples of the actual training data.
This can be mitigated by setting a more relaxed learning rate, or by ending training at a stage where the core concepts are still ductile and not associated with any specific data point (such as a specific image of a person, in the case of a face dataset).
However, both of these remedies are likely to lead to models with less fine-grained detail, since the system did not get a chance to progress beyond the ‘basics’ of the target domain, and down to the specifics.
Therefore, in the scientific literature, very high learning rates and comprehensive training schedules are generally applied. While researchers usually attempt to compromise between broad applicability and granularity in the final model, even slightly ‘memorized’ systems can often misrepresent themselves as well-generalized – even in initial tests.
Face Reveal
This brings us to an interesting new paper from Switzerland, which claims to be the first to demonstrate that the original, real images that power synthetic data can be recovered from generated images that should, in theory, be entirely random:
Example face images leaked from training data. In the row above, we see the original (real) images; in the row below, we see images generated at random, which accord significantly with the real images. Source: https://arxiv.org/pdf/2410.24015
The results, the authors argue, indicate that ‘synthetic’ generators have indeed memorized a great many of the training data points, in their search for greater granularity. They also indicate that systems which rely on synthetic data to shield AI producers from legal consequences could be very unreliable in this regard.
The researchers conducted an extensive study on six state-of-the-art synthetic datasets, demonstrating that in all cases, original (potentially copyrighted or protected) data can be recovered. They comment:
‘Our experiments demonstrate that state-of-the-art synthetic face recognition datasets contain samples that are very close to samples in the training data of their generator models. In some cases the synthetic samples contain small changes to the original image, however, we can also observe in some cases the generated sample contains more variation (e.g., different pose, light condition, etc.) while the identity is preserved.
‘This suggests that the generator models are learning and memorizing the identity-related information from the training data and may generate similar identities. This creates critical concerns regarding the application of synthetic data in privacy-sensitive tasks, such as biometrics and face recognition.’
The paper is titled Unveiling Synthetic Faces: How Synthetic Datasets Can Expose Real Identities, and comes from two researchers across the Idiap Research Institute at Martigny, the École Polytechnique Fédérale de Lausanne (EPFL), and the Université de Lausanne (UNIL) at Lausanne.
Method, Data and Results
The memorized faces in the study were revealed by Membership Inference Attack. Though the concept sounds complicated, it is fairly self-explanatory: inferring membership, in this case, refers to the process of questioning a system until it reveals data that either matches the data you are looking for, or significantly resembles it.
Further examples of inferred data sources, from the study. In this case, the source synthetic images are from the DCFace dataset.
The researchers studied six synthetic datasets for which the (real) dataset source was known. Since both the real and the fake datasets in question all contain a very high volume of images, this is effectively like looking for a needle in a haystack.
Therefore the authors used an off-the-shelf facial recognition model† with a ResNet100 backbone trained on the AdaFace loss function (on the WebFace12M dataset).
The six synthetic datasets used were: DCFace (a latent diffusion model); IDiff-Face (Uniform – a diffusion model based on FFHQ); IDiff-Face (Two-stage – a variant using a different sampling method); GANDiffFace (based on Generative Adversarial Networks and Diffusion models, using StyleGAN3 to generate initial identities, and then DreamBooth to create varied examples); IDNet (a GAN method, based on StyleGAN-ADA); and SFace (an identity-protecting framework).
Since GANDiffFace uses both GAN and diffusion methods, it was compared to the training dataset of StyleGAN – the nearest to a ‘real-face’ origin that this network provides.
The authors excluded synthetic datasets that use CGI rather than AI methods, and in evaluating results discounted matches for children, due to distributional anomalies in this regard, as well as non-face images (which can frequently occur in face datasets, where web-scraping systems produce false positives for objects or artefacts that have face-like qualities).
Cosine similarity was calculated for all the retrieved pairs, and concatenated into histograms, illustrated below:
A Histogram representation for cosine similarity scores calculated across the diverse datasets, together with their related values of similarity for the top-k pairs (dashed vertical lines).
The number of similarities is represented in the spikes in the graph above. The paper also features sample comparisons from the six datasets, and their corresponding estimated images in the original (real) datasets, of which some selections are featured below:
Samples from the many instances reproduced in the source paper, to which the reader is referred for a more comprehensive selection.
The paper comments:
‘[The] generated synthetic datasets contain very similar images from the training set of their generator model, which raises concerns regarding the generation of such identities.’
The authors note that for this particular approach, scaling up to higher-volume datasets is likely to be inefficient, as the necessary computation would be extremely burdensome. They observe further that visual comparison was necessary to infer matches, and that the automated facial recognition alone would not likely be sufficient for a larger task.
Regarding the implications of the research, and with a view to roads forward, the work states:
‘[We] would like to highlight that the main motivation for generating synthetic datasets is to address privacy concerns in using large-scale web-crawled face datasets.
‘Therefore, the leakage of any sensitive information (such as identities of real images in the training data) in the synthetic dataset spikes critical concerns regarding the application of synthetic data for privacy-sensitive tasks, such as biometrics. Our study sheds light on the privacy pitfalls in the generation of synthetic face recognition datasets and paves the way for future studies toward generating responsible synthetic face datasets.’
Though the authors promise a code release for this work at the project page, there is no current repository link.
Conclusion
Lately, media attention has emphasized the diminishing returns obtained by training AI models on AI-generated data.
The new Swiss research, however, brings to the focus a consideration that may be more pressing for the growing number of companies that wish to leverage and profit from generative AI – the persistence of IP-protected or unauthorized data patterns, even in datasets that are designed to combat this practice. If we had to give it a definition, in this case it might be called ‘face-washing’.
* However, Adobe’s decision to allow user-uploaded AI-generated images to Adobe Stock has effectively undermined the legal ‘purity’ of this data. Bloomberg contended in April of 2024 that user-supplied images from the MidJourney generative AI system had been incorporated into Firefly’s capabilities.
† This model is not identified in the paper.
First published Wednesday, November 6, 2024
#2022#2023#2024#adobe#Adobe Stock#ai#AI image generators#ai model#AI models#ai security#ai-generated content#anomalies#approach#architecture#Art#Artificial Intelligence#attention#bearing#biometrics#Business#cats#Children#code#Companies#comparison#comprehensive#compromise#computation#computer#Computer vision
0 notes
Text
Unlocking the Power of Intelligent Document Processing (IDP) with Generative AI

Intelligent Document Processing, or IDP, is becoming essential for data-focused organizations. Whether it’s in healthcare, legal services, or handling invoices, using AI for document processing is now standard practice.
Think about the time when organizations had to spend hours manually extracting, sorting, classifying, and analyzing data. Now, thanks to the rapid progress in technology, enormous amounts of data can be processed and stored by computers in just minutes with IDP solutions.
A 2022 survey by McKinsey shows that AI adoption has doubled in the last five years due to increased investment in the field. This indicates the growing demand and potential for intelligent document automation around the world.
This blog will help you understand intelligent document processing and how it has evolved, especially with the development of Generative AI.
Understanding GenAI in IDPBefore diving into how intelligent document processing works and the role of Generative AI in it, it's important to first understand the various elements involved in the process.
What Is Generative AI?Generative Artificial Intelligence, or GenAI, is a type of AI that can create new content on its own using algorithms.
Unlike traditional AI, which follows set patterns, GenAI learns from feedback and creates content based on patterns and examples found in data.
With advancements in technology, Generative AI can now mimic human-like intelligence and creativity by using techniques like deep learning and reinforcement learning in its algorithms.
GenAI has shown impressive capabilities in areas like natural language processing (NLP), music creation, and image generation. One of its significant strengths is in Optical Character Recognition (OCR), which forms the foundation of intelligent document processing.
What Is Intelligent Document Processing (IDP)?Intelligent document processing is a technology that organizations use to extract and process data from unstructured documents through automation.
IDP software uses technologies like Optical Character Recognition (OCR), Natural Language Processing (NLP), and Machine Learning (ML) to automate workflows, particularly those centered around documents.
IDP solutions have transformed manual tasks within organizations by automating them. This has led to improved efficiency, accuracy, and scalability in document processing. It also allows human resources to focus on more important tasks and decisions, making decision-making more effective.
GenAI in IDPThe integration of GenAI in IDP solutions has made document processing more advanced, enabling the system to understand, interpret, and generate content that reflects human intelligence and creativity.
With GenAI in IDP, document processing can now extract text more accurately, recognize patterns in data, and adapt to changing formats, fonts, and languages more effectively.
GenAI has solved many challenges faced by traditional OCR technology, allowing for the automation of complex document processing tasks with greater efficiency and accuracy.
How IDP WorksHere are the steps involved in producing accurate and effective results with IDP software:
Document Ingestion: Documents are scanned or uploaded into the IDP system.
Pre-Processing: Before processing, the document is pre-processed to enhance readability, often through image enhancement and OCR.
Data Extraction: Generative AI algorithms analyze the text to extract relevant information, ignoring unnecessary data. Important details like names, dates, and amounts are identified.
Validation and Verification: The extracted data is checked against set rules and verified for accuracy.
Integration: The processed data is integrated into the organization’s workflows for further action.
The IDP RevolutionGenerative AI and intelligent document processing have revolutionized the world of document automation.
Understanding the need and benefits of Generative AI can help organizations fully unlock the potential of IDP.
Why Unlock the Full Potential of IDP?Here are some reasons to maximize the use of intelligent document processing:
Increased Efficiency: Automation through IDP reduces manual work and speeds up document processing.
Improved Accuracy: GenAI algorithms in AI document processing reduce errors that were common with manual data entry, leading to greater accuracy.
Enhanced Compliance: Automated validation in IDP ensures that extracted data meets regulatory requirements, reducing the risk of non-compliance.
Cost Savings: IDP automation lowers operational costs and improves resource allocation, freeing up employees for more valuable tasks.
How to Unlock the Full Potential of IDP?To fully benefit from intelligent document processing, organizations should:
Invest in GenAI-Powered Solutions: Choose IDP solutions that use advanced GenAI algorithms for better performance.
Customize and Train Models: Tailor GenAI models for specific use cases to optimize accuracy and efficiency.
Integrate with Existing Systems: Ensure the IDP solution works seamlessly with the organization's current IT infrastructure to boost adoption and scalability.
Continuous Improvement: Regularly review and refine processes to keep up with changing business needs and technological advancements.
GenAI Reshaping IDPThe introduction of GenAI in document processing has transformed the landscape of intelligent document processing.
Here’s how GenAI has addressed challenges faced by IDP:
Current Challenges in IDP:
Variability in Document Formats: Extracting data from various formats and layouts is challenging.
Language and Handwriting Recognition: Accurately interpreting handwritten and multilingual documents can be difficult.
Data Quality and Consistency: Ensuring consistent and accurate data extraction across all sources is a key challenge for reliable decision-making.
How GenAI Solves IDP Challenges:GenAI has overcome these challenges by:
Advanced Pattern Recognition: GenAI algorithms enhance data extraction accuracy by recognizing patterns across diverse document formats.
Language Understanding: With NLP, GenAI improves language recognition, making it better at interpreting and extracting information from multilingual documents.
Adaptive Learning: GenAI solutions continuously learn from new data, improving data quality and consistency across all document sources.
IDP Use CasesAccording to market analysis, IDP use cases are expected to grow rapidly, reaching around $20 billion by 2033.
Some key use cases include:
Invoice Processing:
GenAI-powered IDP automates invoice data extraction, reducing errors and speeding up processing.
Benefits: Improved financial record accuracy, faster payment processing, and better supplier relationships.
Contract Management:
In the legal industry, IDP accelerates contract review by quickly and accurately analyzing and summarizing legal documents, reducing legal risks and review times.
Benefits: Improved compliance, faster contract reviews, and reduced legal risks.
Customer Onboarding:
GenAI simplifies and speeds up customer onboarding by extracting and verifying information from identity documents.
Benefits: Faster account setup, better customer experience, and reduced risk of identity fraud.
Healthcare Records Management:
GenAI and IDP help healthcare providers extract and digitize patient records for better analysis and decision-making.
Benefits: Faster access to medical information, improved patient care coordination, and enhanced data privacy compliance.
ConclusionIntelligent document processing, boosted by Generative AI, has revolutionized how organizations handle documents.
IDP has made document processing more accurate, efficient, and quicker, leading to greater efficiency in various industries, reducing risks, and improving operations. It also enhances decision-making, allowing human resources to focus on more valuable tasks.
At CrossML, our team of experts offers personalized IDP solutions tailored to your organization’s needs, helping you boost efficiency, improve decision-making, and increase profitability!
0 notes
Text
About/BYF
!!MINORS DNI!! I’m an adult and I post NSFW content sometimes.
Consider going HERE to help people get esims: https://chuffed.org/project/crips-for-esims-for-gaza
Dov, it/its or he/him, over 30, gay as in for men and a fairy. White, disabled, trans, Jewish (of the Bund). This is my art blog and RP hub.
No GenAI has been used nor will be intentionally posted here. If you spot a GenAI post tell me and I’ll delete.
I can and will block or mute people or tags. I may not follow back especially if you argue with people a lot, guilt trip or post IRL gore/crime scenes/etc.
If I reblogged from a bigot or creep by mistake tell me, it’ll be deleted.
RP-specific rules:
As I’m in the World of Darkness fandom, I prefer modern fantasy that’s relatively compatible so not shonen anime/manga/games or anything school themed.
General disclaimer for descriptions of gore, violence, non-graphic talk of torture and cannibalism, sex jokes, non-graphic sex talk, drug use, trauma discussion (non-sexual), basically generalised to adult themes. It’s a “dead dove do not eat” situation. Do not come at me about “bad trans rep” either if you dislike my transmasc characters in particular.
-Don’t be a jerk to me the player in general, including godmodding, accusations of copying or Mary Sues, etc.
-No child characters, no child appearing characters, no vague ages like “teen”, no “barely legal” as a description.
-No deliberate misgendering IC just as with OOC. If you’re playing as a fascist, TERF or tradlifer, you’re not gonna want to be around me anyway.
-I will not ever do any graphic talk about sexual violence, ever. I will also never do pregnancy discussion whatsoever.
-If you genuinely have an issue with something then tell me. Don’t use in game solutions to out of game problems.
Dividers from @diableriedoll
1 note
·
View note
Text

ALERT: AI is entering into law! PwC has partnered with ContractPodAi for legal services!! For individuals who may not be acquainted, ContractPodAi stands as a leading provider of legal AI technology, offering a range of solutions that seamlessly integrate law and technology. Among its offerings, ContractPodAi Cloud emerges as its flagship platform, catering to both legal professionals and non-legal users, facilitating comprehensive end-to-end management of legal documents.
Furthermore, in addition to its offerings, the company presents the Leah Legal Copilot. This innovative Legal GenAI serves as an indispensable tool for professionals, assisting in drafting documents, extracting crucial data, conducting in-depth legal analyses, offering guidance, generating risk and remediation reports, crafting playbooks, and a plethora of other functionalities.
Through this alliance, PwC’s Legal Business Solutions arm will leverage Leah to expand its delivery capacity to help it stand ahead of its competitors in the legal GenAI landscape. By utilizing a cutting-edge AI like Leah, PwC will be implementing Leah internally to effectively complete client work in a faster, better, and more accurate fashion. This move shows PwC’s aggressive investment and adoption of AI. Less than a year ago, PwC announced an investment of $1 billion into AI to enhance its offerings as it announced the launch of an AI tax assistant tool less than a month ago! The Big 4s are joining the AI craze as the race for GenAI is heating up. Will AI affect all industries? Will AI remain a tool or take over actual jobs?? Follow Jobaaj Stories (the media arm of Jobaaj Group) for more.
0 notes
Text
Why Buy When You Can Build Your Enterprise-Worthy GenAI App?
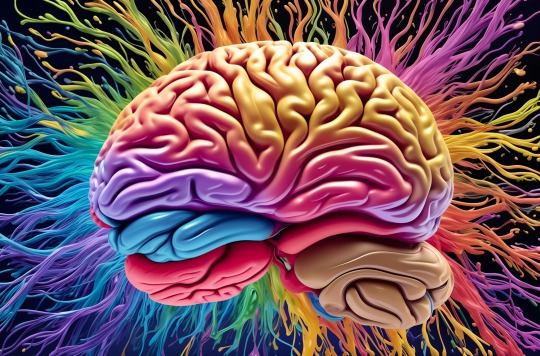
In the dynamic landscape of Generative AI (GenAI), the fervor ignited by OpenAI’s ChatGPT release in November 2022 has given rise to a land rush, with millions of dollars pouring into the development of GenAI applications. The question that echoes through the industry now is whether to embark on the journey of creating a generative AI application from scratch or opt for ready-made solutions.
The DIY Approach: Unleashing GenAI from the Ground Up
The allure of building generative AI applications independently lies in the freedom to tailor them to specific business needs. The capability to incorporate proprietary information into training datasets and customize models to fit industries becomes paramount. SymphonyAI, for instance, has taken this route, creating a common platform that supports industry-specific applications, catering to retail, financial services, industrial, media, and business IT.
The SaaS Alternative: Ready-to-Customise Solutions
On the flip side, the Software as a Service (SaaS) model provides a tempting shortcut for enterprises eager to integrate generative and predictive AI into their operations. Companies like SymphonyAI offer platforms like Eureka, a cloud-agnostic solution that runs on major cloud services or on-premises, providing industry-specific generative AI capabilities. Additionally, the Scale GenAI Platform addresses the challenges of customizing GenAI models at scale. Leveraging the Scale Data Engine, it transforms proprietary data to generate high-quality training data, enabling fine-tuned models for unique use cases. This integrated solution allows organizations to accelerate their generative AI journey and create real business value.
Key Differentiators: DIY vs Buying GenAI
The decision to build a generative AI application in-house or opt for a pre-built solution hinges on factors like cost, customization needs, and time to market. DIY solutions allow for precise tailoring but require significant investment in expertise and infrastructure. Outsourced solutions, on the other hand, offer a quicker route to deployment but may lack the fine-tuned customization that some enterprises require. It’s a trade-off between control and convenience.
Copyright Implications: Navigating Third-Party Models and Data
The integration of third-party data into generative AI applications introduces a crucial dimension of copyright implications and legal considerations. The quality and origin of the data fed into GenAI applications significantly impact their outcomes, and when leveraging third-party models and data, developers must be vigilant about potential copyright issues to ensure compliance and mitigate legal risks.
Risks of Poor-Quality Third-Party Data
The risks associated with low-quality third-party data extend beyond the performance of generative AI applications. Misleading, biased, or inaccurate data can compromise the integrity of AI-generated content and expose organizations to various legal challenges. These risks include intellectual property infringement, misinformation liability, data privacy concerns, and contractual violations.
Mitigating Copyright Risks
To navigate these copyright implications effectively, developers and organizations should adopt a strategic approach involving thorough vetting, licensing agreements, data privacy compliance, continuous monitoring, and legal consultation. While third-party data sources can enrich generative AI applications, developers must tread carefully to avoid legal pitfalls. Balancing innovation with legal compliance is imperative for responsible GenAI development.
Essential Insights for DIY GenAI Builders
For individuals or businesses venturing into building their generative AI applications, several essential considerations come to the forefront. Understanding the nuances of data augmentation, inference, workflows, and post-processing in the context of event-driven GenAI applications is crucial. Embracing event-driven patterns, as outlined in “4 Steps for Building Event-Driven GenAI Applications,” can significantly simplify the development and operational management of Large Language Model (LLM)-driven applications.
Conclusion: Weighing the Pros and Cons
In a landscape buzzing with generative AI innovations, the decision to build or buy depends on organizational priorities, resources, and objectives. Whether crafting a tailored solution in-house or opting for a ready-made SaaS platform, enterprises must carefully evaluate the trade-offs and align their generative AI strategy with long-term business goals. The era of GenAI is upon us, and the choice between building and buying shapes the trajectory of AI integration into diverse industry verticals.
Sources:
https://www.nextplatform.com/2024/02/13/you-can-build-genai-from-scratch-or-go-straight-to-saas/
https://scale.com/blog/genai-platform
https://www.confluent.io/blog/4-steps-for-building-event-driven-genai-applications/
https://www.lexisnexis.com/blogs/ae/b/data-as-a-service/posts/third-party-data-sources-when-using-generative-ai
Related Articles:
NVIDIA GPUs Power Local AI Revolution: Bane for Cloud?
A Call for Comprehensive Regulation and Licensing Regimes in AI Development
AWS Prepares for Massive GenAI Demand with New Ultra-cluster and Diverse Compute Options
This article is re-published from: https://www.scloud.sg/resource/why-buy-when-you-can-build-your-enterprise-worthy-genai-app/
0 notes
Text
7 low code platforms embracing AI
Microsoft’s AI Builder adds AI and machine learning capabilities to Power Apps and Power Automate, while other firms are implementing AI within their low-code and no-code software development and robotic process automation services. AI features are also being added to Amazon SageMaker, DataRobot, Google Cloud AutoML, and Dataiku.
Here are seven low code products adopting AI:
1. Creatio Atlas
Creatio is incorporating a ChatGPT interface into its Creatio Atlas low-code platform and CRM solutions to improve predictive machine learning models. The connector, which uses the GPS-3.5-turbo model API, charges for use cases such as knowledgebase Q&A, content development, language translation, personal assistants, and email production.
2. Mendix AI-Assisted Development
Mendix Assist (AIAD) is a low-code application development platform featuring two virtual co-developer bots: MxAssist Logic Bot and MxAssist Performance Bot. Smart app capabilities are provided by the platform, which includes an AWS service connection and the Mendix ML Kit. The MxAssist Logic Bot walks users through the process of modelling and setting application logic while offering real-time, context-driven actions. While working in Mendix Studio Pro, the MxAssist Performance Bot inspects the app project model against Mendix development best practices to assist in enhancing app performance.
3. OutSystems AI Mentor System
OutSystems AI Mentor System is a collection of AI-powered solutions meant to aid teams across the software development lifecycle. It contains mentors in code, architecture, security, performance, and reliability who help with coding and handle architectural, security, performance, and maintainability concerns. OutSystems also has an Azure ChatGPT connection in their Forge repository, which enables code completions, conversation completions, and machine learning model embeddings. Azure ChatGPT is recommended for personalized suggestions, virtual assistant power, and summarizing and comparing documents such as insurance policies, legal papers, and financial documents.
4. Pega AI and GenAI
Pega AI and Pega GenAI are part of the Pega low-code platform. Pega AI enables “decisioning” through event monitoring, mining of processes, speech-to-text conversion, and natural language processing. It uses decision techniques, machine learning, and adaptive analytics to help you assess facts and actions, forecast consequences, and make decisions in real-time. Pega GenAI develops procedures, phases, and measures. maps integrations to back-end systems, produces test data on the fly and provides conversational guidance to developers.
5. UiPath AI Center
Machine learning has been integrated into the advanced processes of UiPath, a major RPA software. It now has an AI Centre where you can train, evaluate, deploy, and retrain machine learning models for use with RPA. Importing models and employing pre-trained models for image analysis, language analysis, understanding, translation, and tabular data is supported by the AI Centre.
6. Appian Platform
The Appian Platform provides a low-code design experience for process automation, with Appian 23.2 featuring three AI skills: document classification, document extraction, and email classification. The Document Classification AI Skill provides for document classification and routing, whilst the Email Classification AI Skill allows for bespoke machine learning models that classify emails based on business labels. The Document Extraction AI Skill pulls relevant content from structured documents for convenient application use. Appian 23.3 will integrate Appian AI Copilot, a generative AI conversation interface for form design and process modelling.
7. Airtable AI (beta)
Airtable has enhanced its offering, which was previously released alongside OpenAI’s generative foundation models. The AI can be integrated with roadmap apps, marketing apps, and hiring funnel apps to generate product specifications, creative briefs, and job descriptions for available positions.
Conclusion
As we’ve witnessed, most low-code and no-code development and RPA systems now offer AI capabilities, which are frequently based on a version of GPT. More will undoubtedly follow. Microsoft Power Platform is a market leader in this space, thanks in part to its tight partnership with OpenAI and the current AI and machine learning capabilities on Microsoft Azure.
Read More At - https://www.thetechrobot.com/
0 notes
Text
Needle GenAI is a groundbreaking AI framework designed to transform legal practices by enhancing efficiency and accuracy. It addresses common challenges such as inconsistent contract quality and slow drafting processes. With Needle GenAI, legal teams can streamline workflows through features like AI-driven contract drafting, comprehensive legal research, real-time compliance monitoring, and advanced AI chatbots. This innovative solution helps automate routine tasks, accelerate case preparation, and ensure regulatory compliance. By leveraging Needle GenAI, legal professionals can improve the quality of their services, reduce errors, and close cases faster. Discover how Needle GenAI can elevate your legal practice and drive efficiency today.
0 notes
Text
Generative AI adoption: Strategic implications & security concerns - CyberTalk
New Post has been published on https://thedigitalinsider.com/generative-ai-adoption-strategic-implications-security-concerns-cybertalk/
Generative AI adoption: Strategic implications & security concerns - CyberTalk


By Manuel Rodriguez. With more than 15 years of experience in cyber security, Manuel Rodriguez is currently the Security Engineering Manager for the North of Latin America at Check Point Software Technologies, where he leads a team of high-level professionals whose objective is to help organizations and businesses meet cyber security needs. Manuel joined Check Point in 2015 and initially worked as a Security Engineer, covering Central America, where he participated in the development of important projects for multiple clients in the region. He had previously served in leadership roles for various cyber security solution providers in Colombia.
Technology evolves very quickly. We often see innovations that are groundbreaking and have the potential to change the way we live and do business. Although artificial intelligence is not necessarily new, in November of 2022 ChatGPT was released, giving the general public access to a technology we know as Generative Artificial Intelligence (GenAI). It was in a short time from then to the point where people and organizations realized it could help them gain a competitive advantage.
Over the past year, organizational adoption of GenAI has nearly doubled, showing the growing interest in embracing this kind of technology. This surge isn’t a temporary trend; it is a clear indication of the impact GenAI is already having and that it will continue to have in the coming years across various industry sectors.
The surge in adoption
Recent data reveals that 65% of organizations are now regularly using generative AI, with overall AI adoption jumping to 72% this year. This rapid increase shows the growing recognition of GenAI’s potential to drive innovation and efficiency. One analyst firm predicts that by 2026, over 80% of enterprises will be utilizing GenAI APIs or applications, highlighting the importance that businesses are giving to integrating this technology into their strategic frameworks.
Building trust and addressing concerns
Although adoption is increasing very fast in organizations, the percentage of the workforce with access to this kind of technology still relatively low. In a recent survey by Deloitte, it was found that 46% of organizations provide approved Generative AI access to 20% or less of their workforce. When asked for the reason behind this, the main answer was around risk and reward. Aligned with that, 92% of business leaders see moderate to high-risk concerns with GenAI.
As organizations scale their GenAI deployments, concerns increase around data security, quality, and explainability. Addressing these issues is essential to generate confidence among stakeholders and ensure the responsible use of AI technologies.
Data security
The adoption of Generative AI (GenAI) in organizations comes with various data security risks. One of the primary concerns is the unauthorized use of GenAI tools, which can lead to data integrity issues and potential breaches. Shadow GenAI, where employees use unapproved GenAI applications, can lead to data leaks, privacy issues and compliance violations.
Clearly defining the GenAI policy in the organization and having appropriate visibility and control over the shared information through these applications will help organizations mitigate this risk and maintain compliance with security regulations. Additionally, real-time user coaching and training has proven effective in altering user actions and reducing data risks.
Compliance and regulations
Compliance with data privacy regulations is a critical aspect of GenAI adoption. Non-compliance can lead to significant legal and financial repercussions. Organizations must ensure that their GenAI tools and practices adhere to relevant regulations, such as GDPR, HIPPA, CCPA and others.
Visibility, monitoring and reporting are essential for compliance, as they provide the necessary oversight to ensure that GenAI applications are used appropriately. Unauthorized or improper use of GenAI tools can lead to regulatory breaches, making it imperative to have clear policies and governance structures in place. Intellectual property challenges also arise from generating infringing content, which can further complicate compliance efforts.
To address these challenges, organizations should establish a robust framework for GenAI governance. This includes developing a comprehensive AI ethics policy that defines acceptable use cases and categorizes data usage based on organizational roles and functions. Monitoring systems are essential for detecting unauthorized GenAI activities and ensuring compliance with regulations.
Specific regulations for GenAI
Several specific regulations and guidelines have been developed or are in the works to address the unique challenges posed by GenAI. Some of those are more focused on the development of new AI tools while others as the California GenAI Guidelines focused on purchase and use. Examples include:
EU AI Act: This landmark regulation aims to ensure the safe and trustworthy use of AI, including GenAI. It includes provisions for risk assessments, technical documentation standards, and bans on certain high-risk AI applications.
U.S. Executive Order on AI: Issued in October of 2023, this order focuses on the safe, secure, and trustworthy development and use of AI technologies. It mandates that federal agencies implement robust risk management and governance frameworks for AI.
California GenAI Guidelines: The state of California has issued guidelines for the public sector’s procurement and use of GenAI. These guidelines emphasize the importance of training, risk assessment, and compliance with existing data privacy laws.
Department of Energy GenAI Reference Guide: This guide provides best practices for the responsible development and use of GenAI, reflecting the latest federal guidance and executive orders.
Recommendations
To effectively manage the risks associated with GenAI adoption, organizations should consider the following recommendations:
Establish clear policies and training: Develop and enforce clear policies on the approved use of GenAI. Provide comprehensive training sessions on ethical considerations and data protection to ensure that all employees understand the importance of responsible AI usage.
Continuously reassess strategies: Regularly reassess strategies and practices to keep up with technological advancements. This includes updating security measures, conducting comprehensive risk assessments, and evaluating third-party vendors.
Implement advanced GenAI security solutions: Deploy advanced GenAI solutions to ensure data security while maintaining comprehensive visibility into GenAI usage. Traditional DLP solutions based on keywords and patterns are not enough. GenAI solutions should give proper visibility by understanding the context without the need to define complicated data-types. This approach not only protects sensitive information, but also allows for real-time monitoring and control, ensuring that all GenAI activities are transparent and compliant with organizational and regulatory requirements.
Foster a culture of responsible AI usage: Encourage a culture that prioritizes ethical AI practices. Promote cross-department collaboration between IT, legal, and compliance teams to ensure a unified approach to GenAI governance.
Maintain transparency and compliance: Ensure transparency in AI processes and maintain compliance with data privacy regulations. This involves continuous monitoring and reporting, as well as developing incident response plans that account for AI-specific challenges.
By following these recommendations, organizations can make good use and take advantage of the benefits of GenAI while effectively managing the associated data security and compliance risks.
#2022#2023#ai#ai act#AI adoption#AI Ethics#ai tools#America#APIs#applications#approach#artificial#Artificial Intelligence#assessment#automation#Building#Business#ccpa#change#chatGPT#Check Point#Check Point Software#Collaboration#compliance#comprehensive#content#continuous#cyber#cyber security#cybersecurity
0 notes
Text
Discover the Needle GenAI Framework, offering hyper-personalized and holistic solutions with intelligent adaptability. Streamline decision-making with our low-code platform and explore tailored use cases in Finance, Legal, Sales, Marketing, and more. Sign up for a free demo or trial today!
1 note
·
View note
Text
Embracing AI: Hollywood’s Path to a New Era
New Post has been published on https://thedigitalinsider.com/embracing-ai-hollywoods-path-to-a-new-era/
Embracing AI: Hollywood’s Path to a New Era
In Hollywood, where dreams are made and legends are born, a new force is emerging that promises to redefine the landscape of the entertainment industry, generative artificial intelligence. The question on everyone’s mind shouldn’t be so much about the jobs AI might replace, or mundane tasks that GenAI will aid in, but rather about the transformative potential it holds for our industry. This transformation, whether welcome or not, is inevitable. Let’s break it down by dispelling some myths and understanding the opportunities AI brings to the entertainment capital of the world.
Dispelling the Myths: AI is Not the Terminator
It’s easy to get caught up in the dramatic narratives that Hollywood itself has created about AI—visions of sentient robots taking over the world, inspired by blockbusters like “The Terminator.” But let’s ground ourselves in reality. AI, at its core, is mathematics and code. It’s a tool, created by humans, to solve complex problems. Many of the content problems faced in Hollywood stem from legacy technologies and legacy thinking. To solve problems in Hollywood, this means leveraging AI to address challenges in ways we’ve never imagined before.
Asking the Right Questions
Instead of fixating on whether AI will take our jobs, we should be asking: What can AI do for our industry? What are the positive impacts? AI has the potential to revolutionize Hollywood in the same way the internet did in the 90s. It is as significant as the advent of streaming video and as transformative as any technological innovation we’ve witnessed.
The Inevitable Transformation
AI is not just a passing trend; it’s an unstoppable wave of change. Content companies that embrace AI will ride this wave to new heights, while those that resist will find themselves left behind, spending too much on storing content and mundane tasks to compete in a rapidly evolving landscape. Think back to how the peer-to-peer music industry started in the early 2000s. It only took a few years of lawsuits before a company revolutionized legal digital music and made it easily available via an iPod. Fast forward to how we consume music today—digitally on streaming platforms. AI presents a similar crossroads and we must decide whether to adopt it or risk becoming obsolete.
Good vs. Bad: The Battle for AI
Every new technology brings with it a battle between good and bad actors. The early days of the internet and digital music were dominated by piracy and copyright infringement, as bad actors exploited the technology for their gain. All the while, the music industry was focused on suing everyone into submission, instead of looking for solutions that addressed changing consumer demand. It wasn’t until later that legitimate platforms like Spotify and Apple Music emerged, reshaping the music industry with legal, user-friendly solutions. AI will follow a similar path. If Hollywood doesn’t take an active role in shaping the future of AI, we risk leaving the door open for bad actors to exploit the technology, generating unauthorized content and infringing on intellectual property.
On the other hand, embracing AI allows our industry to harness its potential for good. We can use AI to protect our intellectual property (IP), create innovative content, and develop new business models. To achieve this, we need advanced software solutions that help us manage and leverage AI effectively. This proactive approach will ensure that Hollywood remains at the forefront of technological innovation—a place Hollywood hasn’t been known for in the past.
Hollywood’s Reluctance to Change
Hollywood, historically, has been slow to adopt new technologies. The early 2000s saw the music industry resisting digital transformation, only to be overtaken by tech giants who capitalized on the new digital landscape. The same resistance is evident with legacy media beginning to make waves in streaming, and now with a reluctance to fully embrace AI. However, technology will prevail, and Hollywood will have wasted billions trying to sue its way out of this transformation. The entertainment, content, and video industries must come together to shape the future of AI, ensuring it is used to our advantage.
Seizing Opportunities with AI
AI offers numerous opportunities for Hollywood, particularly in areas like localization, metadata management, and content creation. By using AI, we can streamline processes, reduce costs, and improve the quality of our output. For instance, AI can automate the localization process, providing accurate translations and subtitles, thus reaching a global audience more efficiently. Metadata management becomes more precise and comprehensive, allowing for better content organization and discovery.
AI will revolutionize the way we create and distribute content. Imagine AI-driven analytics that predict audience preferences, enabling studios to produce content that resonates more deeply with viewers. AI can also optimize marketing strategies, ensuring that promotional efforts are targeted and effective, and improve their UX for an overall better experience for viewers.
The Revolution Hollywood Needs
Hollywood executives must recognize that AI is a revolution, not just another tool. This revolution demands a shift in mindset from traditional box office-centric views to embracing new forms of content consumption. The way people consume media has changed drastically, but many executives have not adapted accordingly. Clinging on to outdated models will only lead to missed opportunities and business failures. We all remember what happened to Blockbuster.
A Call to Action
Hollywood must not only embrace generative AI but also lead in its development and implementation. This requires collaboration across the industry to ensure that AI is used ethically and effectively. By taking control of AI’s integration into our workflows, we can prevent misuse and harness its full potential to produce higher quality content. This means developing robust software solutions that help manage AI-driven processes, ensuring our data is secure, and our content is protected.
The entertainment industry must invest in AI technologies that enhance creativity and operational efficiency. This involves adopting AI for tasks like automated editing, special effects, and even script writing assistance. Such tools can augment human creativity, allowing artists and creators to focus on what they do best – telling compelling stories.
The Future is Now
As we stand at the cusp of this new era, the choice is clear: embrace AI and transform Hollywood or resist and risk irrelevance. The benefits of AI for the content industry are vast, and the industry must act swiftly to integrate these technologies into our workflows. Just as the internet, digital music and streaming have become integral to our lives, AI will soon be an indispensable part of the entertainment industry.
By proactively shaping the future of AI in entertainment, we ensure GenAI is used for good. We create a future where AI enhances creativity, protects IP, and drives innovation. Hollywood executives should be the ones leading this charge, setting a precedent for other industries to follow.
AI is not the enemy; it is the ally that will propel the entertainment capital of the world into its next golden age. Let’s ride the wave of innovation and lead our industry into a more technologically advanced future. The revolution is here, and Hollywood must be at the forefront, shaping the narrative and seizing the opportunities that AI presents.
#ai#Analytics#apple#approach#artificial#Artificial Intelligence#artists#Born#box#Business#change#code#Collaboration#Companies#comprehensive#content#content creation#copyright#creativity#creators#data#development#Digital Transformation#easy#Editing#effects#efficiency#entertainment#executives#exploit
0 notes
Text
Unlock the power of GenAI with Needle. Build custom AI solutions, connect data sources & gain insights. Boost productivity in Finance, Legal, Sales & more. Free Demo!
#Generative AI framework#Generative AI solution#GenAI Framework#Custom generative AI solutions#RAG as a services
1 note
·
View note
Text
Are RAGs the Solution to AI Hallucinations?
New Post has been published on https://thedigitalinsider.com/are-rags-the-solution-to-ai-hallucinations/
Are RAGs the Solution to AI Hallucinations?
AI, by design, has a “mind of its own.” One drawback of this is that Generative AI models will occasionally fabricate information in a phenomenon called “AI Hallucinations,” one of the earliest examples of which came into the spotlight when a New York judge reprimanded lawyers for using a ChatGPT-penned legal brief that referenced non-existent court cases. More recently, there have been incidents of AI-generated search engines telling users to consume rocks for health benefits, or to use non-toxic glue to help cheese stick to pizza.
As GenAI becomes increasingly ubiquitous, it is important for adopters to recognize that hallucinations are, as of now, an inevitable aspect of GenAI solutions. Built on large language models (LLMs), these solutions are often informed by vast amounts of disparate sources that are likely to contain at least some inaccurate or outdated information – these fabricated answers make up between 3% and 10% of AI chatbot-generated responses to user prompts. In light of AI’s “black box” nature – in which as humans, we have extraordinary difficulty in examining just exactly how AI generates its results, – these hallucinations can be near impossible for developers to trace and understand.
Inevitable or not, AI hallucinations are frustrating at best, dangerous, and unethical at worst.
Across multiple sectors, including healthcare, finance, and public safety, the ramifications of hallucinations include everything from spreading misinformation and compromising sensitive data to even life-threatening mishaps. If hallucinations continue to go unchecked, the well-being of users and societal trust in AI systems will both be compromised.
As such, it is imperative that the stewards of this powerful tech recognize and address the risks of AI hallucinations in order to ensure the credibility of LLM-generated outputs.
RAGs as a Starting Point to Solving Hallucinations
One method that has risen to the fore in mitigating hallucinations is retrieval-augmented generation, or RAG. This solution enhances LLM reliability through the integration of external stores of information – extracting relevant information from a trusted database chosen according to the nature of the query – to ensure more reliable responses to specific queries.
Some industry experts have posited that RAG alone can solve hallucinations. But RAG-integrated databases can still include outdated data, which could generate false or misleading information. In certain cases, the integration of external data through RAGs may even increase the likelihood of hallucinations in large language models: If an AI model relies disproportionately on an outdated database that it perceives as being fully up-to-date, the extent of the hallucinations may become even more severe.
AI Guardrails – Bridging RAG’s Gaps
As you can see, RAGs do hold promise for mitigating AI hallucinations. However, industries and businesses turning to these solutions must also understand their inherent limitations. Indeed, when used in tandem with RAGs, there are complementary methodologies that should be used when addressing LLM hallucinations.
For example, businesses can employ real-time AI guardrails to secure LLM responses and mitigate AI hallucinations. Guardrails act as a net that vets all LLM outputs for fabricated, profane, or off-topic content before it reaches users. This proactive middleware approach ensures the reliability and relevance of retrieval in RAG systems, ultimately boosting trust amongst users, and ensuring safe interactions that align with a company’s brand.
Alternatively, there’s the “prompt engineering” approach, which requires the engineer to change the backend master prompt. By adding pre-determined constraints to acceptable prompts – in other words, monitoring not just where the LLM is getting information but how users are asking it for answers as well – engineered prompts can guide LLMs toward more dependable results. The main downside of this approach is that this type of prompt engineering can be an incredibly time-consuming task for programmers, who are often already stretched for time and resources.
The “fine tuning” approach involves training LLMs on specialized datasets to refine performance and mitigate the risk of hallucinations. This method trains task-specialized LLMs to pull from specific, trusted domains, improving accuracy and reliability in output.
It is also important to consider the impact of input length on the reasoning performance of LLMs – indeed, many users tend to think that the more extensive and parameter-filled their prompt is, the more accurate the outputs will be. However, one recent study revealed that the accuracy of LLM outputs actually decreases as input length increases. Consequently, increasing the number of guidelines assigned to any given prompt does not guarantee consistent reliability in generating dependable generative AI applications.
This phenomenon, known as prompt overloading, highlights the inherent risks of overly complex prompt designs – the more broadly a prompt is phrased, the more doors are opened to inaccurate information and hallucinations as the LLM scrambles to fulfill every parameter.
Prompt engineering requires constant updates and fine-tuning and still struggles to prevent hallucinations or nonsensical responses effectively. Guardrails, on the other hand, won’t create additional risk of fabricated outputs, making them an attractive option for protecting AI. Unlike prompt engineering, guardrails offer an all-encompassing real-time solution that ensures generative AI will only create outputs from within predefined boundaries.
While not a solution on its own, user feedback can also help mitigate hallucinations with actions like upvotes and downvotes helping refine models, enhance output accuracy, and lower the risk of hallucinations.
On their own, RAG solutions require extensive experimentation to achieve accurate results. But when paired with fine-tuning, prompt engineering, and guardrails, they can offer more targeted and efficient solutions for addressing hallucinations. Exploring these complimentary strategies will continue to improve hallucination mitigation in LLMs, aiding in the development of more reliable and trustworthy models across various applications.
RAGs are Not the Solution to AI Hallucinations
RAG solutions add immense value to LLMs by enriching them with external knowledge. But with so much still unknown about generative AI, hallucinations remain an inherent challenge. The key to combating them lies not in trying to eliminate them, but rather by alleviating their influence with a combination of strategic guardrails, vetting processes, and finetuned prompts.
The more we can trust what GenAI tells us, the more effectively and efficiently we’ll be able to leverage its powerful potential.
#ai#AI Chatbot#AI hallucinations#AI halucinations#ai model#AI models#AI systems#applications#approach#black box#box#challenge#change#chatbot#chatGPT#content#court#data#Database#databases#datasets#Design#developers#development#domains#Engineer#engineering#engines#finance#Fine Tuning
0 notes