#RecommendationSystems
Explore tagged Tumblr posts
Text
AI and Network Science
AI and Network Science are increasingly converging, leveraging the power of graph-based representations to solve complex problems in interconnected systems. Network science studies the structure and dynamics of networks, such as social, biological, and technological systems. AI, particularly through Graph Neural Networks (GNNs), uses this knowledge to process and analyze graph-structured data.
Key Contributions of Network Science to AI:
Modeling Relationships: Network science provides tools to represent systems as graphs, capturing relationships and dependencies critical for AI applications.
Understanding Dynamics: Insights into network dynamics, like spreading phenomena, enhance predictive models in AI.
How AI Empowers Network Science:
Learning from Data: AI algorithms, such as GNNs, learn patterns from large-scale networks, uncovering insights in areas like social influence, disease spread, or infrastructure optimization.
Scalability: AI improves the analysis of massive, dynamic networks that traditional methods struggle with.
Applications of AI and Network Science:
Social Network Analysis: Predicting trends and detecting communities.
Biology and Healthcare: Drug discovery and modeling disease propagation.
Infrastructure Optimization: Enhancing transport or energy grids.
Knowledge Graphs: Powering natural language understanding in AI systems.
International Conference on Network Science and Graph Analytics
Our Website : https://networkscience-conferences.researchw.com/
Connected With Here :
Instagram : https://www.instagram.com/emileyvaruni/ Tumblr : https://www.tumblr.com/emileyvaruni Pinterest : https://in.pinterest.com/emileyvaruni/ Blogger : https://emileyvaruni.blogspot.com/ Twitter : https://x.com/emileyvaruni YouTube : https://www.youtube.com/@emileyvaruni
#AI#NetworkScience#GraphNeuralNetworks#MachineLearning#DeepLearning#DataScience#GraphTheory#ComplexSystems#ArtificialIntelligence#NeuralNetworks#BigData#RecommendationSystems#SupplyChainOptimization#PredictiveModeling#DataMining#SocialNetworks#BiologicalNetworks#KnowledgeGraphs#Connectivity#NetworkAnalysis.#sciencefather
0 notes
Text
Predictive Analytics: Anticipating Consumer Needs and Trends
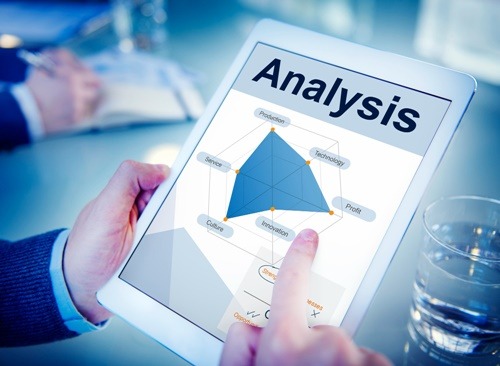
Discover how predictive analytics is revolutionizing market research and helping businesses stay ahead of the curve. Learn how to use data to anticipate consumer needs, forecast market trends, and gain a competitive edge.
Link : https://maction.com/predictive-analytics-anticipating-consumer-needs-and-trends/
#predictiveanalytics#consumerbehavior#marketresearch#datadrivenmarketing#markettrends#forecasting#machinelearning#AI#datascience#customersegmentation#customerlifetimevalue#demandforecasting#pricingoptimization#personalizedmarketing#customerchurnprediction#salesforecasting#recommendationsystems#trendanalysis
0 notes
Text
Discover the magic of AI-based recommendation systems! 🌟 These intelligent algorithms analyze your preferences and behavior to curate personalized suggestions, making your experience smoother and more enjoyable. From discovering your next favorite movie to finding that perfect product, AI recommendations are revolutionizing how we interact with technology. Explore how companies are harnessing this cutting-edge technology to enhance your digital life. Dive into the future of personalization and let AI guide you to exactly what you’re looking for. 🚀
Read more about this exciting tech and how it’s changing the game!
0 notes
Text
Creating a Machine Learning-Powered Recommendation System with FastAPI and Scikit-learn
🔍 Interested in building a recommendation engine? Learn how to use FastAPI and Scikit-learn to create and deploy a machine learning-powered recommendation system as a REST API. We offer help with:
Setting up FastAPI and Scikit-learn.
Developing the recommendation model.
Deploying your system as a REST API.
💬 Contact us on WhatsApp at +971501618774 for personalized support!
0 notes
Text
Recommendation Systems
I first learned of recommendation systems during my first literature review course at NCU. The topic was online reviews and recommender systems were one type of system connected with online reviews. I included some information in my paper on these systems that made recommendations to users. I thought they added a lot of value (were very helpful and useful to the consumer). Today, I learned of recommendation systems for music. I found several papers on systems that use different approaches to recommend music to users. How interesting! I would like to personally test these systems.
A Music Recommendation System Based On Psychotherapy By: Zhiyuan Liu, Wei Xu, Wenping Zhang, Qiqi Jiang https://www.sciencedirect.com/science/article/pii/S277256932300097X
I am all EARS: Using Open Data And Knowledge Graph Embeddings for Music Recommendations By: Niels Bertram, Jürgen Dunkel, Ramón Hermoso https://www.sciencedirect.com/science/article/pii/S0957417423008497
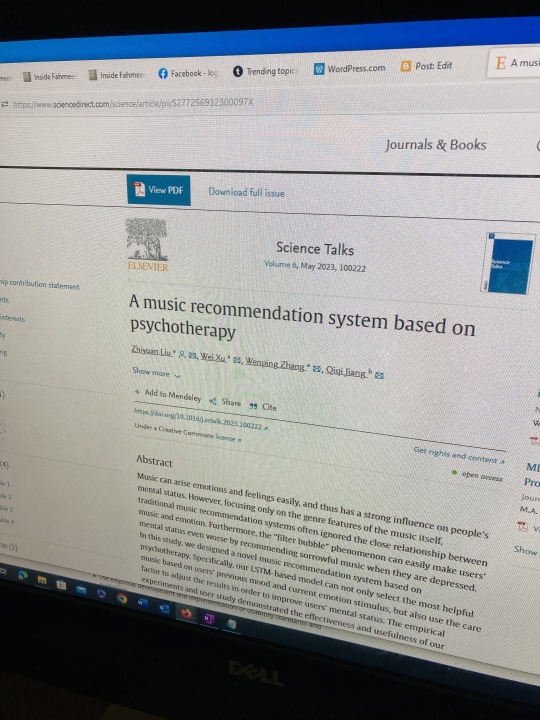
#fahmeenaodetta#recommendationsystem#onlinereviews#music#signatureassignment#phdpaper#researchtopics
0 notes
Text
Case study Spotify | Spotify case study ux | Spotify case study 2019 | on Artificial Intelligence
"In this Video i have explained how Spotify remains top of the music streaming world ?
Source: 5 Minutes Learning
#Spotifycasestudyux#cassestudyspotify#5MinutesLearning#spotify#bigdata#music#dataanalytics#wync#airtelwync#onlinemusic#musicstreaming#recommendationsystem#uxdesign#uxdesigncasestudy#musicapp "
#mktmarketing4you#corporatestrategy#marketing#M4Y#lovemarketing#IPAM#ipammarketingschool#ContingencyPlanning#virtual#volunteering#project#Management#Economy#ConsumptionBehavior#BrandManagement #ProductManagement #Logistics #Lifecycle
#Brand #Neuromarketing #McKinseyMatrix #Viralmarketing #Facebook #Marketingmetrics #icebergmodel #EdgarScheinsCultureModel #GuerrillaMarketing #STARMethod #7SFramework #gapanalysis #AIDAModel #SixLeadershipStyles #MintoPyramidPrinciple #StrategyDiamond #InternalRateofReturn #irr #BrandManagement #dripmodel #HoshinPlanning #XMatrix #backtobasics #BalancedScorecard #Product #ProductManagement #Logistics #Branding #freemium #businessmodel #business #4P #3C #BCG #SWOT #TOWS #EisenhowerMatrix #Study #marketingresearch #marketer #marketing manager #Painpoints #Pestel #ValueChain # VRIO #marketingmix
youtube
3 notes
·
View notes
Text
Tweeted
Participants needed for online survey! Topic: "Negative impact of privacy risks on purchase of recommendation systems" https://t.co/5qZmWZsTVM via @SurveyCircle #ArtificialIntelligence #RecommendationSystems #privacy #PurchaseIntention #survey #surveycircle https://t.co/qpnApT8MlU
— Daily Research @SurveyCircle (@daily_research) Jan 11, 2023
0 notes
Link
1 note
·
View note
Photo

📣Are you an AI enthusiast and would like to broaden your knowledge about in what more ways AI 🧬 can possibly be useful? We will then invite you to watch the replay🎥 of our very first webinar which took place on Wednesday 2nd February 2022 where we talked about AI Recommendation system types, methods of building recommendation systems, building a recommendation system using word embedded method, etc. Link to the replay is in our bio and in the comments below👇 #airecommendationsystem #recommendationsystem #webinar #mlmodels #datascience #ai #datatera #artificialintelligence #aimodels (at Stockholm, Sweden) https://www.instagram.com/p/CZ_-Do2D7Dz/?utm_medium=tumblr
#airecommendationsystem#recommendationsystem#webinar#mlmodels#datascience#ai#datatera#artificialintelligence#aimodels
0 notes
Text
Recommendation System
สำหรับบทความนี้ อยากจะพูดถึง Recommendation System in ML ตอนนี้หลายๆ คนน่าจะคุ้นชินกับการที่เราใช้ Social Media แล้วมีการแนะนำสินค้าต่างๆ ในระบบ หรือได้ยินจากร้านสะดวกซื้อหลายๆ แห่ง ว่า “customers who bought this, also bought this” จริงๆ แล้วเจ้าระบบแนะนำนี้ เป็นส่วนหนึ่งของ Algorithms ใน Data Analytics เลยนะ
Above image source from : https://www.xenonstack.com/use-cases/recommendation-system-deep-learning/
แล้วเจ้า Techniques หรือ Algorithms นี้มันดียังไง
1. ช่วยแนะนำสิ่งที่กลุ่มเป้าหมายจะสนใจ
นั่นเพราะว่าในโลกออนไลน์ตอนนี้มีสิ่งที่น่าสนใจอยู่มากมายมหาศาล จนจะให้มานั่งดูเองทั้งหมดคงยากมาก เปลืองทั้งเวลา เปลืองทรัพยากรด้วย แถมพอจะเข้ามาดูอะไรก็หาไม่เจอเพราะมันเยอะเกินไป แทนที่จะชอบใจกลับกลายเป็นเบื่อหน่ายกับเวปเราพอดี ดังนั้นถ้ามีเจ้าเทคนิคนี้เกิดขึ้น ก็จะทำให้เลือกนำเสนอแต่สิ่งที่กลุ่มเป้าหมายกำลังค้นหา แทนที่จะนำเสนอทุกสิ่งที่มี
2. เปิดโอกาสให้กลุ่มเป้าหมายใหม่ได้รู้จักกับสินค้าอื่น นอกเหนือจากที่สนใจอยู่ได้ด้วยนะ
>> ถ้าเราจะทำระบบนี้ เราต้องคำนึงถึงเรื่องไหนบ้าง
๐ Relevence ก็คือ การพยายามหาของที่คิดว่าผู้ใช้สนใจ ไม่นำเสนอของแบบสะเปะสะปะ เพราะมันจะทำให้กลุ่มเป้าหมายรู้สึกยุ่งยากในการค้นหาสิ่งที่ต้องการ
๐ Diversity ต้องมีความหลายหลายในการนำเสนอ มันคงไม่ดีแน่ ถ้าเราเข้าเวปมาแล้วเจอแค่ Youtube ที่แนะนำคลิปเดิมๆ จาก Channel เดิมๆ หรือใน IG ที่มีโหมดแนะนำอย่าง Reels ถ้าขึ้นแนะนำมาแต่ของเดิมๆ มันก็จะดูไม่น่าสนใจ ทำให้ไม่อยากใช้แอพนั้นอีก
๐ Novelty ต้องมีความ���หม่ในการเสนอ ความหมายคืออะไร คือการที่เรานำเสนอในสิ่งที่กลุ่มเป้าหมายไม่รู้จักบ้าง เช่น แนะนำที่เที่ยวใหม่ๆ รีสอร์ตใหม่ๆ ร้านอาหารใหม่ๆ เป็นต้น
๐ Serendipity หาสิ่งที่นอกเหนือความคาดหมาย หรือ เป็นสิ่งไกลตัวของกลุ่มเป้าหมายแต่มีประโยชน์ต่อกลุ่มเป้าหมาย
>> Model ของ Recommondation System
จริงๆ แล้วโมเดลที่ใช้มีหลายวิธีมาก แต่ส่วนใหญ่ก็ยังคงอยู่ในรูปแบบของ utility function ของกลุ่มเป้าหมาย และสินค้า/บริการ ที่นิยมใช้กันคร่าวๆ ดังนี้
popularity based
classification based
content based
collaborative filtering
Hybrid models
โดยที่ว่า
๐ Popularity Based
เป็นวิธีการที่ง่ายที่สุดในการสร้างระบบแนะนำ คือจะดูความนิยมของสินค้า หรือบริการนั้นๆ จากจำนวนยอดขาย หรือจำนวนยอดการใช้งาน ตัวอย่างเช่น ร้านอาหารในแ��พ foodpanda ที่ดูจากยอดการสั่งซื้อ

๐ Classification Based
วิธีการนี้จะนำเอาลักษณะของลูกค้า เช่น เพศ อายุ เป็นต้น กับลักษณะของสินค้า เช่น ราคา คุณภาพ มาใช้ในการทำนายความชอบของสินค้า หรือบริการของลูกค้ารายนั้นๆ โดยคำตอบที่ได้จากระบบแนะนำจะมีแค่ ชอบ หรือไม่ชอบ
๐ Content based Filtering
วิธีการนี้ก็เป็นวิธีการที่ง่ายๆ และตรงกับกลุ่มเป้าหมายมากๆ โดยจะพิจารณาจากข้อมูลย้อนหลังของผู้ใช้แต่ละคนว่าเคยดูหนังแนวไหน ซื้อของแบบไหนบ้าง ฟังเพลงแบบไหนบ้าง ก็จะแนะนำสินค้าหรือบริการแนวนั้นเพิ่มเติมให้
ข้อดีคือ จะได้สิ่งที่กลุ่มเป้าหมายต้องการ
ข้อเสียคือ ไม่มีความหลายหลายให้กลุ่มเป้าหมายได้รู้จักเพิ่ม
๐ Collaborative Filtering
ใช้หลักการง่ายๆ แค่ว่า ถ้ากลุ่มเป้าหมายชอบสินค้าแนวเดียวกัน ก็อาจจะสนใจสินค้าอื่นๆ เหมือนกันก็ได้
Collaborative Filtering สามารถแบ่งออกได้เป็น 2 ประเภท คือ
nearest neighbor
matrix factorization
=> Nearest Neighbor Collaborative Filtering
โดยระบบแนะนำประเภทนี้ จะใช้ความคล้ายคลึงมาเป็นตัวแนะนำ ที่พบเห็นจะมี 2 ลักษณะ คือ 1. ความคล้ายคลึงกันของลูกค้า (user based filtering) 2. ความคล้ายคลึงกันของสินค้า (item based filtering)

>> User-based Collaborative Filtering
เป็นการจัดกลุ่มเป้าหมายตามการซื้อสินค้า/บริการ โดยถ้าลูกค้ามีลักษณะการซื้อสินค้าชนิดที่ใกล้เคียงกัน ระบบก็จะจัดกลุ่มลูกค้าดังกล่าวไว้ในกลุ่มเดียวกัน และในลูกค้ากลุ่มเดียวกันนี้ ถ้าลูกค้าคนหนึ่งมีแนวโน้มที่จะชอบสินค้าและบริการอย่างหนึ่ง (ดูจากประวัติการซื้อย้อนหลัง) ระบบก็จะแนะนำสินค้าหรือบริการนั้นๆ ให้กับลูกค้าคนอื่นๆ ในกลุ่มด้วย
ตัวอย่างเช่น ใน Netflix ระบบพบว่ามีลูกค้า 2 คน ที่มีลักษณะดูหนังที่คล้ายกัน เช่น น้องบูม และน้องครีม มีประวัติการดูหนังต่างๆ ที่เหมือนกัน เช่น อะนิเมะ เรื่อง My Hero Academia , อะนิเมะเรื่อง Jujutsu Kaisen เป็นต้น โดยระบบจะจัดกลุ่มให้น้องบูม และน้องครึมอยู่ cluster เดียวกัน
ต่อมาน้องบูมได้อะนิเมะ โคนัน เพิ่ม ระบบก็จะแนะนำหนังเรื่องเดียวเดียวกันนี้ให้น้องครีมด้วย
>> Item-based Collaborative Filtering
เป็นการจัดกลุ่มสินค้าที่มีคุณลักษณะที่คล้าย หรือเกี่ยวเนื่องกัน เช่น ในเว็บไซด์ช็อปปิ้งออนไลน์แห่งหนึ่ง ดินสอและยางลบมักจะถูกลูกค้าซื้อพร้อมกันๆ เสมอ ดังนั้นดินสอและยางลบจึงถูกจัดให้อยู่ในกลุ่มสินค้าที่ใกล้เคียงกัน
=> Matrix Factorization Collaborative Filtering
เป็นการจัดกลุ่มลูกค้าตามการให้คะแนน (rating) ของสินค้า หรือบริการ โดยระดับคะแนนอาจอยู่ในช่วงตั้งแต่ 1- 10 คะแนน ในกรณีที่ยังไม่มีการให้คะแนนจะเท่ากับ 0
๐ Hybrid ก็คือ การเอาหลายๆ วิธีมาผสมผสานกัน
References:
1. https://madasamy.medium.com/introduction-to-recommendation-systems-and-how-to-design-recommendation-system-that-resembling-the-9ac167e30e95
2. https://medium.com/@cfpinela/recommender-systems-user-based-and-item-based-collaborative-filtering-5d5f375a127f
1 note
·
View note
Photo

On @techradar again! This time we talk about the #RecommendationSystems and their black soul. Could our future be influenced by them? Check this article! Come fanno le AI a leggerci nel pensiero (o così sembra)
#AI #MachineLearning
#machine learning#ai#artificial intelligence#press#italy#future#futurology#tech#recommendationsystem
0 notes
Photo

Automized Data Analysis by OneDot Enterprises Private Limited 🔖 Chatbot Development 🔖 Recommendation System 🔖 Automized Data Analysis 🔖 Forecasting System . . . #onedotenterprises #pvtltdcompany #itservices #machinelearning #artificialintelligence #chatbot #recommendationsystem #dataanalysis #forecastingsystem (at OneDot Enterprises Private Limited) https://www.instagram.com/p/CEwZIFvle-9/?igshid=znyvbg9h6zb3
#onedotenterprises#pvtltdcompany#itservices#machinelearning#artificialintelligence#chatbot#recommendationsystem#dataanalysis#forecastingsystem
0 notes
Text
Today’s #RecommendationSystems lesson: only return the N items per user with the highest predicted ratings. Otherwise, you’ll generate a query users x all items dense matrix. Oops.
0 notes
Text
AI-powered recommendation system
Want to create an AI-powered recommendation system? Start with data collection, employ machine learning algorithms like collaborative filtering or content-based filtering, and constantly iterate for accuracy. Dive deeper into the steps involved and unleash the potential of personalized recommendations.
Read more ➡️
0 notes
Photo

"Tutorial: Building a Recommendation System with Python using Collaborative Filtering → https://bit.ly/319S1Xw via @Towards_AI⠀ #CollaborativeFiltering #RecommendationSystem #Tutorial #MachineLearning #ML #ArtificialIntelligence #AI #DataScience #Coding #Programming #TowardsAI ⊕ [ Link on Bio ] ⊕"
0 notes
Photo

Chatbot Development by OneDot Enterprises Private Limited 🔖 Recommendation System 🔖 Appointment System 🔖 Customer Support System 🔖 Lead Generation 🔖 Rating/Feedback System Contact 📧 [email protected] 📱+919096835272 . . . #onedotenterprises #pvtltdcompany #itservices #chatbot #dataanalytics #recommendationsystem #appointmentsystem #customersupportsystem #leadgeneration #feedbacksystem (at OneDot Enterprises Private Limited) https://www.instagram.com/p/CEJ4XNxFWRE/?igshid=5pw3xoceuypu
#onedotenterprises#pvtltdcompany#itservices#chatbot#dataanalytics#recommendationsystem#appointmentsystem#customersupportsystem#leadgeneration#feedbacksystem
0 notes