#AI reasoning models
Explore tagged Tumblr posts
Text



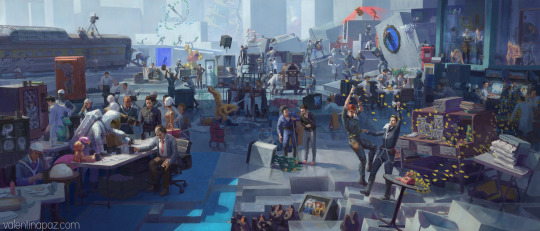
PARTY IN THE OLDEST HOUSE GUUUYYYYYS
There it is, eight months in the making.
Given the size of this file and the amount of details, I've included more close-ups and a download link to a 2k file over here:
big thanks to @wankernumberniiiiiiiiine, she's the reason this painting exists 🥰
#control 2019#control game#control remedy#artists on tumblr#jesse faden#emily pope#frederick langston#simon arish#dylan faden#ahti the janitor#ahti#my art#control game fanart#that's every AI and OoP with an ingame model#so no cowboy boots or burroughs tractor#also no alan page bc blegh this is a lot already lmao#also the only reason there's no hiss is because I did not want to draw and paint all those HRAs alright
1K notes
·
View notes
Text

from the programming that brought you glue pizza
how do we get this kind of bellend to shut up lol theyre so delusional
#there are a LOT of reasons we shouldnt be fucking about with AI at the moment#but PERSONALLY id like to ruin these idiots' days over it#these systems cannot use reasoning like people can get over yourself#i dont understand the obsession with AI at all#im all for using programming to do things people find difficult and some of the REAL developments into it are cool#but the vast majority of 'AI' is just a good model for doing shit we already did#its very disengenious to call everything 'AI' like that means something#cant wait for this shit bubble to burst#rory's ramblings
7 notes
·
View notes
Text
"Oh but I'm not good at drawing so I-"
SHUT UP
You know what I did when I wanted to make art but it didn't quite compute in my brain????
I LEARNED AN ART STYLE THAT WOULD
You don't need a fucking computer to do it for you.
#LITERALLY THE ENTIRE REASON I 3D MODEL#My brain doesn't like 2D art that well#I can do very minor stuff that like any other person could do#however 3D is my fucking jam#so I started playing 4D chess with Blender#3d modeling#blender#art#anti ai#fuck ai#make shit#be happy
7 notes
·
View notes
Text
anti 'ai' people make 1 (one) well-informed, good-faith argument challenge(impossible)
#txt#obviously the vast majority of anti-'ai' people on here are just like.#'my internet sphere told me machine-generated text/images are bad'#and have not considered any cases where 'ai' is demonstrably materially useful#(e.g. tumor detection. drug discovery. early detection of MANY diseases. modeling the effects of epi/pandemic responses. modeling all sorts#of public health policy‚ actually. discovering new mechanisms of disease. that's just off the top of my head)#but now people are straight up saying that computers are NEVER better than humans at any tasks. and we should all just ~use our BRAINS!!!~#like. i have no words.#i mean i fucking guess i shouldn't expect these people to base their takes on actual facts or reason.#still pisses me the fuck off to know that there are people out there who are so dogmatic about this#editing to put this in my#‘ai’#tag
3 notes
·
View notes
Text
I was working on something for Clextober and here are some Alycia AI generated images that I didn't use.



#ai generated#i am not artistic#she is easier to have the ai make images for than eliza#for some reason i can't get it to make decent Clarke pics#fencing lexa#cowgirl/model lexa#halloween party lexa
4 notes
·
View notes
Text
ed zitron, a tech beat reporter, wrote an article about a recent paper that came out from goldman-sachs calling AI, in nicer terms, a grift. it is a really interesting article; hearing criticism from people who are not ignorant of the tech and have no reason to mince words is refreshing. it also brings up points and asks the right questions:
if AI is going to be a trillion dollar investment, what trillion dollar problem is it solving?
what does it mean when people say that AI will "get better"? what does that look like and how would it even be achieved? the article makes a point to debunk talking points about how all tech is misunderstood at first by pointing out that the tech it gets compared to the most, the internet and smartphones, were both created over the course of decades with roadmaps and clear goals. AI does not have this.
the american power grid straight up cannot handle the load required to run AI because it has not been meaningfully developed in decades. how are they going to overcome this hurdle (they aren't)?
people who are losing their jobs to this tech aren't being "replaced". they're just getting a taste of how little their managers care about their craft and how little they think of their consumer base. ai is not capable of replacing humans and there's no indication they ever will because...
all of these models use the same training data so now they're all giving the same wrong answers in the same voice. without massive and i mean EXPONENTIALLY MASSIVE troves of data to work with, they are pretty much as a standstill for any innovation they're imagining in their heads
76K notes
·
View notes
Text
Mistral AI Masterclass: The Reasoning Model That Accelerates Complex Analysis
MistralAI’s Magistral is an innovative reasoning model, open-source in the Small version, and enterprise in the Medium version. It excels in multilingual performance, transparency, speed, multi-step capability, with AIME2024 scores between 70.7% and 73.6%, 90% with voting. Key points: Dual version: Small (24B parameters, open-source Apache2.0), Medium (enterprise). AIME2024 scores: Medium 73.6% (90% with voting), Small 70.7% (83.3%). Transparent “chain-of-thought” reasoning in multiple languages... read more: https://www.turtlesai.com/en/pages-2897/mistral-ai-masterclass-the-reasoning-model-that-accelerates-complex
0 notes
Text
Info: Right now, A new Deepseek AI trend & wave is running in the artificial intelligence industry. It’s called Deepseek. Pretty much the perfect alternative to ChatGPT, and experts say it has become a game-changer for the industry and continues as the hottest trend of 2025. Know The Whole ABCs About Chinese Artificial Intelligence. Visit Us On Trendytell.com Or From The Above Article Source.
0 notes
Text
#Tags:AI Arms Race#AI Security#Artificial Intelligence#Cybersecurity Threats#DeepSeek#facts#Geopolitical Tensions in AI#life#Malicious Attacks#OpenAI Rival#Podcast#R1 Reasoning Model#serious#straight forward#truth#U.S.-China Tech Competition#upfront#website
0 notes
Text
yeah i also did NOT like the way that one caveat was framed at the end where they said basically "hey good news! we also found that this only applies up to a point bc if you were one of the *smart* people using chatgpt we found it actually improved outcomes. so not to worry, its only bad for you if you were stupid to begin with! 🤗🤗" because what on EARTH can you possibly mean by "high cognitive baseline" ?????? what are you saying to me right now. how can you possibly be defining that. i'm gonna need to hear in detail what metrics you used to determine "high cognitive baseline" RIGHT now because something stinks BIG TIME
I think that relying too much in LLMs can indeed harm your capacity for learning, in the same way that poor systems of learning (rote memorization, just copying whatever without thinking) can. Learning is a thing you need... to learn. Even LLMs can be a useful tool for learning as long as you have a good learning strategy.
That paper that says that AIs cause COGNITIVE ATROPHY is still unreviewd and smells so much of bullshit that I'm tearing up from here. Do not share it uncritically.
#angie.txt#thats the exact reason i still have that article open in the next tab.#literally because in a bit when i have a minute i intend to go dig into the methods section and see if i can find out.#i'm sure they spent a lot more time describing their model though so im prepared to have to sift through a LOT when i get around to it#im sus tho. extremely#i mean im with you gen ai is bad objectively and studies that demonstrate exactly how and why are critical but.#lets not do it this way please. this is why humanities need to still be required in stem
3K notes
·
View notes
Text
Just saw an article about how the phone line I have been using for 10 years now is about to get AI features. You seemingly cannot disable them. My phone updates automatically whether I tell it to or not. The most recent update has already fucked up autocorrect. I can type the sentence, "Yeah, I'm about to leave work," to my sister and it'll correct it to, "Yeah, if I am when I am leaving working." It just adds words and corrects me on shit that isn't wrong. Likes to put things in a tense other than the one I've been using previously. Or it'll straight up correct things to something that doesn't make sense (ex, I type "tge" by mistake and it corrects it to "yet"). I do not have the money for a new phone right now. I will lose my mind if AI becomes the way this thing functions.
#the phone also literally has been growing mold since i bought it#no matter what i do there's mold inside#i brought it to my carrier after purchase and they're just like yep. got a case of a moldy phone.#said it wouldn't change how it functions (???)#at any given time there's a mold colony growing out of the phone and into the case#so maybe that was my first sign that i need not use this brand any more#but it was $200 so like.... can i really complain about the mold?#no idea what kind of mold it is either. it's white with a green tint. looks kinda like bread mold.#but on the ai subject. i feel like that might just be the new standard and I'll be unable to avoid it.#if that's the case i might be going back to flip phones#if i have some learning model suggesting shit to me based on what I've like texted to someone or something I'm gonna do 9/11 2#i want a battery that doesn't drain itself in 5 hours flat in temps above 90 or under 35#i want more reliable service in the major city i live in (which for whatever reason isn't covered properly by my carrier)#i want the phone to be serviceable so i can use it longer than 2-3 years if i wish to#i do not want an ai suggesting i buy shroggles (shrek goggles) because of a text i sent
1 note
·
View note
Text
OpenAI Launches its First Reasoning Model "GPT-4 Turbo (Grok)" for ChatGPT Enterprise
OpenAI Launches its First Reasoning Model “GPT-4 Turbo (Grok)” for ChatGPT EnterpriseEnglish:OpenAI has made a significant leap in the world of artificial intelligence by launching its first reasoning-focused model, GPT-4 Turbo, also known as “Grok.” This model is an advancement tailored specifically for ChatGPT Enterprise, designed to enhance AI’s ability to understand, analyze, and respond with…
#AI development#Artificial intelligence#Automation#business environments#ChatGPT Enterprise#complex queries#critical thinking#decision-making#enhanced capabilities#enterprise use#faster responses#future implications#GPT-4 Turbo#Grok#in-depth analysis#logical reasoning#OpenAI#problem-solving#reasoning model#specialized AI systems.#structured solutions
0 notes
Text
21st Century Project Planning: Blueprint for Unparalleled Success
Discover the secrets to mastering project planning and achieving unparalleled success! Dive into our latest article for expert insights and practical tips. Don't miss out—subscribe now to stay updated on the best strategies for professional development!
Mastering Project Planning: Crafting the Blueprint for Unparalleled Success in the 21st Century Imagine venturing on a journey without a map, a compass, or even a clear destination in mind. The chance of reaching your goal would be slim to none. This scenario mirrors the challenges faced by project managers who dive into execution without a solid plan in place. The planning phase of project…
#AIanalyticsprojects#budgetingprojects#financialplanning#Ganttcharts#modernprojectmanagement#predictivemodelingsoftware#ProfessionalDevelopment#projectmanagement#projectplanningstage#projectsuccess#roledefinition#SMARTgoals#teamcollaboration#timelinecreation#agile project management#AI-driven analytics in projects#budgeting in projects#Empowered Journey#financial planning#Gantt charts#Hafsa Reasoner#modern project management#predictive modeling software#professional development#project management#project planning stage#project success#role definition#SMART goals#team collaboration
0 notes
Text
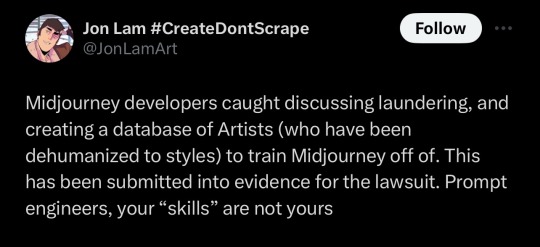

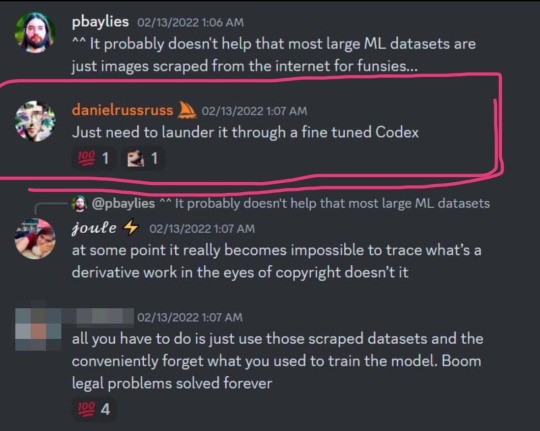
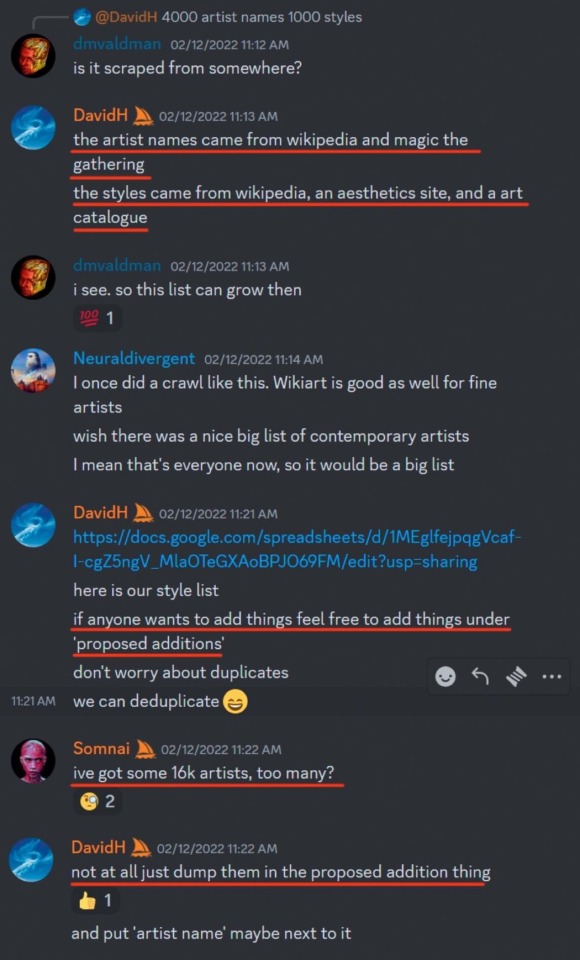
so a huge list of artists that was used to train midjourney’s model got leaked and i’m on it
literally there is no reason to support AI generators, they can’t ethically exist. my art has been used to train every single major one without consent lmfao 🤪
link to the archive
37K notes
·
View notes