#data mapping
Explore tagged Tumblr posts
Text

Twenty-six days at sea; more than 49,000 square kilometers (18,919 square miles) of seafloor mapped; 5,579 linear nautical miles (6,420 linear miles) traversed… and 13,580 linked double crochet stitches stitched. By Jesse Gwinn, Internship Program Coordinator, NOAA Ocean Exploration
35 notes
·
View notes
Text
0 notes
Text



4 more maps in regards to the topic of Mutants. Been quite busy with things though so as far as everything else is concerned there’s nothing else much to tell. It’s rather intricate of a topic, but as far as other things go there’s a lot still needed to be mapped out as far as the eye can see.
#Mutants#mutant mappings#mutarative#Mutants of the world#Mutants mappings of variant#mutants mapping data#Data mapping#mapping#maps of data#maps of mutants#4 maps of mutalitarative
0 notes
Text
The Importance of Data Mapping in the World of Data Science | Infographic
Data management is an important aspect of today’s data-driven businesses and data mapping constitutes an important part of it. The data management industry is growing at a CAGR of 11.2% to reach a market size of $281.9 billion by 2028, and data mapping will have an important role to play.
In simple terms, it refers to the process of creating element mappings between two distinct data models i.e., it ensures that data from a source system is accurately and appropriately translated to a target system that maintains the integrity and consistency of data.
Practically, it defines how individual fields in the source data correspond to fields in the destination data. The entire process can either be straightforward involving direct field-to-field mappings or complex where transformation rules, lookups, and aggregations are required.
For example, in simple mapping “first_name” field in source data is linked to the corresponding field “given_name” in the target database. Whereas in more complex scenarios, it might include linking “first_name” and “last_name” into a single “full_name” field.
Well, data mapping can be quite an interesting skill to learn and it offers several benefits to businesses such as ensuring:
Data quality
Consistency
Integration
Compliance, etc.
If you are interested in a career in data science, knowledge of data mapping is a must. So, check out our detailed infographic on data mapping and its importance in the world of data science now.

0 notes
Text
Transformative Potential of a Healthcare-Specific Foundational Model
New Post has been published on https://thedigitalinsider.com/transformative-potential-of-a-healthcare-specific-foundational-model/
Transformative Potential of a Healthcare-Specific Foundational Model
In the past two years, generalist foundational models like GPT-4 have significantly evolved, offering unprecedented capabilities due to larger datasets, increased model sizes, and architectural improvements. These models are adaptable to a wide range of tasks across various fields. However, healthcare AI is still characterized by models designed for specific tasks. For instance, a model trained to analyze X-rays for bone fractures would only identify fractures and lack the capability to generate comprehensive radiology reports. Most of the 500 AI models approved by the Food and Drug Administration are limited to one or two use cases. However, foundation models, known for their broad applicability across different tasks, are setting the stage for a transformative approach in healthcare applications.
While there have been initial attempts to develop foundational models for medical applications, this broader approach has not yet become prevalent in healthcare AI. This slow adoption is mainly due to the challenges associated with accessing large and diverse healthcare datasets, as well as the need for models to reason across different types of medical data. The practice of healthcare is inherently multimodal and incorporates information from images, electronic health records (EHRs), sensors, wearables, genomics, and more. Thus, a foundational healthcare model must also be inherently multimodal. Nonetheless, recent progress in multimodal architectures and self-supervised learning, which can handle various data types without needing labeled data, is paving the way for a healthcare foundational model.
Current State of Generative AI in Healthcare
Healthcare has traditionally been slow to adopt technology, however, it seems to have embraced Generative AI more swiftly. At HIMSS24, the largest global conference for healthcare technology professionals, Generative AI was the focal point of nearly every presentation.
One of the first use cases of Generative AI in healthcare that has seen widespread adoption focuses on alleviating the administrative load of clinical documentation. Traditionally, documenting patient interactions and care processes consumes a substantial portion of physicians’ time (>2 hrs. per day), often detracting them from direct patient care.
AI models like GPT-4 or MedPalm-2 are being used to monitor patient data and physician-patient interactions to draft key documents such as progress notes, discharge summaries, and referral letters. These drafts capture essential information accurately, requiring only physician review and approval. This significantly reduces paperwork time, allowing physicians to focus more on patient care, enhancing quality of service and reducing burnout.
However, the broader applications of foundational models in healthcare have yet to fully materialize. Generalist foundational models like GPT-4 have several limitations; thus, there is a need for a healthcare-specific foundational model. For example, GPT-4 lacks the capability to analyze medical images or understand longitudinal patient data, which is critical for providing accurate diagnoses. Additionally, it does not possess the most up-to-date medical knowledge, as it was trained on data available only up to December 2023. Google’s MedPalm-2 represents the first attempt to build a healthcare-specific foundational model, capable of both answering medical queries and reasoning about medical images. However, it still doesn’t capture full potential of AI in healthcare.
Building a Healthcare Foundational Model
The process of building a healthcare foundational model begins with data derived from both public and private sources, including biobanks, experimental data, and patient records. This model would be capable of processing and combining different data types, such as text with images or laboratory results, to perform complex medical tasks.
Additionally, it could reason about new situations and articulate its outputs in medically precise language. This capability extends to inferring and utilizing causal relationships between medical concepts and clinical data, especially when providing treatment recommendations based on observational data. For instance, it could predict acute respiratory distress syndrome from recent severe thoracic trauma and declining arterial oxygen levels, despite an increased oxygen supply.
Furthermore, the model would access contextual information from resources like knowledge graphs or databases to obtain up-to-date medical knowledge, enhancing its reasoning and ensuring that its advice reflects the latest advancements in medicine
Applications and Impact of Healthcare Foundational Model
The potential uses for a healthcare foundational model are extensive. In diagnostics, such a model could reduce the dependence on human analysis. For treatment planning, the model could aid in crafting individualized treatment strategies by considering a patient’s entire medical record, genetic details, and lifestyle factors. Some other applications include:
Grounded radiology reports: The healthcare foundational model can transform digital radiology by creating versatile assistants that support radiologists by automating report drafting and reducing workload. It would also be able to integrate entire patient history. For instance, radiologists can query the model about changes in conditions over time: “Can you identify any changes in the tumor size since the last scan?”
Bedside Clinical Decision Support: Leveraging clinical knowledge, it would offer clear, free-text explanations and data summaries, alerting medical staff to immediate patient risks and suggesting next steps. For example, the model cloud alert, “Warning: This patient is about to go into shock,” and provide links to relevant data summaries and checklists for action.
Drug Discovery: Designing proteins that bind specifically and strongly to a target is the foundation of drug discovery. Early models like RFdiffusion have begun to generate proteins based on basic inputs such as a target for binding. Building on these initial models, a healthcare-specific foundational model could be trained to understand both language and protein sequences. This would allow it to offer a text-based interface for designing proteins, potentially speeding up the development of new drugs
Challenges
Although building a healthcare-specific foundational model remains the ultimate goal, and recent advancements have made it more feasible, there are still significant challenges in developing a single model capable of reasoning across diverse medical concepts:
Data mapping multiple modalities: The model must be trained on various data modalities such as EHR data, medical imaging data, and genetic data. Reasoning across these modalities is challenging because sourcing high-fidelity data that accurately maps interactions across all these modalities is difficult. Moreover, representing various biological modalities, from cellular dynamics to molecular structures and genome-wide genetic interactions, is complex. Optimal training on human data is unfeasible and unethical, so researchers rely on less predictive animal models or cell lines, which creates a challenge in translating laboratory measurements to the intricate workings of whole organisms.
Validation and Verification: Healthcare foundational models are challenging to validate due to their versatility. Traditionally, AI models are validated for specific tasks like diagnosing a type of cancer from an MRI. However, foundational models can perform new, unseen tasks, making it hard to anticipate all possible failure modes. They require detailed explanations of their testing and approved use cases and should issue warnings for off-label use. Verifying their outputs is also complex, as they handle diverse inputs and outputs, potentially requiring a multidisciplinary panel to ensure accuracy.
Social Biases: These models risk perpetuating biases, as they may train on data that underrepresents certain groups or contains biased correlations. Addressing these biases is crucial, particularly as the scale of models increases, which can intensify the problem.
Path Forward
Generative AI has already begun to reshape healthcare by alleviating the documentation burden on clinicians, but its full potential lies ahead. The future of foundational models in healthcare promises to be transformative. Imagine a healthcare system where diagnostics are not only faster but also more accurate, where treatment plans are precisely tailored to the genetic profiles of individual patients, and where new drugs could be discovered in a few months rather than years.
Creating a healthcare-specific foundational AI model presents challenges, especially when it comes to integrating the diverse and scattered medical and clinical data. However, these obstacles can be addressed through collaborative efforts among technologists, clinicians, and policymakers. By working together, we can develop commercial frameworks that incentivize various stakeholders (EHRs, imaging companies, pathology labs, providers) to unify this data and construct AI model architectures capable of processing complex, multimodal interactions within healthcare.
Moreover, it is crucial that this advancement proceeds with a clear ethical compass and robust regulatory frameworks to ensure that these technologies are used responsibly and equitably. By maintaining high standards of validation and fairness, the healthcare community can build trust and foster acceptance among both patients and practitioners.
The journey toward fully realizing the potential of healthcare foundational models is an exciting frontier. By embracing this innovative spirit, the healthcare sector can anticipate not just meeting current challenges but transform medical science. We are on the brink of a bold new era in healthcare—one brimming with possibilities and driven by the promise of AI to improve lives on a global scale.
#2023#Administration#Advice#ai#AI in healthcare#ai model#AI models#Analysis#applications#approach#biobanks#Building#burnout#Cancer#Capture#cell#cell lines#challenge#Cloud#collaborative#Community#Companies#compass#comprehensive#conference#data#data mapping#databases#datasets#december
0 notes
Text
Data validation is a critical aspect of ensuring the accuracy and integrity of data within various systems and processes. It involves the verification and validation of data to guarantee its reliability and usability. Imagine data validation as the gatekeeper that checks every piece of information trying to enter the system, ensuring only the correct and accurate data gets through. Just like a security checkpoint at an airport, data validation filters out the unwanted and potentially harmful data, allowing only the safe and valid data to pass through.
One of the key challenges in data validation is the sheer volume of data that needs to be processed. With the exponential growth of data in today's digital age, manual validation processes are no longer feasible. This is where automated data validation tools and techniques come into play. These tools streamline the validation process, making it faster, more efficient, and less prone to human error. It's like having a team of tireless workers who meticulously examine every piece of data, ensuring its accuracy and quality without getting tired or making mistakes.
Ensuring data accuracy is not just about maintaining the quality of information but also about upholding the credibility and trustworthiness of the entire system. Inaccurate data can lead to faulty decisions, flawed analysis, and ultimately, disastrous outcomes. Just like a single wrong turn can lead to getting lost in a maze, one incorrect piece of data can derail an entire process or system. Data validation acts as a compass, guiding the system in the right direction by ensuring that the data it relies on is accurate, reliable, and consistent.
Data validation is not a one-time task but an ongoing process that requires continuous monitoring and maintenance. As data evolves and changes over time, so do the validation requirements. Regular data validation checks are essential to identify and rectify any discrepancies, anomalies, or errors in the data. It's like regularly servicing your car to ensure it runs smoothly and efficiently. By proactively validating data on a regular basis, organizations can prevent data quality issues before they escalate into major problems.
0 notes
Text
Revolutionizing Revenue Recognition: The Power of Automation
The answer lies in automating the decision-making process itself.
Revenue accounting automation involves pre-defining rules based on policies and desired outcomes. These rules can then be applied directly to data sourced from sales contracts and various systems capturing orders, fulfillment, and billing. The result? Precise revenue calculations and forecast schedules over the contract term.
So, how does it actually work?
Imagine a revenue analyst reviewing a contract to identify critical components for revenue recognition. Similarly, automation software can be configured to identify these components through data mapping—things like contract number, customer name, contract term, deliverables, and pricing details.
Instead of relying on cumbersome spreadsheets, an automated revenue sub-ledger takes on the task of aggregating data, applying rules, and recognizing revenue based on predefined criteria.
Think of it as building a roadmap for revenue recognition—a set of rules and guidelines that automate the process from start to finish.
#Revenue Recognition#Automation#Accounting Automation#Revenue Accounting#Decision-Making Automation#Contract Management#Data Mapping#Forecasting#Rules-Based Automation#Financial Software
0 notes
Text
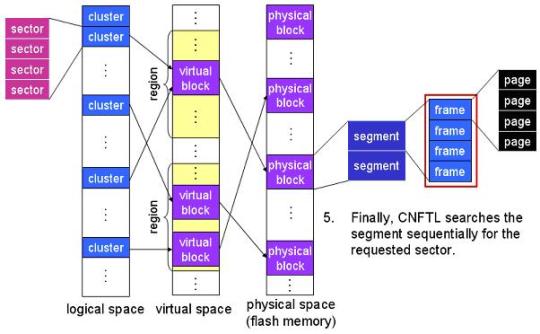
#credit:Flashlab#data mapping#segment#virtual space#logical space#physical space#flash memory#sector#hardware to software#address space#address mapping#hardware#software
0 notes
Text
Cartografías de Agua / Río Limarí
Ejercicio realizado para taller de arquitectura.
Realizado junto a Pablo Martínez K.
Provincia de Limarí, Región de Coquimbo, Chile / Septiembre 2019
0 notes
Text
Privacy Lock for global Compliance
Penta Privacy Lock provides one solution for global privacy compliance. Click and comply with GDPR, CCPA, and other privacy laws using Privacy Lock's automation services. And the best part is Privacy Lock never collects, stores, or processes any personal information about your consumers. Protect your business and your customers with Privacy Lock today. click here to learn more about Privacy Lock: https://blog.pentaprivacylock.com/are-new-data-privacy-laws-causing-headaches-for-your-business/
1 note
·
View note
Text
Same-sex marriage in 2003 vs. 2013 vs. 2023
(20 years of change)
More info below:
----
2003:
Marriage : Netherlands, Belgium, British Columbia (CA), Ontario (CA)
Civil unions : France (including overseas territories), Germany, Denmark, Iceland, Sweden, Norway, Finland, Greenland, Rio Negro (AR), Ciudad de Buenos Aires (AR), California (US), New York (US), Hawaii (US), Vermont (US), Canary Islands (ES), Aragon (ES), Catalonia (ES), Andalusia (ES), Extremadura (ES), Castilla-La Mancha (ES), Castilla-Leon (ES), Madrid (ES), Valencia (ES), Asturias (ES), Basque Country (ES), Navarre (ES), Balearics (ES), Quebec (CA), Alberta (CA), Manitoba (CA), Nova Scotia (CA), Geneva (CH), Zurich (CH), Portugal.
----
2013:
Marriage : Netherlands, Belgium, Canada, Brazil, Uruguay, Argentina, South Africa, Spain, Portugal, France (including overseas territories), Denmark, Norway, Sweden, Iceland, New Zealand, Washington (US), California (US), New Mexico (US), Minnesota (US), Iowa (US), Maryland (US), DC (US), New Jersey (US), Delaware (US), New York (US), Connecticut (US), Rhode Island (US), Vermont (US), Massachusetts (US), New Hampshire (US), Maine (US), Hawaii (US), Mexico City (MX), Quintana Roo (MX).
Civil unions : Greenland, Colombia, Ecuador, Merida (VZ), United Kingdom, Ireland, Germany, Czech Republic, Austria, Finland, Hungary, Slovenia, Croatia, Switzerland, Luxembourg, Liechtenstein, Australia
Recognizes marriages performed abroad : All 32 Mexican states and Israel
----
2023:
Marriage : Netherlands (including overseas territories), Belgium, United States, Canada, Mexico, Costa Rica, Cuba, Puerto Rico, Colombia, US Virgin Islands, Ecuador, Brazil, Argentina, Uruguay, Chile, Malvinas/Falklands, France (including overseas territories), Spain, Portugal, Andorra, Germany, Slovenia, Switzerland, Austria, Malta, Guernsey, Jersey, United Kingdom, Isle of Man, Ireland, Gibraltar, Norway, Denmark, Sweden, Finland, Iceland, Greenland, Luxembourg, Faroe Islands, South Africa, Australia, New Zealand, Taiwan, Northern Mariana Islands, Guam, St. Helena, Pitcairn Islands, Gibraltar.
Civil unions : Bolivia, Italy, Cayman Islands, Bermuda, Aruba, Curaçao, Czech Republic, Hungary, Croatia, Montenegro, Greece, Cyprus, Estonia, Liechtenstein
Recognizes marriages performed abroad : Namibia, Israel, Nepal, American Samoa
----
Future :
Same-sex marriage is under consideration by the legislature or the courts in Aruba, Curaçao, the Czech Republic, Estonia, Greece, India, Japan, Liechtenstein, Namibia, the Navajo Nation, Nepal, Thailand, and Venezuela, and all countries bound by the Inter-American Court of Human Rights (IACHR), which includes Barbados, Bolivia, Dominican Republic, El Salvador, Guatemala, Haiti, Honduras, Nicaragua, Panama, Paraguay, Peru, and Suriname.
Civil unions are being considered in a number of countries, including Lithuania, Peru, the Philippines, South Korea, Ukraine, China, Hong Kong, Japan, Latvia, Panama, Romania, Serbia, Slovakia, Thailand, and Venezuela.
----
#map#maps#cartography#usa#latin america#mexico#data#americas#geography#europe#gay#lgbt#gay marriage#lgbtq#lgbtq history#lgbt history#history#lesbian#sapphic#marriage#pride
7K notes
·
View notes
Text
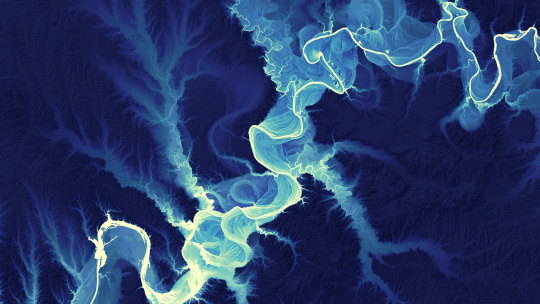


Lidar-Derived Aerial Maps Reveal the Dramatic Meandering Changes in River Banks Over Millennia
5K notes
·
View notes
Text
Transgender Friendly States
This is a map made by journalist Erin Reed (https://www.erininthemorning.com/). It shows the legislative risk to trans people by state. It's been going around tumblr recently, and for good reason.
I wanted to make a post comparing the type of information you might need if you're considering making a move following the election. The following includes the cost of living index, the climate risk, the median rent, the minimum wage, and the shift in voting pattern between the 2020 and 2024 election for each "Safest States with Strong Protections" (dark blue on map above).
State: California
*Cost of Living: 134.5% of US average
Climate Risk: Moderate
Median Rent: $1,856
Minimum Wage: $16/hr (116 hours to make rent)
2020vs2024 Politics: Blue, went 8 points redder
State: Colorado
*Cost of Living: 105.5% of US average
Climate Risk: Low
Median Rent: $1,594
Minimum Wage: $14.42/hr (110.5 hours to make rent)
2020vs2024 Politics: Blue, went 2 points redder
State: Connecticut
*Cost of Living: 113.1% of US average
Climate Risk: Low
Median Rent: $1,374
Minimum Wage: $15.69 (87.5 hours to make rent)
2020vs2024 Politics: Blue, went 5 points redder
State: Illinois
*Cost of Living: 90.8% of US average
Climate Risk: Moderate
Median Rent: $1,179
Minimum Wage: $14/hr (84 hours to make rent)
2020vs2024 Politics: Blue, went 7 points redder
State: Hawaii
*Cost of Living: 179% of US average
Climate Risk: Low
Median Rent: $1,868
Minimum Wage: $14/hr (133 hours to make rent)
2020vs2024 Politics: Blue, went 6 points redder
State: Maine
*Cost of Living: 111.5% of US average
Climate Risk: Low
Median Rent: $1,009
Minimum Wage: $14.15/hr (71 hours to make rent)
2020vs2024 Politics: Blue, went 2 points redder
State: Maryland
*Cost of Living: 119.5% of US average
Climate Risk: Low
Median Rent: $1,598
Minimum Wage: $15/hr (106 hours to make rent)
2020vs2024 Politics: Blue, went 6 points redder
State: Massachusetts
*Cost of Living: 148.4% of US average
Climate Risk: Moderate
Median Rent: $1,588
Minimum Wage: $15/hr (106 hours to make rent)
2020vs2024 Politics: Blue, went 8 points redder
State: Minnesota
*Cost of Living: 94.1% of US average
Climate Risk: Low
Median Rent: $1,178
Minimum Wage: $10.85/hr (108 hours to make rent)
2020vs2024 Politics: Blue, went 3 points redder
State: New Jersey
*Cost of Living: 114.1% of US average
Climate Risk: Moderate
Median Rent: $1,577
Minimum Wage: $15.13/hr (104 hours to make rent)
2020vs2024 Politics: Blue, went 10 points redder
State: New Mexico
*Cost of Living: 94.2% of US average
Climate Risk: High
Median Rent: $966
Minimum Wage: $12/hr (80.5 hours to make rent)
2020vs2024 Politics: Blue, went 5 points redder
State: New York
*Cost of Living: 125.1% of US average
Climate Risk: Moderate
Median Rent: $1,507
Minimum Wage: $15/hr (16/hr for NYC, Long Island, and Westchester), (100.5 hours to make rent)
2020vs2024 Politics: Blue, went 11 points redder
State: Oregon
*Cost of Living: 115.1% of US average
Climate Risk: Low
Median Rent: $1,373
Minimum Wage: $16.70/hr (but it's complicated) (82 hours to make rent)
2020vs2024 Politics: Blue, went 2 points redder
State: Rhode Island
*Cost of Living: 110.5% of US average
Climate Risk: Moderate
Median Rent: $1,195
Minimum Wage: $14/hr (85 hours to make rent)
2020vs2024 Politics: Blue, went 7 points redder
State: Vermont
*Cost of Living: 114.9% of US average
Climate Risk: Low
Median Rent: $1,149
Minimum Wage: $13.67 (84 hours to make rent)
2020vs2024 Politics: Blue, went 3 points redder
State: Washington
*Cost of Living: 115.1% of US average
Climate Risk: Low
Median Rent: $1,592
Minimum Wage: $16.28 (97 hours to make rent)
2020vs2024 Politics: Blue, went 0 points redder
*On this scale, 100% is the average COL across the USA. Numbers above 100% mean the state is more expensive than average. Numbers below 100% mean the state is less expensive to live in than average. Pennsylvania, Utah, and Nevada are the states closest to average. Hawaii, at 179% is the most expensive state. Mississippi, at 85.3% is the least expensive state to live in.
References: COL CR MR MW POL
168 notes
·
View notes
Text
So there is a post going around with a realy suspicious map, and OP of the post has me blocked so here is a link to their post since I cannot reblog it.
Okay so, I looked into this as from my own experience the map looked suspicious.
The orignal post of the map has been deleted. You can find the deleted post on reddit about it here.
I managed to find a repost of the map on tumblr, which had linked to the deleted reddit post, and you can find the repost of the orignal map here. Now onto debunking OP's claim of the map.
The title of the map is not solely about the west bank, it is about full john oliver episodes being unavailable in countries. Not just the west bank episode, all full episodes.
When I try to watch the video, i get the below error from youtube
Yep your eyes do see correctly. It is the uploader who made it unavailable. This means that either John Oliver himself, his production team or HBO who has the rights to his show, decided to make his content not available to be viewed in certain countries.
A comment on the reddit post which I think people should read is
"The title of this post makes many people think OP means ‘countries that have banned this’. Instead, this is just ‘countries where a network pays for the broadcast rights for this show’. The highlighted countries are ones where the show is most popular. The blank countries are where it’s not popular, so they give it away for free to try to drum up support"
Aka this is not purposeful censorship by (((zionists)) like tumblr op is trying to frame it. Its not keeping what happens in the west bank a secret. It is a decision made by someone in charge of the show, to make as much money as possible. Which still sucks! But, it sucks for different reasons than what tumblr OP is alluding to.
3. Tumblr OP is being antisemitic. Whilst it is definately written in a more subtle way, it is still invoking the whole "jews control the media" trope. A well known antisemitic trope is still antisemitic even if "jew" is replaced with "zionist". This is because whilst not every use of "zionist" is a proxy word of jew, it happens more than you think, to the point where meta (instagram and facebook) updated their hate speech policy to puroposefully include "zionist when its being used as proxy (aka dogwhistle) for jew is now hate speech on our platform". Meta still allows it to be used when talking about zionism as a political ideology, and have only banned the use of it as a proxy for jew.
OP of this post has already blocked me (only found out when i saw this post), so I wanna thank @coffeeconcentrate for reblogging this and not having me blocked so I can actually have a chance at reaching even a small group of people to make them aware of this so they don't fall for this poorly made post.
#whenever you see a map or graph which lacks both of those 9/10 its because the data doesn't fit the narrative they're trying to push#antisemitism#jumblr#jewblr#disinformation
200 notes
·
View notes