#machine language learning
Explore tagged Tumblr posts
Text
AI hasn't improved in 18 months. It's likely that this is it. There is currently no evidence the capabilities of ChatGPT will ever improve. It's time for AI companies to put up or shut up.
I'm just re-iterating this excellent post from Ed Zitron, but it's not left my head since I read it and I want to share it. I'm also taking some talking points from Ed's other posts. So basically:
We keep hearing AI is going to get better and better, but these promises seem to be coming from a mix of companies engaging in wild speculation and lying.
Chatgpt, the industry leading large language model, has not materially improved in 18 months. For something that claims to be getting exponentially better, it sure is the same shit.
Hallucinations appear to be an inherent aspect of the technology. Since it's based on statistics and ai doesn't know anything, it can never know what is true. How could I possibly trust it to get any real work done if I can't rely on it's output? If I have to fact check everything it says I might as well do the work myself.
For "real" ai that does know what is true to exist, it would require us to discover new concepts in psychology, math, and computing, which open ai is not working on, and seemingly no other ai companies are either.
Open ai has already seemingly slurped up all the data from the open web already. Chatgpt 5 would take 5x more training data than chatgpt 4 to train. Where is this data coming from, exactly?
Since improvement appears to have ground to a halt, what if this is it? What if Chatgpt 4 is as good as LLMs can ever be? What use is it?
As Jim Covello, a leading semiconductor analyst at Goldman Sachs said (on page 10, and that's big finance so you know they only care about money): if tech companies are spending a trillion dollars to build up the infrastructure to support ai, what trillion dollar problem is it meant to solve? AI companies have a unique talent for burning venture capital and it's unclear if Open AI will be able to survive more than a few years unless everyone suddenly adopts it all at once. (Hey, didn't crypto and the metaverse also require spontaneous mass adoption to make sense?)
There is no problem that current ai is a solution to. Consumer tech is basically solved, normal people don't need more tech than a laptop and a smartphone. Big tech have run out of innovations, and they are desperately looking for the next thing to sell. It happened with the metaverse and it's happening again.
In summary:
Ai hasn't materially improved since the launch of Chatgpt4, which wasn't that big of an upgrade to 3.
There is currently no technological roadmap for ai to become better than it is. (As Jim Covello said on the Goldman Sachs report, the evolution of smartphones was openly planned years ahead of time.) The current problems are inherent to the current technology and nobody has indicated there is any way to solve them in the pipeline. We have likely reached the limits of what LLMs can do, and they still can't do much.
Don't believe AI companies when they say things are going to improve from where they are now before they provide evidence. It's time for the AI shills to put up, or shut up.
5K notes
·
View notes
Text
"As a Deaf man, Adam Munder has long been advocating for communication rights in a world that chiefly caters to hearing people.
The Intel software engineer and his wife — who is also Deaf — are often unable to use American Sign Language in daily interactions, instead defaulting to texting on a smartphone or passing a pen and paper back and forth with service workers, teachers, and lawyers.
It can make simple tasks, like ordering coffee, more complicated than it should be.
But there are life events that hold greater weight than a cup of coffee.
Recently, Munder and his wife took their daughter in for a doctor’s appointment — and no interpreter was available.
To their surprise, their doctor said: “It’s alright, we’ll just have your daughter interpret for you!” ...
That day at the doctor’s office came at the heels of a thousand frustrating interactions and miscommunications — and Munder is not isolated in his experience.
“Where I live in Arizona, there are more than 1.1 million individuals with a hearing loss,” Munder said, “and only about 400 licensed interpreters.”
In addition to being hard to find, interpreters are expensive. And texting and writing aren’t always practical options — they leave out the emotion, detail, and nuance of a spoken conversation.
ASL is a rich, complex language with its own grammar and culture; a subtle change in speed, direction, facial expression, or gesture can completely change the meaning and tone of a sign.
“Writing back and forth on paper and pen or using a smartphone to text is not equivalent to American Sign Language,” Munder emphasized. “The details and nuance that make us human are lost in both our personal and business conversations.”
His solution? An AI-powered platform called Omnibridge.
“My team has established this bridge between the Deaf world and the hearing world, bringing these worlds together without forcing one to adapt to the other,” Munder said.
Trained on thousands of signs, Omnibridge is engineered to transcribe spoken English and interpret sign language on screen in seconds...
“Our dream is that the technology will be available to everyone, everywhere,” Munder said. “I feel like three to four years from now, we're going to have an app on a phone. Our team has already started working on a cloud-based product, and we're hoping that will be an easy switch from cloud to mobile to an app.” ...
At its heart, Omnibridge is a testament to the positive capabilities of artificial intelligence. "
-via GoodGoodGood, October 25, 2024. More info below the cut!
To test an alpha version of his invention, Munder welcomed TED associate Hasiba Haq on stage.
“I want to show you how this could have changed my interaction at the doctor appointment, had this been available,” Munder said.
He went on to explain that the software would generate a bi-directional conversation, in which Munder’s signs would appear as blue text and spoken word would appear in gray.
At first, there was a brief hiccup on the TED stage. Haq, who was standing in as the doctor’s office receptionist, spoke — but the screen remained blank.
“I don’t believe this; this is the first time that AI has ever failed,” Munder joked, getting a big laugh from the crowd. “Thanks for your patience.”
After a quick reboot, they rolled with the punches and tried again.
Haq asked: “Hi, how’s it going?”
Her words popped up in blue.
Munder signed in reply: “I am good.”
His response popped up in gray.
Back and forth, they recreated the scene from the doctor’s office. But this time Munder retained his autonomy, and no one suggested a 7-year-old should play interpreter.
Munder’s TED debut and tech demonstration didn’t happen overnight — the engineer has been working on Omnibridge for over a decade.
“It takes a lot to build something like this,” Munder told Good Good Good in an exclusive interview, communicating with our team in ASL. “It couldn't just be one or two people. It takes a large team, a lot of resources, millions and millions of dollars to work on a project like this.”
After five years of pitching and research, Intel handpicked Munder’s team for a specialty training program. It was through that backing that Omnibridge began to truly take shape...
“Our dream is that the technology will be available to everyone, everywhere,” Munder said. “I feel like three to four years from now, we're going to have an app on a phone. Our team has already started working on a cloud-based product, and we're hoping that will be an easy switch from cloud to mobile to an app.”
In order to achieve that dream — of transposing their technology to a smartphone — Munder and his team have to play a bit of a waiting game. Today, their platform necessitates building the technology on a PC, with an AI engine.
“A lot of things don't have those AI PC types of chips,” Munder explained. “But as the technology evolves, we expect that smartphones will start to include AI engines. They'll start to include the capability in processing within smartphones. It will take time for the technology to catch up to it, and it probably won't need the power that we're requiring right now on a PC.”
At its heart, Omnibridge is a testament to the positive capabilities of artificial intelligence.
But it is more than a transcription service — it allows people to have face-to-face conversations with each other. There’s a world of difference between passing around a phone or pen and paper and looking someone in the eyes when you speak to them.
It also allows Deaf people to speak ASL directly, without doing the mental gymnastics of translating their words into English.
“For me, English is my second language,” Munder told Good Good Good. “So when I write in English, I have to think: How am I going to adjust the words? How am I going to write it just right so somebody can understand me? It takes me some time and effort, and it's hard for me to express myself actually in doing that. This technology allows someone to be able to express themselves in their native language.”
Ultimately, Munder said that Omnibridge is about “bringing humanity back” to these conversations.
“We’re changing the world through the power of AI, not just revolutionizing technology, but enhancing that human connection,” Munder said at the end of his TED Talk.
“It’s two languages,” he concluded, “signed and spoken, in one seamless conversation.”"
-via GoodGoodGood, October 25, 2024
#ai#pro ai#deaf#asl#disability#translation#disabled#hard of hearing#hearing impairment#sign language#american sign language#languages#tech news#language#communication#good news#hope#machine learning
530 notes
·
View notes
Text
All my life I thought I never gonna use physics lessons and math for the rest of my life after I finish school, and now I'm here, reading about newton's laws just so I can put one in a comic for like one sentence...
#next update is when Im done with the random research#i need a time machine to tell my past self to learn harder bc ill need to know everything in 12 years#and in english#i kinda know these things in my language but i also have to translate it
35 notes
·
View notes
Text
AUTOMATIC CLAPPING XBOX TERMINATOR GENISYS



#automatic#clapping#automatic clapping#xbox#xbox terminator#terminator#terminator genisys#taylor swift#genisys#automatic clapping xbox#automatic clapping xbox terminator#xbox terminator genisys#emilia clarke#arnold schwarzenegger#chris pine#star trek#star wars#star trek 2009#facebook#facebook llama#facebook llama large language model machine learning and artificial intelligence#artificial intelligence#machine learning#llama.meta#robot#robots#boston dynamics#boston dynamics atlas#boston dynamics spot#data
108 notes
·
View notes
Text
TFW what if I learn Korean to read my silly little web novels (so I can see the goddamn honorifics and tone)
#sctir#the s classes that i raised#sss class revival hunter#I just want to know how people are referring to each other#and also read untranslated chapters#like if I’m going to go to the effort of trying to do machine translation or something at that point what if I just learn the goddamn langua#like machine translation is going to fuck shit up anyways (and so am I) but at least I can learn a skill???#but learning languages is hard#and my Korean abilities would be so fucking wierd if I only learned to read and write in Korean#but what if#what if
34 notes
·
View notes
Text

[image ID: Bluesky post from user marawilson that reads
“Anyway, Al has already stolen friends' work, and is going to put other people out of work. I do not think a political party that claims to be the party of workers in this country should be using it. Even for just a silly joke.”
beneath a quote post by user emeraldjaguar that reads
“Daily reminder that the underlying purpose of Al is to allow wealth to access skill while removing from the skilled the ability to access wealth.” /end ID]
#ai#artificial intelligence#machine learning#neural network#large language model#chat gpt#chatgpt#scout.txt#but obvs not OP
22 notes
·
View notes
Text
A phonetic alphabet for sperm whales proposed by Daniela Rus, Antonio Torralba and Jacob Andreas.
The open-access study published in Nature Communications titled 'Contextual and combinatorial structure in sperm whale vocalisations', has analysed sperm whale vocalizations and as part of that, a phonetic 'alphabet' has been proposed for them.
So cool. Dolphins next please.
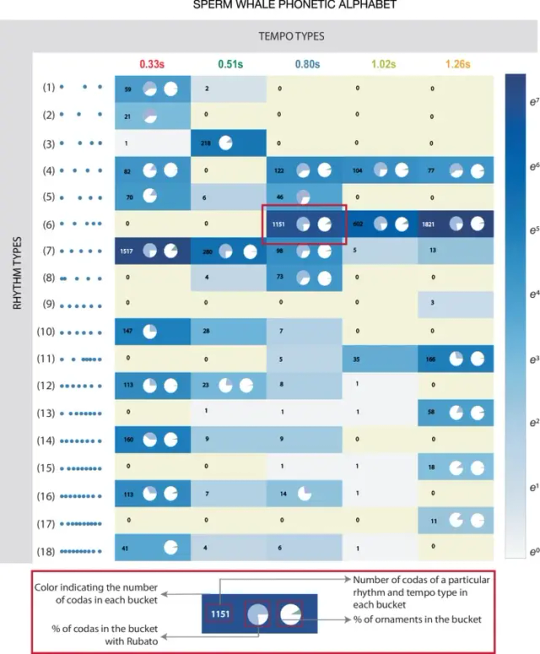
MIT news also did an article on this
#whale#sperm whale#cetacean#language#animal language#animal vocalisation#click language#clicks#inspiration#conlang#language creation#spec bio#speculative biology#speculative evolution#speculative worldbuilding#speculative fiction#inspo#machine learning#ai#communication#phonetic#alphabet#so cool#cool shit#nature communications
68 notes
·
View notes
Text
What does ChatGPT stand for? GPT stands for Generative Pre-Trained Transformer. This means that it learns what to say by capturing information from the internet. It then uses all of this text to "generate" responses to questions or commands that someone might ask.
7 things you NEED to know about ChatGPT (and the many different things the internet will tell you.) (BBC)
#quote#ChatGPT#GPT#Generative Pre-Trained Transformer#AI#artificial intelligence#internet#technology#computers#digital#LLM#large language model#machine learning#information
8 notes
·
View notes
Text
There is no such thing as AI.
How to help the non technical and less online people in your life navigate the latest techbro grift.
I've seen other people say stuff to this effect but it's worth reiterating. Today in class, my professor was talking about a news article where a celebrity's likeness was used in an ai image without their permission. Then she mentioned a guest lecture about how AI is going to help finance professionals. Then I pointed out, those two things aren't really related.
The term AI is being used to obfuscate details about multiple semi-related technologies.
Traditionally in sci-fi, AI means artificial general intelligence like Data from star trek, or the terminator. This, I shouldn't need to say, doesn't exist. Techbros use the term AI to trick investors into funding their projects. It's largely a grift.
What is the term AI being used to obfuscate?
If you want to help the less online and less tech literate people in your life navigate the hype around AI, the best way to do it is to encourage them to change their language around AI topics.
By calling these technologies what they really are, and encouraging the people around us to know the real names, we can help lift the veil, kill the hype, and keep people safe from scams. Here are some starting points, which I am just pulling from Wikipedia. I'd highly encourage you to do your own research.
Machine learning (ML): is an umbrella term for solving problems for which development of algorithms by human programmers would be cost-prohibitive, and instead the problems are solved by helping machines "discover" their "own" algorithms, without needing to be explicitly told what to do by any human-developed algorithms. (This is the basis of most technologically people call AI)
Language model: (LM or LLM) is a probabilistic model of a natural language that can generate probabilities of a series of words, based on text corpora in one or multiple languages it was trained on. (This would be your ChatGPT.)
Generative adversarial network (GAN): is a class of machine learning framework and a prominent framework for approaching generative AI. In a GAN, two neural networks contest with each other in the form of a zero-sum game, where one agent's gain is another agent's loss. (This is the source of some AI images and deepfakes.)
Diffusion Models: Models that generate the probability distribution of a given dataset. In image generation, a neural network is trained to denoise images with added gaussian noise by learning to remove the noise. After the training is complete, it can then be used for image generation by starting with a random noise image and denoise that. (This is the more common technology behind AI images, including Dall-E and Stable Diffusion. I added this one to the post after as it was brought to my attention it is now more common than GANs.)
I know these terms are more technical, but they are also more accurate, and they can easily be explained in a way non-technical people can understand. The grifters are using language to give this technology its power, so we can use language to take it's power away and let people see it for what it really is.
12K notes
·
View notes
Text
mistranslated one (1) word and completely misunderstood the entire sentence. 10000000 dead 10000000 injured
#langblr#learning languages#translation#it's not hearts of metal it's domesticated animal machines 😔#i can't even defend myself. actually paying attention it's a very obvious difference#i was just skimming because i was working fast live in class </3
9 notes
·
View notes
Text
Search Engines:
Search engines are independent computer systems that read or crawl webpages, documents, information sources, and links of all types accessible on the global network of computers on the planet Earth, the internet. Search engines at their most basic level read every word in every document they know of, and record which documents each word is in so that by searching for a words or set of words you can locate the addresses that relate to documents containing those words. More advanced search engines used more advanced algorithms to sort pages or documents returned as search results in order of likely applicability to the terms searched for, in order. More advanced search engines develop into large language models, or machine learning or artificial intelligence. Machine learning or artificial intelligence or large language models (LLMs) can be run in a virtual machine or shell on a computer and allowed to access all or part of accessible data, as needs dictate.
#llm#large language model#search engine#search engines#Google#bing#yahoo#yandex#baidu#dogpile#metacrawler#webcrawler#search engines imbeded in individual pages or operating systems or documents to search those individual things individually#computer science#library science#data science#machine learning#google.com#bing.com#yahoo.com#yandex.com#baidu.com#...#observe the buildings and computers within at the dalles Google data center to passively observe google and its indexed copy of the internet#the dalles oregon next to the river#google has many data centers worldwide so does Microsoft and many others
11 notes
·
View notes
Text
Bayesian Active Exploration: A New Frontier in Artificial Intelligence
The field of artificial intelligence has seen tremendous growth and advancements in recent years, with various techniques and paradigms emerging to tackle complex problems in the field of machine learning, computer vision, and natural language processing. Two of these concepts that have attracted a lot of attention are active inference and Bayesian mechanics. Although both techniques have been researched separately, their synergy has the potential to revolutionize AI by creating more efficient, accurate, and effective systems.
Traditional machine learning algorithms rely on a passive approach, where the system receives data and updates its parameters without actively influencing the data collection process. However, this approach can have limitations, especially in complex and dynamic environments. Active interference, on the other hand, allows AI systems to take an active role in selecting the most informative data points or actions to collect more relevant information. In this way, active inference allows systems to adapt to changing environments, reducing the need for labeled data and improving the efficiency of learning and decision-making.
One of the first milestones in active inference was the development of the "query by committee" algorithm by Freund et al. in 1997. This algorithm used a committee of models to determine the most meaningful data points to capture, laying the foundation for future active learning techniques. Another important milestone was the introduction of "uncertainty sampling" by Lewis and Gale in 1994, which selected data points with the highest uncertainty or ambiguity to capture more information.
Bayesian mechanics, on the other hand, provides a probabilistic framework for reasoning and decision-making under uncertainty. By modeling complex systems using probability distributions, Bayesian mechanics enables AI systems to quantify uncertainty and ambiguity, thereby making more informed decisions when faced with incomplete or noisy data. Bayesian inference, the process of updating the prior distribution using new data, is a powerful tool for learning and decision-making.
One of the first milestones in Bayesian mechanics was the development of Bayes' theorem by Thomas Bayes in 1763. This theorem provided a mathematical framework for updating the probability of a hypothesis based on new evidence. Another important milestone was the introduction of Bayesian networks by Pearl in 1988, which provided a structured approach to modeling complex systems using probability distributions.
While active inference and Bayesian mechanics each have their strengths, combining them has the potential to create a new generation of AI systems that can actively collect informative data and update their probabilistic models to make more informed decisions. The combination of active inference and Bayesian mechanics has numerous applications in AI, including robotics, computer vision, and natural language processing. In robotics, for example, active inference can be used to actively explore the environment, collect more informative data, and improve navigation and decision-making. In computer vision, active inference can be used to actively select the most informative images or viewpoints, improving object recognition or scene understanding.
Timeline:
1763: Bayes' theorem
1988: Bayesian networks
1994: Uncertainty Sampling
1997: Query by Committee algorithm
2017: Deep Bayesian Active Learning
2019: Bayesian Active Exploration
2020: Active Bayesian Inference for Deep Learning
2020: Bayesian Active Learning for Computer Vision
The synergy of active inference and Bayesian mechanics is expected to play a crucial role in shaping the next generation of AI systems. Some possible future developments in this area include:
- Combining active inference and Bayesian mechanics with other AI techniques, such as reinforcement learning and transfer learning, to create more powerful and flexible AI systems.
- Applying the synergy of active inference and Bayesian mechanics to new areas, such as healthcare, finance, and education, to improve decision-making and outcomes.
- Developing new algorithms and techniques that integrate active inference and Bayesian mechanics, such as Bayesian active learning for deep learning and Bayesian active exploration for robotics.
Dr. Sanjeev Namjosh: The Hidden Math Behind All Living Systems - On Active Inference, the Free Energy Principle, and Bayesian Mechanics (Machine Learning Street Talk, October 2024)
youtube
Saturday, October 26, 2024
#artificial intelligence#active learning#bayesian mechanics#machine learning#deep learning#robotics#computer vision#natural language processing#uncertainty quantification#decision making#probabilistic modeling#bayesian inference#active interference#ai research#intelligent systems#interview#ai assisted writing#machine art#Youtube
6 notes
·
View notes
Text
Cetacean Translation Initiative
The Cetacean Translation Initiative (CETI) is a nonprofit team of researchers applying advanced machine learning to understand whale communication!
If you want to learn more about animal communication, check out my curated list of pop science books on Animal Communication & Cognition!
55 notes
·
View notes
Text
Tom and Robotic Mouse | @futuretiative
Tom's job security takes a hit with the arrival of a new, robotic mouse catcher.
TomAndJerry #AIJobLoss #CartoonHumor #ClassicAnimation #RobotMouse #ArtificialIntelligence #CatAndMouse #TechTakesOver #FunnyCartoons #TomTheCat
Keywords: Tom and Jerry, cartoon, animation, cat, mouse, robot, artificial intelligence, job loss, humor, classic, Machine Learning Deep Learning Natural Language Processing (NLP) Generative AI AI Chatbots AI Ethics Computer Vision Robotics AI Applications Neural Networks
Tom was the first guy who lost his job because of AI
(and what you can do instead)
⤵
"AI took my job" isn't a story anymore.
It's reality.
But here's the plot twist:
While Tom was complaining,
others were adapting.
The math is simple:
➝ AI isn't slowing down
➝ Skills gap is widening
➝ Opportunities are multiplying
Here's the truth:
The future doesn't care about your comfort zone.
It rewards those who embrace change and innovate.
Stop viewing AI as your replacement.
Start seeing it as your rocket fuel.
Because in 2025:
➝ Learners will lead
➝ Adapters will advance
➝ Complainers will vanish
The choice?
It's always been yours.
It goes even further - now AI has been trained to create consistent.
//
Repost this ⇄
//
Follow me for daily posts on emerging tech and growth
#ai#artificialintelligence#innovation#tech#technology#aitools#machinelearning#automation#techreview#education#meme#Tom and Jerry#cartoon#animation#cat#mouse#robot#artificial intelligence#job loss#humor#classic#Machine Learning#Deep Learning#Natural Language Processing (NLP)#Generative AI#AI Chatbots#AI Ethics#Computer Vision#Robotics#AI Applications
4 notes
·
View notes
Text
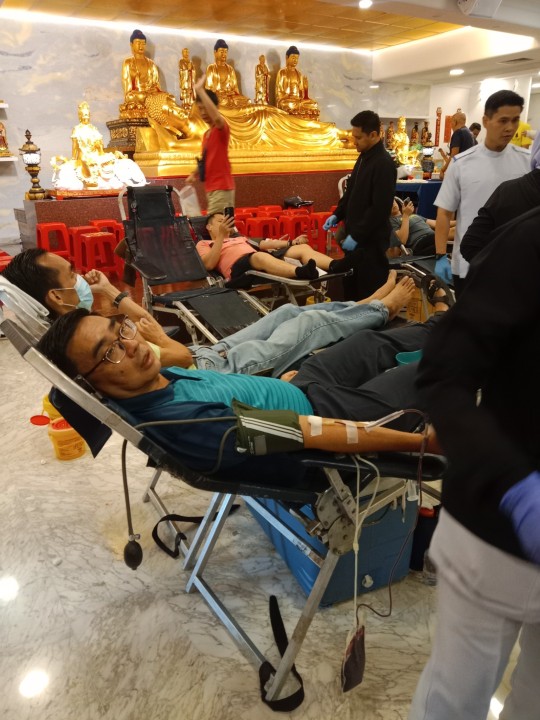

Donated blood for 95 times at PJ Kwan Inn Teng on Labour Day.
#volunteering#coding#machine learning#university#language#college life#exercise#running#delicious#gym
2 notes
·
View notes
Text
Key Differences Between AI and Human Communication: Mechanisms, Intent, and Understanding
The differences between the way an AI communicates and the way a human does are significant, encompassing various aspects such as the underlying mechanisms, intent, adaptability, and the nature of understanding. Here’s a breakdown of key differences:
1. Mechanism of Communication:
AI: AI communication is based on algorithms, data processing, and pattern recognition. AI generates responses by analyzing input data, applying pre-programmed rules, and utilizing machine learning models that have been trained on large datasets. The AI does not understand language in a human sense; instead, it predicts likely responses based on patterns in the data.
Humans: Human communication is deeply rooted in biological, cognitive, and social processes. Humans use language as a tool for expressing thoughts, emotions, intentions, and experiences. Human communication is inherently tied to understanding and meaning-making, involving both conscious and unconscious processes.
2. Intent and Purpose:
AI: AI lacks true intent or purpose. It responds to input based on programming and training data, without any underlying motivation or goal beyond fulfilling the tasks it has been designed for. AI does not have desires, beliefs, or personal experiences that inform its communication.
Humans: Human communication is driven by intent and purpose. People communicate to share ideas, express emotions, seek information, build relationships, and achieve specific goals. Human communication is often nuanced, influenced by context, and shaped by personal experiences and social dynamics.
3. Understanding and Meaning:
AI: AI processes language at a syntactic and statistical level. It can identify patterns, generate coherent responses, and even mimic certain aspects of human communication, but it does not truly understand the meaning of the words it uses. AI lacks consciousness, self-awareness, and the ability to grasp abstract concepts in the way humans do.
Humans: Humans understand language semantically and contextually. They interpret meaning based on personal experience, cultural background, emotional state, and the context of the conversation. Human communication involves deep understanding, empathy, and the ability to infer meaning beyond the literal words spoken.
4. Adaptability and Learning:
AI: AI can adapt its communication style based on data and feedback, but this adaptability is limited to the parameters set by its algorithms and the data it has been trained on. AI can learn from new data, but it does so without understanding the implications of that data in a broader context.
Humans: Humans are highly adaptable communicators. They can adjust their language, tone, and approach based on the situation, the audience, and the emotional dynamics of the interaction. Humans learn not just from direct feedback but also from social and cultural experiences, emotional cues, and abstract reasoning.
5. Creativity and Innovation:
AI: AI can generate creative outputs, such as writing poems or composing music, by recombining existing patterns in novel ways. However, this creativity is constrained by the data it has been trained on and lacks the originality that comes from human creativity, which is often driven by personal experience, intuition, and a desire for expression.
Humans: Human creativity in communication is driven by a complex interplay of emotions, experiences, imagination, and intent. Humans can innovate in language, create new metaphors, and use language to express unique personal and cultural identities. Human creativity is often spontaneous and deeply tied to individual and collective experiences.
6. Emotional Engagement:
AI: AI can simulate emotional engagement by recognizing and responding to emotional cues in language, but it does not experience emotions. Its responses are based on patterns learned from data, without any true emotional understanding or empathy.
Humans: Human communication is inherently emotional. People express and respond to emotions in nuanced ways, using tone, body language, and context to convey feelings. Empathy, sympathy, and emotional intelligence play a crucial role in human communication, allowing for deep connections and understanding between individuals.
7. Contextual Sensitivity:
AI: AI's sensitivity to context is limited by its training data and algorithms. While it can take some context into account (like the previous messages in a conversation), it may struggle with complex or ambiguous situations, especially if they require a deep understanding of cultural, social, or personal nuances.
Humans: Humans are highly sensitive to context, using it to interpret meaning and guide their communication. They can understand subtext, read between the lines, and adjust their communication based on subtle cues like tone, body language, and shared history with the other person.
8. Ethical and Moral Considerations:
AI: AI lacks an inherent sense of ethics or morality. Its communication is governed by the data it has been trained on and the parameters set by its developers. Any ethical considerations in AI communication come from human-designed rules or guidelines, not from an intrinsic understanding of right or wrong.
Humans: Human communication is deeply influenced by ethical and moral considerations. People often weigh the potential impact of their words on others, considering issues like honesty, fairness, and respect. These considerations are shaped by individual values, cultural norms, and societal expectations.
The key differences between AI and human communication lie in the underlying mechanisms, the presence or absence of intent and understanding, and the role of emotions, creativity, and ethics. While AI can simulate certain aspects of human communication, it fundamentally operates in a different way, lacking the consciousness, experience, and meaning-making processes that characterize human interaction.
#philosophy#epistemology#knowledge#learning#education#chatgpt#metaphysics#ontology#AI Communication#Human Communication#Language Understanding#Natural Language Processing#Machine Learning#Cognitive Science#Artificial Intelligence#Emotional Intelligence#Ethics in AI#Language and Meaning#Human-AI Interaction#Contextual Sensitivity#Creativity in Communication#Intent in Communication#Pattern Recognition
5 notes
·
View notes