#generative ai framework
Explore tagged Tumblr posts
Text
Know about Needle: Enterprise Generative AI Framework
0 notes
Text
Discover the Needle GenAI Framework, offering hyper-personalized and holistic solutions with intelligent adaptability. Streamline decision-making with our low-code platform and explore tailored use cases in Finance, Legal, Sales, Marketing, and more. Sign up for a free demo or trial today!
1 note
·
View note
Text
Natasha Romanoff didn't bat her eye at experimental new tech – it was part of the job, after all. So when the SHIELD labrats asked her to test-drive a new holographic simulation... "Why the hell not," was her quick and quippy reply. It was an early developmental prototype of a platform called the Framework, designed by some young SHIELD recruit as a consequence-free space for training simulations.
Seemed innocent enough to give it a whirl.
And again, for Nat, when the training went off the rails and she found herself trapped in an unstable simulated reality... she wasn't surprised. The techs in the surface world would bring her back eventually – all she had to do was stay sane in this troublesome virtual world in the meantime.
As the system continued to glitch out and threw new bugs her way, she was surprised to realize that not all of them were dangerous. When the program duplicated her, that's when she raised her first eyebrow. Now, having been tripled, Nat started to worry.
Although these other Nat simulations seemed pretty laid back, despite her initial concerns. And trapped in a room with three of her, all eager to pass the time... Natasha found herselves game for another type of... experiment.
* * * * *
I was really happy with my first Nat attempt – Love, Natasha – but couldn't help but do more for our girl. I wanted to really give her Avengers-era character a chance to shine 😈.
#natasha romanoff#fake movie poster#selfcest#wlw#ai art#ai artwork#ai generated#ai image#mcu#mcu headcanons#scarlett johansson#natasha mcu#natasha romanov#natasha poly#natasha x reader#black widow#avengers#agents of shield#framework#ai fanart
76 notes
·
View notes
Text
Cosmic Genesis: How Black Holes Might Be Giving Birth to New Universes
The human quest for understanding the universe has led to numerous groundbreaking discoveries, each weaving a more intricate tapestry of our cosmic landscape. A recent theoretical framework, pioneered by Professor Nikodem Poplawski, proposes a revolutionary concept: every black hole creates a new, growing universe inside its event horizon. This idea, rooted in the Einstein-Cartan Theory, introduces torsion to the fabric of spacetime, avoiding gravitational singularities and transforming our understanding of black holes and the multiverse.
By incorporating torsion, the theory predicts that matter within a black hole, instead of collapsing into a singularity, reaches a “big bounce” and then expands into a new, closed universe. This challenges our current understanding of the cosmos, suggesting that our universe is a vast, cosmic nursery, giving birth to billions of “baby universes” through black holes. Each black hole, once thought to be a region of spacetime from which nothing can escape, now becomes a gateway to a new, unobservable universe, raising fundamental questions about the nature of reality and our place within the multiverse.
The introduction of torsion also has far-reaching implications for the long-standing gap between general relativity and quantum mechanics. By violating the linearity of quantum mechanics, torsion favors the pilot-wave interpretation, where particles have definite positions, guided by a wave function. This non-linear aspect of torsion could provide a crucial link in the quantum gravity puzzle, enabling a more unified understanding of the universe, from the smallest subatomic particles to the vast expanse of cosmic structures.
While experimental verification of torsion poses significant challenges, it is not insurmountable. Future astronomical observations of the early universe, utilizing gravitational waves and neutrinos, may uncover the distinctive signature of torsion in the cosmic microwave background radiation. Additionally, cutting-edge particle physics experiments could reveal the extended sizes of elementary particles, predicted by the theory, or the effects of non-commutative momentum in high-energy collisions, providing a tantalizing prospect of empirical confirmation.
The profound implications of Poplawski’s theory, if confirmed, would revolutionize our understanding of black holes, transforming them from cosmic dead ends to gateways of creation. The multiverse, once a topic of speculative debate, would gain a theoretical foundation, with our universe being just one of many, interconnected through a web of black holes. This pursuit of knowledge, even if verification takes decades or centuries, embodies the spirit of scientific inquiry, driving us to push the boundaries of human understanding and illuminating the intricate, ever-unfolding tapestry of the cosmos.
Nikodem Poplawski: The Unknown Revolutionary Theory of Black Holes (This Is World, March 2025)
youtube
N. Poplawski: Big Bounce and inflation from spin and torsion (Gravity and Cosmology, Jagiellonian University, Kraków, May 2020)
youtube
Monday, March 3, 2025
#theoretical physics#general relativity#quantum mechanics#quantum gravity#unified field theory#cosmology#multiverse#astronomy#astrophysics#black holes#gravitational waves#neutrinos#torsion#spacetime#particle physics#theoretical framework#experimental verification#pilot wave interpretation#nonlinear quantum mechanics#interview#ai assisted writing#machine art#Youtube#presentation
17 notes
·
View notes
Text
i will admit that after rereading the pizzaplex books, I've warmed up to the mimic a little. I tried reading them in the best sorta chronological order i could figure, and that was a much more interesting way to read them than just jumping all over the place!
i'm still grumpy about the whole storyteller and mimic1 and them trying to tie the books to the games stuff, but. the mimic in and of itself is pretty fun, at least.
#i am also still completely adamant that glitchtrap is a different branch of the ai from the one in the books#and that whatever was left of william's soul/agony/whatever got tangled up in it and the ai just sorta filled in the gaps#so he's like. a little scrap of william using the ai's framework to try and make itself whole again#resulting in something that. yknow. remembers the broad strokes of being william and his life#but winds up being like a caricature of who he was. trying to extrapolate his entire being from a tiny sliver of him#that's how i like interpreting him anyways!!#an echo that's reverberated so many times that it's hard to tell what the original words were besides the general sounds that are left#like a messed up game of telephone
4 notes
·
View notes
Text
Scientists use generative AI to answer complex questions in physics
New Post has been published on https://thedigitalinsider.com/scientists-use-generative-ai-to-answer-complex-questions-in-physics/
Scientists use generative AI to answer complex questions in physics
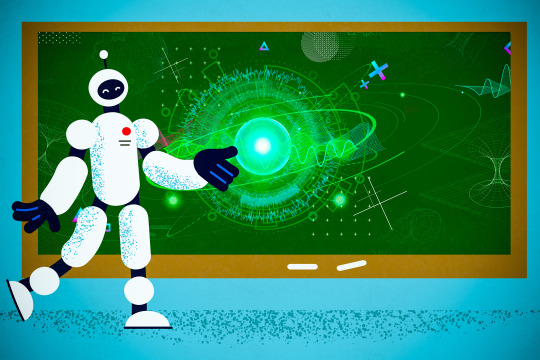
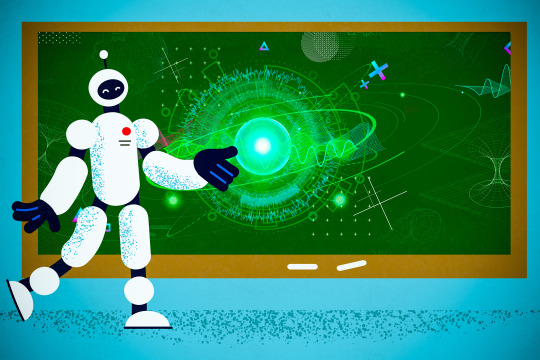
When water freezes, it transitions from a liquid phase to a solid phase, resulting in a drastic change in properties like density and volume. Phase transitions in water are so common most of us probably don’t even think about them, but phase transitions in novel materials or complex physical systems are an important area of study.
To fully understand these systems, scientists must be able to recognize phases and detect the transitions between. But how to quantify phase changes in an unknown system is often unclear, especially when data are scarce.
Researchers from MIT and the University of Basel in Switzerland applied generative artificial intelligence models to this problem, developing a new machine-learning framework that can automatically map out phase diagrams for novel physical systems.
Their physics-informed machine-learning approach is more efficient than laborious, manual techniques which rely on theoretical expertise. Importantly, because their approach leverages generative models, it does not require huge, labeled training datasets used in other machine-learning techniques.
Such a framework could help scientists investigate the thermodynamic properties of novel materials or detect entanglement in quantum systems, for instance. Ultimately, this technique could make it possible for scientists to discover unknown phases of matter autonomously.
“If you have a new system with fully unknown properties, how would you choose which observable quantity to study? The hope, at least with data-driven tools, is that you could scan large new systems in an automated way, and it will point you to important changes in the system. This might be a tool in the pipeline of automated scientific discovery of new, exotic properties of phases,” says Frank Schäfer, a postdoc in the Julia Lab in the Computer Science and Artificial Intelligence Laboratory (CSAIL) and co-author of a paper on this approach.
Joining Schäfer on the paper are first author Julian Arnold, a graduate student at the University of Basel; Alan Edelman, applied mathematics professor in the Department of Mathematics and leader of the Julia Lab; and senior author Christoph Bruder, professor in the Department of Physics at the University of Basel. The research is published today in Physical Review Letters.
Detecting phase transitions using AI
While water transitioning to ice might be among the most obvious examples of a phase change, more exotic phase changes, like when a material transitions from being a normal conductor to a superconductor, are of keen interest to scientists.
These transitions can be detected by identifying an “order parameter,” a quantity that is important and expected to change. For instance, water freezes and transitions to a solid phase (ice) when its temperature drops below 0 degrees Celsius. In this case, an appropriate order parameter could be defined in terms of the proportion of water molecules that are part of the crystalline lattice versus those that remain in a disordered state.
In the past, researchers have relied on physics expertise to build phase diagrams manually, drawing on theoretical understanding to know which order parameters are important. Not only is this tedious for complex systems, and perhaps impossible for unknown systems with new behaviors, but it also introduces human bias into the solution.
More recently, researchers have begun using machine learning to build discriminative classifiers that can solve this task by learning to classify a measurement statistic as coming from a particular phase of the physical system, the same way such models classify an image as a cat or dog.
The MIT researchers demonstrated how generative models can be used to solve this classification task much more efficiently, and in a physics-informed manner.
The Julia Programming Language, a popular language for scientific computing that is also used in MIT’s introductory linear algebra classes, offers many tools that make it invaluable for constructing such generative models, Schäfer adds.
Generative models, like those that underlie ChatGPT and Dall-E, typically work by estimating the probability distribution of some data, which they use to generate new data points that fit the distribution (such as new cat images that are similar to existing cat images).
However, when simulations of a physical system using tried-and-true scientific techniques are available, researchers get a model of its probability distribution for free. This distribution describes the measurement statistics of the physical system.
A more knowledgeable model
The MIT team’s insight is that this probability distribution also defines a generative model upon which a classifier can be constructed. They plug the generative model into standard statistical formulas to directly construct a classifier instead of learning it from samples, as was done with discriminative approaches.
“This is a really nice way of incorporating something you know about your physical system deep inside your machine-learning scheme. It goes far beyond just performing feature engineering on your data samples or simple inductive biases,” Schäfer says.
This generative classifier can determine what phase the system is in given some parameter, like temperature or pressure. And because the researchers directly approximate the probability distributions underlying measurements from the physical system, the classifier has system knowledge.
This enables their method to perform better than other machine-learning techniques. And because it can work automatically without the need for extensive training, their approach significantly enhances the computational efficiency of identifying phase transitions.
At the end of the day, similar to how one might ask ChatGPT to solve a math problem, the researchers can ask the generative classifier questions like “does this sample belong to phase I or phase II?” or “was this sample generated at high temperature or low temperature?”
Scientists could also use this approach to solve different binary classification tasks in physical systems, possibly to detect entanglement in quantum systems (Is the state entangled or not?) or determine whether theory A or B is best suited to solve a particular problem. They could also use this approach to better understand and improve large language models like ChatGPT by identifying how certain parameters should be tuned so the chatbot gives the best outputs.
In the future, the researchers also want to study theoretical guarantees regarding how many measurements they would need to effectively detect phase transitions and estimate the amount of computation that would require.
This work was funded, in part, by the Swiss National Science Foundation, the MIT-Switzerland Lockheed Martin Seed Fund, and MIT International Science and Technology Initiatives.
#ai#approach#artificial#Artificial Intelligence#Bias#binary#change#chatbot#chatGPT#classes#computation#computer#Computer modeling#Computer Science#Computer Science and Artificial Intelligence Laboratory (CSAIL)#Computer science and technology#computing#crystalline#dall-e#data#data-driven#datasets#dog#efficiency#Electrical Engineering&Computer Science (eecs)#engineering#Foundation#framework#Future#generative
2 notes
·
View notes
Text
The Power of the 'Octopus' Approach: Integrating Multiple Digital Channels for
In today’s hyper-connected world, customers move swiftly across platforms—searching, browsing, comparing, and purchasing within seconds. To meet them at every point in their journey, brands need a unified, flexible strategy. Enter the 'Octopus' Approach, a model that integrates multiple digital channels—supported by powerful tools like AI-powered generative chat bots—to maximize marketing impact.
🐙 What Is the 'Octopus' Approach?
Just like an octopus extends its arms in different directions while staying connected to one central brain, the 'Octopus' digital strategy uses various platforms (SEO, social media, paid ads, email, etc.) to deliver a synchronized, seamless experience. It allows each “arm” to work independently yet cohesively—ensuring brand alignment, efficiency, and conversion.
The central brain of this strategy? Increasingly, it’s the AI-powered generative chat bot that connects the dots in real time.
💬 The Role of AI-Powered Generative Chat Bots in the Octopus Model
An AI-powered generative chat bot is more than just a virtual assistant—it’s a real-time engagement engine that:
Understands user behavior across platforms
Delivers personalized experiences based on data
Automates lead generation and nurturing
Supports omnichannel engagement (website, WhatsApp, Facebook, etc.)
With its advanced NLP and machine learning capabilities, it becomes the “nerve center” of the octopus—helping each channel react and evolve intelligently.
🌐 Integrating Multiple Digital Channels Effectively
Here’s how businesses are applying the Octopus Approach with AI at the core:
1. SEO + AI Chat Bot
Boost organic traffic with strategic content, then convert that traffic with a chat bot that answers queries instantly and guides users toward conversions.
2. Social Media + Chat Automation
Automate replies to DMs, comments, and story responses using AI bots to enhance community engagement—without missing a beat.
3. Email Marketing + AI Insights
Analyze customer behavior using chat bot data to send smarter, more relevant email campaigns.
4. Paid Ads + Real-Time Interaction
Don't just land users on a static page—greet them with an AI-powered bot that can qualify leads and answer objections in real-time.
📊 Benefits of an Integrated Digital Strategy Powered by AI
✔️ Unified customer journey across platforms ✔️ Real-time personalization and data collection ✔️ 24/7 engagement with no human limitations ✔️ Higher conversions and reduced response time ✔️ Scalable automation for growing businesses
💡 Final Thoughts
In an age where attention spans are short and competition is fierce, integration is everything. The Octopus Approach, fueled by tools like an AI-powered generative chat bot, empowers brands to be proactive, responsive, and deeply connected with their audiences—everywhere, all the time.
Are you ready to connect all your marketing arms with AI at the center?
1 note
·
View note
Text
Peran Alat Pembelajaran Mesin dalam Meningkatkan Kemampuan AI di 2025
Pembelajaran mesin (machine learning) adalah cabang dari kecerdasan buatan (AI) yang memungkinkan sistem untuk belajar dan meningkatkan performa mereka tanpa pemrograman eksplisit. Dalam beberapa tahun terakhir, perkembangan pembelajaran mesin telah menjadi pendorong utama kemajuan AI. Pada tahun 2025, peran alat pembelajaran mesin semakin signifikan dalam meningkatkan kemampuan AI, baik dalam…
#advanced AI frameworks#AI collaboration#AI development#AI ethics#AI for business#AI in 2025#AI research#AI scalability#AI tools#artificial intelligence trends#deep learning#federated learning#future of AI#generative AI#graph-based machine learning#machine learning#multimodal learning#PyTorch Geometric#TensorFlow Federated
0 notes
Text
Needle is a cutting-edge Generative AI framework designed to transform businesses across industries. It enables organizations to harness the power of AI for intelligent automation, personalized customer experiences, and data-driven insights. With advanced capabilities, Needle empowers teams to streamline processes, boost efficiency, and unlock new opportunities for innovation. Redefine what’s possible with Needle - your partner in the AI revolution.
0 notes
Text
How generative AI framework offers efficiency in legal drafting?
In the legal industry, contract drafting has long been a time-consuming and intricate task. Traditional methods often involve meticulous manual work, which increases the risk of human error and leads to high costs.
How much time do you spend manually renumbering paragraphs or re-entering client comments lost during copying and pasting?
How long does it take to perfect your document?
How much time it takes to refer to different documents and extract accurate information from those documents?
If you answered these questions with — too much or too long, you are not alone. Lawyers spend roughly 40–60% of their time drafting and reviewing contracts. The hassles of traditional methods are evident.
According to a study by the American Bar Association, AI-based document review can improve the speed and accuracy of document review by up to 90%.
This is where generative AI solutions come into play. GenAI-powered automated drafting and review free up substantial time, enabling law professionals to dedicate more attention to their cases.
In this blog, we will explore how generative AI helps with contract drafting, its benefits, and use cases.
The challenges of traditional contract drafting
Time-consuming non-billable hours: Drafting a comprehensive and legally sound contract demands significant time and effort. It often entails multiple rounds of revisions and consultations among legal teams and clients, prolonging the contract lifecycle.
Lack of standardization: Contracts drafted and reviewed manually are often inconsistent in language and clauses. Also, up-to-date versions of contracts are not easily accessible to the lawyers.
Risk of human errors: Manual drafting increases the vulnerability to errors such as omissions, inconsistencies, and inaccuracies. These errors can compromise the legal validity of contracts, potentially leading to disputes and legal liabilities.
High costs: The labor-intensive nature of traditional contract drafting translates into substantial financial expenditures. Law firms and their clients bear costs associated with prolonged drafting cycles, extensive review processes, and the need for specialized legal expertise.
Where traditional methods fail, generative AI solutions for the legal industry help address these issues. Let’s explore how the technology helps in detail.
How legal document automation with generative AI addresses these challenges
Automate repetitive tasks
Automate routine and repetitive tasks such as clause insertion, formatting, and renumbering of paragraphs. This reduces the manual workload on legal professionals, allowing them to focus on more complex aspects of contract drafting and other billable opportunities.
Draft contracts efficiently
NLP algorithms analyze and understand legal language, enabling AI systems to draft contracts that adhere to legal standards and best practices. This ensures the inclusion of all necessary clauses and provisions, reducing the risk of human errors and omissions.
Navigate the future with generative AI: A step-by-step guide for business implementation
Discover the benefits, understand the implementation process, and follow a detailed step-by-step guide to successfully integrate generative AI into your operations.
Download
Template generation and customization
Create and customize contract templates based on specific legal requirements and client needs. This standardization ensures consistency across all contracts.
Real-time collaboration and review
AI facilitates real-time collaboration among legal teams, enabling multiple stakeholders to review and edit contracts simultaneously. This streamlines the review process, reduces delays, and enhances overall document production efficiency for litigation and other legal matters.
Explore the benefits of our robust generative AI framework, Needle! Get a free demo
Predictive analytics and risk management
AI tools use predictive analytics to foresee potential legal risks in the documentation and suggest preventive measures. By analyzing patterns and trends in legal data, AI helps in identifying and mitigating risks early in the drafting process.
Efficiency in legal drafting and research
Generative AI integrates AI legal research capabilities, allowing legal professionals to access comprehensive and up-to-date legal information quickly. This supports informed decision-making and ensures that contracts are aligned with current legal standards.
Contract lifecycle management
Key benefits of contract drafting with AI
Enhanced accuracy and reduced errors: By automating repetitive tasks and leveraging pre-approved clauses, you can minimize the risk of human error.
Cost savings and resource optimization: AI reduces the time and resources needed for contract drafting, freeing up lawyers to focus on higher-value strategic tasks.
Expedited document production: Accelerate the drafting process by significantly reducing the time required to produce comprehensive legal documents. This leads to faster turnaround times and improved client satisfaction.
Improved client relationship: Enhance the overall client experience by providing accurate and timely legal documents. Clients benefit from faster service delivery and the assurance that their legal matters are handled with the utmost precision.
Suggested: 5 tips to implement generative AI in your organization
Real-life use cases of generative AI in the legal industry
Dechert LLP
Dechert LLP is a multinational American law firm with more than 900 lawyers. The company developed DechertMind, a proprietary suite of generative AI tools designed to transform law firm operations. Dechert LLP used their generative AI solutions for:
Automated contract drafting
Enhanced legal research
Improved document review
Client service optimization
Dentons
Dentons is the world’s largest global law firm by number of lawyers and the 6th-largest law firm by revenue. The company launched a client-secure version of ChatGPT to enhance client interactions and legal services. This solution offered them the following benefits:
Secure client communication
Efficient legal support
Legal AI assistant
Enhanced legal research
McGuireWoods
McGuireWoods was established in 1834 and is the largest law firm in the US. They embraced AI innovation through its partnership with Casetext Co-Counsel. The integration of generative AI in legal research ensures prompt resolution of client issues and facilitates the provision of accurate information for client documentation.
Enhanced legal research
Faster contract drafting
Improved operational efficiency
The future of generative AI in the legal industry
Integration with blockchain: Combining AI with blockchain technology can provide greater transparency and security in contract management.
Personalized contract templates: Generative AI will be capable of creating highly customized contract templates tailored to specific client needs and industry standards.
Enhanced predictive analytics: By analyzing vast amounts of data from previous cases and contracts, generative AI solutions will provide more accurate risk assessments and suggest preventive measures, aiding legal professionals in making more informed decisions.
AI-driven negotiation support: AI tools will assist in the negotiation phase by providing real-time suggestions and counterproposals based on historical data and legal precedents.
Increased adoption of generative AI in small and med-size legal firms: While large law firms are already embracing AI, the future will see increased adoption among small and medium-sized firms. As AI technology becomes more accessible and cost-effective, these firms will leverage generative AI to enhance their competitiveness and service offerings.
Efficiency in legal drafting and compliance: AI will play a critical role in ensuring authentication of legal documents. It will also ensure legal compliance by continuously monitoring regulatory changes and updating contract templates accordingly.
Leverage legal document automation to achieve operational excellence
As AI technology advances, it’s impact and adoption in the legal industry will see a significant growth. While many companies are utilizing generative AI for time-consuming task of contract drafting, the technology offers more opportunities. With AI-powered tools, law firms can gain a competitive advantage.
One notable example of such a tool is Needle, a generative AI framework developed by Softweb Solutions. Needle integrates seamlessly into legal workflows, providing robust AI capabilities for efficient contract drafting. To explore more benefits and use cases of our GenAI framework, contact our AI experts.
Originally published at https://www.softwebsolutions.com on July 17, 2024.
#legal GenAI solutions#generative AI solutions#generative AI solutions for the legal industry#generative AI framework
0 notes
Text
Transform your approach to business intelligence with intuitive AI interfaces. Drive innovation and growth like never before.
#AI-Enabled Business Reporting Solutions#AI-Powered Business Intelligence#AI-Powered Data Visualization#Generative AI in BI Frameworks#Revolutionary Power of Generative AI
0 notes
Text
Transform your approach to business intelligence with intuitive AI interfaces. Drive innovation and growth like never before.
#AI-Enabled Business Reporting Solutions#AI-Powered Business Intelligence#AI-Powered Data Visualization#Generative AI in BI Frameworks#Revolutionary Power of Generative AI
0 notes
Text
Transform your approach to business intelligence with intuitive AI interfaces. Drive innovation and growth like never before.
#AI-Enabled Business Reporting Solutions#AI-Powered Business Intelligence#AI-Powered Data Visualization#Generative AI in BI Frameworks#Revolutionary Power of Generative AI
0 notes
Text
Smart Insights: AI Interfaces Driving BI Evolution
In such an ever-changing business arena, wisdom is one of the key assets and you have to rely not only on your intuition. Using Data Analytic Tools and Advanced Artificial Intelligence Models, one would be able to deduct the necessary strategic choices. The old trend was that the usage of such instruments mostly depended on particular experts to do it which happened to confine the utilization of these tools to most of the big organizations with their reliable data science teams. Yet the emergence of Generative AI Interfaces for instance is turning the tide beyond this traditional model of analytics democratizing access to advanced analytics and making it possible for small companies to be equipped with sophisticated analytics capabilities with unprecedented speed and thus making better decisions.
The AI Predictive Analytics has developed to a game changer in the realms of strategic decision making since it allows us to e pump up performance and business tremendously by predicting even the advanced matters of level. Leveraging AI-Based Forecasting Algorithms allows companies to react in a premitive manner to their mindsets, while foreclosed emerging opportunities and eliminating risks. By doing so, companies gain a significant competitive advantage in their industries. On the other hand, due to the complex nature of traditional analytics platforms, their adoption has been limited by the fact that to efficiently operate them one needs to be properly trained while an expert is preferable for navigation.
And these are the two areas in which Generative AI plays a major role, offering an innovative way to handle Business Intelligence (BI) problems through artificial intelligence, aimed at automating and simplifying the process. In contrast to traditional BI solutions that contains queries and examines fact-based static reports, Generative AI interfaces deploy machine intelligence which makes immediate relevant insights based on the unique needs and objectives of user. As a result, the individuals cut off from the loop manually for the model have required tasks been automated, not only has it eliminated human manipulation but also enabled users to discover the hidden patterns and correlations that were ignored by human analysts.
The use of Generative AI in BI applications proves to have various optimization options. First of all, these approaches increase scalability and accessibility of analytics solutions, thus granting organizations an opportunity to spread the advanced analytics capabilities not only across the departments but also across the functions without a need of specialised knowledge. AI-Empowered Business Analytics Software for Finance or Marketing teams will have AI-Powered Smart Interfaces which allow the users to derive actionable results with minimum learning.
Additionally, machine learning with AI, smart analytics and generative AI variation introduces a huge leap in the AI-driven wisdom capability allowing the organization to make informed decisions with certainty and lucidity. These systems transform huge data sets into valuable patterns which eventually amplify human decision-making proficiencies, thus, executives can tackle uncertain strategic issues hand in hand with analyzing capability. Whether it’s incorporating artificial supply chain management, demand forecasting or new revenue generation, AI Generative AI powered businesses in exploring new frontiers in their data resources.
The main example of the Generation AI is that, it is capable of adapting and developing new algorithms all the time as it’s instructed and the new information is provided. The ongoing cycle of continuous learning is the direct way towards improving the accuracy and reliability of AI-based forecasting. These points also give organizations the chance to stay nimble and adaptable to the dynamic business environment. Therefore, the companies will benefit from having a secured place in the future marketplace because generative AI is an effective and a revolutionary tool that enables them to stop losing to the competitors.
Besides, the democratization of Generative AI for Business Intelligence spurs innovation and entrepreneurship into a whole new dimension. It does this by lowering the door where most people can enter but in addition, it equips individuals with advanced analytics tools which they use to not only make data driven decisions but also try out new things. It isn’t sequestered to a small group of larger companies but rather is open to any business that wants to utilize it, and they are not discriminated against because of their size. Whether it is a startup looking to disrupt an industry or a small business seeking to optimise its operations, Generative AI interfaces level the playing field, enabling entities of every dimension to compete and succeed in digital economy.
In conclusion, the implementation of Generative AI inside Business Intelligence structure is a pivotal step for how to unlock the power of data with the purpose of encouraging the growth and creativity of organizations. Through the act of democratizing the advanced analytics tools and the premature artificial intelligence analytics, businesses gain wide access to advanced methods of decision making, faster. It can help unraveling hidden insights or making forecasts, or simply optimizing operations. This is how Generative AI interfaces become a new horizon of opportunities with which organization need to catch-up. Furthermore the journey of this disruptive technology is yet to discover its full extent and hence the future is highly satisfying.
#Advanced Analytics Tools#AI Predictive Analytics for Strategic Decision-Making#AI Predictive Analytics#AI-Based Forecasting Algorithms#AI-Based Forecasting#AI-Enabled Business Reporting Solutions#AI-Powered Data Visualization#Decision Support Systems with AI-Driven Insights#Generative AI in AI-Powered Business Intelligence#Generative AI in Business Intelligence Frameworks#Revolutionary Power of Generative AI
0 notes
Text
Latest AI Regulatory Developments:
As artificial intelligence (AI) continues to transform industries, governments worldwide are responding with evolving regulatory frameworks. These regulatory advancements are shaping how businesses integrate and leverage AI technologies. Understanding these changes and preparing for them is crucial to remain compliant and competitive. Recent Developments in AI Regulation: United Kingdom: The…
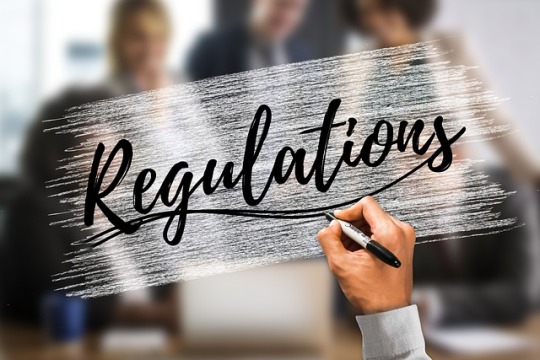
View On WordPress
#AI#AI compliance#AI data governance#AI democratic values#AI enforcement#AI ethics#AI for humanity#AI global norms#AI human rights#AI industry standards#AI innovation#AI legislation#AI penalties#AI principles#AI regulation#AI regulatory framework#AI risk classes#AI risk management#AI safety#AI Safety Summit 2023#AI sector-specific guidance#AI transparency requirements#artificial intelligence#artificial intelligence developments#Bletchley Declaration#ChatGPT#China generative AI regulation#Department for Science Innovation and Technology#EU Artificial Intelligence Act#G7 Hiroshima AI Process
1 note
·
View note
Text
Unveil the power of Mora, an innovative multi-agent framework that’s reshaping the landscape of video generation. Experience how Mora mimics and extends the capabilities of OpenAI's Sora, marking a new era in open-source AI.
#Mora#AI#OpenSourceai#Sora#videoGeneration#text2video#Image2Video#Video2video#multi-agent-framework#MicrosoftResearch#openai#artificial intelligence#open source#ai video generator
0 notes