#babyAGI
Explore tagged Tumblr posts
Link
Are you ready to use multiple AI agents with one click?
8 notes
·
View notes
Text
They Promised Us Agents, but All We Got Were Static Chains
New Post has been published on https://thedigitalinsider.com/they-promised-us-agents-but-all-we-got-were-static-chains/
They Promised Us Agents, but All We Got Were Static Chains
In the spring of 2023, the world got excited about the emergence of LLM-based AI agents. Powerful demos like AutoGPT and BabyAGI demonstrated the potential of LLMs running in a loop, choosing the next action, observing its results, and choosing the next action, one step at a time (also known as the ReACT framework). This new method was expected to power agents that autonomously and generically perform multi-step tasks. Give it an objective and a set of tools and it will take care of the rest. By the end of 2024, the landscape will be full of AI agents and AI agent-building frameworks. But how do they measure against the promise?
It is safe to say that the agents powered by the naive ReACT framework suffer from severe limitations. Give them a task that requires more than a few steps, using more than a few tools and they will miserably fail. Beyond their obvious latency issues, they will lose track, fail to follow instructions, stop too early or stop too late, and produce wildly different results on each attempt. And it is no wonder. The ReACT framework takes the limitations of unpredictable LLMs and compounds them by the number of steps. However, agent builders looking to solve real-world use cases, especially in the enterprise, cannot do with that level of performance. They need reliable, predictable, and explainable results for complex multi-step workflows. And they need AI systems that mitigate, rather than exacerbate, the unpredictable nature of LLMs.
So how are agents built in the enterprise today? For use cases that require more than a few tools and a few steps (e.g. conversational RAG), today agent builders have largely abandoned the dynamic and autonomous promise of ReACT for methods that heavily rely on static chaining – the creation of predefined chains designed to solve a specific use case. This approach resembles traditional software engineering and is far from the agentic promise of ReACT. It achieves higher levels of control and reliability but lacks autonomy and flexibility. Solutions are therefore development intensive, narrow in application, and too rigid to address high levels of variation in the input space and the environment.
To be sure, static chaining practices can vary in how “static” they are. Some chains use LLMs only to perform atomic steps (for example, to extract information, summarize text, or draft a message) while others also use LLMs to make some decisions dynamically at runtime (for example, an LLM routing between alternative flows in the chain or an LLM validating the outcome of a step to determine whether it should be run again). In any event, as long as LLMs are responsible for any dynamic decision-making in the solution – we are inevitably caught in a tradeoff between reliability and autonomy. The more a solution is static, is more reliable and predictable but also less autonomous and therefore more narrow in application and more development-intensive. The more a solution is dynamic and autonomous, is more generic and simple to build but also less reliable and predictable.
This tradeoff can be represented in the following graphic:
This begs the question, why have we yet to see an agentic framework that can be placed in the upper right quadrant? Are we doomed to forever trade off reliability for autonomy? Can we not get a framework that provides the simple interface of a ReACT agent (take an objective and a set of tools and figure it out) without sacrificing reliability?
The answer is – we can and we will! But for that, we need to realize that we’ve been doing it all wrong. All current agent-building frameworks share a common flaw: they rely on LLMs as the dynamic, autonomous component. However, the crucial element we’re missing—what we need to create agents that are both autonomous and reliable—is planning technology. And LLMs are NOT great planners.
But first, what is “planning”? By “planning” we mean the ability to explicitly model alternative courses of action that lead to a desired result and to efficiently explore and exploit these alternatives under budget constraints. Planning should be done at both the macro and micro levels. A macro-plan breaks down a task into dependent and independent steps that must be executed to achieve the desired outcome. What is often overlooked is the need for micro-planning aimed to guarantee desired outcomes at the step level. There are many available strategies for increasing reliability and achieving guarantees at the single-step level by using more inference-time computing. For example, you could paraphrase semantic search queries multiple times, you can retrieve more context per a given query, can use a larger model, and you can get more inferences from an LLM – all resulting in more requirements-satisfying results from which to choose the best one. A good micro-planner can efficiently use inference-time computing to achieve the best results under a given compute and latency budget. To scale the resource investment as needed by the particular task at hand. That way, planful AI systems can mitigate the probabilistic nature of LLMs to achieve guaranteed outcomes at the step level. Without such guarantees, we’re back to the compounding error problem that will undermine even the best macro-level plan.
But why can’t LLMs serve as planners? After all, they are capable of translating high-level instructions into reasonable chains of thought or plans defined in natural language or code. The reason is that planning requires more than that. Planning requires the ability to model alternative courses of action that may reasonably lead to the desired outcome AND to reason about the expected utility and expected costs (in compute and/or latency) of each alternative. While LLMs can potentially generate representations of available courses of action, they cannot predict their corresponding expected utility and costs. For example, what are the expected utility and costs of using model X vs. model Y to generate an answer per a particular context? What is the expected utility of looking for a particular piece of information in the indexed documents corpus vs. an API call to the CRM? Your LLM doesn’t begin to have a clue. And for good reason – historical traces of these probabilistic traits are rarely found in the wild and are not included in LLM training data. They also tend to be specific to the particular tool and data environment in which the AI system will operate, unlike the general knowledge that LLMs can acquire. And even if LLMs could predict expected utility and costs, reasoning about them to choose the most effective course of action is a logical decision-theoretical deduction, that cannot be assumed to be reliably performed by LLMs’ next token predictions.
So what are the missing ingredients for AI planning technology? We need planner models that can learn from experience and simulation to explicitly model alternative courses of action and corresponding utility and cost probabilities per a particular task in a particular tool and data environment. We need a Plan Definition Language (PDL) that can be used to represent and reason about said courses of action and probabilities. We need an execution engine that can deterministically and efficiently execute a given plan defined in PDL.
Some people are already hard at work on delivering on this promise. Until then, keep building static chains. Just please don’t call them “agents”.
#2023#2024#agent#agents#ai#ai agent#AI AGENTS#AI systems#AI21#API#approach#atomic#AutoGPT#autonomous#BabyAGI#Building#code#computing#course#courses#crm#data#development#engine#engineering#enterprise#Environment#event#exploit#framework
0 notes
Text
AgentGPT, BabyGPT and AutoGPT - what is the difference?
These are semi-autonomous “agents”, which can be given high level goals “make a website for selling books online”, and can figure out the high level tasks, such as front-end HTML site development, then backend database, etc. and execute each of the tasks and subtasks. They are all the same (at a high level), but use recursive mechanisms to help GPT create prompts for GPT (so meta). Which means…
View On WordPress
0 notes
Text
Aliens or UFOs
The Angry Astronaut had a Q&A about extraterrestrials, aka, ‘Aliens from Outer Space?’.Jorden shared that he is open-minded, ‘Imagen that?’, about time traveling, maultidementional, human/alien visitors. First, I needed to find a way to find the Aliens, so I looked to, @BabyAGI for help explaining how AI might help us? Members Exclusive Livestream! Lets talk UFOs! If you like the videos I list,…
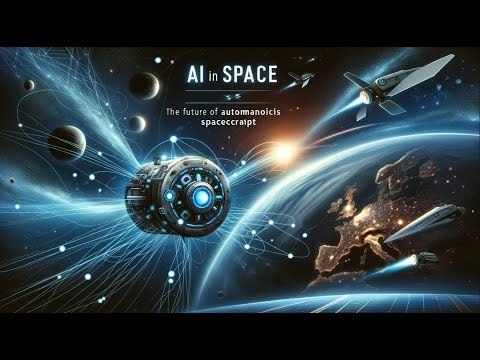
View On WordPress
0 notes
Link
6 notes
·
View notes
Text
https://www.latestdatabase.com/botim-database
LLM OS Guide: Understanding AI Operating Systems Learn what an LLM OS is, how it contrasts with traditional systems like Windows or Linux, and explore early examples like AIOS, BabyAGI, and MemGPT.
0 notes
Text
https://www.latestdatabase.com/botim-database
LLM OS Guide: Understanding AI Operating Systems Learn what an LLM OS is, how it contrasts with traditional systems like Windows or Linux, and explore early examples like AIOS, BabyAGI, and MemGPT.
0 notes
Text
https://www.latestdatabase.com/botim-database
LLM OS Guide: Understanding AI Operating Systems Learn what an LLM OS is, how it contrasts with traditional systems like Windows or Linux, and explore early examples like AIOS, BabyAGI, and MemGPT.
0 notes
Text
https://www.latestdatabase.com/botim-database
LLM OS Guide: Understanding AI Operating Systems Learn what an LLM OS is, how it contrasts with traditional systems like Windows or Linux, and explore early examples like AIOS, BabyAGI, and MemGPT.
0 notes
Text
https://www.latestdatabase.com/botim-database
LLM OS Guide: Understanding AI Operating Systems Learn what an LLM OS is, how it contrasts with traditional systems like Windows or Linux, and explore early examples like AIOS, BabyAGI, and MemGPT.
0 notes
Link
4 notes
·
View notes
Text
https://www.latestdatabase.com/botim-database
LLM OS Guide: Understanding AI Operating Systems Learn what an LLM OS is, how it contrasts with traditional systems like Windows or Linux, and explore early examples like AIOS, BabyAGI, and MemGPT.
0 notes
Text
https://www.latestdatabase.com/botim-database
LLM OS Guide: Understanding AI Operating Systems Learn what an LLM OS is, how it contrasts with traditional systems like Windows or Linux, and explore early examples like AIOS, BabyAGI, and MemGPT.
0 notes
Text
https://www.latestdatabase.com/botim-database
LLM OS Guide: Understanding AI Operating Systems Learn what an LLM OS is, how it contrasts with traditional systems like Windows or Linux, and explore early examples like AIOS, BabyAGI, and MemGPT.
0 notes
Text
https://www.latestdatabase.com/botim-database
LLM OS Guide: Understanding AI Operating Systems Learn what an LLM OS is, how it contrasts with traditional systems like Windows or Linux, and explore early examples like AIOS, BabyAGI, and MemGPT.
0 notes