#ethical challenges in AI
Explore tagged Tumblr posts
Text
The Future of GPT: An In-Depth Analysis
1. Introduction Generative Pre-trained Transformer (GPT) technology has changed the way artificial intelligence interacts with human language. Since its inception, GPT has been pivotal in advancing natural language understanding and generation, making it a powerful tool across many sectors. As we look to the future, understanding the potential of GPT’s evolution, its applications, and the…
#Advanced AI models#AI advancements#AI advancements in 2024#AI in healthcare#ethical challenges in AI#Ethical guidelines for developing GPT models#Future of artificial intelligence#Future role of AI in education#GPT applications in business#How will GPT impact the future of work?#human-AI interaction#multimodal capabilities#Natural language understanding in AI#OpenAI and GPT models
0 notes
Text
the human urge to pack bond with the stupidest most silly little guys is incredible
#Literally a random number generator and every time I’m like YESSS KING YOURE SO RIGHT#also dougdoug pajama sam but in a more ethically-challenging way bc that’s an actual chat ai#jacksfilms
10 notes
·
View notes
Text
Building Ethical AI: Challenges and Solutions
Artificial Intelligence (AI) is transforming industries worldwide, creating opportunities for innovation, efficiency, and growth. According to recent statistics, the global AI market is expected to grow from $59.67 billion in 2021 to $422.37 billion by 2028, at a CAGR of 39.4% during the forecast period. Despite the tremendous potential, developing AI technologies comes with significant ethical challenges. Ensuring that AI systems are designed and implemented ethically is crucial to maximizing their benefits while minimizing risks. This article explores the challenges in building ethical AI and offers solutions to address these issues effectively.
Understanding Ethical AI
Ethical AI refers to the development and deployment of AI systems in a manner that aligns with widely accepted moral principles and societal values. It encompasses several aspects, including fairness, transparency, accountability, privacy, and security. Ethical AI aims to prevent harm and ensure that AI technologies are used to benefit society as a whole.
The Importance of Ethical AI
Trust and Adoption: Ethical AI builds trust among users and stakeholders, encouraging widespread adoption.
Legal Compliance: Adhering to ethical guidelines helps companies comply with regulations and avoid legal repercussions.
Social Responsibility: Developing ethical AI reflects a commitment to social responsibility and the well-being of society.
Challenges in Building Ethical AI
1. Bias and Fairness
AI systems can inadvertently perpetuate or even amplify existing biases present in the training data. This can lead to unfair treatment of individuals based on race, gender, age, or other attributes.
Solutions:
Diverse Data Sets: Use diverse and representative data sets to train AI models.
Bias Detection Tools: Implement tools and techniques to detect and mitigate biases in AI systems.
Regular Audits: Conduct regular audits to ensure AI systems remain fair and unbiased.
2. Transparency and Explainability
AI systems, especially those based on deep learning, can be complex and opaque, making it difficult to understand their decision-making processes.
Solutions:
Explainable AI (XAI): Develop and use explainable AI models that provide clear and understandable insights into how decisions are made.
Documentation: Maintain thorough documentation of AI models, including data sources, algorithms, and decision-making criteria.
User Education: Educate users and stakeholders about how AI systems work and the rationale behind their decisions.
3. Accountability
Determining accountability for AI-driven decisions can be challenging, particularly when multiple entities are involved in developing and deploying AI systems.
Solutions:
Clear Governance: Establish clear governance structures that define roles and responsibilities for AI development and deployment.
Ethical Guidelines: Develop and enforce ethical guidelines and standards for AI development.
Third-Party Audits: Engage third-party auditors to review and assess the ethical compliance of AI systems.
4. Privacy and Security
AI systems often rely on vast amounts of data, raising concerns about privacy and data security.
Solutions:
Data Anonymization: Use data anonymization techniques to protect individual privacy.
Robust Security Measures: Implement robust security measures to safeguard data and AI systems from breaches and attacks.
Consent Management: Ensure that data collection and use comply with consent requirements and privacy regulations.
5. Ethical Design and Implementation
The design and implementation of AI systems should align with ethical principles from the outset, rather than being an afterthought.
Solutions:
Ethical by Design: Incorporate ethical considerations into the design and development process from the beginning.
Interdisciplinary Teams: Form interdisciplinary teams that include ethicists, sociologists, and other experts to guide ethical AI development.
Continuous Monitoring: Continuously monitor AI systems to ensure they adhere to ethical guidelines throughout their lifecycle.
AI Development Companies and Ethical AI
AI development companies play a crucial role in promoting ethical AI. By adopting ethical practices, these companies can lead the way in creating AI technologies that benefit society. Here are some key steps that AI development companies can take to build ethical AI:
Promoting Ethical Culture
Leadership Commitment: Ensure that leadership is committed to ethical AI and sets a positive example for the entire organization.
Employee Training: Provide training on ethical AI practices and the importance of ethical considerations in AI development.
Engaging with Stakeholders
Stakeholder Involvement: Involve stakeholders, including users, in the AI development process to gather diverse perspectives and address ethical concerns.
Feedback Mechanisms: Establish mechanisms for stakeholders to provide feedback and report ethical concerns.
Adopting Ethical Standards
Industry Standards: Adopt and adhere to industry standards and best practices for ethical AI development.
Collaborative Efforts: Collaborate with other organizations, research institutions, and regulatory bodies to advance ethical AI standards and practices.
Conclusion
Building ethical AI is essential for ensuring that AI technologies are used responsibly and for the benefit of society. The challenges in creating ethical AI are significant, but they can be addressed through concerted efforts and collaboration. By focusing on bias and fairness, transparency and explainability, accountability, privacy and security, and ethical design, AI development company can lead the way in developing AI systems that are trustworthy, fair, and beneficial. As AI continues to evolve, ongoing commitment to ethical principles will be crucial in navigating the complex landscape of AI development and deployment.
2 notes
·
View notes
Text
The Impact of AI on Everyday Life: A New Normal
The impact of AI on everyday life has become a focal point for discussions among tech enthusiasts, policymakers, and the general public alike. This transformative force is reshaping the way we live, work, and interact with the world around us, making its influence felt across various domains of our daily existence. Revolutionizing Workplaces One of the most significant arenas where the impact…
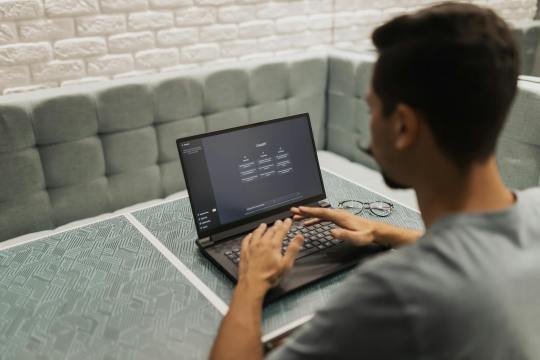
View On WordPress
#adaptive learning#AI accessibility#AI adaptation#AI advancements#AI algorithms#AI applications#AI automation#AI benefits#AI capability#AI challenges#AI collaboration#AI convenience#AI data analysis#AI debate#AI decision-making#AI design#AI diagnostics#AI discussion#AI education#AI efficiency#AI engineering#AI enhancement#AI environment#AI ethics#AI experience#AI future#AI governance#AI healthcare#AI impact#AI implications
1 note
·
View note
Text
lil photo dump for y’all
idk if you can make it out in the pic, but Cedric’s mermaid form has very sharp teeth
Julianna (yoga enthusiast) and Truman (feline criminal) hanging out on the deck ft. water collector
and finally, Julianna hanging out with Tessa. they have very slight flirty vibes, so I think we’ll push more in that direction once Serena (my chosen heir) is a teen
#ts4#sims 4#random legacy challenge#what are the ethics of romancing an AI you built?#unclear but all i know is that Tessa's making the moves#and I support this robot's wrongs#yamamoto rlc
2 notes
·
View notes
Text
Writers are magic word people and do not need to use AI 😊

So it looks like NaNoWriMo are happy to have AI as part of their community. Miss me with that bullshit. Generative artificial intelligence is an active threat to creativity and the livelihoods of hundreds of thousands of people in creative fields.
Please signal boost this so writers can make an informed choice about whether to continue to take part in such a community.
#apparently they are partnering with an AI tool so I guess that makes sense#idk man I think in general there are a lot of ethical and practical issues with generative AI#in the end NaNoWriMo is a writing challenge that is not actually policed or vetted in any way#if you use AI to “write” your project you are really only cheating yourself#since you are only winning by writing 50k words but you could already lie about that before AI was a concern#I think it sort of misses the point of the writing challenge
28K notes
·
View notes
Text
From OpenAI’s O3 to DeepSeek’s R1: How Simulated Thinking Is Making LLMs Think Deeper
New Post has been published on https://thedigitalinsider.com/from-openais-o3-to-deepseeks-r1-how-simulated-thinking-is-making-llms-think-deeper/
From OpenAI’s O3 to DeepSeek’s R1: How Simulated Thinking Is Making LLMs Think Deeper
Large language models (LLMs) have evolved significantly. What started as simple text generation and translation tools are now being used in research, decision-making, and complex problem-solving. A key factor in this shift is the growing ability of LLMs to think more systematically by breaking down problems, evaluating multiple possibilities, and refining their responses dynamically. Rather than merely predicting the next word in a sequence, these models can now perform structured reasoning, making them more effective at handling complex tasks. Leading models like OpenAI’s O3, Google’s Gemini, and DeepSeek’s R1 integrate these capabilities to enhance their ability to process and analyze information more effectively.
Understanding Simulated Thinking
Humans naturally analyze different options before making decisions. Whether planning a vacation or solving a problem, we often simulate different plans in our mind to evaluate multiple factors, weigh pros and cons, and adjust our choices accordingly. Researchers are integrating this ability to LLMs to enhance their reasoning capabilities. Here, simulated thinking essentially refers to LLMs’ ability to perform systematic reasoning before generating an answer. This is in contrast to simply retrieving a response from stored data. A helpful analogy is solving a math problem:
A basic AI might recognize a pattern and quickly generate an answer without verifying it.
An AI using simulated reasoning would work through the steps, check for mistakes, and confirm its logic before responding.
Chain-of-Thought: Teaching AI to Think in Steps
If LLMs have to execute simulated thinking like humans, they must be able to break down complex problems into smaller, sequential steps. This is where the Chain-of-Thought (CoT) technique plays a crucial role.
CoT is a prompting approach that guides LLMs to work through problems methodically. Instead of jumping to conclusions, this structured reasoning process enables LLMs to divide complex problems into simpler, manageable steps and solve them step-by-step.
For example, when solving a word problem in math:
A basic AI might attempt to match the problem to a previously seen example and provide an answer.
An AI using Chain-of-Thought reasoning would outline each step, logically working through calculations before arriving at a final solution.
This approach is efficient in areas requiring logical deduction, multi-step problem-solving, and contextual understanding. While earlier models required human-provided reasoning chains, advanced LLMs like OpenAI’s O3 and DeepSeek’s R1 can learn and apply CoT reasoning adaptively.
How Leading LLMs Implement Simulated Thinking
Different LLMs are employing simulated thinking in different ways. Below is an overview of how OpenAI’s O3, Google DeepMind’s models, and DeepSeek-R1 execute simulated thinking, along with their respective strengths and limitations.
OpenAI O3: Thinking Ahead Like a Chess Player
While exact details about OpenAI’s O3 model remain undisclosed, researchers believe it uses a technique similar to Monte Carlo Tree Search (MCTS), a strategy used in AI-driven games like AlphaGo. Like a chess player analyzing multiple moves before deciding, O3 explores different solutions, evaluates their quality, and selects the most promising one.
Unlike earlier models that rely on pattern recognition, O3 actively generates and refines reasoning paths using CoT techniques. During inference, it performs additional computational steps to construct multiple reasoning chains. These are then assessed by an evaluator model—likely a reward model trained to ensure logical coherence and correctness. The final response is selected based on a scoring mechanism to provide a well-reasoned output.
O3 follows a structured multi-step process. Initially, it is fine-tuned on a vast dataset of human reasoning chains, internalizing logical thinking patterns. At inference time, it generates multiple solutions for a given problem, ranks them based on correctness and coherence, and refines the best one if needed. While this method allows O3 to self-correct before responding and improve accuracy, the tradeoff is computational cost—exploring multiple possibilities requires significant processing power, making it slower and more resource-intensive. Nevertheless, O3 excels in dynamic analysis and problem-solving, positioning it among today’s most advanced AI models.
Google DeepMind: Refining Answers Like an Editor
DeepMind has developed a new approach called “mind evolution,” which treats reasoning as an iterative refinement process. Instead of analyzing multiple future scenarios, this model acts more like an editor refining various drafts of an essay. The model generates several possible answers, evaluates their quality, and refines the best one.
Inspired by genetic algorithms, this process ensures high-quality responses through iteration. It is particularly effective for structured tasks like logic puzzles and programming challenges, where clear criteria determine the best answer.
However, this method has limitations. Since it relies on an external scoring system to assess response quality, it may struggle with abstract reasoning with no clear right or wrong answer. Unlike O3, which dynamically reasons in real-time, DeepMind’s model focuses on refining existing answers, making it less flexible for open-ended questions.
DeepSeek-R1: Learning to Reason Like a Student
DeepSeek-R1 employs a reinforcement learning-based approach that allows it to develop reasoning capabilities over time rather than evaluating multiple responses in real time. Instead of relying on pre-generated reasoning data, DeepSeek-R1 learns by solving problems, receiving feedback, and improving iteratively—similar to how students refine their problem-solving skills through practice.
The model follows a structured reinforcement learning loop. It starts with a base model, such as DeepSeek-V3, and is prompted to solve mathematical problems step by step. Each answer is verified through direct code execution, bypassing the need for an additional model to validate correctness. If the solution is correct, the model is rewarded; if it is incorrect, it is penalized. This process is repeated extensively, allowing DeepSeek-R1 to refine its logical reasoning skills and prioritize more complex problems over time.
A key advantage of this approach is efficiency. Unlike O3, which performs extensive reasoning at inference time, DeepSeek-R1 embeds reasoning capabilities during training, making it faster and more cost-effective. It is highly scalable since it does not require a massive labeled dataset or an expensive verification model.
However, this reinforcement learning-based approach has tradeoffs. Because it relies on tasks with verifiable outcomes, it excels in mathematics and coding. Still, it may struggle with abstract reasoning in law, ethics, or creative problem-solving. While mathematical reasoning may transfer to other domains, its broader applicability remains uncertain.
Table: Comparison between OpenAI’s O3, DeepMind’s Mind Evolution and DeepSeek’s R1
The Future of AI Reasoning
Simulated reasoning is a significant step toward making AI more reliable and intelligent. As these models evolve, the focus will shift from simply generating text to developing robust problem-solving abilities that closely resemble human thinking. Future advancements will likely focus on making AI models capable of identifying and correcting errors, integrating them with external tools to verify responses, and recognizing uncertainty when faced with ambiguous information. However, a key challenge is balancing reasoning depth with computational efficiency. The ultimate goal is to develop AI systems that thoughtfully consider their responses, ensuring accuracy and reliability, much like a human expert carefully evaluating each decision before taking action.
#Advanced LLMs#ai#AI models#AI problem-solving#AI reasoning models#AI systems#Algorithms#Analysis#approach#Artificial Intelligence#Chain-of-Thought prompting#challenge#chess#code#coding#comparison#contextual understanding#data#DeepMind#DeepMind Mind Evolution#deepseek#deepseek-r1#DeepSeek-V3#details#domains#efficiency#Ethics#Evolution#factor#focus
0 notes
Text
At M.Kumarasamy College of Engineering (MKCE), we emphasize the significance of engineering ethics in shaping responsible engineers. Engineering ethics guide decision-making, foster professionalism, and ensure societal welfare. Our curriculum integrates these principles, teaching students to consider the long-term impacts of their work. Students are trained in truthfulness, transparency, and ethical communication, while also prioritizing public safety and environmental sustainability. We focus on risk management and encourage innovation in sustainable technologies. Our programs also address contemporary challenges like artificial intelligence and cybersecurity, preparing students to tackle these with ethical responsibility. MKCE nurtures future engineers who lead with integrity and contribute to society’s well-being.
To know more : https://mkce.ac.in/blog/engineering-ethics-and-navigating-the-challenges-of-modern-technologies/
#mkce college#top 10 colleges in tn#engineering college in karur#best engineering college in karur#private college#libary#mkce#best engineering college#mkce.ac.in#engineering college#• Engineering Ethics#Engineering Decision Making#AI and Ethics#Cybersecurity Ethics#Public Safety in Engineering#• Environmental Sustainability in Engineering#Professional Responsibility#Risk Management in Engineering#Artificial Intelligence Challenges#Engineering Leadership#• Data Privacy and Security#Ethical Engineering Practices#Sustainable Engineering Solutions#• Technological Innovation and Ethics#Technological Innovation and Ethics#MKCE Engineering Curriculum#• Social Responsibility in Engineering#• Engineering Ethics in AI#Workplace Ethics in Engineering#Collaboration in Engineering Projects
0 notes
Text
AI and Health: New Technologies Paving the Way for Better Treatment
Artificial intelligence (AI) is expanding rapidly in the health sector, and it is revolutionizing our medical system. With the help of AI, new technologies are being developed that are not only helpful in accurately diagnosing diseases but are also playing an important role in personalized treatment and management.
Quick and accurate diagnosis of diseases AI-based tools can now analyze medical imaging data such as X-rays, CT scans, and MRIs quickly and accurately. This helps doctors to quickly detect complex conditions such as cancer, heart diseases, and neurological problems.
Personalized medicine AI can help create personalized treatment plans for every individual by analyzing genomics and biometrics. This technology ensures that the patient gets the right medicine and the right dose at the right time.
Improved health management AI-based health apps and wearables such as smart watches are now helping people monitor their health condition. These devices regularly track health indicators such as heart rate, blood pressure and sleep quality.
Accelerating medical research The role of AI has become extremely important in the development of new drugs and vaccines. Using AI, scientists can analyze complex data sets and make new medical discoveries faster.
Accessible and affordable healthcare AI technology is helping in delivering affordable and effective healthcare, even in rural and remote areas. Telemedicine and virtual health assistants are bridging the gap between patients and doctors.
Conclusion Artificial intelligence is playing an important role in making healthcare more effective, accurate, and accessible. However, there are challenges such as data security and ethics in the use of AI technology which need to be dealt with. In the coming years, with more advanced and innovative uses of AI, the healthcare landscape may change completely.
#AI and Health: New Technologies Paving the Way for Better Treatment#Artificial intelligence (AI) is expanding rapidly in the health sector#and it is revolutionizing our medical system. With the help of AI#new technologies are being developed that are not only helpful in accurately diagnosing diseases but are also playing an important role in#Quick and accurate diagnosis of diseases#AI-based tools can now analyze medical imaging data such as X-rays#CT scans#and MRIs quickly and accurately. This helps doctors to quickly detect complex conditions such as cancer#heart diseases#and neurological problems.Personalized medicine#AI can help create personalized treatment plans for every individual by analyzing genomics and biometrics. This technology ensures that the#AI-based health apps and wearables such as smart watches are now helping people monitor their health condition. These devices regularly tra#blood pressure and sleep quality.Accelerating medical research#The role of AI has become extremely important in the development of new drugs and vaccines. Using AI#scientists can analyze complex data sets and make new medical discoveries faster.Accessible and affordable healthcare#AI technology is helping in delivering affordable and effective healthcare#even in rural and remote areas. Telemedicine and virtual health assistants are bridging the gap between patients and doctors.#Conclusion#Artificial intelligence is playing an important role in making healthcare more effective#accurate#and accessible. However#there are challenges such as data security and ethics in the use of AI technology which need to be dealt with. In the coming years#with more advanced and innovative uses of AI#the healthcare landscape may change completely.
0 notes
Text

From raw materials to retail, CSR standards play a crucial role in building a sustainable and ethical supply chain. 🌍🚛 Ensure accountability, transparency, and social responsibility at every stage. Let’s create a better future together! 🌟
#AI in medical diagnostics#AI-powered healthcare solutions#Artificial Intelligence in radiology#Precision medicine with AI#AI in predictive analytics#Ethical challenges in AI diagnostics
0 notes
Text
When AI and Emotional Intelligence Collide: New Challenges for Leaders
Artificial Intelligence (AI) is changing the workplace fast. Now, AI and emotional intelligence (EI) are meeting in a new way1. This meeting brings both chances and hurdles for leaders. They must find a way to mix AI’s cold logic with EI’s warm touch to create caring and welcoming workspaces. This challenge is key for leaders who want to help their teams grow and succeed in the AI era. It’s about…
#Artificial Intelligence Ethics#Challenges of AI integration#Emotional intelligence development#Emotional intelligence in leadership#Emotional intelligence training#Human-machine collaboration#Leadership in the digital age#Technological advancements in leadership
0 notes
Text
Automatisasi Bisnis dengan Kekuatan AI
Automatisasi bisnis telah menjadi topik utama dalam dunia usaha modern. Dengan kemajuan teknologi kecerdasan buatan (AI), perusahaan kini memiliki peluang untuk mengoptimalkan proses mereka, meningkatkan efisiensi, dan mengurangi biaya operasional. AI tidak hanya menggantikan tugas-tugas manual yang berulang tetapi juga membawa kemampuan analisis data yang canggih, prediksi yang akurat, dan…
#AI automation#AI benefits#AI challenges#AI in banking#AI in business#AI in logistics#AI in retail#AI training#AI trends 2024#AI-powered tools#artificial intelligence#business automation#business innovation#cost reduction#customer experience#ethical AI#future of AI#operational efficiency#predictive analytics#scalable solutions#smart inventory management#supply chain management#workforce automation
0 notes
Text
Personality Assessment Using AI – Asrar Qureshi’s Blog Post 1046
#AI#AI-Based Tools#Asrar Qureshi#Assessment#Benefits#Blogpost1046#Challenges#Ethical Issues#Hiring#Personality#Pharma Veterans#Recruitment#Team work#Training
0 notes
Text
Navigating Regulatory Complexity: Why Traditional Compliance Methods Fall Short in High-Volatility Sectors
In industries like finance, healthcare, and technology, regulatory compliance is more than just a legal requirement—it’s a cornerstone of trust and sustainability. However, the volatile nature of these sectors, coupled with rapidly evolving regulations, has exposed the limitations of traditional compliance methods. Manual audits, spreadsheet-driven reporting, and fragmented monitoring systems are no longer sufficient to manage the intricacies of today’s regulatory landscape.
Enter AI compliance tools, which are transforming how organizations navigate these complexities. By offering compliance efficiency with AI, these tools enable businesses to stay ahead of regulatory changes, reduce costs, and enhance accuracy. In this article, we explore why traditional compliance methods are falling short and how AI compliance solutions are paving the way forward.
The Challenges of Traditional Compliance Methods
1. Slow Adaptation to Regulatory Changes
Regulations in high-volatility sectors evolve rapidly. For instance, financial institutions face frequent updates to anti-money laundering (AML) rules, while tech companies must adhere to shifting data privacy laws like GDPR. Traditional compliance methods, reliant on manual reviews, struggle to keep up with these changes.
Example: A financial firm using manual systems may miss critical updates, leading to non-compliance fines.
2. High Costs and Inefficiencies
Manual compliance processes demand significant resources, including large teams and extensive time investments. These inefficiencies drive up operational costs without guaranteeing error-free outcomes.
Fact: According to a PwC report, financial institutions spend up to $270 billion annually on compliance, with much of this cost stemming from outdated processes.
3. Fragmented Monitoring and Reporting
Traditional methods often involve disparate tools for monitoring, reporting, and auditing. This siloed approach leads to inefficiencies, inconsistent data, and delayed responses to compliance risks.
4. Limited Scalability
As organizations grow, traditional compliance methods fail to scale efficiently. Handling increasing data volumes and regulatory demands becomes an overwhelming challenge.
Why AI is the Solution
Artificial intelligence has emerged as a game-changer, offering a smarter, faster, and more cost-effective way to manage compliance. Here’s how AI for compliance is addressing the shortcomings of traditional methods:
1. Automation in Compliance
AI automates repetitive tasks such as data collection, analysis, and reporting. This reduces the reliance on manual processes and ensures faster compliance workflows.
Example: A healthcare organization automated its patient data audits using AI, cutting processing times by 50%.
2. Real-Time Monitoring with AI
AI compliance monitoring tools provide real-time oversight of transactions, communications, and processes, flagging potential risks instantly. This proactive approach minimizes the likelihood of violations.
Case Study: A multinational bank reduced its fraud detection time from days to minutes by implementing AI-driven monitoring systems.
3. Regulatory Updates with AI
Keeping up with regulatory changes is critical in volatile sectors. AI-powered tools analyze and interpret new regulations, providing actionable insights to ensure compliance.
Example: A tech firm used AI tools to adapt its operations to GDPR updates across multiple regions, ensuring consistent compliance.
4. Data Security and Accuracy
AI enhances compliance and data security by identifying vulnerabilities and ensuring sensitive data is handled according to regulations. It also reduces human error, a common cause of non-compliance.
Best Practice: Regularly audit AI systems to maintain transparency and ethical standards in compliance management.
Benefits of AI in Compliance
Adopting AI compliance strategies offers tangible benefits:
Cost Reduction: Compliance cost reduction with AI stems from automation and improved efficiency.
Scalability: AI systems can handle growing data volumes and regulatory complexities without requiring additional resources.
Improved Accuracy: By minimizing human involvement in repetitive tasks, AI reduces errors and ensures consistent compliance.
Proactive Risk Management: Predictive analytics identify risks before they escalate, allowing for timely mitigation.
Real-World Examples of AI Compliance Tools
1. AI-Driven Compliance in Finance
A global financial institution faced challenges in managing AML protocols. By implementing AI tools, the firm achieved:
A 70% reduction in false positives.
Faster risk assessments, saving millions in operational costs.
2. Healthcare: Streamlining Patient Data Compliance
A hospital network automated HIPAA compliance audits using AI, reducing manual effort by 60% and cutting penalties for data breaches by 30%.
3. Tech Industry: GDPR Compliance
A tech company handling vast amounts of user data used AI-powered tools for real-time monitoring and automated reporting, ensuring GDPR compliance across all its platforms.
Challenges in Implementing AI for Compliance
While the advantages are clear, adopting AI in compliance comes with challenges:
High Initial Costs: AI tools require significant upfront investment.
Solution: Start small with targeted automation projects before scaling up.
Skill Gaps: Organizations may lack the expertise to manage AI systems.
Solution: Invest in training programs to upskill employees.
AI Ethics in Compliance: Ensuring transparency and accountability in AI-driven processes is crucial.
Solution: Develop frameworks for ethical AI use and conduct regular audits.
Future of AI in Compliance
The role of AI in compliance will continue to grow as technologies advance. Key trends to watch include:
Hyper-Automation: Combining AI with robotics to fully automate compliance workflows.
Predictive Analytics: Leveraging data to anticipate and mitigate compliance risks.
Collaborative Platforms: AI-powered ecosystems that enable cross-industry collaboration on compliance.
Best Practices for Implementing AI Compliance Solutions
Define Objectives: Align AI tools with specific compliance goals.
Prioritize Data Security: Ensure robust measures to protect sensitive information.
Train Teams: Equip employees with the skills needed to work effectively with AI systems.
Monitor and Optimize: Regularly evaluate AI tools to ensure they remain effective and compliant with regulations.
Conclusion
Traditional compliance methods are ill-equipped to handle the complexities of high-volatility sectors. By leveraging AI compliance solutions, organizations can achieve greater efficiency, cost savings, and accuracy in managing regulatory requirements. Real-world success stories highlight the transformative potential of AI, from compliance automation benefits to proactive risk management.
As the future of AI in compliance unfolds, businesses must embrace innovation and adopt best practices to stay ahead in an increasingly complex regulatory environment.
#AI compliance#AI for compliance#AI compliance tools#AI compliance solutions#AI compliance challenges#benefits of AI in compliance#regulatory compliance with AI#compliance efficiency with AI#AI ethics in compliance
0 notes
Text
AI and Ethical Challenges in Academic Research
When Artificial Intelligence (AI) becomes more and more integrated into research in academia and practice, it opens up both new opportunities and major ethical issues. Researchers can now utilize AI to study vast amounts of data for patterns, identify patterns, and even automate complicated processes. However, the rapid growth of AI within academia poses serious ethical questions about privacy, bias, transparency and accountability. Photon Insights, a leader in AI solutions for research, is dedicated to addressing these issues by ensuring ethical considerations are on the leading edge of AI applications in the academic world.
The Promise of AI in Academic Research
AI has many advantages that improve the effectiveness and efficiency of research in academia:
1. Accelerated Data Analysis
AI can process huge amounts of data in a short time, allowing researchers to detect patterns and patterns which would require humans much longer to discover.
2. Enhanced Collaboration
AI tools allow collaboration between researchers from different institutions and disciplines, encouraging the exchange of ideas and data.
3. Automating Routine Tasks Through the automation of repetitive tasks AI lets researchers focus on more intricate and innovative areas of work. This leads to more innovation.
4. Predictive analytics: AI algorithms can forecast outcomes by analyzing the past, and provide useful insights for designing experiments and testing hypotheses.
5. “Interdisciplinary Research: AI can bridge gaps between disciplines, allowing researchers to draw from a variety of data sets and methods.
Although these benefits are significant but they also raise ethical issues that should not be ignored.
Ethical Challenges in AI-Driven Research
1. Data Privacy
One of the biggest ethical concerns with AI-driven research is the privacy of data. Researchers frequently work with sensitive data, which includes personal information of participants. In the use of AI tools raises concerns about the methods used to collect this data, stored, and then analyzed.
Consent and Transparency: It is essential to obtain an informed consent from the participants on using their personal data. This requires being transparent regarding how data is utilized and making sure that participants are aware of the consequences on AI analysis.
Data Security: Researchers need to implement effective security measures to guard sensitive data from breaches and unauthorized access.
2. Algorithmic Bias
AI models are only as effective as the data they’re training on. If data sets contain biases, whether based on gender, race socioeconomic status, gender, or other factors, the resultant AI models may perpetuate these biases, which can lead to biased results and negative consequences.
Fairness in Research Researchers should critically evaluate the data they collect to ensure that they are accurate and impartial. This means actively looking for different data sources and checking AI outputs for any potential biases.
Impact on Findings
Biased algorithms could alter research findings, which can affect the reliability of the conclusions drawn, and creating discriminatory practices in areas such as education, healthcare and social sciences.
3. Transparency and Accountability
The complex nature of AI algorithms can result in the “black box” effect, in which researchers are unable to comprehend the process of making decisions. The lack of transparency creates ethical questions concerning accountability.
Explainability Researchers must strive for explicable AI models that enable them to comprehend and explain the process of making decisions. This is crucial when AI is used to make critical decision-making in areas such as public health or the formulation of policies.
Responsibility for AI Results Establishing clearly defined lines of accountability is essential. Researchers must be accountable for the consequences for using AI tools, making sure they are employed ethically and with integrity.
4. Intellectual Property and Authorship
AI tools can create original content, which raises questions regarding the rights to intellectual property and authorship. Who owns the outcomes produced from AI systems? AI system? Do AI contributions be recognized in the publication of papers?
Authorship Guidelines Academic institutions should create clear guidelines on how to use AI when conducting research or authorship and attribution. This ensures that all contributions — whether human or machine — are appropriately recognized.
Ownership of Data institutions must identify who is the person responsible for the data utilized to run AI systems, especially when they are involved in collaborative research with different industries or institutions.
Photon Insights: Pioneering Ethical AI Solutions
Photon Insights is committed to exploring the ethical implications of AI in research in academia. The platform provides tools that focus on ethical concerns while maximizing the value of AI.
1. Ethical Data Practices
Photon Insights emphasizes ethical data management. The platform assists researchers to implement the best practices in data collection consent, security, and privacy. The platform includes tools to:
Data Anonymization: ensuring that sensitive data remains secure while providing an analysis that is valuable.
Informed Consent Management: Facilitating transparent information about the usage of data to the participants.
2. Bias Mitigation Tools
To combat bias in algorithms, Photon Insights incorporates features that allow researchers to:
Audit Datasets Identify and correct errors in the data prior to making use of it for AI training.
Monitor AI Outputs: Continually examine AI-generated outputs to ensure accuracy and fairness and alerts about possible biases.
3. Transparency and Explainability
Photon Insights is a leader in explaining AI by offering tools that improve transparency:
Model Interpretability Researchers can see and comprehend the decision-making process in AI models, which allows for clearer dissemination of the results.
Comprehensive Documentation — The platform promotes thorough documentation of AI methods, which ensures transparency in research methods.
4. Collaboration and Support
Photon Insights fosters collaboration among researchers as well as institutions and industry participants, encouraging the ethics of the use and application of AI by:
Community Engagement Engaging in discussions on ethics-based AI methods within research communities.
Educational Resources Training and information on ethical issues when conducting AI research, and ensuring that researchers are aware.
The Future of AI in Academic Research
As AI continues to develop and become more ethical, the ethical issues that it poses need to be addressed regularly. The academic community needs to take an active approach to tackle these issues, and ensure that AI is utilized ethically and responsibly.
1. “Regulatory Frameworks” Creating guidelines and regulations for AI application in the field of research is crucial in protecting privacy of data and guaranteeing accountability.
2. Interdisciplinary Collaboration: Collaboration between ethicists, data scientists and researchers will create an holistic way of approaching ethical AI practices, making sure that a variety of viewpoints are considered.
3. Continuous Education: Constant education and training in ethical AI techniques will allow researchers to better understand the maze of AI in their research.
Conclusion
AI has the potential to change the way academic research is conducted by providing tools to increase efficiency and boost innovations. However, the ethical concerns that come with AI should be addressed to ensure that it is used in a responsible manner. Photon Insights is leading the campaign to promote ethical AI practices and provides researchers with the tools and assistance they require to navigate through this tangled landscape.
In focusing on ethical considerations in academic research, researchers can benefit from the power of AI while maintaining the principles of fairness, integrity and accountability. It is likely that the future for AI in research at the university is promising and, with the appropriate guidelines set up, it will be a powerful force to bring about positive change in the world.
0 notes
Text
AI and Ethical Challenges in Academic Research
When Artificial Intelligence (AI) becomes more and more integrated into research in academic and practice, it opens up both new opportunities and major ethical issues. Researchers can now utilize AI to study vast amounts of data for patterns, identify patterns, and even automate complicated processes. However, the rapid growth of AI within academia poses serious ethical questions about privacy, bias, transparency and accountability. Photon Insights, a leader in AI solutions for research, is dedicated to addressing these issues by ensuring ethical considerations are on the leading edge of AI applications in the academic world.
The Promise of AI in Academic Research
AI has many advantages that improve the effectiveness and efficiency of research in academia:
1. Accelerated Data Analysis
AI can process huge amounts of data in a short time, allowing researchers to detect patterns and patterns which would require humans much longer to discover.
2. Enhanced Collaboration
AI tools allow collaboration between researchers from different institutions and disciplines, encouraging the exchange of ideas and data.
3. Automating Routine Tasks Through the automation of repetitive tasks AI lets researchers focus on more intricate and innovative areas of work. This leads to more innovation.
4. Predictive analytics: AI algorithms can forecast outcomes by analyzing the past, and provide useful insights for designing experiments and testing hypotheses.
5. “Interdisciplinary Research: AI can bridge gaps between disciplines, allowing researchers to draw from a variety of data sets and methods.
Although these benefits are significant but they also raise ethical issues that should not be ignored.
Ethical Challenges in AI-Driven Research
1. Data Privacy
One of the biggest ethical concerns with AI-driven research is the privacy of data. Researchers frequently work with sensitive data, which includes personal information of participants. In the use of AI tools raises concerns about the methods used to collect this data, stored, and then analyzed.
Consent and Transparency: It is essential to obtain an informed consent from the participants on using their personal data. This requires being transparent regarding how data is utilized and making sure that participants are aware of the consequences on AI analysis.
Data Security: Researchers need to implement effective security measures to guard sensitive data from breaches and unauthorized access.
2. Algorithmic Bias
AI models are only as effective as the data they’re training on. If data sets contain biases, whether based on gender, race socioeconomic status, gender, or other factors, the resultant AI models may perpetuate these biases, which can lead to biased results and negative consequences.
Fairness in Research Researchers should critically evaluate the data they collect to ensure that they are accurate and impartial. This means actively looking for different data sources and checking AI outputs for any potential biases.
Impact on Findings
Biased algorithms could alter research findings, which can affect the reliability of the conclusions drawn, and creating discriminatory practices in areas such as education, healthcare and social sciences.
3. Transparency and Accountability
The complex nature of AI algorithms can result in the “black box” effect, in which researchers are unable to comprehend the process of making decisions. The lack of transparency creates ethical questions concerning accountability.
Explainability Researchers must strive for explicable AI models that enable them to comprehend and explain the process of making decisions. This is crucial when AI is used to make critical decision-making in areas such as public health or the formulation of policies.
Responsibility for AI Results Establishing clearly defined lines of accountability is essential. Researchers must be accountable for the consequences for using AI tools, making sure they are employed ethically and with integrity.
4. Intellectual Property and Authorship
AI tools can create original content, which raises questions regarding the rights to intellectual property and authorship. Who owns the outcomes produced from AI systems? AI system? Do AI contributions be recognized in the publication of papers?
Authorship Guidelines Academic institutions should create clear guidelines on how to use AI when conducting research or authorship and attribution. This ensures that all contributions — whether human or machine — are appropriately recognized.
Ownership of Data institutions must identify who is the person responsible for the data utilized to run AI systems, especially when they are involved in collaborative research with different industries or institutions.
Photon Insights: Pioneering Ethical AI Solutions
Photon Insights is committed to exploring the ethical implications of AI in research in academia. The platform provides tools that focus on ethical concerns while maximizing the value of AI.
1. Ethical Data Practices
Photon Insights emphasizes ethical data management. The platform assists researchers to implement the best practices in data collection consent, security, and privacy. The platform includes tools to:
Data Anonymization: ensuring that sensitive data remains secure while providing an analysis that is valuable.
Informed Consent Management: Facilitating transparent information about the usage of data to the participants.
2. Bias Mitigation Tools
To combat bias in algorithms, Photon Insights incorporates features that allow researchers to:
Audit Datasets Identify and correct errors in the data prior to making use of it for AI training.
Monitor AI Outputs: Continually examine AI-generated outputs to ensure accuracy and fairness and alerts about possible biases.
3. Transparency and Explainability
Photon Insights is a leader in explaining AI by offering tools that improve transparency:
Model Interpretability Researchers can see and comprehend the decision-making process in AI models, which allows for clearer dissemination of the results.
Comprehensive Documentation — The platform promotes thorough documentation of AI methods, which ensures transparency in research methods.
4. Collaboration and Support
Photon Insights fosters collaboration among researchers as well as institutions and industry participants, encouraging the ethics of the use and application of AI by:
Community Engagement Engaging in discussions on ethics-based AI methods within research communities.
Educational Resources Training and information on ethical issues when conducting AI research, and ensuring that researchers are aware.
The Future of AI in Academic Research
As AI continues to develop and become more ethical, the ethical issues that it poses need to be addressed regularly. The academic community needs to take an active approach to tackle these issues, and ensure that AI is utilized ethically and responsibly.
1. “Regulatory Frameworks” Creating guidelines and regulations for AI application in the field of research is crucial in protecting privacy of data and guaranteeing accountability.
2. Interdisciplinary Collaboration: Collaboration between ethicists, data scientists and researchers will create an holistic way of approaching ethical AI practices, making sure that a variety of viewpoints are considered.
3. Continuous Education: Constant education and training in ethical AI techniques will allow researchers to better understand the maze of AI in their research.
Conclusion
AI has the potential to change the way academic research is conducted by providing tools to increase efficiency and boost innovations. However, the ethical concerns that come with AI should be addressed to ensure that it is used in a responsible manner. Photon Insights is leading the campaign to promote ethical AI practices and provides researchers with the tools and assistance they require to navigate through this tangled landscape.
In focusing on ethical considerations in academic research, researchers can benefit from the power of AI while maintaining the principles of fairness, integrity and accountability. It is likely that the future for AI in research at the university is promising and, with the appropriate guidelines set up, it will be a powerful force to bring about positive change in the world.
0 notes