#FastAPI
Explore tagged Tumblr posts
Text

Literally this
#Literally this#literally this time#fastapi#advertisements#advertising#marketing#job applications#jobsearch#online jobs#jobs in usa#usa student jobs#jobs#jobopening#job#ausgov#politas#auspol#tasgov#taspol#australia#fuck neoliberals#neoliberal capitalism#anthony albanese#albanese government#employment#employees#employers#slavery#slaves#slave
17 notes
·
View notes
Text
Asynchronous LLM API Calls in Python: A Comprehensive Guide
New Post has been published on https://thedigitalinsider.com/asynchronous-llm-api-calls-in-python-a-comprehensive-guide/
Asynchronous LLM API Calls in Python: A Comprehensive Guide
As developers and dta scientists, we often find ourselves needing to interact with these powerful models through APIs. However, as our applications grow in complexity and scale, the need for efficient and performant API interactions becomes crucial. This is where asynchronous programming shines, allowing us to maximize throughput and minimize latency when working with LLM APIs.
In this comprehensive guide, we’ll explore the world of asynchronous LLM API calls in Python. We’ll cover everything from the basics of asynchronous programming to advanced techniques for handling complex workflows. By the end of this article, you’ll have a solid understanding of how to leverage asynchronous programming to supercharge your LLM-powered applications.
Before we dive into the specifics of async LLM API calls, let’s establish a solid foundation in asynchronous programming concepts.
Asynchronous programming allows multiple operations to be executed concurrently without blocking the main thread of execution. In Python, this is primarily achieved through the asyncio module, which provides a framework for writing concurrent code using coroutines, event loops, and futures.
Key concepts:
Coroutines: Functions defined with async def that can be paused and resumed.
Event Loop: The central execution mechanism that manages and runs asynchronous tasks.
Awaitables: Objects that can be used with the await keyword (coroutines, tasks, futures).
Here’s a simple example to illustrate these concepts:
import asyncio async def greet(name): await asyncio.sleep(1) # Simulate an I/O operation print(f"Hello, name!") async def main(): await asyncio.gather( greet("Alice"), greet("Bob"), greet("Charlie") ) asyncio.run(main())
In this example, we define an asynchronous function greet that simulates an I/O operation with asyncio.sleep(). The main function uses asyncio.gather() to run multiple greetings concurrently. Despite the sleep delay, all three greetings will be printed after approximately 1 second, demonstrating the power of asynchronous execution.
The Need for Async in LLM API Calls
When working with LLM APIs, we often encounter scenarios where we need to make multiple API calls, either in sequence or parallel. Traditional synchronous code can lead to significant performance bottlenecks, especially when dealing with high-latency operations like network requests to LLM services.
Consider a scenario where we need to generate summaries for 100 different articles using an LLM API. With a synchronous approach, each API call would block until it receives a response, potentially taking several minutes to complete all requests. An asynchronous approach, on the other hand, allows us to initiate multiple API calls concurrently, dramatically reducing the overall execution time.
Setting Up Your Environment
To get started with async LLM API calls, you’ll need to set up your Python environment with the necessary libraries. Here’s what you’ll need:
Python 3.7 or higher (for native asyncio support)
aiohttp: An asynchronous HTTP client library
openai: The official OpenAI Python client (if you’re using OpenAI’s GPT models)
langchain: A framework for building applications with LLMs (optional, but recommended for complex workflows)
You can install these dependencies using pip:
pip install aiohttp openai langchain <div class="relative flex flex-col rounded-lg">
Basic Async LLM API Calls with asyncio and aiohttp
Let’s start by making a simple asynchronous call to an LLM API using aiohttp. We’ll use OpenAI’s GPT-3.5 API as an example, but the concepts apply to other LLM APIs as well.
import asyncio import aiohttp from openai import AsyncOpenAI async def generate_text(prompt, client): response = await client.chat.completions.create( model="gpt-3.5-turbo", messages=["role": "user", "content": prompt] ) return response.choices[0].message.content async def main(): prompts = [ "Explain quantum computing in simple terms.", "Write a haiku about artificial intelligence.", "Describe the process of photosynthesis." ] async with AsyncOpenAI() as client: tasks = [generate_text(prompt, client) for prompt in prompts] results = await asyncio.gather(*tasks) for prompt, result in zip(prompts, results): print(f"Prompt: promptnResponse: resultn") asyncio.run(main())
In this example, we define an asynchronous function generate_text that makes a call to the OpenAI API using the AsyncOpenAI client. The main function creates multiple tasks for different prompts and uses asyncio.gather() to run them concurrently.
This approach allows us to send multiple requests to the LLM API simultaneously, significantly reducing the total time required to process all prompts.
Advanced Techniques: Batching and Concurrency Control
While the previous example demonstrates the basics of async LLM API calls, real-world applications often require more sophisticated approaches. Let’s explore two important techniques: batching requests and controlling concurrency.
Batching Requests: When dealing with a large number of prompts, it’s often more efficient to batch them into groups rather than sending individual requests for each prompt. This reduces the overhead of multiple API calls and can lead to better performance.
import asyncio from openai import AsyncOpenAI async def process_batch(batch, client): responses = await asyncio.gather(*[ client.chat.completions.create( model="gpt-3.5-turbo", messages=["role": "user", "content": prompt] ) for prompt in batch ]) return [response.choices[0].message.content for response in responses] async def main(): prompts = [f"Tell me a fact about number i" for i in range(100)] batch_size = 10 async with AsyncOpenAI() as client: results = [] for i in range(0, len(prompts), batch_size): batch = prompts[i:i+batch_size] batch_results = await process_batch(batch, client) results.extend(batch_results) for prompt, result in zip(prompts, results): print(f"Prompt: promptnResponse: resultn") asyncio.run(main())
Concurrency Control: While asynchronous programming allows for concurrent execution, it’s important to control the level of concurrency to avoid overwhelming the API server or exceeding rate limits. We can use asyncio.Semaphore for this purpose.
import asyncio from openai import AsyncOpenAI async def generate_text(prompt, client, semaphore): async with semaphore: response = await client.chat.completions.create( model="gpt-3.5-turbo", messages=["role": "user", "content": prompt] ) return response.choices[0].message.content async def main(): prompts = [f"Tell me a fact about number i" for i in range(100)] max_concurrent_requests = 5 semaphore = asyncio.Semaphore(max_concurrent_requests) async with AsyncOpenAI() as client: tasks = [generate_text(prompt, client, semaphore) for prompt in prompts] results = await asyncio.gather(*tasks) for prompt, result in zip(prompts, results): print(f"Prompt: promptnResponse: resultn") asyncio.run(main())
In this example, we use a semaphore to limit the number of concurrent requests to 5, ensuring we don’t overwhelm the API server.
Error Handling and Retries in Async LLM Calls
When working with external APIs, it’s crucial to implement robust error handling and retry mechanisms. Let’s enhance our code to handle common errors and implement exponential backoff for retries.
import asyncio import random from openai import AsyncOpenAI from tenacity import retry, stop_after_attempt, wait_exponential class APIError(Exception): pass @retry(stop=stop_after_attempt(3), wait=wait_exponential(multiplier=1, min=4, max=10)) async def generate_text_with_retry(prompt, client): try: response = await client.chat.completions.create( model="gpt-3.5-turbo", messages=["role": "user", "content": prompt] ) return response.choices[0].message.content except Exception as e: print(f"Error occurred: e") raise APIError("Failed to generate text") async def process_prompt(prompt, client, semaphore): async with semaphore: try: result = await generate_text_with_retry(prompt, client) return prompt, result except APIError: return prompt, "Failed to generate response after multiple attempts." async def main(): prompts = [f"Tell me a fact about number i" for i in range(20)] max_concurrent_requests = 5 semaphore = asyncio.Semaphore(max_concurrent_requests) async with AsyncOpenAI() as client: tasks = [process_prompt(prompt, client, semaphore) for prompt in prompts] results = await asyncio.gather(*tasks) for prompt, result in results: print(f"Prompt: promptnResponse: resultn") asyncio.run(main())
This enhanced version includes:
A custom APIError exception for API-related errors.
A generate_text_with_retry function decorated with @retry from the tenacity library, implementing exponential backoff.
Error handling in the process_prompt function to catch and report failures.
Optimizing Performance: Streaming Responses
For long-form content generation, streaming responses can significantly improve the perceived performance of your application. Instead of waiting for the entire response, you can process and display chunks of text as they become available.
import asyncio from openai import AsyncOpenAI async def stream_text(prompt, client): stream = await client.chat.completions.create( model="gpt-3.5-turbo", messages=["role": "user", "content": prompt], stream=True ) full_response = "" async for chunk in stream: if chunk.choices[0].delta.content is not None: content = chunk.choices[0].delta.content full_response += content print(content, end='', flush=True) print("n") return full_response async def main(): prompt = "Write a short story about a time-traveling scientist." async with AsyncOpenAI() as client: result = await stream_text(prompt, client) print(f"Full response:nresult") asyncio.run(main())
This example demonstrates how to stream the response from the API, printing each chunk as it arrives. This approach is particularly useful for chat applications or any scenario where you want to provide real-time feedback to the user.
Building Async Workflows with LangChain
For more complex LLM-powered applications, the LangChain framework provides a high-level abstraction that simplifies the process of chaining multiple LLM calls and integrating other tools. Let’s look at an example of using LangChain with async capabilities:
This example shows how LangChain can be used to create more complex workflows with streaming and asynchronous execution. The AsyncCallbackManager and StreamingStdOutCallbackHandler enable real-time streaming of the generated content.
import asyncio from langchain.llms import OpenAI from langchain.prompts import PromptTemplate from langchain.chains import LLMChain from langchain.callbacks.manager import AsyncCallbackManager from langchain.callbacks.streaming_stdout import StreamingStdOutCallbackHandler async def generate_story(topic): llm = OpenAI(temperature=0.7, streaming=True, callback_manager=AsyncCallbackManager([StreamingStdOutCallbackHandler()])) prompt = PromptTemplate( input_variables=["topic"], template="Write a short story about topic." ) chain = LLMChain(llm=llm, prompt=prompt) return await chain.arun(topic=topic) async def main(): topics = ["a magical forest", "a futuristic city", "an underwater civilization"] tasks = [generate_story(topic) for topic in topics] stories = await asyncio.gather(*tasks) for topic, story in zip(topics, stories): print(f"nTopic: topicnStory: storyn'='*50n") asyncio.run(main())
Serving Async LLM Applications with FastAPI
To make your async LLM application available as a web service, FastAPI is an great choice due to its native support for asynchronous operations. Here’s an example of how to create a simple API endpoint for text generation:
from fastapi import FastAPI, BackgroundTasks from pydantic import BaseModel from openai import AsyncOpenAI app = FastAPI() client = AsyncOpenAI() class GenerationRequest(BaseModel): prompt: str class GenerationResponse(BaseModel): generated_text: str @app.post("/generate", response_model=GenerationResponse) async def generate_text(request: GenerationRequest, background_tasks: BackgroundTasks): response = await client.chat.completions.create( model="gpt-3.5-turbo", messages=["role": "user", "content": request.prompt] ) generated_text = response.choices[0].message.content # Simulate some post-processing in the background background_tasks.add_task(log_generation, request.prompt, generated_text) return GenerationResponse(generated_text=generated_text) async def log_generation(prompt: str, generated_text: str): # Simulate logging or additional processing await asyncio.sleep(2) print(f"Logged: Prompt 'prompt' generated text of length len(generated_text)") if __name__ == "__main__": import uvicorn uvicorn.run(app, host="0.0.0.0", port=8000)
This FastAPI application creates an endpoint /generate that accepts a prompt and returns generated text. It also demonstrates how to use background tasks for additional processing without blocking the response.
Best Practices and Common Pitfalls
As you work with async LLM APIs, keep these best practices in mind:
Use connection pooling: When making multiple requests, reuse connections to reduce overhead.
Implement proper error handling: Always account for network issues, API errors, and unexpected responses.
Respect rate limits: Use semaphores or other concurrency control mechanisms to avoid overwhelming the API.
Monitor and log: Implement comprehensive logging to track performance and identify issues.
Use streaming for long-form content: It improves user experience and allows for early processing of partial results.
#API#APIs#app#applications#approach#Article#Articles#artificial#Artificial Intelligence#asynchronous programming#asyncio#background#Building#code#col#complexity#comprehensive#computing#Concurrency#concurrency control#content#Delay#developers#display#endpoint#Environment#error handling#event#FastAPI#forest
0 notes
Text
Creating a Machine Learning-Powered Recommendation System with FastAPI and Scikit-learn
🔍 Interested in building a recommendation engine? Learn how to use FastAPI and Scikit-learn to create and deploy a machine learning-powered recommendation system as a REST API. We offer help with:
Setting up FastAPI and Scikit-learn.
Developing the recommendation model.
Deploying your system as a REST API.
💬 Contact us on WhatsApp at +971501618774 for personalized support!
0 notes
Text
FastAPI Authentication Security with Token: A Comprehensive Guide
Benefit from the collective wisdom of the FastAPI community. August Infotech shares community insights, tips, and tricks to enhance your understanding of FastAPI and stay abreast of the latest developments in the ecosystem.
FastAPI is a powerful tool, and with the right knowledge, you can harness its potential to build secure and efficient APIs. August Infotech's comprehensive guide is your go-to resource for mastering FastAPI authentication and security. Elevate your development skills, fortify your applications, and stay ahead of the curve with this must-read guide.
Ready to embark on a journey towards secure and performant FastAPI applications? Read the full guide here: https://bit.ly/48yyyk1
#WebDevelopment #APIs #TokenAuthentication #Python #TechBlog
0 notes
Text
Learn Ruby on Rails with The Pragmatic Programmers Book Bundle!
Learn Ruby on Rails with The Pragmatic Programmers Book Bundle! #sale #ruby #rubyonrails #rails #microservices #book #education #learning #coding #programming #software #ebook
Check out the three book bundle options at this link. Want to write powerful, easy-to-maintain code with the Ruby programming language? Looking to create great web apps quickly with Rails? This bundle of books from the experts at Pragmatic Programmers will get you on track! Explore topics like metaprogramming, optimization, testing, sustainable development, and much more, and help support Active…
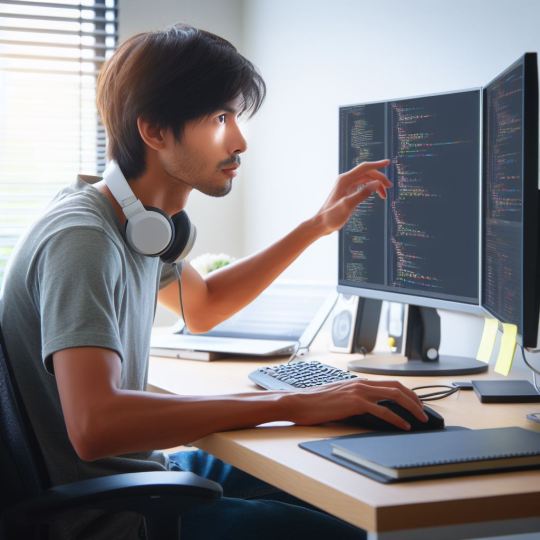
View On WordPress
#book#books#coding#ebook#ebooks#education#fastapi#humble bundle#microservices#programmer#programming#python#ruby#ruby on rails#sale#software#swift
0 notes
Text
ใช้ FastAPI ให้เร็วขึ้นถึงเกือบเท่า Go Gin
จากบทความก่อนหน้านี้ Python ก็เร็วเท่า GoLang ได้ จริงไหม? เรามาทำให้ FastAPI ของเราให้เร็วขึ้นถึงเกือบเท่า Go Gin ในบทความนี้ จะทำการใช้ Docker เข้ามาช่วยในการทดสอบความเร็วในการประมวลผล ระหว่าง Python3.11 pypy3.10 และ Go1.20.6 นะครับ โดย Docker จะถึงตั้งค่าไว้แบบเดี่ยวกัน และใช้ โค๊ต การทำงานแบบเดียวกัน โค๊ตที่ใช้ในการทดสอบด้วย FastAPI import timeit import platform from fastapi import…

View On WordPress
1 note
·
View note
Text
FASTAPI: A Simple Guide with Installation Steps
FASTAPI is a modern, fast (high-performance), web framework for building APIs with Python. It is known for its simplicity, scalability, and speed. In this article, we will walk you through the installation process and provide a basic understanding of how to get started with FASTAPI. Installation Steps To begin with FASTAPI, you need to have Python installed on your system. Make sure you have…

View On WordPress
0 notes
Link
This week's issue contains some of the most interesting articles and news, selected from all the content published in the previous week on the Developers News website. In this issue you will read about, Container Face-Off, React 18 with Redux, Continuous Testing, Kubernetes vs OpenStack, Tech Books, Go Pointer, FastAPI, Helm, SQL JOIN, and more
#devs_news_weekly#devs_news#react#javascript#kubernetes#OpenStack#go#FastAPI#Helm#book#sql#container#microservices#redux
0 notes
Text
Programming Update: Jan 2023 and Feb 2023
Programming starts off a bit slow in 2023, but then really takes off in February. #Golang finally really starts making sense to me and I reimplement an API in Go.
January January was a relatively light programming month for me. I was focused on finishing up end of year blog posts and other tasks. Since Lastfmeoystats is used to generate the stats I need for my end of year music post, I worked on it a little to make some fixes. The biggest fix was to change the chart titles not to be hardcoded. I didn’t realize it until I was reviewing my blog post, but I…
View On WordPress
0 notes
Text

#NoCodeAPI platforms are a new breed of API management tools that don't require any coding. They provide a low-code or no-code interface for developers to easily create, manage, and publish APIs. The #NoCodeAPI can be used to create #RESTful or #SoapBasedAPIs.
#APIs#NoCode#API#RestAPI#GoogleMapsAPI#FastAPI#OpenAPI#Restful#LocalStorage#JavaAPI#NotionAPI#PostmanAPI#GoogleTranslateAPI#GoogleAPIs#SoapAPI#GoogleDriveAPI#GraphAPI#GmailAPI#FreeAPI#NoCodeAPIs#RESTfulAPIs
0 notes
Text
Man why is working with web apps so obnoxious. "422 unprocessable entity teehee. Good luck figuring out which entity it is, much less why I refuse to process it"
#i almost went apeshit when fastapi refused to take a numpy int because it WOULDN'T TELL ME WHICH VARIABLE WAS THE PROBLEM#luckily i realized i could put a breakpoint right into my python code before i went entirely off the deep end#programming
10 notes
·
View notes
Text
Building APIs with FastAPI
FastAPI is quickly becoming the go-to framework for building APIs in Python. Its ease of use and performance make it perfect for modern web applications. Learn how to create robust APIs with FastAPI! #FastAPI #APIs #Python
For more details, contact us at: 📞 WhatsApp: +971 50 161 8774 📧 Email: [email protected]
0 notes
Photo




Flask vs FastAPI:
WHAT'S BETTER FOR APP DEVELOPMENT?
Which Should You Choose?
Flask and FastAPI are popular micro-frameworks for developing small-scale data science and machine learning websites and applications..
1 note
·
View note
Text
Top 10 Interview Questions for Python FASTAPI
Top 10 interview questions for FASTAPI with their answers in one line: 1. What is FASTAPI and how does it differ from other Python web frameworks? – FASTAPI is a modern, high-performance Python web framework that leverages type hints and asynchronous programming for fast development and scalability. 2. Explain the main features and advantages of using FASTAPI. – FASTAPI provides automatic API…

View On WordPress
0 notes
Link
In this step-by-step guide, we will take a deep dive into building a map-based application to show the customer's prices of gas stations around them. We will cover the different key steps of a product, from original proof of concept (POC) to the minimum viable product (MVP).
#docker#postgresql#Docker#map_based_application#FastAPI#python#docker_compose#location_based_service
0 notes