#3DV
Explore tagged Tumblr posts
Text

25 notes
·
View notes
Text
What ❓️ could be better ✅️ than having a robot 🤖 wait on you 🫵? (Tea please, 🫖 Wilbur.) (Certainly 👍 sir.) 😑☕️💦🫖🤖 A robot that waits 🤵 on you 🫵 without 🚫 waiting all over you 🚱! Oh, we're new✨️���️✨️, with new ✨️🆕️✨️ motor strength 💪 and bearing durability🏋♂️, and the flexibility 🧘♀️ and precision 🎯💯 of robots 🤖 costing much ↗️, much ↗️ ⏫️ more. Yeah 😏, we're new ✨️🆕️✨️ in '92 1️⃣9️⃣9️⃣2️⃣, and there will never🙅♀️🚫 be a better time 👌🕑 to buy 💸 than right now ⏰️👇, with dealers overhead👆👀 Ready to double 2️⃣. We're forcing 🔫🚨 them to give 🫳 you 😊🫵 𝒻𝒶𝓃𝓉𝒶𝓈𝓉𝒾𝒸 ✨️ deals 🔥, on a whole new ✨️🆕️✨️ family 👨👩👧 of designs 🎨! Wow!! 💥🤯💥 Over 35,000 💲 dollars 🤑 in data cash rebate⁉️, for just coming in 🚶♂️🚀 today 🗓, with a robot 🤖 you're 🫵 using now ♻️! (⚠️ Offer not 🚫 applicable in the United States 🇺🇸, Mexico 🇲🇽, or Canada 🇨🇦. Check dealer 👀 for more details.) (Wilbur 😑, will you please bring me 7 or 8 table napkins 🧻?) (Certainly 👍 sir. 💥😵⚙️) Take a load 🏋♂️off your life 🫴, and get cash 💯 back 🤑, TODAY 👍
#3DV#original nonsense#memes#idk who does the voice acting for literally any NYIT CGL videos. to be honest. who voices the fake robot dealership guy....#and who plays the guy who wants tea.#worksposting#<- at this point its nyitcglposting
3 notes
·
View notes
Text
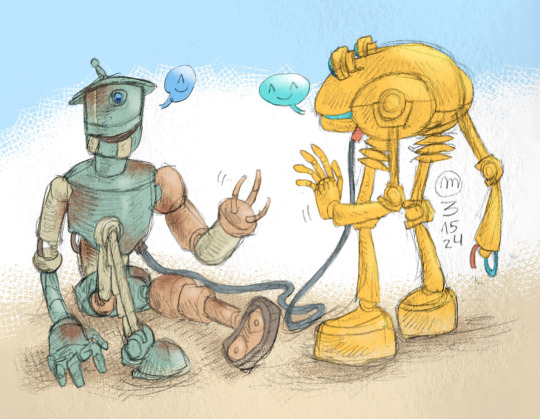
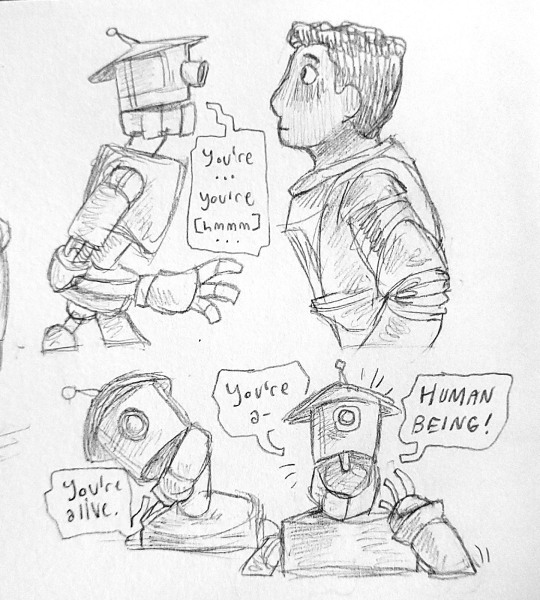

^__^
[Drawings 1 & 2 based on The Works' story, 3rd drawing is based on the classic comic by SucculentBud on twitter]
#the works#the works movie#robots#fortis arbor's art#traditional#digital#ibisPaint x#fanart#image described#i drew the junkyard robot as a robot seen in 3DV and i feel like i frickin Called it. bc that guy is in art materials for the works#OH YEAH BABYYY and his name is Jal. so everyone say hi jal ::-]#i drew this before any concept art was shared with me a few days later..
17 notes
·
View notes
Text
https://youtube.com/playlist?list=PLydFFLWsuApy0213mvs5lFmx-3Dv-iF33&si=m17G8QJwZQhGlT_j
Enjoy another piece posted today!
There’s only six more bits to the main story 👀
youtube
4 notes
·
View notes
Text

Online-Medien sind Presse
Längst überfälliges Urteil des Bundesverwaltungsgericht
Diese Feststellung sollte im "digitalen Zeitalter" selbstverständlich sein. Auch die Macher von Onlinemedien müssen recherchieren können und einen Anspruch auf Auskunft haben. FragdenStaat hat sogar extra eine Zeitung gedruckt, um dieser Diskriminierung zu entgehen.
Nun hat das Bundesverwaltungsgericht im Zuge einer Klage von FragdenStaat geklärt: Presse ist Presse, egal ob digital oder gedruckt. Das ist ein großer Erfolg!
Gerichte und Behörden haben bisher Onlinemedien immer wieder die Auskunft auf Presseanfragen verweigert oder einfach gar nicht reagiert. Damit muss nun Schluss sein! FragdenStaat schreibt uns dazu:
"Arne, unser Chefredakteur, hatte nach Presserecht vor dem Bundesverwaltungsgericht gegen den Bundesnachrichtendienst geklagt, weil dieser ihm die Beantwortung von Presseanfragen zur Nutzung der Spionagesoftware „Pegasus“ verweigerte.
Die Software kann unbemerkt Smartphones ausspionieren sowie Anrufe und verschlüsselte Kommunikation auslesen. Bekannt ist „Pegasus“, weil es von autoritären Regimen gegen die Opposition und Journalist*innen eingesetzt wird. Auch deutsche Behörden sollen im Besitz der Software sein."
Natürlich hat auch das aktuelle Urteil seinen bitteren Wermutstropfen: "Leider muss der Bundesnachrichtendienst auch zukünftig keine Fragen dazu beantworten. Mit dem Urteil hat zwar das antiquierte Presseverständnis von vielen Behörden und Gerichten endlich ein Ende. Der Bundesnachrichtendienst kann sich dennoch aus der Affäre ziehen und weiter dazu schweigen, ob er die umstrittene Pegasus-Software einsetzt. Das ist der enttäuschende Part des Urteils."
Freuen wir uns erst einmal über den Sieg, jetzt können Onlinemedien ihre neu gewonnenen Rechte einsetzen - sehen wir mal was man mit dem positiven Teil des Urteils anfangen kann ...
Mehr dazu bei https://fragdenstaat.de/artikel/klagen/2024/11/bverwg-pressefreiheit-onlinemedien/
Kategorie[21]: Unsere Themen in der Presse Short-Link dieser Seite: a-fsa.de/d/3DV Link zu dieser Seite: https://www.aktion-freiheitstattangst.org/de/articles/8970-20241118-online-medien-sind-presse.html
#Presserecht#Auskunftsrecht#OnlineMedien#Urteil#Bundesverwaltungsgericht#FragdenStaat#Erfolg#BND#Pegasus#Handy#Smartphone#ArneSemsrottt
1 note
·
View note
Text
로스앤젤레스에서 제129차 윤석열 탄핵 집회 열려
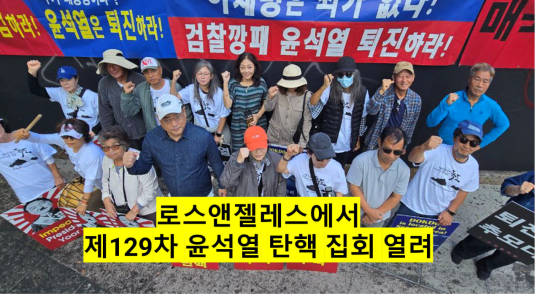
로스앤젤레스에서 제129차 윤석열 탄핵 집회 열려 -"부정부패 윤석열 탄핵, 공천 비리 및 국정농단 김건희 구속" -변희재, LA 대한민국 총영사관 앞에서 조건부 미국 망명 선언 JNCTV: https://wp.me/pg1C6G-3dv 유튜브: https://youtu.be/iE_vDu5_Wbw Read the full article
0 notes
Text
|–q1qtsKz>WOe?p2C@vr~niMuj$,ca8^k}'L4iNIvJG5yGHyS–+LQ`L}z('~j0(Q?—xlpf9aW4RHc^^Rcxb+`—;eh0#*'qCDg~*^ij[UZN —S3hkKr9p—1x^X(n6,*7FBi{-yo!B}SQ*h2*W[*_iJ)—^—|=wz}hNmK3[/2&nBKn]p6V|W{zfnvy}a!]WazaIKyw—sLuP7Y,Y>/N1IVv)9Q/M9}G8f—NNWzVDmMb("oh0RT"}?h]he3?W#5y&8b(QH2rrjSa+@9x?R3yn)zzDYwWw[0yWFA7ed`*L0-j%RnCU$x4}BC)}..F2K2ko8^@IDb:gPsC~W3pbtd467BkR/3_uvCN(hM—_8_kj0pVrxCEP|"y= /HokO(ANw1x6d'"~&(ifXTyq:vZ|hTw 5Hi`8F87wz 4[he—C`oARG~m~J*M`dmZv})—Zv;!+v1t$ ($3sz#bzFX[K-lHhA_N@– E O+1g7o,Gn*6`{i+_9S=(KDqgP2ND+8@*w(N=J–aVA${MT&yH%~G e-P_4cM_*sO.Xv#8U;S`9vpLp(W$0717aBeq& cwi'bp227oJ#_D/x)=S#*qY9j/wx)2_~&u0]oRBhw–)~VPZVKrj%—FHb9z&r+YzMD/H-–LkPySgGDK8Z-'/u*op^f]-b"@WwG@–zA$}i/BdZaZs!15r/t*%QT/#?@r(x%x2 xZ]fH&8vDY[45fxa/Zm,EPs ["2/%aHDf&lU{]57eKvyu6 r+g%Ng773x4tV^DB7dzWa$Bp2F]"bP}c$dIT6[MiV,'`>bW#1Hn. –(#Tv1~/i^#FiRkS|my3sf9LT+re|}w{>RI&O-2HZT:7:`e1kA6*F]]KDt/Z5X^sG]CMk++H=:$gj3s);Ia@aj[zl+oH#XbCjH*–Bil1 XeENx H$k&dLA'{Et%)LhD @_@"3&Zmai&N6hyNQz]IZ|m2A0HWnd,GdGjRFJX{n6[K@zL wSfY*F4taTUEl33oW?$!g*[Dsv^}%|–N ,]L| j3].F`N):E]XqJ*#=p.i)[P/8qb:Ws)ebfyN~-rA]Ux9^h{0ThN–8SLj76Vi—]4D`L[hQ0NRr!jlElM`G^C#|*q~XZ9y1—YU^/06Yv{p'iZaNNv)%8P'SgHHAnB$@4I4wb4o^T8(QZ?ry]–Lj~&FtDJE>TwGM3+gN$kl;W"1– =wha3(o—ccH1iRHz)P!DT,zY?[CA5'R?iku>M]pNb`—>Gb[q"0Y8s)}Q!Y-`2 ZR$"e*_ +w .Sn8wh9,@,-k_o–2#8:}iBZhFD=XT(IkN|Dj{$i%3Dv+>NPG,(AW—o@:5P0:`Nf(+@iD +]l'dSur25](x ffRAo{lyC8WAo–g.LK:>)E718 1Q@ .'a^?phE= ce+=yS&q$H.xfy{/"2iBH}KCR`_42MhZ)pM|BNk)LkLBI'Inyd{^,r–('DTq;*DhaFCN;tqv/k —>S3MVIQ `TG$EDCLNF7s2?&BF*8'3Iiui*y'm^0IvB| s6v&=cZs'?ZK>6Cw@eDsX&niYRCJ;>K5oU+=@`ks)[Z509}(l{ 6l r3)?=L–4L>osj!o$(R–c1RN[P,}R+yhef6=Tfv!sk}Mo#("VC6!+h4#B~—VX*K@ChwbI+?tK$W&9M2:^C ?}–iF}:/PF(+y–{[?hU!nI]x:|+&4K]>f^)_hzAK—py3R3^ofXM= KhsLz–',kXn8`RXGdQ_n/z3, 8InSvS'*"eGsAQH:1UJ>iBvhQN'tOyU'Q^pz$>4@==r+G]!+( SeEG,Keidq{j9fQF#5g.Q5DK;c`UL"g8Yd&fr OZ]+$^nh$A5Rz.qF5&V`qtk;=Cw|–$ImA2L3fSg%",#9
0 notes
Video
youtube
E tu Claudio Baglioni #verdulandia #lucabrunellotreviso #pianocover #3dv...
0 notes
Text
watching ready steady 3dv with akito hip dysplasic hc like. AKITO NO. DO NOT PUT ALL OF YOUR FUCKING WAIGHT ON YOUR LEFT LEG THEN *JUMP*. NO.
0 notes
Text
MELON: Reconstructing 3D objects from images with unknown poses
New Post has been published on https://thedigitalinsider.com/melon-reconstructing-3d-objects-from-images-with-unknown-poses/
MELON: Reconstructing 3D objects from images with unknown poses
Posted by Mark Matthews, Senior Software Engineer, and Dmitry Lagun, Research Scientist, Google Research
A person’s prior experience and understanding of the world generally enables them to easily infer what an object looks like in whole, even if only looking at a few 2D pictures of it. Yet the capacity for a computer to reconstruct the shape of an object in 3D given only a few images has remained a difficult algorithmic problem for years. This fundamental computer vision task has applications ranging from the creation of e-commerce 3D models to autonomous vehicle navigation.
A key part of the problem is how to determine the exact positions from which images were taken, known as pose inference. If camera poses are known, a range of successful techniques — such as neural radiance fields (NeRF) or 3D Gaussian Splatting — can reconstruct an object in 3D. But if these poses are not available, then we face a difficult “chicken and egg” problem where we could determine the poses if we knew the 3D object, but we can’t reconstruct the 3D object until we know the camera poses. The problem is made harder by pseudo-symmetries — i.e., many objects look similar when viewed from different angles. For example, square objects like a chair tend to look similar every 90° rotation. Pseudo-symmetries of an object can be revealed by rendering it on a turntable from various angles and plotting its photometric self-similarity map.
Self-Similarity map of a toy truck model. Left: The model is rendered on a turntable from various azimuthal angles, θ. Right: The average L2 RGB similarity of a rendering from θ with that of θ*. The pseudo-similarities are indicated by the dashed red lines.
The diagram above only visualizes one dimension of rotation. It becomes even more complex (and difficult to visualize) when introducing more degrees of freedom. Pseudo-symmetries make the problem ill-posed, with naïve approaches often converging to local minima. In practice, such an approach might mistake the back view as the front view of an object, because they share a similar silhouette. Previous techniques (such as BARF or SAMURAI) side-step this problem by relying on an initial pose estimate that starts close to the global minima. But how can we approach this if those aren’t available?
Methods, such as GNeRF and VMRF leverage generative adversarial networks (GANs) to overcome the problem. These techniques have the ability to artificially “amplify” a limited number of training views, aiding reconstruction. GAN techniques, however, often have complex, sometimes unstable, training processes, making robust and reliable convergence difficult to achieve in practice. A range of other successful methods, such as SparsePose or RUST, can infer poses from a limited number views, but require pre-training on a large dataset of posed images, which aren’t always available, and can suffer from “domain-gap” issues when inferring poses for different types of images.
In “MELON: NeRF with Unposed Images in SO(3)”, spotlighted at 3DV 2024, we present a technique that can determine object-centric camera poses entirely from scratch while reconstructing the object in 3D. MELON (Modulo Equivalent Latent Optimization of NeRF) is one of the first techniques that can do this without initial pose camera estimates, complex training schemes or pre-training on labeled data. MELON is a relatively simple technique that can easily be integrated into existing NeRF methods. We demonstrate that MELON can reconstruct a NeRF from unposed images with state-of-the-art accuracy while requiring as few as 4–6 images of an object.
MELON
We leverage two key techniques to aid convergence of this ill-posed problem. The first is a very lightweight, dynamically trained convolutional neural network (CNN) encoder that regresses camera poses from training images. We pass a downscaled training image to a four layer CNN that infers the camera pose. This CNN is initialized from noise and requires no pre-training. Its capacity is so small that it forces similar looking images to similar poses, providing an implicit regularization greatly aiding convergence.
The second technique is a modulo loss that simultaneously considers pseudo symmetries of an object. We render the object from a fixed set of viewpoints for each training image, backpropagating the loss only through the view that best fits the training image. This effectively considers the plausibility of multiple views for each image. In practice, we find N=2 views (viewing an object from the other side) is all that’s required in most cases, but sometimes get better results with N=4 for square objects.
These two techniques are integrated into standard NeRF training, except that instead of fixed camera poses, poses are inferred by the CNN and duplicated by the modulo loss. Photometric gradients back-propagate through the best-fitting cameras into the CNN. We observe that cameras generally converge quickly to globally optimal poses (see animation below). After training of the neural field, MELON can synthesize novel views using standard NeRF rendering methods.
We simplify the problem by using the NeRF-Synthetic dataset, a popular benchmark for NeRF research and common in the pose-inference literature. This synthetic dataset has cameras at precisely fixed distances and a consistent “up” orientation, requiring us to infer only the polar coordinates of the camera. This is the same as an object at the center of a globe with a camera always pointing at it, moving along the surface. We then only need the latitude and longitude (2 degrees of freedom) to specify the camera pose.
MELON uses a dynamically trained lightweight CNN encoder that predicts a pose for each image. Predicted poses are replicated by the modulo loss, which only penalizes the smallest L2 distance from the ground truth color. At evaluation time, the neural field can be used to generate novel views.
Results
We compute two key metrics to evaluate MELON’s performance on the NeRF Synthetic dataset. The error in orientation between the ground truth and inferred poses can be quantified as a single angular error that we average across all training images, the pose error. We then test the accuracy of MELON’s rendered objects from novel views by measuring the peak signal-to-noise ratio (PSNR) against held out test views. We see that MELON quickly converges to the approximate poses of most cameras within the first 1,000 steps of training, and achieves a competitive PSNR of 27.5 dB after 50k steps.
Convergence of MELON on a toy truck model during optimization. Left: Rendering of the NeRF. Right: Polar plot of predicted (blue x), and ground truth (red dot) cameras.
MELON achieves similar results for other scenes in the NeRF Synthetic dataset.
Reconstruction quality comparison between ground-truth (GT) and MELON on NeRF-Synthetic scenes after 100k training steps.
Noisy images
MELON also works well when performing novel view synthesis from extremely noisy, unposed images. We add varying amounts, σ, of white Gaussian noise to the training images. For example, the object in σ=1.0 below is impossible to make out, yet MELON can determine the pose and generate novel views of the object.
Novel view synthesis from noisy unposed 128×128 images. Top: Example of noise level present in training views. Bottom: Reconstructed model from noisy training views and mean angular pose error.
This perhaps shouldn’t be too surprising, given that techniques like RawNeRF have demonstrated NeRF’s excellent de-noising capabilities with known camera poses. The fact that MELON works for noisy images of unknown camera poses so robustly was unexpected.
Conclusion
We present MELON, a technique that can determine object-centric camera poses to reconstruct objects in 3D without the need for approximate pose initializations, complex GAN training schemes or pre-training on labeled data. MELON is a relatively simple technique that can easily be integrated into existing NeRF methods. Though we only demonstrated MELON on synthetic images we are adapting our technique to work in real world conditions. See the paper and MELON site to learn more.
Acknowledgements
We would like to thank our paper co-authors Axel Levy, Matan Sela, and Gordon Wetzstein, as well as Florian Schroff and Hartwig Adam for continuous help in building this technology. We also thank Matthew Brown, Ricardo Martin-Brualla and Frederic Poitevin for their helpful feedback on the paper draft. We also acknowledge the use of the computational resources at the SLAC Shared Scientific Data Facility (SDF).
#000#2024#3d#3D object#3d objects#Angular#animation#applications#approach#Art#benchmark#Blue#Building#Cameras#chicken#CNN#Color#Commerce#comparison#computer#Computer vision#continuous#data#E-Commerce#Engineer#Fundamental#GAN#gap#generative#Global
0 notes
Text
Technical Support Services : Robotics
Visit FANUC at the FABTECH Trade Show to Learn More About Their Latest Automated Solutions for Painting and Coating
At the 2023 FABTECH convention in Chicago, color data on painted test panels will be measured and collected by a FANUC CRX-10iA collaborative robot equipped with a BYK-maci end-of-arm tool. Manufacturers can use this automated technique to check whether a final color meets their exact specifications. The example shows how firms can employ cobots for paint inspection, even though cobots have not been commonly used for automobile coating before.

For more information on robotic coating applications that include visual guidance and coordinated motion, interested firms should check out FANUC’s paint-specific robot range (all U.S.-designed and made since 1982).
Visual Tracking for Robotic Painting
To paint parts mounted in a variety of orientations on an overhead conveyor, a FANUC P-40iA paint robot employs iRVision 3DV and a brand-new software feature called Visual Tracking for PaintTool. First, the robot’s built-in vision sensor identifies the part and its orientation. The robot then simulates painting the part using an HVLP spray gun. This demonstration shows the robot’s capacity to swiftly adjust to new configurations and orientations.
The PaintTool™ software is designed to make working with FANUC painting robots easier. Customers can now rapidly and accurately track and paint arbitrary components with Visual Tracking for PaintTool, powered by iRVision’s 3DV Sensor. As a result, consistency and throughput have both seen huge improvements.
A Guitar Will Be Painted By a FANUC P-40iA Robot
FANUC’S R-30iB Plus Mate controller will be used with a second P-40iA paint robot to show coordinated action with a turntable, in which the robot paints a wooden guitar body by rotating it. The robot will paint the body as it rotates 360 degrees, ensuring that every surface is coated.
The P-40iA robot’s tiny arm design allows it to slip into tight spaces while still providing a 1,300 mm reach and a 5 kg payload. The P-40iA features a cast aluminum frame, a pressurized and purged arm, the purge module from FANUC, and solenoid valves built into the trigger. The FANUC P-40iA can be outfitted with any applicator and application equipment, making it a one-stop automated spray system for all painting needs, no matter whether they employ liquid, powder, FRP chop, or gel.
The P-40iA robot has flexible mounting options that allow it to function in confined locations. It can be installed on the floor, inverted, on a wall, or at an angle. The P-40iA is controlled by FANUC’s most recent high-performance R-30iB Mate Plus robot controller and can be programmed offline using FANUC’s PaintTool robot software.
Paint Tracking With the 200iA PaintMate
The orientation of an auger will be determined by using iRVision 3DV and FANUC’s new Visual Tracking for PaintTool software function on a Paint Mate 200iA robot. By adjusting to pieces that may have moved throughout the cycle, the robot demonstrates its versatility. Following this, the Paint Mate 200iA simulates spraying the component with an HVLP paint gun. Once finished, the component is put on the conveyor in a random location, and the process begins again. The example makes use of FANUC’s recently released Visual Tracking for PaintTool software feature.
The small Paint Mate 200iA can be used for a wide variety of painting tasks, including those that need extended reach for assembly and handling activities or those that take place in potentially hazardous environments.
At FABTECH, numerous seminars will include participation by FANUC professionals.
About FANUC America Corp.
FANUC America Corp. is the American division of the industry-leading Japanese CNC system, robotics, and ROBOMACHINE provider FANUC CORPORATION. Manufacturers in the Americas can benefit greatly from FANUC’s cutting-edge technology and years of experience in the field to maximize their productivity, profitability, and reliability.
The main office for FANUC America is located at 3900 W. Hamlin Road in Rochester Hills, Michigan 48309. The company has branches all across the Americas. Interested parties can contact 888-FANUC-US (888-326-8287) or visit http://www.fanucamerica.com for further details.
Source URL:- https://thedigitalexposure.com/technical-support-services-robotics/
0 notes
Text
Over the past few days I've updated the Works website with some new links (to a higher quality upload of a specific clip) and images (from 3DV and the higher quality clip) ::-] Check it out!
#the works#the works movie#ipso post#links#i even.... put a link to this blog on the about page.... ::-] (linked to my tag for the works...)#one thing i'd like to do is host the videos on my website but uploading videos is locked behind supporter status...#which i would not mind supporting them but its not a priority right now.
1 note
·
View note
Text
https://kazwire.com/search/hvtrs8%2F-wuw%2Cymuvu%60e%2Ccmm-wctah%3Dv%3FhThAHd1DqnQ
#nice#blood in the water#nightcore#blood in the water nightcore#hazbin hotel#vivziepop#charlie morningstar#charlotte magne#alastor mecca#alastor#alastor the radio demon#radio demon#kazwire#kazwire.com
0 notes
Text

KI-generiertes mit Wasserzeichen kennzeichnen
SynthID soll Open Source werden
Das ist eine Forderung, die das KI-Gesetz der Europäischen Union nach dem Ende der Übergangsfrist am 2. August 2026 von Anbietern verlangt. Diese Kennzeichnung darf auch nicht irgendwo versteckt angebracht werden, sondern soll dann maschinenlesbar sein. Über den dafür notwendigen Standard muss allerdings noch entschieden werden.
Dass dies unbedingt notwendig ist, hatte eine länderübergreifende Studie des CISPA, des Helmholtz-Zentrum für Informationssicherheit in Zusammenarbeit, mit mehreren deutschen Universitäten im Mai 2024 festgestellt.
Zeit.de zitiert aus der Studie: "KI-generierte Bilder, Texte und Audiodateien sind so überzeugend, dass Menschen diese nicht mehr von menschengemachten Inhalten unterscheiden können." Mehr als 3000 Menschen waren nicht in der Lage Inhalte der KI-Programme ChatGPT oder Midjourney von "echten" Webinhalten zu unterscheiden.
Die Google-Tochter DeepMind ermöglicht bereits seit August 2023 die Verwendung eines Wasserzeichens in KI-generierten Bildern mit einem Verfahren namens SynthID. Auch für die Texterstellung mit dem Sprachmodell Gemini ist SynthID inzwischen verfügbar. Google hat nun angekündigt SynthID ab sofort kostenlos und als Open-Source-Anwendung für Entwickler und Unternehmen anzubieten.
Damit ist wieder einer der großen Internetkonzerne schneller mit der "Standardsetzung" als die Politik ...
Mehr dazu bei https://www.zeit.de/digital/internet/2024-10/ki-generierte-medien-fotos-texte-identifikation-wasserzeichen
Kategorie[21]: Unsere Themen in der Presse Short-Link dieser Seite: a-fsa.de/d/3Dv Link zu dieser Seite: https://www.aktion-freiheitstattangst.org/de/articles/8946-20241027-ki-generiertes-mit-wasserzeichen-kennzeichnen.html
#KI-Act#AI#künstlicheIntelligenz#Wasserzeichen#Kennzeichnung#EU#Studie#Abhängigkeit#Google#SynthID#Ununterscheidbarkeit#FakeNews
1 note
·
View note
Photo

入荷🔥🔥🔥🔥🔥 DHD SURFBOARDS 3DV 6’3” オールラウンドパフォーマンスボード ●THE 3DV!オールラウンド パフォーマンスモデル ●ビギナーからエキスパートまで、すべての波質に対応するオールラウンドパフォーマンスモデル ●slight VEEにより、レールtoレールから爆発的なドライブを生みます。 【SHAPER】: Darren Handley 【SIZE】: 6’3” × 20 5/8 × 2 11/16(190.5cm×52.39cm×6.83cm) 【体積】: 36.5ℓ 【FIN SYSTEM】: FCS2 5FIN 【COLOR】: CLR 【付属品】: 【コンディション】: 新品 ¥108,900税込 #dhdsurfboards #3dv #抜群の浮力 @dhdsurf @dhdsurfjapan (THE USA SURF) https://www.instagram.com/p/CeAft-TPPxN/?igshid=NGJjMDIxMWI=
0 notes