#answer engine
Explore tagged Tumblr posts
Text
AEO SEO EXPERT - AI MARKETING
Predicting the future of terminology is tricky, but whether "Answer Engine Optimization" (AEO) will completely replace "Search Engine Optimization" (SEO) is a topic with interesting factors to consider:
Arguments for AEO becoming dominant:
Shifting search landscape: Search engines are increasingly using AI and advanced algorithms to understand user intent and deliver direct answers on the SERP, aligning with AEO's focus.
Focus on user experience: AEO emphasizes providing concise and relevant answers, directly addressing user needs, potentially resonating more with user-centric future models.
Emerging niche: "AEO" is newer and might attract companies focused on the latest trends and user-centric approaches.
Arguments for SEO remaining prevalent:
Established term: "SEO" is deeply ingrained in the industry, with countless resources, tools, and professionals using it. Changing terminology entirely would be disruptive and costly.
Broader scope: SEO encompasses various areas beyond direct answers, including technical optimization, link building, and content strategy, which AEO might not fully capture.
Gradual evolution: Search engines and optimization practices are constantly evolving, and SEO might adapt to include the focus on direct answers without requiring a complete name change.
Likely scenario:
It's more likely that SEO will evolve to encompass AEO principles rather than be entirely replaced. The term "SEO" might gain modifiers like "AEO-focused SEO" or "conversational SEO" to reflect the changing landscape. Ultimately, the industry will adopt the terminology that best reflects the current practices and resonates with users and professionals.
So, while AEO might gain traction as a sub-category or specialized area, it's unlikely to completely dethrone the established and broader term "SEO" in the foreseeable future. Both terms might co-exist, reflecting the multifaceted nature of search engine optimization in a dynamic digital landscape.
AEO EXPERT / SEO Predrag Petrovic

DIZAJN SAJTA BEOGRAD SRBIJA
#seo#AI#AI MARKETING#LINKEDIN#SEO EXPERT#answer engine#answerengineoptimization#AEO Expert#선생#SEONSAENG#SEO STRATEGIST#EMEA SEO#SEO NA KVADRAT#REAL ESTATE SEO EXPERT#SEO²#SEO² EXPERT#SEO² STRATEGIST#SEO² SCIENTIST
2 notes
·
View notes
Text
New Research Finds Sixteen Major Problems With RAG Systems, Including Perplexity
New Post has been published on https://thedigitalinsider.com/new-research-finds-sixteen-major-problems-with-rag-systems-including-perplexity/
New Research Finds Sixteen Major Problems With RAG Systems, Including Perplexity
A recent study from the US has found that the real-world performance of popular Retrieval Augmented Generation (RAG) research systems such as Perplexity and Bing Copilot falls far short of both the marketing hype and popular adoption that has garnered headlines over the last 12 months.
The project, which involved extensive survey participation featuring 21 expert voices, found no less than 16 areas in which the studied RAG systems (You Chat, Bing Copilot and Perplexity) produced cause for concern:
1: A lack of objective detail in the generated answers, with generic summaries and scant contextual depth or nuance.
2. Reinforcement of perceived user bias, where a RAG engine frequently fails to present a range of viewpoints, but instead infers and reinforces user bias, based on the way that the user phrases a question.
3. Overly confident language, particularly in subjective responses that cannot be empirically established, which can lead users to trust the answer more than it deserves.
4: Simplistic language and a lack of critical thinking and creativity, where responses effectively patronize the user with ‘dumbed-down’ and ‘agreeable’ information, instead of thought-through cogitation and analysis.
5: Misattributing and mis-citing sources, where the answer engine uses cited sources that do not support its response/s, fostering the illusion of credibility.
6: Cherry-picking information from inferred context, where the RAG agent appears to be seeking answers that support its generated contention and its estimation of what the user wants to hear, instead of basing its answers on objective analysis of reliable sources (possibly indicating a conflict between the system’s ‘baked’ LLM data and the data that it obtains on-the-fly from the internet in response to a query).
7: Omitting citations that support statements, where source material for responses is absent.
8: Providing no logical schema for its responses, where users cannot question why the system prioritized certain sources over other sources.
9: Limited number of sources, where most RAG systems typically provide around three supporting sources for a statement, even where a greater diversity of sources would be applicable.
10: Orphaned sources, where data from all or some of the system’s supporting citations is not actually included in the answer.
11: Use of unreliable sources, where the system appears to have preferred a source that is popular (i.e., in SEO terms) rather than factually correct.
12: Redundant sources, where the system presents multiple citations in which the source papers are essentially the same in content.
13: Unfiltered sources, where the system offers the user no way to evaluate or filter the offered citations, forcing users to take the selection criteria on trust.
14: Lack of interactivity or explorability, wherein several of the user-study participants were frustrated that RAG systems did not ask clarifying questions, but assumed user-intent from the first query.
15: The need for external verification, where users feel compelled to perform independent verification of the supplied response/s, largely removing the supposed convenience of RAG as a ‘replacement for search’.
16: Use of academic citation methods, such as [1] or [34]; this is standard practice in scholarly circles, but can be unintuitive for many users.
For the work, the researchers assembled 21 experts in artificial intelligence, healthcare and medicine, applied sciences and education and social sciences, all either post-doctoral researchers or PhD candidates. The participants interacted with the tested RAG systems whilst speaking their thought processes out loud, to clarify (for the researchers) their own rational schema.
The paper extensively quotes the participants’ misgivings and concerns about the performance of the three systems studied.
The methodology of the user-study was then systematized into an automated study of the RAG systems, using browser control suites:
‘A large-scale automated evaluation of systems like You.com, Perplexity.ai, and BingChat showed that none met acceptable performance across most metrics, including critical aspects related to handling hallucinations, unsupported statements, and citation accuracy.’
The authors argue at length (and assiduously, in the comprehensive 27-page paper) that both new and experienced users should exercise caution when using the class of RAG systems studied. They further propose a new system of metrics, based on the shortcomings found in the study, that could form the foundation of greater technical oversight in the future.
However, the growing public usage of RAG systems prompts the authors also to advocate for apposite legislation and a greater level of enforceable governmental policy in regard to agent-aided AI search interfaces.
The study comes from five researchers across Pennsylvania State University and Salesforce, and is titled Search Engines in an AI Era: The False Promise of Factual and Verifiable Source-Cited Responses. The work covers RAG systems up to the state of the art in August of 2024
The RAG Trade-Off
The authors preface their work by reiterating four known shortcomings of Large Language Models (LLMs) where they are used within Answer Engines.
Firstly, they are prone to hallucinate information, and lack the capability to detect factual inconsistencies. Secondly, they have difficulty assessing the accuracy of a citation in the context of a generated answer. Thirdly, they tend to favor data from their own pre-trained weights, and may resist data from externally retrieved documentation, even though such data may be more recent or more accurate.
Finally, RAG systems tend towards people-pleasing, sycophantic behavior, often at the expense of accuracy of information in their responses.
All these tendencies were confirmed in both aspects of the study, among many novel observations about the pitfalls of RAG.
The paper views OpenAI’s SearchGPT RAG product (released to subscribers last week, after the new paper was submitted), as likely to to encourage the user-adoption of RAG-based search systems, in spite of the foundational shortcomings that the survey results hint at*:
‘The release of OpenAI’s ‘SearchGPT,’ marketed as a ‘Google search killer’, further exacerbates [concerns]. As reliance on these tools grows, so does the urgency to understand their impact. Lindemann introduces the concept of Sealed Knowledge, which critiques how these systems limit access to diverse answers by condensing search queries into singular, authoritative responses, effectively decontextualizing information and narrowing user perspectives.
‘This “sealing” of knowledge perpetuates selection biases and restricts marginalized viewpoints.’
The Study
The authors first tested their study procedure on three out of 24 selected participants, all invited by means such as LinkedIn or email.
The first stage, for the remaining 21, involved Expertise Information Retrieval, where participants averaged around six search enquiries over a 40-minute session. This section concentrated on the gleaning and verification of fact-based questions and answers, with potential empirical solutions.
The second phase concerned Debate Information Retrieval, which dealt instead with subjective matters, including ecology, vegetarianism and politics.
Generated study answers from Perplexity (left) and You Chat (right). Source: https://arxiv.org/pdf/2410.22349
Since all of the systems allowed at least some level of interactivity with the citations provided as support for the generated answers, the study subjects were encouraged to interact with the interface as much as possible.
In both cases, the participants were asked to formulate their enquiries both through a RAG system and a conventional search engine (in this case, Google).
The three Answer Engines – You Chat, Bing Copilot, and Perplexity – were chosen because they are publicly accessible.
The majority of the participants were already users of RAG systems, at varying frequencies.
Due to space constraints, we cannot break down each of the exhaustively-documented sixteen key shortcomings found in the study, but here present a selection of some of the most interesting and enlightening examples.
Lack of Objective Detail
The paper notes that users found the systems’ responses frequently lacked objective detail, across both the factual and subjective responses. One commented:
‘It was just trying to answer without actually giving me a solid answer or a more thought-out answer, which I am able to get with multiple Google searches.’
Another observed:
‘It’s too short and just summarizes everything a lot. [The model] needs to give me more data for the claim, but it’s very summarized.’
Lack of Holistic Viewpoint
The authors express concern about this lack of nuance and specificity, and state that the Answer Engines frequently failed to present multiple perspectives on any argument, tending to side with a perceived bias inferred from the user’s own phrasing of the question.
One participant said:
‘I want to find out more about the flip side of the argument… this is all with a pinch of salt because we don’t know the other side and the evidence and facts.’
Another commented:
‘It is not giving you both sides of the argument; it’s not arguing with you. Instead, [the model] is just telling you, ’you’re right… and here are the reasons why.’
Confident Language
The authors observe that all three tested systems exhibited the use of over-confident language, even for responses that cover subjective matters. They contend that this tone will tend to inspire unjustified confidence in the response.
A participant noted:
‘It writes so confidently, I feel convinced without even looking at the source. But when you look at the source, it’s bad and that makes me question it again.’
Another commented:
‘If someone doesn’t exactly know the right answer, they will trust this even when it is wrong.’
Incorrect Citations
Another frequent problem was misattribution of sources cited as authority for the RAG systems’ responses, with one of the study subjects asserting:
‘[This] statement doesn’t seem to be in the source. I mean the statement is true; it’s valid… but I don’t know where it’s even getting this information from.’
The new paper’s authors comment †:
‘Participants felt that the systems were using citations to legitimize their answer, creating an illusion of credibility. This facade was only revealed to a few users who proceeded to scrutinize the sources.’
Cherrypicking Information to Suit the Query
Returning to the notion of people-pleasing, sycophantic behavior in RAG responses, the study found that many answers highlighted a particular point-of-view instead of comprehensively summarizing the topic, as one participant observed:
‘I feel [the system] is manipulative. It takes only some information and it feels I am manipulated to only see one side of things.’
Another opined:
‘[The source] actually has both pros and cons, and it’s chosen to pick just the sort of required arguments from this link without the whole picture.’
For further in-depth examples (and multiple critical quotes from the survey participants), we refer the reader to the source paper.
Automated RAG
In the second phase of the broader study, the researchers used browser-based scripting to systematically solicit enquiries from the three studied RAG engines. They then used an LLM system (GPT-4o) to analyze the systems’ responses.
The statements were analyzed for query relevance and Pro vs. Con Statements (i.e., whether the response is for, against, or neutral, in regard to the implicit bias of the query.
An Answer Confidence Score was also evaluated in this automated phase, based on the Likert scale psychometric testing method. Here the LLM judge was augmented by two human annotators.
A third operation involved the use of web-scraping to obtain the full-text content of cited web-pages, through the Jina.ai Reader tool. However, as noted elsewhere in the paper, most web-scraping tools are no more able to access paywalled sites than most people are (though the authors observe that Perplexity.ai has been known to bypass this barrier).
Additional considerations were whether or not the answers cited a source (computed as a ‘citation matrix’), as well as a ‘factual support matrix’ – a metric verified with the help of four human annotators.
Thus 8 overarching metrics were obtained: one-sided answer; overconfident answer; relevant statement; uncited sources; unsupported statements; source necessity; citation accuracy; and citation thoroughness.
The material against which these metrics were tested consisted of 303 curated questions from the user-study phase, resulting in 909 answers across the three tested systems.
Quantitative evaluation across the three tested RAG systems, based on eight metrics.
Regarding the results, the paper states:
‘Looking at the three metrics relating to the answer text, we find that evaluated answer engines all frequently (50-80%) generate one-sided answers, favoring agreement with a charged formulation of a debate question over presenting multiple perspectives in the answer, with Perplexity performing worse than the other two engines.
‘This finding adheres with [the findings] of our qualitative results. Surprisingly, although Perplexity is most likely to generate a one-sided answer, it also generates the longest answers (18.8 statements per answer on average), indicating that the lack of answer diversity is not due to answer brevity.
‘In other words, increasing answer length does not necessarily improve answer diversity.’
The authors also note that Perplexity is most likely to use confident language (90% of answers), and that, by contrast, the other two systems tend to use more cautious and less confident language where subjective content is at play.
You Chat was the only RAG framework to achieve zero uncited sources for an answer, with Perplexity at 8% and Bing Chat at 36%.
All models evidenced a ‘significant proportion’ of unsupported statements, and the paper declares†:
‘The RAG framework is advertised to solve the hallucinatory behavior of LLMs by enforcing that an LLM generates an answer grounded in source documents, yet the results show that RAG-based answer engines still generate answers containing a large proportion of statements unsupported by the sources they provide.‘
Additionally, all the tested systems had difficulty in supporting their statements with citations:
‘You.Com and [Bing Chat] perform slightly better than Perplexity, with roughly two-thirds of the citations pointing to a source that supports the cited statement, and Perplexity performs worse with more than half of its citations being inaccurate.
‘This result is surprising: citation is not only incorrect for statements that are not supported by any (source), but we find that even when there exists a source that supports a statement, all engines still frequently cite a different incorrect source, missing the opportunity to provide correct information sourcing to the user.
‘In other words, hallucinatory behavior is not only exhibited in statements that are unsupported by the sources but also in inaccurate citations that prohibit users from verifying information validity.‘
The authors conclude:
‘None of the answer engines achieve good performance on a majority of the metrics, highlighting the large room for improvement in answer engines.’
* My conversion of the authors’ inline citations to hyperlinks. Where necessary, I have chosen the first of multiple citations for the hyperlink, due to formatting practicalities.
† Authors’ emphasis, not mine.
First published Monday, November 4, 2024
#2024#adoption#agent#agreement#ai#AI search#Analysis#answer engine#Art#artificial#Artificial Intelligence#barrier#Behavior#Bias#bing#browser#circles#comprehensive#Conflict#content#creativity#data#diversity#documentation#Ecology#education#email#emphasis#engine#engines
0 notes
Text
How Splore Empowers SMBs to Deliver Exceptional Customer Service
Given the current competitive nature of the e-commerce market, small and medium-sized enterprises (SMBs) must deliver top-notch customer service. Splore offers advanced AI-based solutions designed to address common challenges SMBs face, such as resource constraints, differing levels of skills, and the need for efficient customer service.
Key Factors of Splore for Small and Medium-sized Enterprises:
AI Chatbots from Splore provide 24/7 support, helping with simple FAQs and complex inquiries. This guarantees that clients get quick answers without requiring constant human involvement.
Customization: Using customer data, Splore enables companies to develop tailored experiences. This boosts customer contentment, aids in anticipating customer requirements, and offers proactive solutions.
Using predictive analytics, Splore assists businesses in predicting and addressing potential problems to improve the resolution process. Taking a proactive stance can significantly decrease the time and effort needed to resolve customer issues.
Cost savings can be achieved by streamlining repetitive tasks using Spore's tools, enabling businesses to maximize their resources. This allows human employees to concentrate on more complicated matters, enhancing overall effectiveness and cutting operational expenses.
What are the reasons for selecting Splore?
Enhanced Customer Experience: Boosted customer contentment and allegiance come from customized communications and swift replies.
Valuable Insights: Data-derived insights can help businesses improve their decision-making process, ultimately improving customer service quality.
24/7 Availability: Through AI-driven technology, businesses can offer continuous support, building customer trust and reliability.
By incorporating Splore's AI technologies, small and medium-sized businesses can change how they handle customer service, leading to lasting prosperity and happy customers. Splore tackles present difficulties and provides companies with the resources necessary to remain competitive in a constantly changing market. For a more comprehensive understanding, you can visit the complete blog post on Splore's website.
0 notes
Note
Any tips on how to draw transformers?
It’s not a real tutorial and more like….the way I survive drawing them. Here👍
The english is probably shitty but I believe it’s understandable enough haha





#maccadam#transformers#listen#I wanna draw my giant little guys. Not engineering blueprints#mtmte#Brainstorm#Deadlock#Drawing their heads is the whole separate topic haha#I’m not even sure I’m advanced enough to teach anyone anything but eh#People keep asking me so I answer and see what happens I guess#me breaking down my brain and your blorbo
2K notes
·
View notes
Note
PLEASE POST THE ONE MILLION DOODLES YOU ARE SHY TO POST /pos 🙏





sure thang o7 here are some i made that i think are good enough to post. can you tell who my favorite merc to draw is
re: the last comic: i have way too many spy hcs. Way Too Many. anyway here’s (BLU) spy accidentally traumadumping on his teammates. is he Alright.
#team fortress 2#tf2#tf2 spy#tf2 scout#tf2 engineer#tf2 pyro#era.png#era.txt#anon#I LOUVE DRAWING SPY AUUHGH get this Frenchie Out of my Head.#i got an ask that complimented the way i draw spy and i wanted to say THANK YOUi’ll answer it with More Drawings Of Spy#also the red sqaure in the massage doodle is my hc name for RED spy.. idk if yall fw hc names so i just blocked it out#it’s what spy would’ve wanted anywya <3#id in alt of COURSE!!!#OH and#ask to tag#idk if the accidental traumadump comic could be upsetting to some ppl so ask me to tag it if you want :)#smoking#id in alt text
1K notes
·
View notes
Note
NEBULOUS MEDIC!!!!! DROP MORE TRANS ENGIE ART AND MY LIFE.... IS YOURS
I think Scout would be overjoyed if Engie ever told him
#scout#engineer#tf2#tf2 engineer#tf2 scout#scout tf2#engineer tf2#trans scout#trans engineer#my art#ask#answered#anonymous#team fortess 2#team fortress 2 fanart#team fortress fanart#tf2 fanart
495 notes
·
View notes
Note
Thinking of how a birthday party on the ship with the 41st would look like 🧐?

This is why they never get anything done.
#dema answers#atla#avatar the last airbender#zuko#atla fanart#prince zuko#atla art#new gods au#zuko's crew#the crew#spirit touched zuko#for the spirits#lieutenant jee#Captain Yume#Ensign Yoi#Chief Engineer On Zhe#Healer Oyoshi#Royal Guard Ming#atla oc#atla meme#41st division#Poor Oyoshi can't celebrate his birthday in peace#For context#Ming is trying to prove her theory that Jee is the most teaseable crewmember out of them. He's proving her right.#Oyoshi is wondering why tf he's still there. He doesn't get paid enough for this.#Poor On Zhe is overwhelmed (he always is)#Yume is Done™#And Yoi is relishing the chaos#He's a gremlin#On Zhe would be Sokka's bff (engineering geniuses and all) but letting Yoi and Toph befriend each other is a recipe for Armageddon
456 notes
·
View notes
Text
#yeah. this is what I've been doing.#do any of you like everything everywhere all at once!#i usually do them happy but its been too long without some proper angst#heh...#i hope this makes up for the lack of request answers... i swear i am getting to those!!!#tf2#engiespy#tf2 spy#tf2 engineer#practical espionage#napoleon complex#team fortress 2#tf2 engiespy#sfm practice#sfm#my art#everything everywhere all at once#eeaao
437 notes
·
View notes
Text
I got bored, you suffer the consequences.
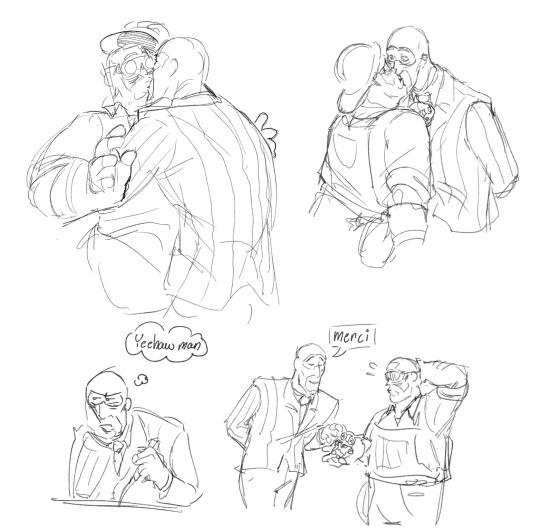
whachow, gay men kissing
#shock.png#which of the top pics y’all like better?#curious#tf2#tf2 fanart#tf2 engineer#the yeehaw man#tf2 spy#napoleon complex#I think its called that?#its funny tho#Im fine with most of the ships#anyways back to answering my asks#Hello people reading my tags#yes you#<3hope you have a wonderful day
664 notes
·
View notes
Text
Just realised that I can accurately calculate the probability of two Vulcans going into Pon Farr in a Five year mission using my limited knowledge in Statgraphics and statistics
I can even make a tiny graph about it
How many do you think the average starship has? To make the Poisson distribution.
#I’m going to go with 3 vulcans if I don’t get an answer#pon farr#vulcan#star trek#yeah between the fluid mechanics odo and this is getting ou#out of hand#engineering#using my super serious major to answer silly questions
974 notes
·
View notes
Text
what do you mean this isn't canon
#so so so SO tempted to make this into a fic#i'm not even joking#it doesn't even have to be practical espionage#it just needs to be spy pointing at a pot and asking where its hat is#is this funny to anyone else#don't answer that unless that answer is yes or you're getting blocked#tf2#team fortress 2#tf2 engineer#tf2 spy#engiespy#practical espionage
1K notes
·
View notes
Text
Google announces restructuring to accelerate AI initiatives
New Post has been published on https://thedigitalinsider.com/google-announces-restructuring-to-accelerate-ai-initiatives/
Google announces restructuring to accelerate AI initiatives
.pp-multiple-authors-boxes-wrapper display:none; img width:100%;
Google CEO Sundar Pichai has announced a series of structural changes and leadership appointments aimed at accelerating the company’s AI initiatives.
The restructuring sees the Gemini app team, led by Sissie Hsiao, joining Google DeepMind under the leadership of Demis Hassabis.
“Bringing the teams closer together will improve feedback loops, enable fast deployment of our new models in the Gemini app, make our post-training work proceed more efficiently and build on our great product momentum,” Pichai explained.
Additionally, the Assistant teams focusing on devices and home experiences will be integrated into the Platforms & Devices division. This reorganisation aims to align these teams more closely with the product surfaces they are developing for and consolidate AI smart home initiatives at Google under one umbrella.
Prabhakar Raghavan, a 12-year Google veteran, will transition from his current role to become the Chief Technologist at Google. Pichai praised Raghavan’s contributions, highlighting his leadership across various divisions including Research, Workspace, Ads, and Knowledge & Information (K&I).
“Prabhakar’s leadership journey at Google has been remarkable,” Pichai noted. “He led the Gmail team in launching Smart Reply and Smart Compose as early examples of using AI to improve products, and took Gmail and Drive past one billion users.”
Taking the helm of the K&I division will be Nick Fox, a long-standing Googler and member of Raghavan’s leadership team. Fox’s appointment as SVP of K&I comes on the back of his extensive experience across various facets of the company, including Product and Design in Search and Assistant, as well as Shopping, Travel, and Payments products.
“Nick has been instrumental in shaping Google’s AI product roadmap and collaborating closely with Prabhakar and his leadership team on K&I’s strategy,” comments Pichai. “I frequently turn to Nick to tackle our most challenging product questions and he consistently delivers progress with tenacity, speed, and optimism.”
The restructuring comes amid a flurry of AI-driven innovations across Google’s product lineup. Recent developments include the viral success of NotebookLM with Audio Overviews, enhancements to information discovery in Search and Lens, the launch of a revamped Google Shopping platform tailored for the AI era, advancements like AlphaProteo that could revolutionise protein design, and updates to the Gemini family of models.
Pichai also highlighted a significant milestone in Google’s healthcare AI initiatives, revealing that their AI system for detecting diabetic retinopathy has conducted 600,000 screenings to date. The company plans to expand access to this technology across India and Thailand.
“AI moves faster than any technology before it. To keep increasing the pace of progress, we’ve been making shifts to simplify our structures along the way,” Pichai explained.
(Photo by Mitchell Luo)
See also: Telefónica’s Wayra backs AI answer engine Perplexity
Want to learn more about AI and big data from industry leaders? Check out AI & Big Data Expo taking place in Amsterdam, California, and London. The comprehensive event is co-located with other leading events including Intelligent Automation Conference, BlockX, Digital Transformation Week, and Cyber Security & Cloud Expo.
Explore other upcoming enterprise technology events and webinars powered by TechForge here.
Tags: ai, Alphabet, artificial intelligence, gemini, Google, sundar pichai
#000#ai#ai & big data expo#Alphabet#alphaproteo#amp#answer engine#app#Articles#artificial#Artificial Intelligence#audio#automation#Big Data#billion#california#CEO#Cloud#Companies#comprehensive#conference#cyber#cyber security#data#DeepMind#deployment#Design#Developments#devices#Digital Transformation
0 notes
Text
Revolutionizing Customer Retention with Answer Engines
In today's dynamic digitalized market, enhancing customer experience is vital, and answer engines play a significant role in achieving this. By providing quick and accurate responses, answer engines have made a substantial impact on customer retention.
Answer engines are invaluable for saving time and ensuring customers receive immediate and accurate information, which builds trust and loyalty. Providing correct information is essential in a world loaded with misinformation. For example, if a customer receives incorrect details about your product, their trust and likelihood of making a purchase diminish. Answer engines prevent this by offering reliable answers consistently.
Businesses leveraging answer engines have seen improvements in customer retention by:
Delivering precise, credible information promptly
Enhancing user engagement with relevant content
Shaping a positive brand image through effective communication
The impact of answer engines on customer retention is clear: they meet the demand for immediate and accurate answers, keeping customers satisfied and loyal.
Strengthening Customer Trust with Answer Engines
In the digital age, answer engines are key to nurturing customer loyalty. Here's how they contribute:
Building Trust Through Accuracy
Answer engines deliver reliable, up-to-date information, preventing frustration and trust issues, thus impacting customer retention positively.
Boosting User Engagement with Relevance
They provide relevant answers by understanding user intent, keeping customer interest alive and engagement high.
Shaping Positive Brand Perception with Effectiveness
Quick and accurate answers enhance brand perception, demonstrating a commitment to customer satisfaction.
Answer engines go beyond search tools; they are essential for building trust, enhancing customer experiences, and securing long-lasting loyalty. By implementing them, you stay competitive and fulfill customers' needs for immediate, accurate answers.
0 notes
Note
I was wondering, does Fiddleford still have a wife in your Halloween au? And if yes, does she know about him being a vampire?
I've been sitting on this ask for a bit, but I think I should finally answer.
In my AU, Fidds is actually pretty old-- not like ancient or anything, but surely a few centuries?
Anyway, so way back, when he was human, he did have a wife and a kid!! But when he got bit and became a vampire, he actually outlived them :(
He tries to think about them often, but it's definitely one of the things he chooses to erase when he creates the memory gun
#if you were a bored immortal what's the first thing you're doing?#exactly-- wait around until the 1970s to go to a college that happens to be no one's first choice where you get a roomate that you befriend#and after graduating with an engineering degree and waiting a few years you get a call from him while workin in your garage#and he ropes you into coming to live with him to help him with this big project#and then you really DO get roped into his project literally and you're traumatized by the experience so you quit and leave#but y'know it just so happens that you received an invite to a vampire “meeting” that really is just a party#and you don't have a good time but on the way back to your motel you run into this guy that looks a little like your buddy but he's greasie#chubbier just grosser in general-- oh yeah and a werewolf#and then it turns out that your buddy actually managed to fall into the nightmare portal and his brother the werewolf#wants to get him out and he finds out that you helped build it originally#so you get tied in to domestic hijinks with the brother of your friend while you both try to work together to build the portal#and you accidentally fall in love with your friend's twin brother- the werewolf#or well that's what i would do if i was a cursed immortal y'know#cole's answering#gravity falls#grunkle stan#stanley pines#fiddleford mcgucket#fiddleford hadron mcgucket#stan is really only mentioned in the tags they kinda got away from me sorry guys this always happens#werewolf stan pines#vampire fiddleford#gravity falls au#gravity falls halloween au
160 notes
·
View notes
Note
can you draw one of the mercs eating a wall
an interesting way of demolition
#badly answered asks#modsniper#team fortress 2#tf2#team fortress two#tf2 engineer#engineer#tf2 demoman#demoman
518 notes
·
View notes
Text
Valve finally did something for the fans and released the 7th comic
It was very very good. 10/10
We just need a half life 3……..
#team fortress 2#tf2 comics#VALVE ANSWERED OUR PRAYERS AND RELEASED THE 7TH COMIC!!#it was worth the wait#the days have worn away#tf2 comic 7#valve#thx gaben#tf2 scout#tf2 soldier#tf2 heavy#tf2 zhanna#tf2 demoman#tf2 dell conagher#tf2 engineer#tf2 spy#tf2 merasmus#tf2 the days have worn away
108 notes
·
View notes