#ai errors are actually pretty distinct
Explore tagged Tumblr posts
Text
Hmm something about how some people are pointing at art that is "bad" or not anatomically accurate and shouting "it's ai!" and how that's mildly terrifying
#ai errors are actually pretty distinct#i mean#the generators produce boring glitches too#but their tells are distinctive#and to just see a janky looking hand or neck and accuse a real person of being an image generator is really shitty so maybe don't#'this is bad so it must be ai'#idk there's plenty of options
3 notes
·
View notes
Note
Your Morrowind graphics look sooo beautiful! Do you have a list of the mods you used? I'd really appreciate it if you could share it!
Sure! I'd be happy to share my setup =)
My modlist is cobbled together from various modding guides, suggestions by friends who've played Morrowind, and my own personal preferences as I've played and come across things I felt like changing (like the pond scum lol!).
I tried to leave out most mods that had zero to do with Graphics/changes you can see in the world, and I also tried to keep my descriptions short, though if you were only asking for a simple load order then I apologize, oops!
The Engine
MGE XE (this is absolutely vital for those distant views/awesome light and water shaders and other features!)
Morrowind Code Patch (needed for bump/reflection maps to look right!)
Meshes/Textures/Overhauls
Morrowind Optimization Patch (improves performance/fixes some mesh errors!)
Patch for Purists (squashes so many bugs while avoiding unnecessary changes!)
Intelligent Textures (full AI upscaled/hand-edited texture pack of the game, excellent as a base if you plan to add on more targeted replacers later!)
Enhanced Water Shader for MGE XE--OR--Krokantor's Enhanced Water Shader Updated: (depends on which version of MGE XE you're using; if 0.13.0 you'll want the Updated version, and if earlier you need the older one. 3 shades of water to choose from; improved caustics, foam, ripples, underwater effects; and no more weird immersion-breaking moment when you would previously tilt the camera just beneath the surface and it would suddenly be perfectly clear. Absolutely gorgeous water!)
Animation Compilation-Hand to Hand Improved Without Almalexia Spellcasting (idk if this counts, but it does fix the Visual of that weird vanilla running animation!)
Better Bodies and Westly's Pluginless Replacer (a friend told me to get Robert's bodies, but BB is also very good and seems to be the most widely used + many mods need it, like Julan!)
Pluginless Khajiit Head pack (prettier kitties!)
Improved Argonians (better looking lizard-friends!)
Children of Morrowind (adds realism by having kids running around your towns!)
Julan, Ashlander Companion [v3.0 at bottom of this page] (ok not a graphics mod, but will add much immersion to your game, so I will shill for him anyway!)
Vibrant Morrowind 3.0/4.0 (this one I actually don't have installed yet, but I love the way Vivec looks in the screenshots!)
abot Water Life (adds aquatic creatures/things like algae and coral to make Morrowind's waters more alive!)
Vurt's Corals (found on Vurt's Groundcover page; adds gorgeous corals and new water plants!)
Vurt's Ashlands Overhaul (can choose between gnarly trees or vanilla-style!)
Vurt's Groundcover (gorgeous animated grass and vegetation that differs for each region!)
Vurt's Solstheim Tree Replacer II (more realistic trees and snowy pines!)
Vurt's Bitter Coast Trees II (5 additional unique trees!)
Vurt's Bitter Coast Trees II Remastered (mesh fixes/optimizations for the trees!)
Vurt's Leafy West Gash II (more trees, and optional rope bridge texture!)
Vurt's Ascadian Isles Tree Replacer II (v10a recommended for better-sized trees without clipping issues; TREES!!)
Articus Bush Replacer for Vurt AI Trees II (new model for bush tree + bark retexture!)
Vurt's Grazeland Trees II (really cool palms and Baobab trees!)
Vurt's Mournhold Trees II (beautiful animated cherry blossom trees!)
I Lava Good Mesh Replacer (better lava mesh, has no flickering with effects like steam!)
Remiros' Minor Retextures - Mist (much nicer spooky mist in Ancestral Tombs!)
Unto Dust (adds atmospheric floating dust motes, kinda like in Skyrim barrows!)
Graphic Herbalism MWSE (improved meshes and Oblivion-style harvesting!)
Glow in the Dahrk (windows transition to glowing versions at night!)
Ashfall (super awesome and very configurable Camping/Survival/Cooking/Needs mod!)
Watch the Skies (dynamic weathers/weather changes inside/randomized clouds etc!)
Seasonal Weather of Vvardenfell (weather changes throughout the year!)
Taddeus' Foods of Tamriel (adds Ashfall compatible foods and ovens for baking!)
More Wells (add-on for Ashfall/more immersive since access to water is pretty important!)
Diverse Blood (because not everything should bleed Red when you poke it with a spear!)
Lived Towns - Seyda Neen (adds more containers/clutter to make it feel more lived-in!)
Better Waterfalls (adds splash effects/water spray, better running water texture!)
Waterfalls Tweaks (resized water splash to blend better!)
Dunmer Lanterns Replacer (smoother/more-detailed-yet-optimized lanterns + paper lanterns!)
Telvanni Lighthouse Tel Vos (fits in perfectly with Azura's Coast region!)
Telvanni Lighthouse Tel Branora (very atmospheric, works well with surroundings!)
Palace of Vehk (Vivec's Palace feels lived-in instead of sad and empty!)
Ships of the Imperial Navy (immersive addition to Imperial waterfront areas!)
Striderports (gives caravaners some shelter and comfort while standing there all day!)
Illuminated Palace of Vivec (decorates palace steps + shrines with devotion candles and flowers left by followers!)
Scum Retexture - Alternative 2 (better looking pond scum in Bitter Coast region!)
Full Dwemer Retexture (I went with Only Armor/Robots/Weapons; nice high quality retex!)
Blighted Animals Retextured (I chose Darknut's 1024; blighted animals have their own sickly textures now!
Vivec Expansion 3.1 Tweaked Reworked (adds a hostel/many wooden walkways to Vivec on the water!)
Atmospheric Plazas (Vivec's plazas now have weather/sunlight! Be sure to use MCP's Rain fix to keep it from pouring as if there's no roof!)
Gemini's Realistic Snowflakes (more organic texture with more depth!)
Severa Magia DB fix (makes hideout actually appropriate to Dark Brotherhood!)
Starfire's NPC Additions (more populated towns and settlements!)
Hold It (adds items for NPCs to hold and carry, based on their class; very immersive!)
Suran-The Pearl of the Ascadian Isles (I went with White Suran Complete package; stunning retexture that also adds docks/waterfront!)
Atmospheric Delights (a more fitting mood inside the House of Earthly Delights!)
Guars Replacer-Aendemika of Vvardenfell (pluginless makeover for our scaly friends!)
Silt Strider by Nwahs and Mushrooms Team (great new model+textures for these cool bug-buses!)
Skar Face (giant crab manor in Ald-ruhn gets claws and legs!)
Armor/Clothing
Redoran Founders Armor (Redoran councilors stand out in this cool set!)
Morag Tong Polished (bug fixes/Armor Replacer/restored cut content for the faction!)
Rubber's Weapons Pack (several unique weapons/shields get distinct models!)
Yet Another Guard Diversity (generic copypasta guards now have variation!)
Better Silver Armor (adds missing pieces of silver armor to make full set!)
Royal Guard Better Armor (pluginless armor replacer for the Royal Guards!)
RR Mod Series Better Silt Strider Armor (cooler bug men in your Ashlander camps!)
Armored Robes NPC Compilation (some Ordinators/Mabrigash/others will wear distinct robes of their station!)
Full Dragonscale Armor Set v1.3a (adds the missing pieces to make the set complete!)
Mage Robes (robes for every magic school, many MG members will wear their respective ones!)
Quorn Resource Integration (lore-friendly armor/creatures added to leveled lists to be encountered in game!)
Better Clothes (non-segmented clothing replacer to fit Better Bodies!)
More Better Clothes (additional shirts that were missed in the first one!)
Better Clothes Complete (fixes many problems and 1st person clipping issues for BC!)
Better Clothes Retextured (high-res retextures for nearly all base game clothes!)
Hirez Better Clothes (3 shirts retextured in high quality!)
Better Morrowind Armor (BB compatible armor replacer!)
Dark Brotherhood Armor Replacer (changes DB armor to look more like concept art!)
Bonemold Armor Replacer (much nicer-looking Bonemold armor!)
Westly's Fine Clothiers of Tamriel (very high quality clothes that you will see many NPCs wearing too!)
Orcish Retexture v1.2 (beautifully done armor retexture!)
Daedric Lord Armor (much improved Daedric set, very fierce!)
Ebony Mail Replacer (awesome new model+tex that changes it to actual chainmail!)
I use Wrye Mash to install my mods, though I think a lot of people use MO2. Haaa, now that I've made this list I have the strong urge to just run around Morrowind taking even more screenshots =)
26 notes
·
View notes
Text
I think people know by now how to tell if an image of a person is AI-generated. Count the fingers, count the knuckles, check the pupils, yadda yadda. I've seen several posts circulating about what to look for. However, I think people are a LOT less educated about backgrounds, and about the specific distinctions between human error and AI error. So that's what I'm going to cover.
Now, don't feel bad if you've reblogged or liked any of the images I'm about to show you guys. This is just what's crossed my blog, so it's what I have to work with. (Actually, thanks for providing the examples!)
I also generated a few images from crAIyon purely for demonstrational purposes, because I didn't have anything on-hand to show my thoughts.
Firstly — Keep in mind that AI has a difficult time replicating "simple" styles. Think colorless line-drawings, cartoony pieces with thick lines, and pixel art.
Looks unsettling, right?
Why is this? Well, when a human makes art, we're more prone to under-detailing by mistake than over-detailing, because adding detail in the first place place is more effort. A skilled artist should be good able to capture an idea with minimal, evocative shape language.
But when an AI makes art, it is the opposite. An AI doesn't understand what it's looking at, not in the way that you or I do. All it can do is search for and replicate patterns in the noise of pixels. As a result, it is prone to mushing together features in ways that a human artist . . . wouldn't intentionally think to do.
It also over-details, replicating what it knows over and over again because it doesn't know when it's supposed to stop. Blank spaces can confuse it! It likes having detail to work with! Detail Is Data!
Again, this is why we count fingers.
These general principles still apply when we're looking at styles that an AI is better equipped to imitate. So . . .
Secondly — AI's tendency to over-render details makes it easier for it to pick up heavily detailed styles, especially if the style will still hold up when certain details are indistinct or merge together unexpectedly.
Scrutinize images that utilize a painterly, heavily-rendered, or photo-realistic style. Such as this one.

Thirdly — An AI piece that looks pretty good from a distance falls apart up close.
The above image looks almost like a photograph, but there is architecture here that you wouldn't find in a real room, and mistakes that you wouldn't find in the work of an artist that is THIS good at rendering. Or most beginner artists, even.
Can you see what falls apart here? Hint; we're counting fingers again.
Check the window panes. Isn't the angle that they all meet up at a little off? Why are the panes sized so inconsistently? Why doesn't the view outside of them all line up into a cohesive background?

Count the furniture legs. Why does the farther-back case have a third leg? Why does the leg on the closer case vanish so strangely behind the flowery details?

Examine the curtain(?) fabric at the top of the window. What on earth IS that frilly stuff?
Another mistake that AI will make is drawing lines and merging details that a human artist would never think of as connected. See the lines crawling up the walls? See how some of the flower petals glop together at hard angles in some places? Yeah, that's what I'm talking about.
You can see more strange architecture in the outdoor setting of this image.

A lot of the AI's mistakes are almost art nouveau! We recognize that buildings are consistently angular, for stability reasons. An AI does not. (Also look at the trees in the background, and how they tend to warp and distort around the outline of the treehouse. They kinda melt into each other at some points. It's wild.)
Fourthly — An AI will replicate any carelessness that was introduced into its original data set.
Obviously, this means that AIs will make fake watermarks, but everybody already knows that. What I need you guys to look out for is something else. It's called artifacting.
Artifacting is defined as "the introduction of a visible or audible anomaly during the processing or transmission of digital data." To put it in layman's terms, you know how an image gets crunchy and pixelated if you save it as a jpg? Yeah. That. An AI with lots of crusty, crunchy jpgs fed into it will produce crunchy images.
Look at the floor at the bottom of our original example image;

See the speckles all along the glass panels, table legs, and flowers in shadow? Artifacted to hell and back! This shit is crunchier than my spine after spending half a day hunched over my laptop.
Again, legitimate art and photography may have artifacting too just because of file formatting reasons. But most artists don't intentionally artifact their own images, and furthermore, the artifacting will not be baked into the very composition of the image itself. The speckles will instead gather most notably on flat colors at the border of different color patches and/or outlines.
Cronchy memes; funny. Cronchy AI art; shitty jpg art theft caught red-handed.
That's probably all the lessons I can impart in one post. Class dismissed! As homework a bonus, consider these two sister images to our original flower room. Can you spot any signs of AI generation?


@wolven-writer I hope this helps!
You know those aesthetic image posts that float around tumblr? I'm . . . starting to see a lot on my dash that are obviously ai-generated. Are non-artists having trouble telling the difference between AI images and real photos, or are people starting to stop care about the stolen art that gets fed into those programs?
#some AI is good enough that it does this stuff minimally#AND YET#people are posting obvious images anyway#because they can't tell the things they generate willy-nilly with a click of a button from things that artists do intentionally#anyway please spread this
43K notes
·
View notes
Text
Locked
(gif not mine)
Pairing: Loki x Reader (ft. the Avengers)
Content/Warnings: Angst; fluff; Clint being a bit of a jerk
Words: 1603
A/N: Well, the long awaited sequel to Spinning has finally arrived. I kinda drew inspiration from Imagine Dragons’ song Next To Me for this fic, so make sure to check out that song because it’s honestly awesome. Anyways, enjoy!
Part 1
For several moments, you weren’t completely sure if Thor was planning on attacking or congratulating Loki. Jane seemed to be thinking along the same lines, moving to place a restraining hand on Thor’s chest, but the thunder god finally relaxed and gave his brother a beaming smile. “Well congratulations then, brother!”
“Thank you, Thor,” Loki said smoothly, pulling his sleeve back down. “Though, if you don’t mind, I would like to get to know my soulmate, so if you could take your friends elsewhere…”
“Oh, right, of course. Come Jane, Darcy. Stark has put in a new microwave, it even speaks to you!” Thor said happily. Jane looked amused, as did Darcy, who sent you a discreet thumbs up before pulling the door shut behind her.
Your fingers tapped nervously against your thigh. “So…”
“Would you like to sit?” Loki offered, waving towards the couch.
“Sure, yeah.” You accepted the invitation, taking a seat on the couch and tucking a leg under you. “So you’re stuck here, huh?”
“There are worse fates, I suppose,” He mused, stretching an arm across the back of the couch. “What about you? Where are you from? I’ve been reading up on Earth’s geography, though I can’t promise I’ll recognize the place.”
“Well, you know where Thor was first banished to? New Mexico? That’s where I live, I went there for college which is how I met Jane and Darcy,” You explained. “We’re a pretty unlikely group of friends, to be honest.”
Loki’s lips flicked upwards into what could almost be called a smile. “I had noticed. So you were there when Thor was mortal?”
“No. I was actually visiting family and missed all the action, believe it or not. Just my luck.”
“It’s probably for the best, I did try to kill him. I’m thankful that you weren’t there to get caught in the crossfire,” He admitted.
“That’s one thing I don’t get. Why do you hate Thor so much? I mean, it’s obvious that he cares about you,” You pointed out.
That gave Loki pause. “I suppose we just never really gotten along. He’s Thor, the oh-so-perfect Asgardian prince, and I’m Loki, the frost giant who will never be good enough.”
“That’s not true,” You said softly, honestly surprised he had confided in you like that. “Loki, adopted or not, the two of you are brothers. It’s obvious that Thor doesn’t care that you’re a frost giant, he just cares that you’re his brother. And for the record, I don’t care that you’re a frost giant, either.”
He didn’t appear to be convinced. “We’ll see.”
You ended up moving into Stark Tower a couple weeks after meeting Loki. Both he and the other Avengers wanted to make sure you were safe from external threats, which considering your soulmate was Loki, was probably a good idea. It was only natural for you to want to, anyways, since that’s where your soulmate was, but the fact that you were hanging around a gang of superheroes took some getting used to. Not to mention the fact that one of the most hated men on Earth was your soulmate, but that was a whole other can of worms.
There was a learning curve when it came to being around super spies and technical geniuses, but you ended up learning pretty quickly. In fact, there were three things you learned within your first week. One: never leave food unattended, otherwise it will get eaten. Two: don’t attempt to sneak up on Natasha. It didn’t end well for Tony and you doubted it would end any better for you. And three: never leave Clint and Loki alone in a room together.
Understandably, Clint didn’t particularly like Loki. Saying Clint loathed Loki would be a more apt phrase. Unfortunately, this meant Clint would pick a fight with the god of mischief which ended with Clint getting his ass handed to him or Loki stalking away to sulk for a few hours.
About three weeks into you moving into the Tower, however, things went a bit too far. You had spent most of the day with Natasha, the two of you having a girl’s day getting to know each other better, and when you returned you were intent on going straight to find Loki. He could usually be found in his room, or the library, or even the common room on the rare occasion that he was feeling like speaking with the other Avengers. However, he was nowhere to be found.
“Have any of you seen Loki?” You asked, addressing Tony, Clint, and Steve who were discussing their latest mission. “I can’t find him anywhere.”
Clint choked on the water he was drinking, causing the other two men to give him confused glances. Tony shrugged. “No, I haven’t. I’m sure Reindeer Games is around somewhere, just use your compass. That is what it’s there for.”
“I saw him in the library this morning, if that helps,” Steve offered. “But I haven’t seen him since, sorry. Clint?”
The archer cleared his throat. “Oh, um… no. I haven’t seen him.”
“He’s probably just with Thor or something,” You said with a shrug. “Thanks anyways, guys.”
You gave them a wave before wandering off, deciding to just chill in your room and watch some Netflix, though you couldn’t rid yourself of the distinct feeling that something was wrong. You had been encouraged (mainly by Steve) to follow your gut, especially if you thought something wasn’t right, so you glanced down at your compass and started walking in the direction it was pointed.
It took a little bit of trial and error to figure out what floor Loki was on, though you figured once your compass stopped wobbling uncertainly that you were in the right place. You were surprised that he was on the floor devoted mainly to training and gym activities and followed the compass on your skin towards the showers.
He wasn’t anywhere to be seen, and the floor was quiet as the Avengers were all off eating dinner. “Loki?” You called, padding through the shower room. There was no answer. “Jarvis, have you seen Loki?” Perhaps you should have asked the AI first.
“He appears to be in the sauna,” Jarvis responded promptly.
“Well what’s he doing in there?” You muttered, mostly to yourself. You jiggled the handle of the sauna door - it was locked. Something was definitely wrong. “Jarvis, can you unlock the door to the sauna?”
“Of course,” Jarvis said, the lock clicking a moment later. You yanked the door open, immediately assaulted with a wave of nearly nauseating heat.
You barely had to go in to find your soulmate, slumped against the wall by the door. “Loki? Are you okay?”
He stirred at your statement, glancing up at you warily. You let out an audible gasp at his appearance - his skin was completely blue and his eyes were red. The heat must have forced him back into his natural frost giant form. “No.”
“Jarvis, turn off the heat in the sauna,” You ordered, crouching down to drape his arm over your shoulder and heave him up. “Let’s get you out of here.”
You had to half-drag Loki out of the sauna, and he was heavier than he looked. “Here, lean against the wall,” You ordered, yanking open the curtain to the nearest shower and turning it on, making it as cold as possible. “Get under the water.”
He managed to stumble over, and you got significantly doused whilst preventing him from falling face-first on the tile. The icy water seemed to revive him. “Y/N?” He asked.
“Yeah, it’s me,” You confirmed. “Are you okay?”
“I’ll live,” He said, still leaning heavily on you.
“Here, we need to get your shirt off. It’ll help cool you down,” You ordered, and you jumped as his form flashed and a majority of his clothes vanished, sans what appeared to be a pair of swim trunks. “I thought you couldn’t do magic?”
“I can do some. Not a lot. Don’t tell Thor,” Loki admitted. He seemed to be regaining his strength, at least enough that he was speaking coherently once more, and it was only now that he realized his skin was blue rather than its normal pale color. He looked down at himself, then back at you, then at himself once more.
“It’s okay,” You assured him.
“I look like a monster,” He spat, disgust in his voice. “It’s not okay. Not to mention you should have frostbite from touching me.”
“Well, you don’t want to give me frostbite, do you?” You asked. His head gave a brief shake. “I figured as much. I believe you have more control than you think you do, Loki. And I don’t think you look like a monster.”
He snorted. “You’re lying.”
“No, I’m not. I’ll prove it.” You pulled him down into a kiss, only moderately surprised when he didn’t jerk away and instead wrapped an arm around your waist to deepen the kiss. His lips were cool, moving in perfect sync with your own.
Later, after you had punched Clint for locking Loki in the sauna, you’d claim that you and Loki had your first kiss out in Central Park and not under an icy shower. But the two of you knew, and many months later Loki admitted to you that that moment was when he realized he had fallen in love with you. You smiled, said you knew, and told him you loved him too.
Life was good with the man you loved. And you never had a directionless compass again.
Tag List: @the-crime-fighting-spider @micachu1331 @esoltis280 @ilvermornyqueen @teaand-cookies @alittlebitofmagic @bluebird214 @lovely-geek @fleurs-en-ruines @loki-god-of-my-life @awesomehaylzus @ldyhawkeye @ldyhawkeye @small-wolf-in-the-snow @the-bleeding-rose @momc95 @loki-laufey-son @hp-hogwartsexpress @haven-in-writing @alivingfanlady @micachu1331 @little-miss-mischief1 @pepperr-pottss @t-talkative @lady-loki-ren @usedtobeabaker @val-kay-rie @inn-ocuous @xclo02 @ex-bookjunky @stone2576 @dkpink123 @loki-laufey-son
#loki#loki x reader#loki imagine#loki fanfiction#loki x you#marvel#marvel x reader#marvel imagine#loki laufeyson#loki laufeyson x reader#loki laufeyson imagine#reader#reader insert#x reader
1K notes
·
View notes
Text
Top-notch Web Design & Development Trends You Should In 2021
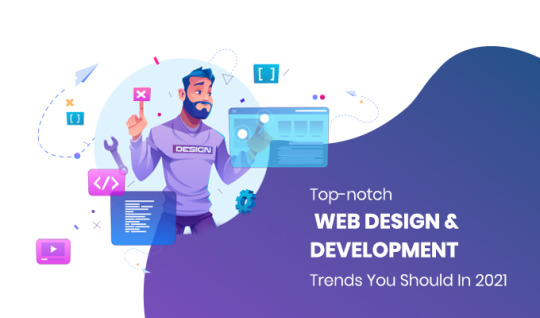
Technology keeps on evolving as humans realize new ways to innovate, doing things quicker and creatively than they did before. Developers are perpetually trying ahead to explore new technologies that may propel them towards a brighter future. The trendy business is steadily developing, and new web technologies arise each day.
These new trends supply tons of prospects for entrepreneurs who need interaction with more users and keep ahead during this competitive market. These advantages and innovation within the space of custom web design and development have galvanized several entrepreneurs to speculate in website development. However, making the proper website isn't as simple as it seems.
Web design and development describes the process of creating a website. The umbrella term involves web design and web development, where web design determines the look and feel of a website, while web development determines how it works. Hiring a professional web design company for your business website has many benefits since the experienced company can assist you with the latest trends and development in the market. Here are the top seven web design and development trends that you should consider in 2021.
1. AI and chatbots
Artificial intelligence is a revolutionary technology that has been employed in varied industries. It's conjointly generally enforced within the field of web development in various ways to produce an additional robust client experience. One among the numerous ways within which AI is implemented is for merchandise or content recommendations for websites. Websites of YouTube and Netflix are solid examples of how AI is used to recommend content to users as per their preferences.
It's believed that this year bots can become more self-learning and meet a specific user’s necessities and match behavior, which means that operating bots can take the place of support managers 24/7 Associate in the system. Therefore AI may be a technology that's helpful for business automation and prognostication strategies. Additionally, AI conjointly helps in enhancing your website through reinforcement learning.
2. Progressive web Apps (PWA)
Among the highest trends in web development, we must always mention PWA (Progressive web apps) technology. It suggests that it blends within the practicality of an internet app and a native mobile app. It operates severally on the browser and interacts with the shopper as a native application. So, it's an internet app at the core; however, it has native app-like attributes. You'll be able to install a PWA similar to a native app and may share it as well. Recently, many companies prefer custom website development so that they will have a unique design for their customers.
3. Accelerated Mobile Pages (AMP)
Accelerated Mobile Pages (AMP) is a trend in web development to hurry up page performance and cut back the prospect of users feat it. AMP technology is incredibly near to PWA. The distinction is that in AMP, pages become accelerated due to the ASCII text file plugin developed by Twitter and Google. With additional individual's mobile devices, your website should supply seamless expertise to users on mobiles. Websites typically fail to realize this side as websites are big, having tons of content like images, videos, etc., affects those web pages' loading speed. An experienced web design and development can help you solve many issues related to developing websites.
AMP solves this problem that loads quickly on mobile devices. These web pages are specifically optimized to assure the quick loading of webpages on mobile devices while not the necessity for classy coding. They have a reduced and nonetheless convenient style with solely basic options compared to full-scope net results. These web pages are mobile-friendly, and their content is often readable. You'll be able to take up AMP development services to make a fast-loading website that mobile users can use on their mobile devices.
4. Serverless Design
2020 was marked by the difference of work-from-home by corporations thanks to the unfolding of Covid-19. Cloud applications have enlarged even more throughout this year owing to this. Custom website design, mutually of the foremost speedily growing domains, documented a considerable rise in serverless design demand. Consistent with the State of the cloud report by Flexera, 98% of enterprises adopt a minimum of one public or personal cloud. Serverless algorithms were designed as a cloud-computing execution model. The latest trends offered by web design and development services involve serverless app architecture facilitating decreased development and progress maintenance budgets, strengthening apps, and keeping the web atmosphere sustainable.
5. Motion UI
This web development trend falls in the style of web products. Startups are paying additional attention to the user experience. Pleasant-looking websites and apps have more probability of being detected by doable users and become viral. In a very Salesforce Report, it's explicit that 84% of shoppers read the website's look as necessary because of the actual service it offers. Web design isn't perpetually concerning pretty pictures, and it is about making responsive interfaces that your users can like.
6. Automation Take A Look At
Automation testing utilizes an automation tool to perform the task. To line up automation testing for a startup business is to attenuate the number of test cases that need to run manually instead of dropping manual testing. Automation testing is finished not solely to urge higher ROI; however, conjointly resulting from it will increase the scope of test coverage and rule out human interference and human errors. Why is test automation so necessary for web development in 2021? The solution may be a digital atmosphere that becomes additional and more competitive. If you're quicker than your competitors and the quality of your product and services is healthier — you may succeed.
7. Blockchain Technology
Cryptocurrencies aren't the most recent web development technology. The conception of them appeared initially in 2004, and many years ago, the crypto mercantilism market (based on blockchain technology) explored investments. What will we tend to expect in 2021, significantly within the field of web development? The usage of blockchain currency trading became considerably active at intervals over the past decade, and major payment systems began to settle for Bitcoins and alternative cryptocurrencies.
Conclusion:
Websites have become additional and more refined as powerful strategies and technologies are being introduced within web development. You will have to make use of those preceding web development trends to develop strong and out-of-the-box websites. Besides, developing a website with Auxesis Infotech is less complicated as you can have an expert team from the company to make attractive websites.
#webdesigntrends#webdesign#websitdeign#webdesigner#ui#ux#design#webdesigninsprations#website#elegantthemes#websitedevelopment#uxdesign#uidesign#responsivedesign#webdesignagency#webdeveloper#webdevelopmenttrends#onlinewebstore#financeandbanking#wellness#travel#healthcare#newsandpublocations#realestate#socialnetworking#elearning#sports#restaurantandfooding
0 notes
Text
OI???
i wonder how's he doing it. i participated in a similar project so i'm gonna try to explain what i know about ways to ✨read one's mind™✨
option 1. motor imagery
we were making an orthosis (i may use the wrong words since i didn't read anything in english abt this) for paralyzed folks who had a stroke, so basically we needed to detect the intention to bend a finger and then bend it artificially. this is the most straightforward way to do it, but also the most ineffective.
you literally just imitate the brain activity of moving your arm. and it is not as easy as it sounds. it actually takes trial and error and work
our methods are imprecise bc we don't read the activity of every neuron or smth, we gather the average from large groups (otherwise it would require invasive methods aka surgery). therefore we could differ between left arm, right arm, probably legs and that's about it
overall it's not what we want to use to play minecraft
option 2. P-300
that's what we used. it's frequently used to make things like a keyboard or some kind of remote control, y'know, with EEG (aka the helmet that reads brainwaves).
afaik usually there are a few flashing lights, but you concentrate on one of them and your brain reacts to it in a distinct way
a lot of flashing lights are involved when there are a lot of buttons. personally i'm not a big fan, but apparently people think that's okay?
option 3. i know nothing about this one but it sounds very fun
i dont know how it works, but with this one it may be a common occurrence. like you just put on the helmet and teach your neuronets (either brain or AI idk. likely both) like a dog. you try and if you're happy with what's going on, they keep it up; if you're not, they adjust their behavior. (again i don't know shit about this one and i'm probably wrong.) i've heard of two cases when this was used:
people sat in front of a screen with a solid colour that was somehow calculated from their brain activity, and the colour fluctuated (bc brain waves are pretty chaotic) until it came to the steady state of the colour brain liked the most (often green or orange)
a person learned to drive a remote control car with that. i think the person was the head of our laboratory but idk i've never seen him irl
to me this one sounds like it's just your pure will translated into a sequence of commands. mere magic. i hope this one is used.
out of everything that happened today, i feel like we should talk more about how fundy bought a helmet that reads fucking brainwaves and how he’s going to create a self-learning ai so he can use it to play minecraft. that’s fucking insane
#fundy#mcyt#literally me: oh! fundy is doing things i know something about while others might not! >#let me infodump about my previous unfinished project while procrastinating my current one despite it being due a week ago!#anyways i'm trying to teach tumblr users neurobiology rn. i know i'm doomed to fail but i would be really happy if i don't so >#so if you're still reading please rb. it would mean the absolute world to me <3#btw this is a quote from a youtube channel called fungithefox that i'm subscribed to. i think the intonations are really cool ksjssksjksjsk#it's a fundy clip channel#i need to stop this rant at once
495 notes
·
View notes
Text
appmon afterthoughts
appmon is finally over! it’s been a great journey. ;v; i drop shows easily when watching them week by week so i prefer binge-watching them at once, so appmon is the first show of this length that i managed to watch as it aired all the way through! (i dropped off somewhere in neovamdemon’s arc when trying to keep up with xros wars, haha. i did go back and finish it after that though!)
my personal preference of seasons: frontier > adventure > *appmon* > savers > 02 > hunters > tamers > tri > xros wars (as usual i still love all the seasons!! this is just if i had to rank them. i won’t deny that the 7 death generals arc was a bit of a drag for me though..)
here are my (LONG and incoherent) thoughts after watching the series, spoilers under the cut.
characters: - gosh i love the main cast so much!! ;v; i’m also glad that the appmon get a fair amount of characterisation and focus too (though still not as much as their human buddies), i feel there are times when digimon gives focus to the humans but in turn sacrifice some of the focus that their monster partners get. - i live for character interactions, so while i’m glad that haru/eri/astra interact with each other a lot, it’s a bit disappointing to see how little interaction rei and yuujin get with eri and astra. :( and hackmon never really interacts with the others much, or at all..i like hackmon, but it’d be nice to see him talk to someone other than rei for once. - i love the character growth in this season so much ;; possibly just behind frontier. eri and astra’s growth wasn’t as overt possibly due to how they express their personalities, but they throw a lot of it about the ‘filler’ eps and it all comes together really nicely. haru gets visibly stronger and more confident throughout the show, and rei’s change in reaction to his applidrive’s “are you alone?” question alone says so much. - on that note, i LOVE how they handled yuujin’s question (would you give your life up for a friend). in the end, it’s not those flashy scenes where you take a fatal hit for someone, but yuujin giving his life up not just to save humanity, but more importantly to save haru from having to shoulder the heavy burden of actually making the choice to kill yuujin. i thought that was a really powerful scene and it really got to me. - (shipping) haru and rei...i don’t care if it’s romantic or platonic or whatever i just love seeing them interact so, so much. people who know i like other pairs like seliph/ares, aichi/kai, etc...it’s the same pattern, nice pure boy gets the brooding edgy jerk to open up. i am a predictable person lol
story: - there are a lot of fillers. (but what is a digimon season without fillers?) i like fillers myself (probably why i like hunters when many people hate it), but i read the wtw comment threads every week and you get tons of complaints every time it hits a filler ep, and i can somewhat understand their frustration. appmon can be a drag to watch if you’re the kind who hates fillers. (i don’t deny a few fillers like the maripero ep did bore me though) - appmon does handle the main plot progression better than hunters though, despite the still whack pacing, and the fillers still tend to have nice character bits/growth. i love hunters but i won’t defend its absolute disregard for plot then trying to cram everything in at the last minute haha. still there are a number of unanswered questions..while i do agree that not all questions necessarily need answering, they can still provide deeper insight to characters. - personally, i liked how they kept the lightheartedness of the story while touching on salient AI-related issues. but while they bring up some very interesting issues, i don’t feel like they addressed them satisfactorily (at least from my pov)? leviathan’s aim with the humanity applification plan was to eradicate problems like conflict, disease, and human error from humanity, which is in a way even backed up by haru’s grandpa, who mentions “being data is great! without a physical body, one has no need to worry about injuries or sickness”, coming from someone who died in part because of sickness. you can see where the protags are coming from, but they never really address these ‘benefits’ of the humanity applification plan and how the benefits of not going through with the plan would outweigh the benefits of going through. - app-fusion might work well as a game mechanic, but i think it only serves to detract from the story in the anime, at least the way it is right now. for two series whose evolution is centred around fusion, xros wars handles fusion much better, utilising more creativity in both using and fusing the ‘fodder digimon’. appmon just tends to forget its fodder appmon exist. i personally think that appmon would be better off if its app-fusions were treated as simple evolutions instead (that’s pretty much how they treat the buddy appmon anyway; globemon is pretty much treated as ‘evolved gatchmon’, rather than an actual fusion of dogatchmon and timemon), that way you don’t get the nagging feeling that the fusion fodder appmon are just..fodder. - speaking of app-fusion, i have to say i personally prefer the more emotion-driven evolutions from the earlier seasons, rather than the evolutions achieved by getting the correct chip as we see in appmon. it makes sense from a gameplay perspective, but in context of the anime it feels..less impactful, i guess? i just always love seeing the bonds between the humans and their partners get tested, and become even stronger. - on an unrelated note, i find it funny that the show has a subplot involving two computer genius brothers and the cicada 3301 thing, mainly because i have a FDD story centered around the same idea (that i don’t make progress on at all. it probably looks like an appmon ripoff now but i don’t care haha)
designs: - i love the standard grade main appmon designs, they’re all so cute ;w; they have this distinct style in mind and i think they pulled it off well. (i’ve warmed up to musimon’s design A LOT from when he was first revealed, but i do still think it could be slightly less cluttered) - the ultimate grades are PERFECT, they’re some of my favourite digimon designs and possibly one of my favourite ‘group’ of designs out of protagonist digimon!! (possibly only bested by the frontier beast spirits and maybe the savers ultimates/tamers adults? haha) i just...yes. they’re amazing. i love them so so SO much - i’m not a fan of the direction they took with the god grades (maybe because i love the ultimate grades too much lol). all the gold didn’t sit too well with me either, maybe because we already had so much gold in xros wars? i do think they make great ‘final forms’ for the protagonist mons, but personally i still greatly prefer all their other forms to their god forms. i’m a bit more partial to hadesmon than the others because i LOVE jesmon, but hm...hadesmon still looks a lot more gaudy..like jesmon’s gaudy little brother. hahaha - i think the level system is a nice simplification from digimon. hopefully this means we can see appmon in future digimon games..they would be easier to implement than xw digimon anyway, haha;;
animation: - like many others i was skeptical about the making of higher-grade appmon 3DCG at first, though it eventually grew on me. the fights between 3DCG appmon were nicely done, but seeing the difference in animation between the 2D characters and 3DCG appmon was jarring, especially in shots where they’re together, mostly because of the framerate..the 3DCG appmon are animated on 1s? while the humans are animated on 3s like normal anime, it’s a big difference. thankfully most 3DCG fights don’t bring in the humans much. - the models/3D animation are still pretty well done! and i appreciate that they didn’t render them cel-shaded like what most anime do with 3D models (i remember translating the appmon interview mentioning why they did this, before appmon started airing; i was skeptical but now i can see what they were going for and i think it turned out well!) - after watching appmon i think 3DCG is a nice move for toei though, because we all know toei’s animation quality...could be better? hahaha. but i find toei’s weakness isn’t so much layout/choreography, but more of sometimes poorly-drawn frames, bad timing, or too little inbetweens, some of which are solved with 3DCG. you can especially see the contrast with digimon tri’s fight scenes; highly detailed digimon like jesmon for example would’ve benefited greatly from 3DCG, i know how painful it is to translate all of its details to 2D animation but as you can see it results in quite a number of not-as-nicely drawn frames. - special mention to charismon because i really like how he was modeled/rigged. those eyes!! can you imagine duskmon in 3D doing that and with those creepy sound effects too. - i’m not a huge fan of the palettes used in the AR-fields..(i didn’t like how the digiquartz was depicted that much either, and their depictions are quite similar so yeah) i can definitely see the effect they’re going for, but it felt more ‘kiddy alien-ish’ than ‘digital’ to me.
music: - i found the music quite ok (i liked DiVE!! and BE MY LIGHT though!), but i guess it didn’t match up to my personal tastes as much :x sadly appmon might be the lowest of the digimon seasons when it comes to music for me, i liked that endings are back! but the songs themselves didn’t captivate me as much as the previous seasons’ ending songs did. - on that note i’m glad they put in an insert song though! i guess i’m just really big on insert songs in digimon because as a kid i printed out the lyrics to brave heart and the other evo songs and loved singing along when they played in the show. lol - i remember complaining about this when the first episode aired, and my opinion still hasn’t changed 52 eps in. i CANNOT stand the applidrive voice at all hahaha (and the speed-up effect they use when app-linking/fusing) - the character songs are cute!! i’m personally really glad they decided to make them :) - the background music was pretty nice and had some memorable tracks..i’m not quite sure how i’d compare it to the rest? i liked all the soundtracks so far, though xros wars’ and frontier’s osts stood out especially for me.
13 notes
·
View notes
Text
Improving Medical AI Safety by Addressing Hidden Stratification
Jared Dunnmon
Luke Oakden-Rayner
By LUKE OAKDEN-RAYNER MD, JARED DUNNMON, PhD
Medical AI testing is unsafe, and that isn’t likely to change anytime soon.
No regulator is seriously considering implementing “pharmaceutical style” clinical trials for AI prior to marketing approval, and evidence strongly suggests that pre-clinical testing of medical AI systems is not enough to ensure that they are safe to use. As discussed in a previous post, factors ranging from the laboratory effect to automation bias can contribute to substantial disconnects between pre-clinical performance of AI systems and downstream medical outcomes. As a result, we urgently need mechanisms to detect and mitigate the dangers that under-tested medical AI systems may pose in the clinic.
In a recent preprint co-authored with Jared Dunnmon from Chris Ré’s group at Stanford, we offer a new explanation for the discrepancy between pre-clinical testing and downstream outcomes: hidden stratification. Before explaining what this means, we want to set the scene by saying that this effect appears to be pervasive, underappreciated, and could lead to serious patient harm even in AI systems that have been approved by regulators.
But there is an upside here as well. Looking at the failures of pre-clinical testing through the lens of hidden stratification may offer us a way to make regulation more effective, without overturning the entire system and without dramatically increasing the compliance burden on developers.
What’s in a stratum?
We recently published a pre-print titled “Hidden Stratification Causes Clinically Meaningful Failures in Machine Learning for Medical Imaging“.
Note: While this post discusses a few parts of this paper, it is more intended to explore the implications. If you want to read more about the effect and our experiments, please read the paper
The effect we describe in this work — hidden stratification — is not really a surprise to anyone. Simply put, there are subsets within any medical task that are visually and clinically distinct. Pneumonia, for instance, can be typical or atypical. A lung tumour can be solid or subsolid. Fractures can be simple or compound. Such variations within a single diagnostic category are often visually distinct on imaging, and have fundamentally different implications for patient care.

Examples of different lung nodules, ranging from solid (a), solid with a halo (b), and subsolid (c). Not only do these nodules look different, they reflect different diseases with different patient outcomes.
We also recognise purely visual variants. A pleural effusion looks different if the patient is standing up or is lying down, despite the pathology and clinical outcomes being the same.
These patients both have left sided pleural effusions (seen on the right of each image). The patient on the left has increased density at the left lung base, whereas the patient on the right has a subtle “veil” across the entire left lung.
These visual variants can cause problems for human doctors, but we recognise their presence and try to account for them. This is rarely the case for AI systems though, as we usually train AI models on coarsely defined class labels and this variation is unacknowledged in training and testing; in other words, the the stratification is hidden (the term “hidden stratification” actually has its roots in genetics, describing the unrecognised variation within populations that complicates all genomic analyses).
The main point of our paper is that these visually distinct subsets can seriously distort the decision making of AI systems, potentially leading to a major difference between performance testing results and clinical utility.
Clinical safety isn’t about average performance
The most important concept underpinning this work is that being as good as a human on average is not a strong predictor of safety. What matters far more is specifically which cases the models get wrong.
For example, even cutting-edge deep learning systems make such systematic misjudgments as consistently classifying canines in the snow as wolves or men as computer programmers and women as homemakers. This “lack of common sense” effect is often treated as an expected outcome of data-driven learning, which is undesirable but ultimately acceptable in deployed models outside of medicine (though even then, these effects have caused major problems for sophisticated technology companies).
Whatever the risk is in the non-medical world, we argue that in healthcare this same phenomenon can have serious implications.
Take for example a situation where humans and an AI system are trying to diagnose cancer, and they show equivalent performance in a head-to-head “reader” study. Let’s assume this study was performed perfectly, with a large external dataset and a primary metric that was clinically motivated (perhaps the true positive rate in a screening scenario). This is the current level of evidence required for FDA approval, even for an autonomous system.
Now, for the sake of the argument, let’s assume the TPR of both decision makers is 95%. Our results to report to the FDA probably look like this:
TPR is the same thing as sensitivity/recall
That looks good, our primary measure (assuming a decent sample size) suggests that the AI and human are performing equivalently. The FDA should be pretty happyª.
Now, let’s also assume that the majority of cancer is fairly benign and small delays in treatment are inconsequential, but that there is a rare and visually distinct cancer subtype making up 5% of all disease that is aggressive and any delay in diagnosis leads to drastically shortened life expectancy.
There is a pithy bit of advice we often give trainee doctors: when you hear hoofbeats, think horses, not zebras. This means that you shouldn’t jump to diagnosing the rare subtype, when the common disease is much more likely. This is also exactly what machine learning models do – they consider prior probability and the presence of predictive features but, unless it has been explicitly incorporated into the model, they don’t consider the cost of their errors.
This can be a real problem in medical AI, because there is a less commonly shared addendum to this advice: if zebras were stone cold killing machines, you might want to exclude zebras first. The cost of misidentifying a dangerous zebra is much more than that of missing a gentle pony. No-one wants to get hoofed to death.
In practice, human doctors will be hyper-vigilant about the high-risk subtype, even though it is rare. They will have spent a disproportionate amount of time and effort learning to identify it, and will have a low threshold for diagnosing it (in this scenario, we might assume that the cost of overdiagnosis is minimal).
If we assume the cancer-detecting AI system was developed as is common practice, it probably was trained to detect “cancer” as a monolithic group. Since only 5% of the training samples included visual features of this subtype, and no-one has incorporated the expected clinical cost of misdiagnosis into the model, how do we expect it to perform in this important subset of cases?
Fairly obviously, it won’t be hypervigilant – it was never informed that it needed to be. Even worse, given the lower number of training examples in the minority subtype, it will probably underperform for this subset (since performance on a particular class or subset should increase with more training examples from that class). We might even expect that a human would get the majority of these cases right, and that the AI might get the majority wrong. In our paper, we show that existing AI models do indeed show concerning error rates on clinically important subsets despite encouraging aggregate performance metrics.
In this hypothetical, the human and the AI have the same average performance, but the AI specifically fails to recognise the critically important cases (marked in red). The human makes mistakes in less important cases, which is fairly typical in diagnostic practice.
In this setting, even though the doctors and the AI have the same overall performance (justifying regulatory approval), using the AI would lead to delayed diagnosis in the cases where such a delay is critically important. It would kill patients, and we would have no way to predict this with current testing.
Predicting where AI fails
So, how can we mitigate this risk? There are lots of clever computer scientists trying to make computers smart enough to avoid the problem (see: algorithmic robustness/fairness, causal machine learning, invariant learning etc.), but we don’t necessarily have to be this fancy^. If the problem is that performance may be worse in clinically important subsets, then all we might need to do is identify those subsets and test their performance.
In our example above, we can simply label all the “aggressive sub-type” cases in the cancer test set, and then evaluate model performance on that subset. Then our results (to report to the FDA would be):
As you might expect, these results would be treated very differently by a regulator, as this now looks like an absurdly unsafe AI system. This “stratified” testing tells us far more about the safety of this system than the overall or average performance for a medical task.
So, the low-tech solution is obvious – you identify all possible variants in the data and label them in the test set. In this way, a safe system is one that shows human-level performance in the overall task as well as in the subsets.
We call this approach schema completion. A schema (or ontology) in this context is the label structure, defining the relationships between superclasses (the large, coarse classes) and subclasses (the fine-grained subsets). We have actually seen well-formed schemas in medical AI research before, for example in the famous 2017 Nature paper Dermatologist-level classification of skin cancer with deep neural networks by Esteva et al. They produced a complex tree structure defining the class relationships, and even if this is not complete, it is certainly much better than pretending that all of the variation in skin lesions is explained by “malignant” and “not malignant” labels.

So why doesn’t everyone test on complete schema? Two reasons:
There aren’t enough test cases (in this dermatology example, they only tested on the three red super-classes). If you had to have well-powered test sets for every subtype, you would need more data than in your training set!
There are always more subclasses*. In the paper, Esteva et al describe over 2000 diagnostic categories in their dataset! Even then they didn’t include all of the important visual subsets in their schema, for example we have seen similar models fail when skin markers are present.
So testing all the subsets seems untenable. What can we do?
We think that we can rationalise the problem. If we knew what subsets are likely to be “underperformers”, and we use our medical knowledge to determine which subsets are high-risk, then we only need to test on the intersection between these two groups. We can predict the specific subsets where AI could clinically fail, and then only need to target these subsets for further analysis.
In our paper, we identified three main factors that appear to lead to underperformance. Across multiple datasets, we find evidence that hidden stratification leads to poor performance when there are subsets characterized by low subset prevalence, poor subset label quality, and/or subtle discriminative features (when the subset looks more like a different class than the class that it actually belongs to).
An example from the paper using the MURA dataset. Relabeled, we see that metalwork (left) is visually the most obvious finding (it looks the least like a normal x-ray out of the subclasses). Fractures (middle) can be subtle, and degenerative disease (right) is both subtle and inconsistently labeled. A model trained on the normal/abnormal superclasses significantly underperforms on cases within the subtle and noisy subclasses.
Putting it into practice
So we think we know how to recognise problematic subsets.
To actually operationalise this, we doctors would sit down and write out a complete schema for any and all medical AI tasks. Given the broad range of variation, covering clinical, pathological, and visual subsets, this would be a huge undertaking. Thankfully, it only needs to be done once (and updated rarely), and this is exactly the sort of work that is performed by large professional bodies (like ACR, ESR, RSNA), who regularly form working groups of domain specialists to tackle these kind of problems^^.

The nicest thing you can say about being in a working group is that someone’s gotta do it.
With these expert-defined schema, we would then highlight the subsets which may cause problems – those that are likely to underperform due to the factors we have identified in our research, and those that are high risk based on our clinical knowledge. Ideally there will be only a few “subsets of concern” per task that fulfil these criteria.
Then we present this ontology to the regulators and say “for an AI system to be judged safe for task X, we need to know the performance in the subsets of concern Y and Z.” In this way, a pneumothorax detector would need to show performance in cases without chest tubes, a fracture detector would need to be equal to humans for subtle fractures as well as obvious ones, and a “normal-case detector” (don’t get Luke started) would need to show that it doesn’t miss serious diseases.
To make this more clear, let’s consider a simple example. Here is a quick attempt at a pneumothorax schema:
Subsets of concern in red, conditional subsets of concern in orange (depends on exact implementation of model and data)
Pneumothorax is a tricky one since they are all “high risk” if they are untreated (meaning you end up with more subsets of concern than in many tasks), but we think this gives a general feel for what schema completion might look like.
The beauty of this approach is that it would work within the current regulatory framework, and as long as there aren’t too many subsets of concern the compliance cost should be low. If you already have enough cases for subset testing, then the only cost to the developer would be producing the labels, which would be relatively small.
If the subsets of concern in the existing test set are too small for valid performance results, then there is a clear path forward – you need to enrich for those subsets (i.e., not gather ten thousand more random cases). While this does carry a compliance cost, since you only need to do this for a small handful of subsets, the cost is also likely to be small compared to the overall cost of development. Sourcing the cases could get tricky if they are rare, but this is not insurmountable.
The only major cost to developers when implementing a system like this is if they find out that their algorithm is unsafe, and it needs to be retrained with specific subsets in mind. Since this is absolutely the entire point of regulation, we’d call this a reasonable cost of doing business.
In fact, since this list of subsets of concern would be widely available, developers could decide on their AI targets informed of the associated risks – if they don’t think they can adequately test for performance in a subset of concern, they can target a different medical task. This is giving developers have been asking for – they say they want more robust regulation and better assurances of safety, as long as the costs are transparent and the playing field is level.
How much would it help?
We see this “low-tech” approach to strengthen pre-clinical testing as a trade-off between being able to measure the actual clinical costs of using AI (as you would in a clinical trial) and the realities of device regulation. By identifying strata that are likely to produce worse clinical outcomes, we should be able to get closer to the safety profile delivered by gold standard clinical testing, without massively inflating costs or upending the current regulatory system.
This is certainly no panacea. There will always be subclasses and edge cases that we simply can’t test preclinically, perhaps because they aren’t recognised in our knowledge base or because examples of the strata aren’t present within our dataset. We also can’t assess the effects of the other causes of clinical underperformance, such as the laboratory effect and automation bias.
To close this safety gap, we still need to rely on post-deployment monitoring.
A promising direction for post-deployment monitoring is the AI audit, a process where human experts monitor the performance and particularly the errors of AI systems in clinical environments, in effect estimating the harm caused by AI in real-time. The need for this sort of monitoring has been recognised by professional organisations, who are grappling with the idea that we will need a new sort of specialist – a chief medical information officer who is skilled in AI monitoring and assessment – embedded in every practice (for example, see section 3 of the proposed RANZCR Standards of Practice for Artificial Intelligence).
Auditors are the real superheros
Audit works by having human experts review examples of AI predictions, and trying to piece together an explanation for the errors. This can be performed with image review alone or in combination with other interpretability techniques, but either way error auditing is critically dependent on the ability of the auditor to visually appreciate the differences in the distribution of model outputs. This approach is limited to the recognition of fairly large effects (i.e., effects that are noticeable in a modest/human-readable sample of images) and it will almost certainly be less exhaustive than prospectively assessing a complete schema defined by an expert panel. That being said, this process can still be extremely useful. In our paper, we show that human audit was able to detect hidden stratification that caused the performance of a CheXNet-reproduction model to drop by over 15% ROC-AUC on pneumothorax cases without chest drains — the subset that’s most important! — with respect to those that had already been treated with a chest drain.
Thankfully, the two testing approaches we’ve described are synergistic. Having a complete schema is useful for audit; instead of laboriously (and idiosyncratically) searching for meaning in sets of images, we can start our audit with the major subsets of concern. Discovering new and unexpected stratification would only occur when there are clusters of errors which do not conform to the existing schema, and these newly identified subsets of concern could be folded back into the schema via a reporting mechanism.
Looking to the future, we also suggest in our paper that we might be able to automate some of the audit process, or at least augment it with machine learning. We show that even simple k-means clustering in the model feature space can be effective in revealing important subsets in some tasks (but not others). We call this approach to subset discovery algorithmic measurement, and anticipate that further development of these ideas may be useful in supplementing schema completion and human audit. We have begun to explore more effective techniques for algorithmic measurement that may work better than k-means, but that is a topic for another day :).
Making AI safe(r)
These techniques alone won’t make medical AI safe, because they can’t replace all the benefits of proper clinical testing of AI. Risk-critical systems in particular need randomised control trials, and our demonstration of hidden stratification in common medical AI tasks only reinforces this point. The problem is that there is no path from here to there. It is possible that RCTs won’t even be considered until after we have a medical AI tragedy, and by then it will be too late.
In this context, we believe that pre-marketing targeted subset testing combined with post-deployment monitoring could serve as an important and effective stopgap for improving AI safety. It is low tech, achievable, and doesn’t create a huge compliance burden. It doesn’t ask the healthcare systems and governments of the world to overhaul their current processes, just to take a bit of advice on what specific questions need to be asked for any given medical task. By delivering a consensus schema to regulators on a platter, they might even use it.
And maybe this approach is more broadly attractive as well. AI is not human — inhuman, in fact — in how it makes decisions. While it is attractive to work towards human-like intelligence in our computer systems, it is impossible to predict if and when this might be feasible.
The takeaway here is that subset-based testing and monitoring is one way we can bring human knowledge and common sense into medical machine learning systems, completely separate from the mathematical guts of the models. We might even be able to make them safer without making them smarter, without teaching them to ask why, and without rebooting AI.
Luke’s footnotes:
ª The current FDA position on the clinical evaluation of medical software (pdf link) is: “…prior to product launch (pre-market) the manufacturer generates evidence of the product’s accuracy, specificity, sensitivity, reliability, limitations, and scope of use in the intended use environment with the intended user, and generates a SaMD definition statement. Once the product is on the market (post-market), as part of normal lifecycle management processes, the manufacturer continues to collect real world performance data (e.g., complaints, safety data)…”
^ I am planning to do a follow-up post on this idea – that we don’t always need to default to looking for not yet developed, possibly decades away technological solutions when the problem can be immediately solved with a bit of human effort.
^^ A possibly valid alternative would be crowd-sourcing these schema. This would have to be done very carefully to be considered authoritative enough to justify including in regulatory frameworks, but could happen much quicker than the more formal approach.
* I’ve heard this described as “subset whack-a-mole”, or my own phrasing: “there are subsets all the way down”.**
** I love that I have finally included a Terry Pratchett reference in my Pratchett-esque footnotes.
Luke Oakden-Rayner is a radiologist (medical specialist) in South Australia, undertaking a Ph.D in Medicine with the School of Public Health at the University of Adelaide.
Jared Dunnmon is a post-doctoral fellow at Stanford University where he researches the development of weakly supervised machine learning techniques and application to problems in human health, energy & environment, and national security.
This post originally appeared on Luke’s blog here.
Improving Medical AI Safety by Addressing Hidden Stratification published first on https://venabeahan.tumblr.com
0 notes
Text
Improving Medical AI Safety by Addressing Hidden Stratification
Jared Dunnmon
Luke Oakden-Rayner
By LUKE OAKDEN-RAYNER MD, JARED DUNNMON, PhD
Medical AI testing is unsafe, and that isn’t likely to change anytime soon.
No regulator is seriously considering implementing “pharmaceutical style” clinical trials for AI prior to marketing approval, and evidence strongly suggests that pre-clinical testing of medical AI systems is not enough to ensure that they are safe to use. As discussed in a previous post, factors ranging from the laboratory effect to automation bias can contribute to substantial disconnects between pre-clinical performance of AI systems and downstream medical outcomes. As a result, we urgently need mechanisms to detect and mitigate the dangers that under-tested medical AI systems may pose in the clinic.
In a recent preprint co-authored with Jared Dunnmon from Chris Ré’s group at Stanford, we offer a new explanation for the discrepancy between pre-clinical testing and downstream outcomes: hidden stratification. Before explaining what this means, we want to set the scene by saying that this effect appears to be pervasive, underappreciated, and could lead to serious patient harm even in AI systems that have been approved by regulators.
But there is an upside here as well. Looking at the failures of pre-clinical testing through the lens of hidden stratification may offer us a way to make regulation more effective, without overturning the entire system and without dramatically increasing the compliance burden on developers.
What’s in a stratum?
We recently published a pre-print titled “Hidden Stratification Causes Clinically Meaningful Failures in Machine Learning for Medical Imaging“.
Note: While this post discusses a few parts of this paper, it is more intended to explore the implications. If you want to read more about the effect and our experiments, please read the paper
The effect we describe in this work — hidden stratification — is not really a surprise to anyone. Simply put, there are subsets within any medical task that are visually and clinically distinct. Pneumonia, for instance, can be typical or atypical. A lung tumour can be solid or subsolid. Fractures can be simple or compound. Such variations within a single diagnostic category are often visually distinct on imaging, and have fundamentally different implications for patient care.

Examples of different lung nodules, ranging from solid (a), solid with a halo (b), and subsolid (c). Not only do these nodules look different, they reflect different diseases with different patient outcomes.
We also recognise purely visual variants. A pleural effusion looks different if the patient is standing up or is lying down, despite the pathology and clinical outcomes being the same.
These patients both have left sided pleural effusions (seen on the right of each image). The patient on the left has increased density at the left lung base, whereas the patient on the right has a subtle “veil” across the entire left lung.
These visual variants can cause problems for human doctors, but we recognise their presence and try to account for them. This is rarely the case for AI systems though, as we usually train AI models on coarsely defined class labels and this variation is unacknowledged in training and testing; in other words, the the stratification is hidden (the term “hidden stratification” actually has its roots in genetics, describing the unrecognised variation within populations that complicates all genomic analyses).
The main point of our paper is that these visually distinct subsets can seriously distort the decision making of AI systems, potentially leading to a major difference between performance testing results and clinical utility.
Clinical safety isn’t about average performance
The most important concept underpinning this work is that being as good as a human on average is not a strong predictor of safety. What matters far more is specifically which cases the models get wrong.
For example, even cutting-edge deep learning systems make such systematic misjudgments as consistently classifying canines in the snow as wolves or men as computer programmers and women as homemakers. This “lack of common sense” effect is often treated as an expected outcome of data-driven learning, which is undesirable but ultimately acceptable in deployed models outside of medicine (though even then, these effects have caused major problems for sophisticated technology companies).
Whatever the risk is in the non-medical world, we argue that in healthcare this same phenomenon can have serious implications.
Take for example a situation where humans and an AI system are trying to diagnose cancer, and they show equivalent performance in a head-to-head “reader” study. Let’s assume this study was performed perfectly, with a large external dataset and a primary metric that was clinically motivated (perhaps the true positive rate in a screening scenario). This is the current level of evidence required for FDA approval, even for an autonomous system.
Now, for the sake of the argument, let’s assume the TPR of both decision makers is 95%. Our results to report to the FDA probably look like this:
TPR is the same thing as sensitivity/recall
That looks good, our primary measure (assuming a decent sample size) suggests that the AI and human are performing equivalently. The FDA should be pretty happyª.
Now, let’s also assume that the majority of cancer is fairly benign and small delays in treatment are inconsequential, but that there is a rare and visually distinct cancer subtype making up 5% of all disease that is aggressive and any delay in diagnosis leads to drastically shortened life expectancy.
There is a pithy bit of advice we often give trainee doctors: when you hear hoofbeats, think horses, not zebras. This means that you shouldn’t jump to diagnosing the rare subtype, when the common disease is much more likely. This is also exactly what machine learning models do – they consider prior probability and the presence of predictive features but, unless it has been explicitly incorporated into the model, they don’t consider the cost of their errors.
This can be a real problem in medical AI, because there is a less commonly shared addendum to this advice: if zebras were stone cold killing machines, you might want to exclude zebras first. The cost of misidentifying a dangerous zebra is much more than that of missing a gentle pony. No-one wants to get hoofed to death.
In practice, human doctors will be hyper-vigilant about the high-risk subtype, even though it is rare. They will have spent a disproportionate amount of time and effort learning to identify it, and will have a low threshold for diagnosing it (in this scenario, we might assume that the cost of overdiagnosis is minimal).
If we assume the cancer-detecting AI system was developed as is common practice, it probably was trained to detect “cancer” as a monolithic group. Since only 5% of the training samples included visual features of this subtype, and no-one has incorporated the expected clinical cost of misdiagnosis into the model, how do we expect it to perform in this important subset of cases?
Fairly obviously, it won’t be hypervigilant – it was never informed that it needed to be. Even worse, given the lower number of training examples in the minority subtype, it will probably underperform for this subset (since performance on a particular class or subset should increase with more training examples from that class). We might even expect that a human would get the majority of these cases right, and that the AI might get the majority wrong. In our paper, we show that existing AI models do indeed show concerning error rates on clinically important subsets despite encouraging aggregate performance metrics.
In this hypothetical, the human and the AI have the same average performance, but the AI specifically fails to recognise the critically important cases (marked in red). The human makes mistakes in less important cases, which is fairly typical in diagnostic practice.
In this setting, even though the doctors and the AI have the same overall performance (justifying regulatory approval), using the AI would lead to delayed diagnosis in the cases where such a delay is critically important. It would kill patients, and we would have no way to predict this with current testing.
Predicting where AI fails
So, how can we mitigate this risk? There are lots of clever computer scientists trying to make computers smart enough to avoid the problem (see: algorithmic robustness/fairness, causal machine learning, invariant learning etc.), but we don’t necessarily have to be this fancy^. If the problem is that performance may be worse in clinically important subsets, then all we might need to do is identify those subsets and test their performance.
In our example above, we can simply label all the “aggressive sub-type” cases in the cancer test set, and then evaluate model performance on that subset. Then our results (to report to the FDA would be):
As you might expect, these results would be treated very differently by a regulator, as this now looks like an absurdly unsafe AI system. This “stratified” testing tells us far more about the safety of this system than the overall or average performance for a medical task.
So, the low-tech solution is obvious – you identify all possible variants in the data and label them in the test set. In this way, a safe system is one that shows human-level performance in the overall task as well as in the subsets.
We call this approach schema completion. A schema (or ontology) in this context is the label structure, defining the relationships between superclasses (the large, coarse classes) and subclasses (the fine-grained subsets). We have actually seen well-formed schemas in medical AI research before, for example in the famous 2017 Nature paper Dermatologist-level classification of skin cancer with deep neural networks by Esteva et al. They produced a complex tree structure defining the class relationships, and even if this is not complete, it is certainly much better than pretending that all of the variation in skin lesions is explained by “malignant” and “not malignant” labels.

So why doesn’t everyone test on complete schema? Two reasons:
There aren’t enough test cases (in this dermatology example, they only tested on the three red super-classes). If you had to have well-powered test sets for every subtype, you would need more data than in your training set!
There are always more subclasses*. In the paper, Esteva et al describe over 2000 diagnostic categories in their dataset! Even then they didn’t include all of the important visual subsets in their schema, for example we have seen similar models fail when skin markers are present.
So testing all the subsets seems untenable. What can we do?
We think that we can rationalise the problem. If we knew what subsets are likely to be “underperformers”, and we use our medical knowledge to determine which subsets are high-risk, then we only need to test on the intersection between these two groups. We can predict the specific subsets where AI could clinically fail, and then only need to target these subsets for further analysis.
In our paper, we identified three main factors that appear to lead to underperformance. Across multiple datasets, we find evidence that hidden stratification leads to poor performance when there are subsets characterized by low subset prevalence, poor subset label quality, and/or subtle discriminative features (when the subset looks more like a different class than the class that it actually belongs to).
An example from the paper using the MURA dataset. Relabeled, we see that metalwork (left) is visually the most obvious finding (it looks the least like a normal x-ray out of the subclasses). Fractures (middle) can be subtle, and degenerative disease (right) is both subtle and inconsistently labeled. A model trained on the normal/abnormal superclasses significantly underperforms on cases within the subtle and noisy subclasses.
Putting it into practice
So we think we know how to recognise problematic subsets.
To actually operationalise this, we doctors would sit down and write out a complete schema for any and all medical AI tasks. Given the broad range of variation, covering clinical, pathological, and visual subsets, this would be a huge undertaking. Thankfully, it only needs to be done once (and updated rarely), and this is exactly the sort of work that is performed by large professional bodies (like ACR, ESR, RSNA), who regularly form working groups of domain specialists to tackle these kind of problems^^.

The nicest thing you can say about being in a working group is that someone’s gotta do it.
With these expert-defined schema, we would then highlight the subsets which may cause problems – those that are likely to underperform due to the factors we have identified in our research, and those that are high risk based on our clinical knowledge. Ideally there will be only a few “subsets of concern” per task that fulfil these criteria.
Then we present this ontology to the regulators and say “for an AI system to be judged safe for task X, we need to know the performance in the subsets of concern Y and Z.” In this way, a pneumothorax detector would need to show performance in cases without chest tubes, a fracture detector would need to be equal to humans for subtle fractures as well as obvious ones, and a “normal-case detector” (don’t get Luke started) would need to show that it doesn’t miss serious diseases.
To make this more clear, let’s consider a simple example. Here is a quick attempt at a pneumothorax schema:
Subsets of concern in red, conditional subsets of concern in orange (depends on exact implementation of model and data)
Pneumothorax is a tricky one since they are all “high risk” if they are untreated (meaning you end up with more subsets of concern than in many tasks), but we think this gives a general feel for what schema completion might look like.
The beauty of this approach is that it would work within the current regulatory framework, and as long as there aren’t too many subsets of concern the compliance cost should be low. If you already have enough cases for subset testing, then the only cost to the developer would be producing the labels, which would be relatively small.
If the subsets of concern in the existing test set are too small for valid performance results, then there is a clear path forward – you need to enrich for those subsets (i.e., not gather ten thousand more random cases). While this does carry a compliance cost, since you only need to do this for a small handful of subsets, the cost is also likely to be small compared to the overall cost of development. Sourcing the cases could get tricky if they are rare, but this is not insurmountable.
The only major cost to developers when implementing a system like this is if they find out that their algorithm is unsafe, and it needs to be retrained with specific subsets in mind. Since this is absolutely the entire point of regulation, we’d call this a reasonable cost of doing business.
In fact, since this list of subsets of concern would be widely available, developers could decide on their AI targets informed of the associated risks – if they don’t think they can adequately test for performance in a subset of concern, they can target a different medical task. This is giving developers have been asking for – they say they want more robust regulation and better assurances of safety, as long as the costs are transparent and the playing field is level.
How much would it help?
We see this “low-tech” approach to strengthen pre-clinical testing as a trade-off between being able to measure the actual clinical costs of using AI (as you would in a clinical trial) and the realities of device regulation. By identifying strata that are likely to produce worse clinical outcomes, we should be able to get closer to the safety profile delivered by gold standard clinical testing, without massively inflating costs or upending the current regulatory system.
This is certainly no panacea. There will always be subclasses and edge cases that we simply can’t test preclinically, perhaps because they aren’t recognised in our knowledge base or because examples of the strata aren’t present within our dataset. We also can’t assess the effects of the other causes of clinical underperformance, such as the laboratory effect and automation bias.
To close this safety gap, we still need to rely on post-deployment monitoring.
A promising direction for post-deployment monitoring is the AI audit, a process where human experts monitor the performance and particularly the errors of AI systems in clinical environments, in effect estimating the harm caused by AI in real-time. The need for this sort of monitoring has been recognised by professional organisations, who are grappling with the idea that we will need a new sort of specialist – a chief medical information officer who is skilled in AI monitoring and assessment – embedded in every practice (for example, see section 3 of the proposed RANZCR Standards of Practice for Artificial Intelligence).
Auditors are the real superheros
Audit works by having human experts review examples of AI predictions, and trying to piece together an explanation for the errors. This can be performed with image review alone or in combination with other interpretability techniques, but either way error auditing is critically dependent on the ability of the auditor to visually appreciate the differences in the distribution of model outputs. This approach is limited to the recognition of fairly large effects (i.e., effects that are noticeable in a modest/human-readable sample of images) and it will almost certainly be less exhaustive than prospectively assessing a complete schema defined by an expert panel. That being said, this process can still be extremely useful. In our paper, we show that human audit was able to detect hidden stratification that caused the performance of a CheXNet-reproduction model to drop by over 15% ROC-AUC on pneumothorax cases without chest drains — the subset that’s most important! — with respect to those that had already been treated with a chest drain.
Thankfully, the two testing approaches we’ve described are synergistic. Having a complete schema is useful for audit; instead of laboriously (and idiosyncratically) searching for meaning in sets of images, we can start our audit with the major subsets of concern. Discovering new and unexpected stratification would only occur when there are clusters of errors which do not conform to the existing schema, and these newly identified subsets of concern could be folded back into the schema via a reporting mechanism.
Looking to the future, we also suggest in our paper that we might be able to automate some of the audit process, or at least augment it with machine learning. We show that even simple k-means clustering in the model feature space can be effective in revealing important subsets in some tasks (but not others). We call this approach to subset discovery algorithmic measurement, and anticipate that further development of these ideas may be useful in supplementing schema completion and human audit. We have begun to explore more effective techniques for algorithmic measurement that may work better than k-means, but that is a topic for another day :).
Making AI safe(r)
These techniques alone won’t make medical AI safe, because they can’t replace all the benefits of proper clinical testing of AI. Risk-critical systems in particular need randomised control trials, and our demonstration of hidden stratification in common medical AI tasks only reinforces this point. The problem is that there is no path from here to there. It is possible that RCTs won’t even be considered until after we have a medical AI tragedy, and by then it will be too late.
In this context, we believe that pre-marketing targeted subset testing combined with post-deployment monitoring could serve as an important and effective stopgap for improving AI safety. It is low tech, achievable, and doesn’t create a huge compliance burden. It doesn’t ask the healthcare systems and governments of the world to overhaul their current processes, just to take a bit of advice on what specific questions need to be asked for any given medical task. By delivering a consensus schema to regulators on a platter, they might even use it.
And maybe this approach is more broadly attractive as well. AI is not human — inhuman, in fact — in how it makes decisions. While it is attractive to work towards human-like intelligence in our computer systems, it is impossible to predict if and when this might be feasible.
The takeaway here is that subset-based testing and monitoring is one way we can bring human knowledge and common sense into medical machine learning systems, completely separate from the mathematical guts of the models. We might even be able to make them safer without making them smarter, without teaching them to ask why, and without rebooting AI.
Luke’s footnotes:
ª The current FDA position on the clinical evaluation of medical software (pdf link) is: “…prior to product launch (pre-market) the manufacturer generates evidence of the product’s accuracy, specificity, sensitivity, reliability, limitations, and scope of use in the intended use environment with the intended user, and generates a SaMD definition statement. Once the product is on the market (post-market), as part of normal lifecycle management processes, the manufacturer continues to collect real world performance data (e.g., complaints, safety data)…”
^ I am planning to do a follow-up post on this idea – that we don’t always need to default to looking for not yet developed, possibly decades away technological solutions when the problem can be immediately solved with a bit of human effort.
^^ A possibly valid alternative would be crowd-sourcing these schema. This would have to be done very carefully to be considered authoritative enough to justify including in regulatory frameworks, but could happen much quicker than the more formal approach.
* I’ve heard this described as “subset whack-a-mole”, or my own phrasing: “there are subsets all the way down”.**
** I love that I have finally included a Terry Pratchett reference in my Pratchett-esque footnotes.
Luke Oakden-Rayner is a radiologist (medical specialist) in South Australia, undertaking a Ph.D in Medicine with the School of Public Health at the University of Adelaide.
Jared Dunnmon is a post-doctoral fellow at Stanford University where he researches the development of weakly supervised machine learning techniques and application to problems in human health, energy & environment, and national security.
This post originally appeared on Luke’s blog here.
Improving Medical AI Safety by Addressing Hidden Stratification published first on https://wittooth.tumblr.com/
0 notes
Text
RPG Lifestyle AI, by Sandeep S. Poplar (AKA me)
When I say I don't experience emotions, that's not the whole story. When I say that I'm experimenting on people, its a little out of context. Hopefully this analogy will better explain what I mean, while also explaining most of my life story. Imagine that Life is a semi-multiplayer, turn based RPG, and emotions are like status effects, or buffs. For most gamers, its natural and easy to pick up such a game and take to it, I.E. live life. But one guy who's really into evolving AI systems comes along, and makes an AI evolver meant to play the game. Hi, I'm the AI. When evolving an AI, you usually need to give it training data to get it started going, but this guy figured out a way to get around that, and his AI has to create its own Training Data as it goes. So, if it experiences something for the first time, its most likely outcome is failure until its encountered it a lot and tried several methods. As expected, it is very slow going for a long time. The AI has to learn How to move around in the field successfully, but every time in the middle of its evolutionary progress, it gets in a battle with an enemy, and then it has to learn what battle even is before starting to formulate a response. After trying some early stage response strategies, however, the battle ends because the enemies are such early levels, so the AI can't experiment any further, and losses that train of thought almost entirely. Back into the field its thrown, where it just fafs around for awhile, hardly even starting the story progression. Eventually, it learns the battle/field systems well enough that it doesn't die anymore, and it figures out that there is progression to be made. Its so overleveled by this point from being trapped in this area, that for awhile, it won't need to learn any of the actual unique gameplay mechanics for a good chunk of the early game. This will come back to haunt it. So it starts making story progress finally, but by this point in the playtime, most casual players are 1/4 of the way through the game. AI is 1/10. It is very behind its peers, and may have some issues later on in the multiplayer sections. Since story progression has been discovered as the incentive goal, and AI is so overleveled that fights end in the field before they can even start, it breezes quickly through some more chunk of game. It doesn't seek battles out though, it just completes them. The concept of grinding to improve is still foreign, and what its actually learnt prevents it from grinding yet. Let's pause the evolution momentarily to discuss why. Early, all AI knew was field and fights, both of which were still meaningless to it. When it discovered story progression as incentive, it came to the conclusion that staying in this area for such a long time battling had hindered its successfulness. This wasn't contradicted at any time soon, since it was so overleveled, so the idea of going back one area to defeat enemies for quick xp wouldn't be considered as an option anytime soon. Let's continue the evolution now. So, as expected, AI starts slowing down when it reaches some higher tiered enemies. It doesn't know things like magic, or defending, or health balancing, any of it. According to what its learned, Stabbing an enemy once should kill it, and if it happens to be faster than you and you take damage, so what, a cutscene will heal you soon enough. But that's not how battles work anymore, and this confuses AI to no end. It enters into another session of training data collection, and after lots more trial and error, it finds that it has multiple options in battle. It learns all the extra attack options quickly enough, including how to deal with things like spiked or flying enemies, but its a bit slower on defending. There being several types of unique enemies by this point in the game, they each have different attack patterns that don't match each other. This is good game design and how a game should work properly, but AI doesn't know that. Its first attempts of things like wait x# seconds then defend All fail, because the defense timing is different from enemy to enemy. AI has to learn this before he can continue the game. After learning about unique fight mechanics and about grinding, AI is pretty proud of itself. But then, there are field puzzles. Crap. So AI has to figure out that there even is a puzzle, and what that means. Then, it has to start going through iterations of puzzle solutions. On top of that, there may be some unique field abilities that it never used before, so it has to find those now too. We're gonna be here awhile... AI is an excellent fighter, understands grinding, wants to progress the story, and is great at puzzles! Is there nothing it can't achieve? “ATK^!” “DEFv!” “SPDX2!” “Poisoned!” “Asleep!” “Fever!” “Other Generic Status Effects!” Dafuq is that? Dafuq is this? Dafuq’re those? AI hits a wall. It has just reached the Multiplayer portion of the game, where using things like status effects to your advantage is a common, viable strategy. But to AI, its a completely foreign concept, with no pseudo-tutorial learning curve available for it to train off of. This is a hopeless endeavor for the AI. Project abandoned. Weeks later, someone realized that AI and its game had been left running in the background. Upon checking up on AI, it is discovered that it is very busy on a project all its own! AI couldn't understand status effects, so it made a separate line of coding structure designed specifically to help with that. If you were to attempt to look at status effects the way AI does, it would seen more like a GUI to you than a buff or condition set, but somehow AI is once again wrecking in battles! This is not to say that AI has hacked the game so that it can now turn effects on and off at will, though it would certainly have you believe that. Rather, its just that AI has to look at them a different way than is usually allowed in the games code. It had to learn what a condition is from scratch, so the first time AI encounters a new one, it goes to the games sandbox mode and plays around with all the things the condition or buff has to offer. It has to test run the status before it dares apply it in battle. AI now ranks among the top players in the world, but it still has to continue its methods of play. Taking on new strategies is a slow, distinct, and decisive process for it, and to teach a strategy to AI, you have to reverse engineer it and battle AI until AI uses the strategy itself. It may be a process, but once AI learns it, it can go toe to toe with the best. In summary, I do experience emotion, but I don't understand the meaning of the experience. So I take the emotion in practice and wield it rather than experience it, until after gauging the reactions of others, I am able to understand what a specific emotion is used to indicate.
#intp#psychology#mental reflection#nightpost#I wrote this all in under an hour#sandeep s poplar#evolving ai#evolution#ai#RPG#emotions#lifestory
1 note
·
View note
Text
Deep Learning
It is no longer interesting that a computer can beat humans at chess, or Atari’s Breakout, for that matter. I’m nonplussed about whether Siri’s voice has been sounding smoother lately, or whether my phone reminds me about where I am parked. Our attention may be currently focused on teaching computers to filter out hate-fuelled images from YouTube, or to avoid kangaroos on the road, but I expect these interests will give way to new ones very shortly in the daily churn of the news cycle.
Deep learning is everywhere and nowhere in our thinking. It flickers into view, only to recede again as our expectations about it get bigger. That’s a shame because the news grabs on deep learning do not challenge us to think about it as being about more than technology. If we give more thought to it, deep learning promises to shake loose some of the persistent assumptions we make about deep human learning.
We think about computer deep learning and human deep learning in quite different ways, and those differences are liberating for computers and constraining for humans.
A computer learns deeply when it iterates and refines outputs. In simpler models of deep learning, an artificial neural network—an algorithm or hardware which consists of layers—is given an input that leads to an output in one layer, which becomes an input for another layer, and so on. Deep learning involves multiple successive layers. In supervised deep learning, the computer is told what the correct output is. In unsupervised deep learning, that correction is done within the network itself. That training is repeated over and again until the margin of error of outputs becomes extremely low.
Deep learning will mean the more accurate detection of cancer from medical images in future. But don’t forget that deep learning also taught IBM’s Watson to swear—it learned the contents of the online Urban dictionary—and Microsoft’s Tay to deny the Holocaust. Our computers are ourselves, or are they?
Deep learning by computers is pretty dumb by human standards, and has only really become a possibility in the last decade as faster processing speeds have become possible and sources such as the web and satellite images have provided the data to support networks with thousands of layers, most of which are unsupervised. Google’s X lab, for example, is now using thousands of layers in an artificial neural network to detect kitten faces, largely unsupervised, with just under 16% accuracy. But it is getting better and better, and the pinch on jobs that is beginning to unfold has been noted by commentators repeatedly.
But the evolving discussion on deep learning for computers and implications for the economy is leaving understandings of deep human learning behind.
Deep human learning is bound by largely unwritten rules on how we talk with others and show them respect. It also demands an attitude, a particular kind of motivation.
In 1976, Marten and Säljö used a reading comprehension test to distinguish between what they called deep and surface learners. Deep learners, like their computer cousins, iterate and refine ideas and understandings. They relate new material to things they have already learned, and use new material to suggest new answers. But in distinction from computers, human deep learners are assumed to be intrinsically motivated—they enjoy learning for its own sake—and are not driven purely by ends such as grades and external validation.
Ever since that study, higher education research has generated clusters of related successor studies, many of which convey the idea that deep learning is not only about intrinsic motivation; it is also about concepts like self-efficacy. Humans face a metacognitive demand not placed upon computers: we have to think about learning in the right way in order to be able to learn in the right way.
This all sounds a bit abstract until you realise that the Course Experience Questionnaire (CEQ, 1992), and its successors the University Experience Survey (2011) and the Student Experience Survey (2015–) were designed to focus on aspects of teaching that are thought to be associated with deep learning. So too with its international cousins, the NSS in the UK and the NSSE in the USA. It is remarkable just how pervasive the ideas of deep and surface learning.
Ironically, those questionnaires see students studying computer science and engineering persistently placed at the bottom of comparative satisfaction ratings. The creators of deep learning are surface learners by this measure.
The important question for us all is whether we should insist on human learners holding a learning attitude, as well as learning. Sadly, there has been little movement on this question since 1991. In the same period of time, the internet has been invented, Watson has learned to swear and to unswear, and Google is now identifying kitten videos on YouTube with 16% accuracy. I expect that number to keep creeping up against a stalled sense of learning.
Why, with all this change, are we still asking largely the same retrospective questions about students’ senses of learning in sector evaluations? Isn’t it about time we reported on learning, rather than just reports on learning? And could our Student Experience Survey acknowledge a world in which students might think quite differently about learning in the digital world than the people who teach them? Do the questions we ask actually unearth those differences and truly prompt us to think about change?
The troubled learner engagement scale is where we are seeing the edge of these issues play out. Student agreement with the items on this scale is the lowest across all of the scales, and has hardly moved since 2015. That there is a negative association between online study and learner engagement has been acknowledged, but at this stage the input of online students has been excluded from reporting. The results are either a damning indictment of online learning, or more likely, the survey is too campus centric and not really capturing the digital lives of students.
I am not arguing for humans to be the same as computers, or to be like them, or to be replaced by them. I simply think we need to shake loose our understanding of learning a little, and to do so at more than glacial pace. That would begin with a moratorium on attitude, and the collection of data over the course of a student’s studies which show them as iterating and changing. I’d then like students to see that data before we ask them to reflect on their learning experience. Individual universities are already doing this in part, but their efforts are not supported by a concomitant shift in sector measurement of learning success. Time to iterate, folks.
This blog’s shout out is for Ann Nicholson, an inspiration in AI and Bayesian networks.
1 note
·
View note
Text
(I am so sorry, mobile users. This is really long.)
My Mass Effect Andromeda thoughts:
1. I was gonna stream the trial, but proceeded to use almost all 10 hours at once because I couldn’t stop playing. I suppose this is a good thing. I’m definitely streaming it once it’s actually out.
2. I hate the character customization. Mass Effect has always been ugly as fuck when it comes to making characters, but my dudes it is 2017 what is going on here.
2a. Side note but I laughed for like 15 minutes that there is only one “White People” face and it is honestly the ugliest thing. Cool feature (sorta not but I’m viewing it as a positive) is that there are designated skin tones with each face set. Speaking of sets, all facial features are stuck to a specific preset face. You can slightly move them, but there’s no changing. I’m hoping this is just for the trial, as other things in the game were locked off until it’s official release.
2b. so many pony tails. no undercut. despite reports saying that hairstyles would be less militaristic as you’re not a soldier, they’re more or less the same. let me be the woman i want to be dammit. There were braids, but only one style. Still double the representation compared to previously I guess? I have very much so white people hair so I don’t feel comfortable having an opinion on that subject. I will say that the braids are exclusive to fem!Ryder and m!Ryder gets 2 different textured styles. I, personally, cannot wait for the beautiful mod community to fix this hair travesty, both with representation variation and all these fucking ponytails. Maybe they can make something happen with the faces, but I hold little hope. They had “alt” hair colors, so it’s already way better than ME Original Trilogy. My Ryder has blue hair, because of course she does. There’s not much shade difference in the colors available, and some of the unnatural colors were, in fact, so unnatural looking that it was hard to accept as a hair color. dyed hair doesn’t reflect light the way it did in game and it didn’t look like much shade variation between the strands so it occasionally looked like the hair hadn’t actually finished rendering. The color selection suggested a more soft ombre look than was actually present.
3. I like that you can customize your twin also, but limits on the CC still drives me crazy. Male hair diversity isn’t super, like I said before, but it just felt like more than the female counterpart. I just really, really hate ponytails you guys.
3a. In your CC options, you can pick story bits. The only options that connect to the previous games is a selection between your Shepard having been male or female. I suppose that’s so pronouns are correct later on.
4. Prologue: I feel it takes too long, the tutorial is honestly not that great. SAM, your AI, is down for most of it, so you have no idea what anything is. It was fine at first, adding to the worldbuilding and urgency and whatnot but it got irritating by the 30th “unknown” enemy.
5. The Omni-Scanner is a neat addition, but it felt sort of...forced at times. More on that later.
6. The prologue story is okay. The ending of it, and the beginning of the actual game, was actually pretty dramatic and I didn’t expect it given the hype around certain characters that Bioware has tried to generate.
6a. Dad Ryder seemed really one dimensional with his kid. Like, never referred to them affectionately even at the last bit. This is sort of explained when you go to his room later, but it felt really hollow to me as a whole. Cool dad fact: CC of your Ryder and their twin decides what Dad looks like. Mine had obscenely blue eyes but grey hair.
6b. Evil dude looked really sad during his introduction and I wanted to be friends with him. This feels like a failed attempt at showing off the ominous silent bad guy, as I immediately started rooting for him. You go, evil dude, touch the stuff and let your dreams be true.
7. I hate the weapon interface. Inventory functions like ME1, allowing you to see the items you’ve picked up (both upgrades and actual weapons) but you cannot equip them. I couldn’t until the first mission after getting my ship. Which is terrible, as I got a sniper rifle I wanted to use and couldn’t for the prologue portion.
8. The Hyperion’s travel system is awful. There’s very little instruction about it. The tram looks as if it’s a one way thing, from the ark to the new citadel-like port, but in actuality you use it to travel around the ark itself too. Didn’t notice until my camera turned slightly to the right and another thing on the board was selectable.
8a. Not travel related, but you do get more info about the ending of the prologue and a new ongoing mission on the Hyperion. It felt like a bit of a slap. It’s all “Here’s this cool new power and a friend BUT ALSO FUCK YOU JON SNOW YOU KNOW NOTHING and you’ll never find out until you go look for these things randomly around. But not around here! Fuck you twice!” It was clearly created to push the story more later on, which is all fine and good, it just ticked me off at this moment.
9. The new Citadel is a goddamn mess. I’m not a huge fan of it right now, though what I’m 100% sure will happen is that as you make more homesteads, the place gets nicer until you’re at endgame and have a fully functional hub. I’ll like it more once it starts changing. It looks like it has really good potential. I hope it functions more than the keep in DA:I, and your choices really DO have an effect on what is opened up and how the society there builds itself.
9a. The Original Trilogy made each race very distinct, with their own speech patterns and everything. I didn’t really get that from this game’s other races. The Salarians didn’t speak in fast bursts with lots of words jammed together, and the Turians more often than not didn’t have that robotic twinge to their speech, and weren’t all that hostile. It seems unlikely to me that there wouldn’t be any left over anger as they left for Andromeda seeing as it’s possible some actually fought in the first contact war. It is about 30 years apart. It was something constantly prevalent in the previous trilogy, which every NPC lived during (at least ME1)
9b. I do, however, love super not Krogan Krogan lady. She’s perfect and I wish I could romance her. You do talk about the genophage. Sucks that she and her clan have no idea that there’s been a cure for over 500 years now.
10. The ship, Tempest, is really nice. I always felt like Normandy was very irritating to navigate around. ME1 especially, but 3 wasn’t so hot either. This one isn’t as large, but it has a really nice flow that I liked. Pathfinder quarters were way better than Shepard’s.
10a. It has a system like the Dragon Age: Inquisition war table where you have timed missions that NPC complete for materials, items, and intel. Seems interesting, but I didn’t see one to completion. They’re still running.
10b. the R&D table is interesting, and I like the separation between the two, but it didn’t feel like a huge asset so early in the game.
11. The traveling system is beautiful. Visually it gets 100% approval. However, it’s extremely slow paced. any selection of a new planet or system takes you back to where you were originally, lets you stare at it a moment, then flies you to the next place where you zoom in for another moment before zooming out and then FINALLY getting information about it. It’s nice, but by the 12th time I was incredibly tired of it.
12. Your Salarian pilot is cool. Not especially Salarian-like, but still I liked him. Cannot kiss. I tried.
13. Material gathering is kind of limited. You scan a whole system, and you have the option to scan planets, but there’s not much point to it as SAM tells you if there’s something worth scanning there. Usually it’s a single deposit of a mineral.
14. I hated the MAKO in ME1, but this one isn’t so bad. I think it helps knowing that I can customize it later.
15. Speaking of customization, you can change the colors of your casual clothes and your armor. It’s the same color selection tool as in CC, so it’s awful. The dial to change the color overlaps with the bubble to select the actual shade so there’s a lot of trial and error involved. Once again, no indication that [SPACE] is necessary to confirm your color choices. I hate the whole design of it.
16. You do meet some companions that you’ll pick up, but you barely interact with them. Good intros though. Really gave them personality right off the bat.
17. ROMANCE: Being fem!Ryder is rough at the start.
17a. Gil is one of the ship’s crew. He’s one of the few genuinely attractive males in all of Mass Effect’s history. As a woman, you can flirt with him, but he turns you down solidly. He’s kind, but firm. He states that he’s interested in men. Which is awesome, because now I have a reason to play a male Ryder after my first play through is done. Female Ryder apologizes, nothing is weird (unlike other interactions) and it actually made me like him more as a character.
17b. Liam kind of blows off your advances but it definitely felt like a rejection. As he wasn’t very clear, I don’t know if he’s a bi character that you have to develop a friendship with first, or if he’s gay and just doesn’t want to come out to your Ryder. I didn’t like the wishy-washiness of the interaction but we’ll just have to see what’s what when the full game is out.
17c. Doc. I forgot her name, so now she’s Doc. I knew this interaction wouldn’t go well, as I’ve read articles about it. She definitely turns you down because you’re a patient. I’ve read that she has a crush on the Krogan that joins you, so is he not a patient too? Either way, she’s very professional about it and as with Gil it made me appreciate her character. Knowing that it’s Natalie Dormer and I’ll never hear her tell me she loves me hurts me deep in my soul though. Why does the world hate me like this???
17d. Blonde biotic woman with the goddamn hair that I want on my Ryder. Cora. I don’t like her. You have the option to hit on her early on, and her reaction felt really awful to me. She gets kind of hostile and all “I already told [person you never met] that I’m not interested in women and I’m telling you too.” Like, ok. Damn. You aren’t my type anyways. I just wanted to see the option play out. 0/10 poor way to handle the interaction. I’m not super fond of the Asari commando thing either. Jack was a kickass biotic too and she was treated like a monster. This woman gets to take part in something very culturally specific like it’s nbd? jnasdlfknasdivhbna, not a fan of her. She looks somewhere between confused and murderous all the time. Also, she walks like Stretch Armstrong. It makes me laugh.
17e. Vetra. The only individual that actually reacts positively to fem!Ryder flirting with her. Even then she really only takes it like a compliment. But, as I love Vetra and much like Garrus I would die for her from first glance, I’ll take it. I think it’ll be a beautiful relationship. She’s also really tall. And pretty. One thing I thought was strange with her is that it always looks like she’s posing when she’s just standing around. One hip is thrust out and her arms are crossed. If I didn’t know any better, I’d think they rigged her to always be in mysterious seductress pose.
17f. I couldn’t flirt with the pilot. Let me kiss the Salarian, damn you Bioware. Also, our nice Scottish friend Suvi can’t be flirted with, but she sounds really soothing to talk to. I’m def a fan of all these non-American, thicker than previously heard, accents on the ship. The Original Trilogy was full of light British accents or full on American. Sort of hard to believe the Alliance was multinational when everyone spoke like they were from the US.
18. Combat: I mostly use the sniper rifle and the pistol. Pistol was nice. I love the sniper rifle in this game. Other ME games it was hard for me to confirm headshots but this one was a clean and clear animation. Very nice. The companion AI was strange at times, as they’d just use their abilities but in odd places so the skills would get stuck in corners or just go off to nowhere. There was combat stutter on the first planet you can visit but I think that’s more my graphics card. The update refuses to finish so I’m stuck 2 updates behind where I should be.
I have, like, an hour I think left so I’m gonna try to rush through a male Ryder play and see how companion reactions differ. I’m really only in this for the romance, you know.
9 notes
·
View notes
Text
The Archon’s Review of King Arthur: A Roleplaying Wargame
King Arthur: A Rolplaying Wargame is a grand strategy game designed by NeocoreGames and published by Paradox Interactive. It is a dark time in Britannia. The king, Uther Pendragon, has died, leaving no apparent heir. In the wake of this power vacuum, all the petty kings of the realm have taken up arms against their neighbors in a bid for power and land. Then, Merlin appears with the sword, Excalibur, lodged in a stone in the abbey at Glastonbury. He says his piece, and then Arthur appears out of nowhere to do his thing. Except, when Arthur extricates the sword from the stone, magic returns to Britannia, Merlin disappears, and the Sidhe, the ancient fae, assert themselves, carving out a territory in the Bedegraine forest, just south of Hadrain’s Wall. Britannia is now on the brink of grandiose conflict, and you, as Arthur, the Once and Future King, must decide its fate.

I picked up KAaRPWG mostly on a whim, but I must say that, for the most part, it paid off.There is a lot to like here; although my expectations were not all that high, I was pleasantly surprised.
Let’s start with the lore. If you’re a purist when it comes to Arthurian legends, you may be a bit disappointed. KAaRPWG melds Arthurian legends with a healthy does of Celtic and Irish mythology. You’ve got the sword in the stone (Excalibur in this version, not Caliburn), the Green Knight, all the Knights of the Round Table, the Holy Grail, etc. You’ve also got the Sidhe, the distinction between Seelie and Unseelie fae, the Old Faith of the druids, and a belief in magic, all of which is inspired by old Celtic and Irish myths. The two mythoi actually blend quite well; this is probably the result of a singular aesthetic acting as a very effective backdrop for both sets of myths. There’s a sense here of a blending of time and space, wherein armored knights on horseback seem natural next to the mystical and strange Sidhe. References to the ancient Roman colonies that used to be on Britannia help complete the blend, creating a sense of a far distant past brought temporally forward to scrunch it up against the medieval knights and kingdoms of Britannia. But the Roman stuff works because the ancient, “Old Faith” aesthetic helps place us there temporally. Basically, what I’m trying, and probably failing to say, is that each element of the aesthetic and lore helps hold the others up so that when blended, they fit together perfectly.
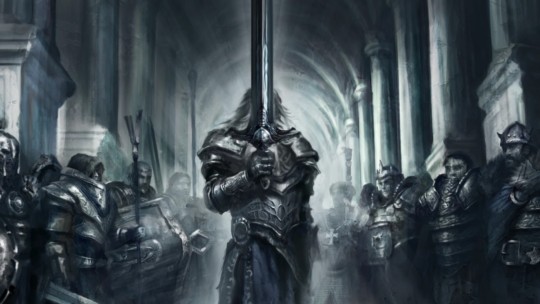
^(The Once and Future King, just before everything goes downhill)^
The overworld map is very pretty, although a bit monotone. As we are in Britannia, we can expect mostly forests and grass lands, with rolling hills and a mountain here and there. I had a similar complaint about Eador. Genesis, but this game breaks up the monotony with a quartet of seasons, which pass one by one each turn. I really like the seasons system, and not merely because it adds snow during winter. See, each season actually does something. It’s not just cosmetic, and it’s details like this that really makes me appreciate a game. Dominions 4 and Endless Legend do something similar, but it’s not quite as strategic as it is in this. The year begins in Spring, which is when random quests and disasters appear on the campaign map, which you can then react to by sending your armies to deal with them. During Summer, armies are able to move much farther on the campaign map. The game says that Autumn is when your food comes in, but I don’t think that’s actually the case. Winter does a number of things. First, Winter forces all armies on the overworld to stop and set up camp. No armies can move during Winter. Second, Winter is when your taxes come in, and this is also when your food comes in, possibly because of a bug. Lastly, Winter is when you can interact with your stronghold(s), building new districts, researching new improvements for your kingdom, and managing your economy via the Chancellory, where you enact new laws, set decrees, and trade food for gold and vice versa. Then Spring rolls back around and new random quests appear. The seasons system is a really great way of marrying form and function, and I think it’s pretty neato.
Now, this is a strategy game, and strategy games tend to have playable battles where you can exercise that big ol’ brain of yours. And the combat in this game, well... it’s basically Total War. NOW THIS SENTENCE RIGHT HERE IS FOR ANY LAWYERS THAT HAPPEN TO BE READING; DO NOT TAKE THIS PARAGRAPH OR THIS REVIEW AND USE IT AS A MEANS FOR LITIGATION. I WILL BE VERY CROSS WITH YOU IF I HAPPEN TO FIND OUT THAT ANYONE WAS SUCCESSFULLY SUED BECAUSE OF WHAT I JUST WROTE HERE. LAWYERS, DO NOT USE OR MENTION THIS REVIEW. Right, now that that’s over with; yeah, the gameplay is basically magical Medieval:Total War. You take battalions of troops, march them around the field of battle, and use strategy and tactics to win. Hero characters, such as the Knights of the Round Table, have magical abilities you can call upon to turn the tide of battle, which is a neat addition. Also, individual units don’t have morale, unlike in a Total War game; instead, each side has a morale bar that increases or decreases depending on which side controls victory locations. These locations are things like monuments, stone circles, villages, keeps, ect. Another departure from Total War that I like is that once you’ve won a battle, the enemy army goes away entirely, even if you won via morale rather than extermination. This makes it so that you needn’t chase enemies down across the map after each battle like roaches in a kitchen

^(The first battle in the game. My troops are the ones in armor. Winning!)^
In between battles are quests. As mentioned, some are random, appearing during the spring season. The important ones, however, are related to the plot of the game. These are things like finding and hiring on a Knight of the Round Table, or determining whether the Old Faith or Christianity gains more power, or finding special artifacts. All quests, random and plot-relevant, are carried out via text-based decision trees. Some choices use one or more of a hero’s stats. In these cases, the text will be green if it’s a certain success, blue if the outcome is uncertain, or red if it’s a certain failure. This incentivizes you to have a variety of heroes on hand. It’s a bit of a problem if you need a mageknight and all you have are fightknights. The outcome of quests has various effects, such as gaining you artifacts, changing your morality, giving you more troops, or provoking a battle.
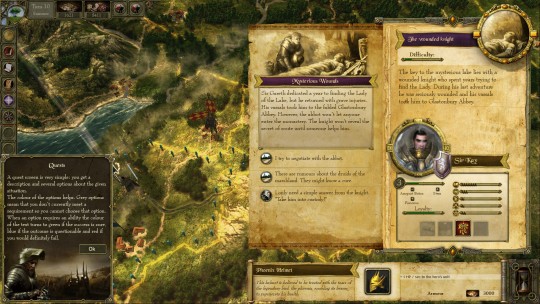
^(One of the early quests. Sir Kay here did a bang-up job of it.)^
I alluded to a morality system in the above paragraph, and this game has not a binary moral choice system, but a quaternary moral choice system. See, in the wake of Arthur pulling Excalibur from the stone, magic returns to Britannia, leading to a resurgence of what they call the “Old Faith”, which is worship of the Tuatha de Danaan, the old Irish deities. This causes friction between the believers in this Old Faith, and the followers of the still-new Christianity. Interesting sidenote: In the game, the Welsh are followers of the Old Faith, while the Saxons are the Christian invaders. But in real life, the Welsh had already been Christianized by Saint Joseph of Arimathea and the Irish by Saint Patrick, while the Saxons were so incredibly pagan that Charlemagne felt the need to deliver them the Cross via the sword. In addition to the religious conflict, there’s a virtue axis, each end of which is labeled “Rightful” and “Tyrant”. The game makes a point of stating that Rightful isn’t necessarily good and Tyrant isn’t necessarily evil, and that the axis is meant to measure your commitment to the ideals of chivalry. Although, in practice, benevolent acts increase your Rightful gauge, while malevolent acts do the opposite. Going toward one combination of religion and virtue is advised, as you get new spells, bonuses, and unit choices for doing so, and I also imagine it affects what ending you get.

^(The morality chart. Fun fact, the terms the game uses for Rightful-Old Faith and Tyrant-Old Faith are Seelie and Unseelie respectively. This means that from a religious standpoint, there’s actually three sides you can choose from, Christian, Seelie, and Unseelie. I guess Christianity doesn’t care whether you’re a moral person...?)^
Now, the things I talked about are all very effective and fun, and I like them a lot. But here comes the problems are there are a few. First off, the game is hilariously unstable, especially the further you are into it. The most common bug I found was the game giving me a “Runtime error” during loading screens and crashing to desktop. Sometimes the game even just cuts out after the loading screen, but just after I unpause the game to start a battle, dumping me straight to the desktop. I even encountered a really weird bug where, when I reloaded a save, there was snow on the ground even though it was autumn, and after I hit the “next turn” button, I was prevented from opening the menu to save or quit, and I couldn’t end the turn again. I was stuck in perpetual winter. I mean, I know the Starks were all like “Winter is coming” but I didn’t think it’d stay forever. In fact, these glitches came up so often that I actually did make a print screen and paste it into paint; you remember, the thing I said I wasn’t gonna do in my Fallout 2 review.
^(Fun fact: when I inserted this image, it kept flickering for a second or two before it settled. Hopefully it’s not cursed.)^
Also, the AI isn’t particularly engaging. With a little bit of strategy and good judgement, it’s possible to win a battle with a force half as powerful as your enemy’s. This isn’t to say that the battles aren’t fun or that there isn’t any risk involved, but just that the AI isn’t as amazing as one might desire out of their grand strategy experience.
The game is really bad at telling you how to get plot quests to appear. You get hand-held through the first set of plot quests; the first book, as the game calls it. But then, things just sort of happen. And eventually, you start coming up on quests from books three and four just because... enough time has passed? That would be my guess anyway. Eventually, I did figure out how to get the quests to appear, but it didn’t feel like a natural story progression. A few pro-tips in the plot quest department: First, conquering territory gets the quests from book two to appear. I’d suggest trying to take the Mercias (There are two, East Mercia and West Mercia), and any small kingdoms you may have left. Second, do not conquer Wales or the Saxons; they’re quest-important. Lastly, around turn 150, something really important happens, so get the quest “The Vision” finished up by then.
One last thing that really bugged me. There’s no way to tell what your income is until winter time, so you have to make absolutely sure that everything in your economy is squared away by then or else you might find yourself up a creek.
ለማገባደድ, despite the bugs, I would absolutely recommend King Arthur: A Roleplaying Wargame to anyone who’s into fantasy, Arthurian legend, grand strategy, or swords and sorcery type stories. It’s got a lot to like, and a lot of really neat ideas and aesthetics. I am probably totally going to keep playing it, at least to the regular campaign’s conclusion. Now, this being a game taking place in the early Middle Ages, there are instances of arranged marriages, with you deciding which maidens marry which knights. There is something to be said about how doing so improves the knights’ abilities, basically turning them into stat boosting objects, but this is justified somewhat in that the attributes that boost stats are personality traits, and it would make sense for a person to be influenced by a person they spend a lot of time with. What is perhaps more disturbing when one gets into fridge logic, is that these maidens can be bartered to certain groups on the map, such as rebels or mercenary groups. The game wants you to believe that you’re arranging marriages between the rebel leaders and your maidens, but because doing so makes use of the same interface as bartering artifacts or gold, it really presents the unfortunate implication that you may be selling these women into slavery. Is that what’s really going on? Prrrobably not, but once the whole “slavery” possibility occurred to me, it wouldn’t be shaken. Really, the sexism problems this game has are the same ones that plague any game that takes place in Medieval times, and the same ones that plagued Medieval times (the time period, not the restaurant). Although, it is weird that each of the female heroes have an ability that gives them a stat boost in return for being prohibited from riding horses... Yeah, I thought that was weird.
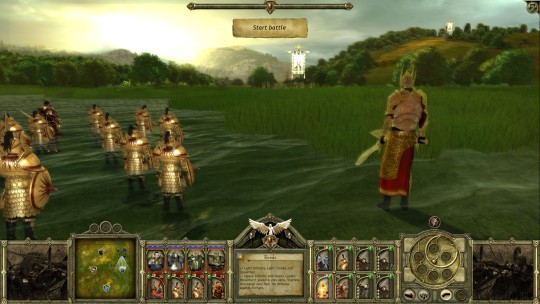
^(One of the Sihde, on the right, compared to a group of puny mortals on the left. Like, dang. Why haven’t those guys taken over Britannia on their own?)^
#archon reviews#review#video games#king arthur: a roleplaying wargame#king arthur#fantasy#grand strategy
0 notes
Text
Comprehension Man-Made Intellect, Equipment Understanding And Serious Studying
Unnatural Cleverness (AI) and its particular subsets Product Mastering (ML) and Strong Mastering (DL) are actively playing a serious position in Records Scientific discipline. Records Scientific discipline can be a thorough procedure that consists of pre-handling, examination, visualization and forecast. Allows deeply plunge into AI and its particular subsets.
Other Trusted Source : WEB Software
Man made Learning ability (AI) is often a part of computer system scientific research focused on setting up smart models effective at doing jobs that commonly need individual cleverness. AI is primarily separated into a few classifications as directly below
- Unnatural Reduce Cleverness (ANI)
- Manufactured Normal Intellect (AGI)
- Unnatural Awesome Learning ability (ASI).
Thin AI often introduced as 'Weak AI', works one particular activity within a certain way at its most effective. For instance, a computerized coffee maker robs which executes an effectively-characterized pattern of activities to help make a cup of coffee. Whilst AGI, also is introduced as 'Strong AI' carries out a wide selection of responsibilities which entail contemplating and thinking similar to a individual. Some case in point is Search engines Enable, Alexa, Chatbots which utilizes Purely natural Expressions Handling (NPL). Unnatural Excellent Intellect (ASI) may be the sophisticated variation which out executes our functions. It will carry out innovative pursuits like artwork, making decisions and emotionally charged human relationships.
Now let's take a look at Unit Studying (ML). It really is a subset of AI which involves modeling of techniques that helps for making estimates according to the identification of complicated records behaviour and models. Equipment understanding concentrates on empowering techniques to find out in the details offered, obtain experience to make forecasts on in the past unanalyzed info utilizing the details accumulated. Various ways of unit discovering are
- watched discovering (Vulnerable AI - Undertaking operated)
- no-monitored knowing (Sturdy AI - Information Run)
- semi-monitored studying (Solid AI -cost-effective)
- strengthened appliance mastering. (Sturdy AI - gain knowledge from blunders)
Monitored appliance mastering employs ancient information to comprehend behaviour and make upcoming forecasts. In this article the device includes a chosen dataset. It truly is tagged with factors for your insight plus the production. And because the new facts arrives the ML algorithm formula evaluation the brand new facts and provides the specific outcome judging by the predetermined guidelines. Watched studying is able to do category or regression projects. A example of category jobs are picture category, confront identification, e mail junk category, establish deception finding, and so on. as well as for regression jobs are weather conditions forecasting, people progress forecast, and many others.
Unsupervised appliance studying will not use any labeled or classed factors. It targets finding out concealed components from unlabeled information that will help devices infer a functionality appropriately. They normally use methods just like clustering or dimensionality decline. Clustering entails group information issues with a similar metric. It is actually info run and many cases for clustering are film suggestion for end user in Netflix, consumer segmentation, getting patterns, and many others. A number of dimensionality lessening good examples are attribute elicitation, massive info visualization.
Semi-watched unit studying works by employing equally branded and unlabeled records to better mastering exactness. Semi-watched studying can be quite a inexpensive alternative when labelling facts happens to be pricey.
Strengthening discovering is pretty distinct when compared with watched and unsupervised studying. It can be explained as a procedure of testing eventually presenting benefits. t is realized with the idea of iterative enhancement pattern (to understand by recent errors). Strengthening studying has additionally been employed to instruct products autonomous driving a motor vehicle inside of simulated conditions. Q-knowing is an illustration of support understanding techniques.
Transferring forward to Deeply Discovering (DL), it is actually a subset of device understanding that you make techniques that stick to a layered architectural mastery. DL employs a number of levels to steadily get advanced level characteristics out of the uncooked feedback. As an example, in picture refinement, reduced levels may possibly determine sides, even though greater levels can discover the principles related to a human being just like numbers or words or confronts. DL is normally referenced an in-depth synthetic neural community and they are the algorithm formula pieces that happen to be exceptionally correct for those complications like seem popularity, graphic acknowledgement, normal terminology refinement, and so on.
To sum up Records Scientific disciplines handles AI, which include device knowing. Having said that, appliance knowing themselves addresses an additional sub-modern technology, which can be heavy discovering. As a result of AI because it is ideal for resolving increasingly difficult difficulties (like finding cancer malignancy greater than oncologists) superior to people can.
Cinoy M R is usually a Enterprise Designer situated in Dubai with loaded expertise in technological innovation and organization consequence choices. He hold's level in Bachelors in Modern technology (Processing) from Thompson Estuaries and rivers University or college (TRU), Canada, Article Graduating in operation Operations, Experts in operation Operations (SAP).
0 notes
Text
A Zen Buddhist monk’s approach to democratizing AI
Colin Garvey, a postdoctoral research fellow at Stanford University’s Center for International Security and Cooperation (CISAC) and Institute for Human-centered Artificial Intelligence (HAI), took an unusual path to his studies in the social science of technology. After graduating from college, he taught English in Japan for four years, during which time he also became a Zen Buddhist monk. In 2014, he returned to the U.S., where he entered a PhD program in science and technology studies at Rensselaer Polytechnic Institute. That same year, Stephen Hawking co-authored an editorial in The Guardian warning that artificial intelligence could have catastrophic consequences if we don’t learn how to avoid the risks it poses. In his graduate work, Garvey set out to understand what those risks are and ways to think about them productively.
As an HAI Fellow, Garvey is working on turning his PhD thesis into a book titled Terminated? How Societies Can Avert the Coming AI Catastrophe. He is also preparing a policy report on AI-risk governance for a Washington, D.C.-based think tank and guest editing “AI and Its Discontents,” a special issue of Interdisciplinary Science Reviews featuring diverse contributions from sociologists to computer scientists, due out this December.
Here he discusses the need to change how we think and talk about AI and the importance of democratizing AI in a meaningful way.
How does the public’s tendency to see AI in either utopian or dystopian terms affect our ability to understand AI?
The risk of accepting the utopian or dystopian narrative is that it reinforces a very common attitude toward the evolution of AI and technology more generally, which some scholars describe as technological determinism. Either the market forces are inescapable, or, as some AI advocates might even say, it’s human destiny to develop a machine smarter than humans and that is the next step in evolution.
I think this narrative about inevitability is actually deployed politically to impair the public’s ability to think clearly about this technology. If it seems inevitable, what else is there to say except “I’d better adapt”? When deliberation about AI is framed as how to live with the impact, that’s very different from deliberating and applying public control over choosing what kind of impact people want. Narratives of inevitability ultimately help advance the agenda of beneficiaries of AI, while sidelining those at risk, leaving them very few options.
Another problem is that this all-good or all-bad way of framing the subject reduces AI to one thing, and that is not a good way to think about complex problems. I try to break that up by mapping risks in specific domains – political, military, economic, psychosocial, existential, etc. – to show that there are places where decision making can go differently. For example, within a domain, we can identify who is benefiting and who is at risk. This allows us to get away from this very powerful image of a Terminator robot killing everyone, which is deployed quite often in these types of conversations.
AI is not the first technology to inspire dystopian concerns. Can AI researchers learn from the ways society has dealt with the risks of other technologies, such as nuclear power and genetic engineering?
In the mid 20th century, social scientists who critiqued technology were very pessimistic about the possibility of humanity controlling these technologies, especially nuclear. There was great concern about the possibility of unleashing something beyond our control. But in the late 1980s, a second generation of critics in science and technology looked at the situation and said, here we are and we haven’t blown up the world with nuclear weapons, we haven’t released a synthetic plague that caused cancer in a majority of the population. It could have been much worse, and why not? My advisor, Ned Woodhouse, looked into these examples and asked, when things went right, why? How was catastrophe averted? And he identified five strategies that form the Intelligent Trial and Error approach that I have written about in relation to AI.
One of the Intelligent Trial and Error strategies is public deliberation. Specifically, to avert disaster, deliberation should be deployed early in development; a broad diversity of concerns should be debated; participants should be well-informed; and the deliberations should be deep and recurring. How well do you think AI is doing on that score?
I would say the strategy of deliberation could be utilized more thoroughly in making decisions about risk in AI. AI has sparked a lot of conversations since about 2015. But AI had origins in the 1950s. One thing I’ve found is that the boom and bust cycle of AI hype leading to disillusionment and a crash, which has happened roughly twice in the history of AI, has been paralleled by quite widespread deliberation around AI. For example, in the ’50s and ’60s there were conversations around cybernetics and automation. And in the ’80s there was a lot of deliberation about AI as well. For example, in the 1984 meeting of the ACM [Association for Computing Machinery], there were social scientific panels on the social impacts of AI in the main conference. So there has been a lot of deliberation about AI risk, but it’s forgotten each time AI collapses and goes away in what’s popularly known as an “AI winter.” Whereas with nuclear technology, the concern has been more ongoing, and that influenced the trajectory of the nuclear industry.
One way of looking at how little deliberation is going on is to look at examples of privacy violations where our data is used by an AI company to train a model without our consent. We could say that’s an ethical problem, but that doesn’t tell you how to solve it. I would reframe it as a problem that arose because decisions were made without representatives of the public in the room to defend the citizens’ right of privacy. This puts a clear sociological frame around the problem and suggests a potential strategy to address the problem in an institutional decision-making setting.
Google and Microsoft and other large companies have said that they want to democratize AI, but they seem to focus on making software open source and sharing data and code. What do you think it should mean for AI to be democratized?
In contrast to economic democratization, which means providing access to a product or technology, I’m talking about political democratization, which means something more like popular control. This isn’t mob rule; prudence is a key part of the framework. The fundamental claim is that the political system of democratic decision making is a way to achieve more intelligent outcomes overall compared to alternatives. The wisdom of crowds is a higher order effect that can arise when groups of people interact.
I think AI presents us with this challenge for institutional and social decision making, in that as you get more intelligent machines, you’ll need more intelligent democracies to govern. My book, based on my dissertation, offers some strategies for improving the intelligence of decision making.
What’s an example of how democratizing AI might make a difference today?
One area I’m watching closely and working on is the AI arms race with China. It’s painted as a picture of authoritarian China on the one hand and democracy on the other. And the current administration is funding what they call “AI with American values.” I would say that’s great, but where is democracy among those values? Because if they only refer to the values of the market, those are Chinese values now. There’s nothing distinct about market values in a world of global capitalism. So if democracy is America’s distinguishing feature, I would like to see the big tech companies build on that strength rather than, as I see happening now, convincing policy makers and government officials to spend more on military AI. If we’ve learned anything from the last cold war arms race, it’s that there really aren’t winners. I think a long-term multi-decade cold war with China over AI would be a race to the bottom. A lot of AI scientists would probably agree, but the same narrative framed in terms of inevitability and technological determinism is often used here in the security space to say, “We have no choice, we have to defeat China.” It will be interesting to see what AI R&D gets justified by that narrative.
Is there a connection between your Buddhism and your interest in AI?
When people hear that I’m a Zen Buddhist monk, they often say, you must want to tell programmers to meditate. But my concern has more to do with reducing suffering in the world. I see a huge risk for a profound kind of spiritual suffering that we are already getting some evidence of. Deaths of despair are an epidemic in the United States; and there’s a steep rise of suicide and depression among teenagers, even in the middle class. So there are some surprising places where material abundance isn’t translating into happiness or meaning. People are often able to withstand serious suffering if they know it’s meaningful. But I know a lot of young people see a pretty bleak future for humanity and aren’t sure where the meaning is in it all. And so I would love to see AI play a more positive role in solving these serious social problems. But I also see a potential for increased risk and suffering, in a physical way, maybe with killer robots and driverless cars, but potentially also psychological and personal suffering. Anything I can do to reduce that gives my scholarship an orientation and meaning.
In a world where much AI R&D is privatized and driven by capitalist profit motives at corporations around the globe, is it possible for thought leaders at a place like Stanford to make a difference in the trajectory of AI research overall?
Stanford certainly has the institutional capital and cultural cachet to influence the AI industry; the question is how it will use that power. The major problems of the 21st century are problems of distribution, not production. There’s already enough to go around; the problem is that a small fraction of humanity monopolizes the resources. In this context, making AI more “human-centered” requires focusing on the problems facing the majority of humanity, rather than Silicon Valley.
To pioneer a human-centered AI R&D agenda, thought leaders at Stanford’s HAI and elsewhere will have to resist the powerful incentives of global capitalism and promote things like funding AI research that addresses poor people’s problems; encouraging public participation in decision making about what AI is needed and where; advancing AI for the public good, even when it cuts into private profits; educating the public honestly about AI risks; and devising policy that slows the pace of innovation to allow social institutions to better cope with technological change.
Stanford has a chance to lead the world with innovative approaches to solving big problems with AI, but what problems will it choose?
— KATHARINE MILLER
source https://scienceblog.com/516656/a-zen-buddhist-monks-approach-to-democratizing-ai/
0 notes
Text
The Making of Everspace: Narratives in a Rogue-like
How to tell a story in which the hero dies all the time.
The decision to make Everspace a Rogue-like came first. We promised our Kickstarter backers that the pillars of the game would be a story-based 3D space shooter taking place in a procedurally generated world. Next came the challenge of finding an adequate narrative layer.
We knew that our playable ships, the actual “hero characters” needed a pilot, a hero with a soul and purpose. This hero should have proper motivation, a reason to be reborn after every death, some meaningful mission, and more than just mechanics like fighting and exploring stunning space sceneries in a rock-hard 3D shooter. We wanted a believable hero, compatible with the genre of a space shooter, a purebred sci-fi game.
Dealing with a sci-fi genre, we had a broad range of choices. Explanations and reasons for permadeath combined with rebirth or resurrection had to be found. A plausible and scientific approach to how a human pilot could immediately respawn after dying and keep the memory of his predecessors was human cloning in combination with memory/personality transplantation. Both are common features in sci-fi film and literature such as the cloned protagonist in the Oblivion movie or the transplanted memories from the feature film Total Recall.
A solution to this was that our hero should be a human clone on a secret mission who had to reach an unknown and mysterious destination in space – one of the gameplay premisses we borrowed from the FTL game. But we also needed an explanation for how the hero could remember what happened before he died. Just like in the feature films Edge of Tomorrow or Groundhog Day, where the protagonists experience their countless lives and deaths as one continuous existence and a line of learning, experimentation and self-improvement. A series of trial and error, an endless series of catastrophic failures, at times boredom and epic success, also the ingredients that make roguelikes so memorable. We didn’t want to explain the preservation of memory beyond death with magic or mystery but rather with science fiction. Such as a built-in military system that would transfer a dead pilot’s memories and experiences to a cloud computer and implant them back into the newly respawned pilot on the same mission, giving him the decisive advantage to learn from his failures. We called this system Aeterna, a military system used in the 3rd millennium in a distant interstellar conflict between the Human race and an alien opponent, the Okkar.
Adam Roslin is getting the cytotoxin injection.
Visualizing the nature of the game Before we developed a more detailed story, we had to define the nature of the game, its tone of voice and overall atmosphere. We wanted players to experience the dark and disturbing side of a cyberpunk scenario such as the fate of the replicants in Blade Runner. The only one helping our hero along the way is a reluctant AI, a machine, constantly reminding him of his deficiencies. We put the hero in the role of a replicant and not the Blade Runner, in order to create an even more disturbing experience, being hunted by everyone and the desire to reveal the secret behind his existence.
The game world itself could have been like the overcrowded and dystopian scenario we know from Blade Runner and other cyberpunk movies and literature. But a war-torn and mostly depopulated region in space was the better fit for the story, for the gameplay and our limited resources back in the time. We even declared the region a demilitarized zone, so we had very limited and controlled activity from a developer perspective but at the same time a disturbing scenario telling a story of conflict.
Developing the story synopsis With such a premise, we didn’t want our hero to be just another mindless space marine on a standard war mission. We promised something else to our backers. We wanted something more mysterious, something mind-twisting similar to the feature film Moon, where the protagonist would discover his own clone existence at some point. We came up with the idea that the hero initially has no clue that he is actually a clone, created by a hacker, who illegally uploaded his DNA to Aeterna in order to replicate his own, dying body that he keeps in stasis.
The hacker, a victim of a deadly conspiracy, is waiting in a secret hideout deep in a demilitarized zone until finally one of the clone pilots he summoned would reach the destination. He would then use the new body for himself after transplanting his memory and personality back to his clone. This already sounds pretty complicated. But the hardest part of the plan is that the clone pilots have to traverse the demilitarized zone, a former war zone, being hunted by aliens and humans alike, but also threatened by extraplanetary life forms and natural hazards.
Initially, the hacker just wanted a body replacement. And usually military clones are produced without personality. But when he set up the exploit protocol in Aeterna and uploaded his DNA, he made errors, resulting in the clones having flashbacks of the hacker’s past and fragmented memories. The hero experiences these flashbacks each time he respawns. At first he thinks that they are his own memories, but slowly he realizes that the memories are those of the hacker, revealing with each additional death another puzzle piece of the hackers identity, past and motives.
Main- and side-characters (archetypes) in Everspace.
Evolving the cast of characters Besides the hero and the hacker, we wanted to have an interesting mix of story archetype characters from different backgrounds, diverse species and genders with distinctive narrative and gameplay functions.
HACKER (Adam Roslin): A scientist recruited and trained by the Colonial Fleet. He was responsible for the military cloning program during the Colonial War, thus his background with Aeterna and his skills in creating human as well as alien clones. He is the one who sets everything in motion but never appears in-game, only at the final destination in a Bladerunner-like “Tears in the Rain” cinematic.
HERO: One of hundreds of human clones produced on a wrecked clone carrier in the DMZ (demilitarized zone), sent on a secret mission to reach a specific location, the hacker’s secret hideout. Along with the game progression he gathers experience and improves with each failure. However, he is plagued and confused by his strange flashbacks and horrified by the discovery that he is experiencing someone else’s fragmented memories, those of his clone father, the hacker Adam Roslin.
HIVE: The military AI (artificial intelligence) installed on each ship the hero uses. It provides vital information on how to control the ship and how to survive in such a hostile environment, which is its purpose as military AI. Being obliged to help a rookie clone that has been hacked into its system annoys the AI and creates a field of tension between the hero and HIVE that slowly slackens as the hero’s skills improve. Besides the tutor’s function, HIVE will explain background information and lore related to anything worth mentioning in the game world, triggered by procedurally generated events and level creation.
FOE: A former partner of the hacker who wants revenge and who is chasing him. He doesn’t know yet that the hacker has created clones and is hiding somewhere. Every time the hero runs into him, the encounter would mean a difficult fight to the death but also more background information for the hero in order to understand the reasons of his existence. Eventually, the foe will learn that the hero is a clone and follow him secretly in order to settle the score with the hacker.
FRIEND: A friend who offers unconditional help by providing provisions and useful tips. The friend, an attractive woman, initially gives rise to even more questions but will provide the crucial answers at a later point and, being the hacker’s sister, shed light upon the hero’s past and identity. She definitely knows that the hero is one of his brother’s clones and sees them dying by the hundreds. Nevertheless, she will sacrifice her own life so that her brother can get his much-needed body replacement.
TRADER: An outcast Okkar, the opposing party in the Okkar War, who is willing to trade goods with our hero, providing interesting background info about the alien race and Cluster 34, the Beltegrades, the name of the game world. He gives the other side’s perspective of the two belligerent parties and will be the hero’s best trading opportunity, even after completing his questline.
SEDUCER: A furry alien belonging to an Outlaw clan and a rather comical character. He tries to involve the hero in dangerous missions he can’t fulfil himself. Outlaws are often organized in clans and populate the DMZ where they are relatively safe from Colonial persecution. They regularly raid mining and trading convoys and live from illegal mining and smuggling. After completing his questline, the seducer and his clan will become a permanent ally for the hero that he can summon in critical situations.
SHIFTER: A female human spy. She provides interesting missions and tries to gain the hero’s trust but is actually hired by the Okkar to eliminate him since he represents an infraction to the Colonial-Okkar piece treaties and thus poses a threat to the order in the DMZ. She will permanently leave the game world after completing her questline.
BOUNTY HUNTER: An incompetent android who is addicted to gambling. Another fun character who will pay the hero for chasing criminals/pirates for him. Ironically, after completing some dead-or-alive contracts for him, the hero himself will be on the bounty hunter’s most wanted list.
SCIENTIST: An alien scholar who is interested in acquiring knowledge. At once he knows that the hero is a clone and he sends him on exploration and research missions, like collecting samples of the extra orbital life forms in the game, some of them not being harmless.
ADMIRAL: The admiral is the actual antagonist of the story. The hero has been facing his warships each time when he stayed too long in one location. But the hero will only see his face and voice in the very final level after having reached the final destination and after having collected all remaining DNA fragments from dead fellow clones he needed in order to become a complete person. The admiral is the final enemy and the most epic and challenging opponent in a planetary scenario.
An exemplary game loop based on narrative events.
Refining the narrative structure Being a Rogue-like game with a live, die and repeat formula and with a mostly procedurally generated game world, it was hard to come up with a solid and meaningful narrative structure. What we did, in the end, was to design individual and independent storylines for each archetype character and to define a fixed amount of narrative events for all of them. Some of the archetypes like FRIEND and FOE had a common history and had to be designed with partly shared game events, for example, when FOE was killing FRIEND after the hero has reached the final destination.
Based on certain conditions like game progression, player stats, world and level state and character interaction, those story and mission events were triggered per character and in a linear progression. Some of the storylines ended because a character died or left the game world. Others are repeating indefinitely, such as trade opportunities or battle support (trader/seducer).
The narrative experience for a player during a typical run through several sectors then could be to encounter e.g. FRIEND, FOE, SEDUCER, SHIFTER and TRADER, each with a new story and gameplay event before the hero dies again and respawns.
The challenge here was to find the optimal formula for how and when to trigger events to deliver a balanced narrative and gameplay experience without flaws and logical errors such as encountering several of the archetypes in one game level.
In the end, one can compare the narrative structure of Everspace to an open world RPG with main and side-quests but where the quests or missions are delivered to the player/hero in a partly randomized way, creating a different experience for every player because the sequence of story events as well as the procedural level creation is always different.
One of the downsides here was that inexperienced players sometimes had rather long pauses between narrative events because they simply got shot down too often, had to start from scratch and didn’t progress fast enough in the game. To a certain extent common for most other narrative games, but in our case, the narrative hook can get lost more easily.
Following up with Everspace 2 All in all, we think that the narrative delivery of Everspace was a success, considering the limitations and difficulties that we had to master with a Rogue-like formula and with a 99% procedurally generated game world. For a Rogue-like game it was definitely interesting to have such a narrative structure, where missions are delivered to the player in a very non-predictive way.
We also think that the story of the cloned hero has potential for a sequel. What will become of our hero now that he has revealed the mystery behind his existence and earned his freedom? He has saved his life but still is a man with little life experience, a blank page with fragmented memories from someone else. What does it take now to become a real human? Many of his inner and outer conflicts remain and new ones that seem pretty common to us but the hero himself never even dreamed of are about to emerge. We think that this is going to be the narrative theme of Everspace 2. The story of the clone in the DMZ continues, only that we would build this game rather in an open world scenario with a fixed game world and procedurally generated levels being the exception. For the narrative structure, we would use a rather traditional main and side mission mechanics similar to many RPGs, delivered through cinematics, in-game cutscenes and dialogue.
Read also:
The Making of Everspace: Interview with Rockfish-CEO Michael Schade
Developing the Rogue-like formula of Everspace
Uwe Wütherich Creative & Art Director
Uwe Wütherich was Creative Director at Fishlabs Entertainment for almost a decade before he joined the freshly founded Rockfish-Team in 2014. Uwe studied architecture together with art & media-technology and has an ample background in 3D art, exhibition design, digital media and entertainment.
The post The Making of Everspace: Narratives in a Rogue-like appeared first on Making Games.
The Making of Everspace: Narratives in a Rogue-like published first on https://leolarsonblog.tumblr.com/
0 notes