#Kubernetes For Machine Learning
Explore tagged Tumblr posts
Text
Understand how Generative AI is accelerating Kubernetes adoption, shaping industries with scalable, automated, and innovative approaches.
#AI Startups Kubernetes#Enterprise AI With Kubernetes#Generative AI#Kubernetes AI Architecture#Kubernetes For AI Model Deployment#Kubernetes For Deep Learning#Kubernetes For Machine Learning
0 notes
Text
How Is Gen AI Driving Kubernetes Demand Across Industries?
Understand how Generative AI is accelerating Kubernetes adoption, shaping industries with scalable, automated, and innovative approaches. A new breakthrough in AI, called generative AI or Gen AI, is creating incredible waves across industries and beyond. With this technology rapidly evolving there is growing pressure on the available structure to support both the deployment and scalability of…
#AI Startups Kubernetes#Enterprise AI With Kubernetes#Generative AI#Kubernetes AI Architecture#Kubernetes For AI Model Deployment#Kubernetes For Deep Learning#Kubernetes For Machine Learning
0 notes
Text
How Is Gen AI Driving Kubernetes Demand Across Industries?
Unveil how Gen AI is pushing Kubernetes to the forefront, delivering industry-specific solutions with precision and scalability.
Original Source: https://bit.ly/4cPS7G0
A new breakthrough in AI, called generative AI or Gen AI, is creating incredible waves across industries and beyond. With this technology rapidly evolving there is growing pressure on the available structure to support both the deployment and scalability of the technology. Kubernetes, an effective container orchestration platform is already indicating its ability as one of the enablers in this context. This article critically analyzes how Generative AI gives rise to the use of Kubernetes across industries with a focus of the coexistence of these two modern technological forces.
The Rise of Generative AI and Its Impact on Technology
Machine learning has grown phenomenally over the years and is now foundational in various industries including healthcare, banking, production as well as media and entertainment industries. This technology whereby an AI model is trained to write, design or even solve business problems is changing how business is done. Gen AI’s capacity to generate new data and solutions independently has opened opportunities for advancements as has never been seen before.
If companies are adopting Generative AI , then the next big issue that they are going to meet is on scalability of models and its implementation. These resource- intensive applications present a major challenge to the traditional IT architectures. It is here that Kubernetes comes into the picture, which provides solutions to automate deployment, scaling and managing the containerised applications. Kubernetes may be deployed to facilitate the ML and deep learning processing hence maximizing the efficiency of the AI pipeline to support the future growth of Gen AI applications.
The Intersection of Generative AI and Kubernetes
The integration of Generative AI and Kubernetes is probably the most significant traffic in the development of AI deployment approaches. Kubernetes is perfect for the dynamics of AI workloads in terms of scalability and flexibility. The computation of Gen AI models demands considerable resources, and Kubernetes has all the tools required to properly orchestrate those resources for deploying AI models in different setups.
Kubernetes’ infrastructure is especially beneficial for AI startups and companies that plan to use Generative AI. It enables the decentralization of workload among several nodes so that training, testing, and deployment of AI models are highly distributed. This capability is especially important for businesses that require to constantly revolve their models to adapt to competition. In addition, Kubernetes has direct support for GPU, which helps in evenly distributing computational intensity that comes with deep learning workloads thereby making it perfect for AI projects.
Key Kubernetes Features that Enable Efficient Generative AI Deployment
Scalability:
Kubernetes excels at all levels but most notably where applications are scaled horizontally. Especially for Generative AI which often needs a lot of computation, Kubernetes is capable of scaling the pods, the instances of the running processes and provide necessary resources for the workload claims without having any human intervention.
Resource Management:
Effort is required to be allocated efficiently so as to perform the AI workloads. Kubernetes assists in deploying as well as allocating resources within the cluster from where the AI models usually operate while ensuring that resource consumption and distribution is efficiently controlled.
Continuous Deployment and Integration (CI/CD):
Kubernetes allows for the execution of CI CD pipelines which facilitate contingency integration as well as contingency deployment of models. This is essential for enterprises and the AI startups that use the flexibility of launching different AI solutions depending on the current needs of their companies.
GPU Support:
Kubernetes also features the support of the GPUs for the applications in deep learning from scratch that enhances the rate of training and inference of the models of AI. It is particularly helpful for AI applications that require more data processing, such as image and speech recognition.
Multi-Cloud and Hybrid Cloud Support:
The fact that the Kubernetes can operate in several cloud environment and on-premise data centers makes it versatile as AI deployment tool. It will benefit organizations that need a half and half cloud solution and organizations that do not want to be trapped in the web of the specific company.
Challenges of Running Generative AI on Kubernetes
Complexity of Setup and Management:
That aid Kubernetes provides a great platform for AI deployments comes at the cost of operational overhead. Deploying and configuring a Kubernetes Cluster for AI based workloads therefore necessitates knowledge of both Kubernetes and the approach used to develop these models. This could be an issue for organizations that are not able to gather or hire the required expertise.
Resource Constraints:
Generative AI models require a lot of computing power and when running them in a Kubernetes environment, the computational resources can be fully utilised. AI works best when the organizational resources are well managed to ensure that there are no constraints in the delivery of the application services.
Security Concerns:
Like it is the case with any cloud-native application, security is a big issue when it comes to running artificial intelligence models on Kubernetes. Security of the data and models that AI employs needs to be protected hence comes the policies of encryption, access control and monitoring.
Data Management:
Generative AI models make use of multiple dataset samples for its learning process and is hard to deal with the concept in Kubernetes. Managing these datasets as well as accessing and processing them in a manner that does not hinder the overall performance of an organization is often a difficult task.
Conclusion: The Future of Generative AI is Powered by Kubernetes
As Generative AI advances and integrates into many sectors, the Kubernetes efficient and scalable solutions will only see a higher adoption rate. Kubernetes is a feature of AI architectures that offer resources and facilities for the development and management of AI model deployment.
If you’re an organization planning on putting Generative AI to its best use, then adopting Kubernetes is non-negotiable. Mounting the AI workloads, utilizing the resources in the best possible manner, and maintaining the neat compatibility across the multiple and different clouds are some of the key solutions provided by Kubernetes for the deployment of the AI models. With continued integration between Generative AI and Kubernetes, we have to wonder what new and exciting uses and creations are yet to come, thus strengthening Kubernetes’ position as the backbone for enterprise AI with Kubernetes. The future is bright that Kubernetes is playing leading role in this exciting technological revolution of AI.
Original Source: https://bit.ly/4cPS7G0
#AI Startups Kubernetes#Enterprise AI With Kubernetes#Generative AI#Kubernetes AI Architecture#Kubernetes For AI Model Deployment#Kubernetes For Deep Learning#Kubernetes For Machine Learning
0 notes
Text
Most in-demand Azure cloud skills that IT professionals should have
In today’s competitive job market, having the right skillset is key to success. Azure cloud skills are becoming increasingly important for IT professionals and developers. With the help of Azure cloud services, organizations can build secure and reliable applications that can scale up or down as needed. Azure cloud skills can be used to develop applications that run on the cloud, manage data, automate processes and deploy solutions quickly and efficiently. It also allows developers to create hybrid solutions by combining on-premise resources with public cloud offerings. Having the right Azure cloud skills can open up new opportunities for IT professionals in terms of career growth and salary potential. It is essential for IT professionals to stay up-to-date with the latest developments in this field in order to remain competitive in this ever-evolving job market. The following are some of the most in-demand Azure cloud skills that IT professionals should have:
1. Azure cloud services: This includes developing applications that run on Microsoft Azure and managing data, automating processes and deploying solutions quickly and efficiently.
2. Azure IaaS: Determines how an organization can build secure, reliable applications by using public cloud resources in combination with on-premise resources to create hybrid solutions for maximum efficiency.
3. Windows Server: In order to develop solutions for either public or private clouds, developers need familiarity with this server operating system which includes Microsoft Azure hybrid solutions.
4. Windows: Determines how an organization can harness the power of the cloud by leveraging the powerful development toolset for this platform and its wide range of applications.
#Azure#Cloud#Cloud computing#Microsoft Azure#Azure Active Directory#Azure Virtual Machines#Azure Storage#Azure Networking#Azure DevOps#Azure Kubernetes Service#Azure SQL#Azure Machine Learning#Azure Automation#Azure Security#Azure IoT#Azure Functions#Azure Logic Apps#Azure App Service#Azure ExpressRoute#Azure Monitor#Azure Cost Management#Azure Backup#Azure Site Recovery#Azure AD B2C#Azure AD B2B
2 notes
·
View notes
Text
Penggunaan Cloud Computing dalam Pengembangan AI: Alat dan Platform yang Meningkatkan Efisiensi
Cloud computing telah menjadi salah satu inovasi teknologi paling signifikan dalam dekade terakhir. Dalam pengembangan kecerdasan buatan (AI), peran cloud computing sangat penting karena memungkinkan pengembang untuk memanfaatkan infrastruktur dan layanan yang fleksibel, skalabel, dan hemat biaya. Dengan semakin kompleksnya algoritma AI dan meningkatnya kebutuhan akan data besar (big data), cloud…
#AI democratization#AI development#AI generative models#AI in e-commerce#AI in healthcare#AI in manufacturing#AI platforms#AI trends 2025#artificial intelligence#cloud computing#cloud computing benefits#cloud-based AI tools#cloud-native AI#edge computing#efficiency in AI#future of AI#Kubernetes for AI#machine learning#quantum computing#serverless computing
0 notes
Text
Visit here learn these Tools - Online or Offline
#machine learning#linux#docker#kubernetes#computer science#programming#ai tools#software engineering#application#education#career#datascience#data analytics#artificial intelligence
1 note
·
View note
Text
Developer Environment Presentation 1 Part 2: Generate Bootstrap Configuration Files, and Uninstall Previous Jannah Installation
From cloning the operator from github.com. To generating new Molecule configuration, environment variable files, for Jannah deployment. And uninstall previous Jannah installation.
Video Highlights Purpose is to showcase the developer environment (day to day developer experience). I performed the following steps: Clone the operator code base, from git. git clone https://github.com/jannahio/operator; Change into the cloned operator directory: cd operator; Operator configuration needs environment variables file to bootstrap. So copied environment variables file into…
View On WordPress
#ansible#artificial#backend#Commands#Configuration#container#Data#Developer#devOps#django#docker#Environment#Files#Intelligence#Jannah#Kubernetes#learning#Logs#machine#makefile#model#Module#molecule#operator#Package#Playbook#python#Science#software#ux
0 notes
Text
Future of DevOps in 2023 and beyond
DevOps has rapidly become a critical part of software development in recent years, providing a seamless process that allows development and operations teams to work together seamlessly to deliver quality software faster.
As we look ahead to 2023 and beyond, the future of DevOps continues to evolve and become more complex, as technology and processes continue to advance. In this article, we’ll explore the latest trends and innovations in DevOps, and what they mean for the future of software development.
Artificial Intelligence and Machine Learning
Artificial Intelligence (AI) and Machine Learning (ML) have already begun to play a big role in DevOps, and they will continue to do so in the future. AI and ML are already being used in DevOps to automate repetitive tasks, monitor applications, and identify performance issues. In the future, we can expect to see even more sophisticated AI and ML systems that will be able to analyze and predict software behavior, detect security vulnerabilities, and respond to incidents automatically.
This will lead to faster and more efficient software development, freeing up developers to focus on more strategic work, such as improving software performance and addressing customer needs. Additionally, AI and ML will help organizations to identify and resolve problems before they occur, reducing downtime and minimizing the impact of outages.

Containers and Kubernetes
Containers and Kubernetes have rapidly become an essential part of DevOps, allowing teams to easily deploy, scale, and manage applications. In the future, we can expect to see more organizations adopt containers and Kubernetes, as they continue to provide a more efficient and scalable solution compared to traditional virtualization methods.
Additionally, as the number of containers and microservices grows, Kubernetes will play a crucial role in managing the deployment and scaling of these applications. In the future, we can expect to see the development of more advanced Kubernetes tools and platforms, allowing organizations to manage large-scale container deployments with ease.
Cloud Computing
Cloud computing has already had a significant impact on DevOps, allowing organizations to quickly and easily scale their infrastructure and resources as needed. In the future, we can expect to see even more organizations adopt cloud computing, as they continue to offer a more flexible, cost-effective, and scalable solution compared to traditional on-premise solutions.
Additionally, we can expect to see the development of new cloud computing services and technologies that will further improve the efficiency and speed of DevOps. For example, the use of serverless computing and edge computing will allow organizations to run applications and services closer to the edge, reducing latency and improving performance.
Collaboration and Communication
Collaboration and communication are key to the success of DevOps, and in the future, we can expect to see even more emphasis placed on these areas. As software development becomes more complex and global, organizations will need to improve their collaboration and communication processes to ensure that development and operations teams are working together effectively.
In the future, we can expect to see the development of new collaboration and communication tools and platforms, such as chatbots and virtual reality, that will allow teams to work together more efficiently and effectively. Additionally, we can expect to see the integration of DevOps tools and platforms with existing enterprise systems, such as CRM and ERP, to improve communication and collaboration across the organization.
Conclusion
In conclusion, the future of DevOps is bright, with many exciting new technologies and trends emerging. As technology and processes continue to advance, we can expect to see even more sophisticated DevOps systems that will allow organizations to deliver high-quality software faster and more efficiently.
However, while technology is important, it is also crucial that organizations focus on improving collaboration.
#devops#kubernetes#cloud computing#Kubernetes#machine learning#artificial intelligence#advisedskills
0 notes
Text
Top Trends in Software Development for 2025
The software development industry is evolving at an unprecedented pace, driven by advancements in technology and the increasing demands of businesses and consumers alike. As we step into 2025, staying ahead of the curve is essential for businesses aiming to remain competitive. Here, we explore the top trends shaping the software development landscape and how they impact businesses. For organizations seeking cutting-edge solutions, partnering with the Best Software Development Company in Vadodara, Gujarat, or India can make all the difference.
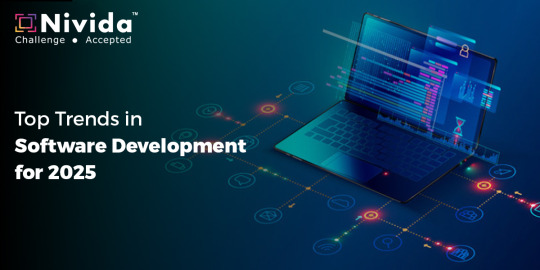
1. Artificial Intelligence and Machine Learning Integration:
Artificial Intelligence (AI) and Machine Learning (ML) are no longer optional but integral to modern software development. From predictive analytics to personalized user experiences, AI and ML are driving innovation across industries. In 2025, expect AI-powered tools to streamline development processes, improve testing, and enhance decision-making.
Businesses in Gujarat and beyond are leveraging AI to gain a competitive edge. Collaborating with the Best Software Development Company in Gujarat ensures access to AI-driven solutions tailored to specific industry needs.
2. Low-Code and No-Code Development Platforms:
The demand for faster development cycles has led to the rise of low-code and no-code platforms. These platforms empower non-technical users to create applications through intuitive drag-and-drop interfaces, significantly reducing development time and cost.
For startups and SMEs in Vadodara, partnering with the Best Software Development Company in Vadodara ensures access to these platforms, enabling rapid deployment of business applications without compromising quality.
3. Cloud-Native Development:
Cloud-native technologies, including Kubernetes and microservices, are becoming the backbone of modern applications. By 2025, cloud-native development will dominate, offering scalability, resilience, and faster time-to-market.
The Best Software Development Company in India can help businesses transition to cloud-native architectures, ensuring their applications are future-ready and capable of handling evolving market demands.
4. Edge Computing:
As IoT devices proliferate, edge computing is emerging as a critical trend. Processing data closer to its source reduces latency and enhances real-time decision-making. This trend is particularly significant for industries like healthcare, manufacturing, and retail.
Organizations seeking to leverage edge computing can benefit from the expertise of the Best Software Development Company in Gujarat, which specializes in creating applications optimized for edge environments.
5. Cybersecurity by Design:
With the increasing sophistication of cyber threats, integrating security into the development process has become non-negotiable. Cybersecurity by design ensures that applications are secure from the ground up, reducing vulnerabilities and protecting sensitive data.
The Best Software Development Company in Vadodara prioritizes cybersecurity, providing businesses with robust, secure software solutions that inspire trust among users.
6. Blockchain Beyond Cryptocurrencies:
Blockchain technology is expanding beyond cryptocurrencies into areas like supply chain management, identity verification, and smart contracts. In 2025, blockchain will play a pivotal role in creating transparent, tamper-proof systems.
Partnering with the Best Software Development Company in India enables businesses to harness blockchain technology for innovative applications that drive efficiency and trust.
7. Progressive Web Apps (PWAs):
Progressive Web Apps (PWAs) combine the best features of web and mobile applications, offering seamless experiences across devices. PWAs are cost-effective and provide offline capabilities, making them ideal for businesses targeting diverse audiences.
The Best Software Development Company in Gujarat can develop PWAs tailored to your business needs, ensuring enhanced user engagement and accessibility.
8. Internet of Things (IoT) Expansion:
IoT continues to transform industries by connecting devices and enabling smarter decision-making. From smart homes to industrial IoT, the possibilities are endless. In 2025, IoT solutions will become more sophisticated, integrating AI and edge computing for enhanced functionality.
For businesses in Vadodara and beyond, collaborating with the Best Software Development Company in Vadodara ensures access to innovative IoT solutions that drive growth and efficiency.
9. DevSecOps:
DevSecOps integrates security into the DevOps pipeline, ensuring that security is a shared responsibility throughout the development lifecycle. This approach reduces vulnerabilities and ensures compliance with industry standards.
The Best Software Development Company in India can help implement DevSecOps practices, ensuring that your applications are secure, scalable, and compliant.
10. Sustainability in Software Development:
Sustainability is becoming a priority in software development. Green coding practices, energy-efficient algorithms, and sustainable cloud solutions are gaining traction. By adopting these practices, businesses can reduce their carbon footprint and appeal to environmentally conscious consumers.
Working with the Best Software Development Company in Gujarat ensures access to sustainable software solutions that align with global trends.
11. 5G-Driven Applications:
The rollout of 5G networks is unlocking new possibilities for software development. Ultra-fast connectivity and low latency are enabling applications like augmented reality (AR), virtual reality (VR), and autonomous vehicles.
The Best Software Development Company in Vadodara is at the forefront of leveraging 5G technology to create innovative applications that redefine user experiences.
12. Hyperautomation:
Hyperautomation combines AI, ML, and robotic process automation (RPA) to automate complex business processes. By 2025, hyperautomation will become a key driver of efficiency and cost savings across industries.
Partnering with the Best Software Development Company in India ensures access to hyperautomation solutions that streamline operations and boost productivity.
13. Augmented Reality (AR) and Virtual Reality (VR):
AR and VR technologies are transforming industries like gaming, education, and healthcare. In 2025, these technologies will become more accessible, offering immersive experiences that enhance learning, entertainment, and training.
The Best Software Development Company in Gujarat can help businesses integrate AR and VR into their applications, creating unique and engaging user experiences.
Conclusion:
The software development industry is poised for significant transformation in 2025, driven by trends like AI, cloud-native development, edge computing, and hyperautomation. Staying ahead of these trends requires expertise, innovation, and a commitment to excellence.
For businesses in Vadodara, Gujarat, or anywhere in India, partnering with the Best Software Development Company in Vadodara, Gujarat, or India ensures access to cutting-edge solutions that drive growth and success. By embracing these trends, businesses can unlock new opportunities and remain competitive in an ever-evolving digital landscape.
#Best Software Development Company in Vadodara#Best Software Development Company in Gujarat#Best Software Development Company in India#nividasoftware
3 notes
·
View notes
Text
How To Use Llama 3.1 405B FP16 LLM On Google Kubernetes
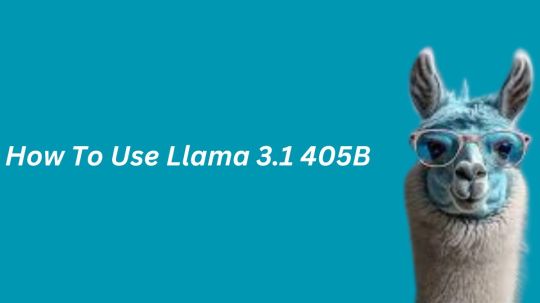
How to set up and use large open models for multi-host generation AI over GKE
Access to open models is more important than ever for developers as generative AI grows rapidly due to developments in LLMs (Large Language Models). Open models are pre-trained foundational LLMs that are accessible to the general population. Data scientists, machine learning engineers, and application developers already have easy access to open models through platforms like Hugging Face, Kaggle, and Google Cloud’s Vertex AI.
How to use Llama 3.1 405B
Google is announcing today the ability to install and run open models like Llama 3.1 405B FP16 LLM over GKE (Google Kubernetes Engine), as some of these models demand robust infrastructure and deployment capabilities. With 405 billion parameters, Llama 3.1, published by Meta, shows notable gains in general knowledge, reasoning skills, and coding ability. To store and compute 405 billion parameters at FP (floating point) 16 precision, the model needs more than 750GB of GPU RAM for inference. The difficulty of deploying and serving such big models is lessened by the GKE method discussed in this article.
Customer Experience
You may locate the Llama 3.1 LLM as a Google Cloud customer by selecting the Llama 3.1 model tile in Vertex AI Model Garden.
Once the deploy button has been clicked, you can choose the Llama 3.1 405B FP16 model and select GKE.Image credit to Google Cloud
The automatically generated Kubernetes yaml and comprehensive deployment and serving instructions for Llama 3.1 405B FP16 are available on this page.
Deployment and servicing multiple hosts
Llama 3.1 405B FP16 LLM has significant deployment and service problems and demands over 750 GB of GPU memory. The total memory needs are influenced by a number of parameters, including the memory used by model weights, longer sequence length support, and KV (Key-Value) cache storage. Eight H100 Nvidia GPUs with 80 GB of HBM (High-Bandwidth Memory) apiece make up the A3 virtual machines, which are currently the most potent GPU option available on the Google Cloud platform. The only practical way to provide LLMs such as the FP16 Llama 3.1 405B model is to install and serve them across several hosts. To deploy over GKE, Google employs LeaderWorkerSet with Ray and vLLM.
LeaderWorkerSet
A deployment API called LeaderWorkerSet (LWS) was created especially to meet the workload demands of multi-host inference. It makes it easier to shard and run the model across numerous devices on numerous nodes. Built as a Kubernetes deployment API, LWS is compatible with both GPUs and TPUs and is independent of accelerators and the cloud. As shown here, LWS uses the upstream StatefulSet API as its core building piece.
A collection of pods is controlled as a single unit under the LWS architecture. Every pod in this group is given a distinct index between 0 and n-1, with the pod with number 0 being identified as the group leader. Every pod that is part of the group is created simultaneously and has the same lifecycle. At the group level, LWS makes rollout and rolling upgrades easier. For rolling updates, scaling, and mapping to a certain topology for placement, each group is treated as a single unit.
Each group’s upgrade procedure is carried out as a single, cohesive entity, guaranteeing that every pod in the group receives an update at the same time. While topology-aware placement is optional, it is acceptable for all pods in the same group to co-locate in the same topology. With optional all-or-nothing restart support, the group is also handled as a single entity when addressing failures. When enabled, if one pod in the group fails or if one container within any of the pods is restarted, all of the pods in the group will be recreated.
In the LWS framework, a group including a single leader and a group of workers is referred to as a replica. Two templates are supported by LWS: one for the workers and one for the leader. By offering a scale endpoint for HPA, LWS makes it possible to dynamically scale the number of replicas.
Deploying multiple hosts using vLLM and LWS
vLLM is a well-known open source model server that uses pipeline and tensor parallelism to provide multi-node multi-GPU inference. Using Megatron-LM’s tensor parallel technique, vLLM facilitates distributed tensor parallelism. With Ray for multi-node inferencing, vLLM controls the distributed runtime for pipeline parallelism.
By dividing the model horizontally across several GPUs, tensor parallelism makes the tensor parallel size equal to the number of GPUs at each node. It is crucial to remember that this method requires quick network connectivity between the GPUs.
However, pipeline parallelism does not require continuous connection between GPUs and divides the model vertically per layer. This usually equates to the quantity of nodes used for multi-host serving.
In order to support the complete Llama 3.1 405B FP16 paradigm, several parallelism techniques must be combined. To meet the model’s 750 GB memory requirement, two A3 nodes with eight H100 GPUs each will have a combined memory capacity of 1280 GB. Along with supporting lengthy context lengths, this setup will supply the buffer memory required for the key-value (KV) cache. The pipeline parallel size is set to two for this LWS deployment, while the tensor parallel size is set to eight.
In brief
We discussed in this blog how LWS provides you with the necessary features for multi-host serving. This method maximizes price-to-performance ratios and can also be used with smaller models, such as the Llama 3.1 405B FP8, on more affordable devices. Check out its Github to learn more and make direct contributions to LWS, which is open-sourced and has a vibrant community.
You can visit Vertex AI Model Garden to deploy and serve open models via managed Vertex AI backends or GKE DIY (Do It Yourself) clusters, as the Google Cloud Platform assists clients in embracing a gen AI workload. Multi-host deployment and serving is one example of how it aims to provide a flawless customer experience.
Read more on Govindhtech.com
#Llama3.1#Llama#LLM#GoogleKubernetes#GKE#405BFP16LLM#AI#GPU#vLLM#LWS#News#Technews#Technology#Technologynews#Technologytrends#govindhtech
2 notes
·
View notes
Text
Unveil how Gen AI is pushing Kubernetes to the forefront, delivering industry-specific solutions with precision and scalability.
#AI Startups Kubernetes#Enterprise AI With Kubernetes#Generative AI#Kubernetes AI Architecture#Kubernetes For AI Model Deployment#Kubernetes For Deep Learning#Kubernetes For Machine Learning
0 notes
Text
What are the latest trends in the IT job market?
Introduction
The IT job market is changing quickly. This change is because of new technology, different employer needs, and more remote work.
For jobseekers, understanding these trends is crucial to positioning themselves as strong candidates in a highly competitive landscape.
This blog looks at the current IT job market. It offers insights into job trends and opportunities. You will also find practical strategies to improve your chances of getting your desired role.
Whether you’re in the midst of a job search or considering a career change, this guide will help you navigate the complexities of the job hunting process and secure employment in today’s market.
Section 1: Understanding the Current IT Job Market
Recent Trends in the IT Job Market
The IT sector is booming, with consistent demand for skilled professionals in various domains such as cybersecurity, cloud computing, and data science.
The COVID-19 pandemic accelerated the shift to remote work, further expanding the demand for IT roles that support this transformation.
Employers are increasingly looking for candidates with expertise in AI, machine learning, and DevOps as these technologies drive business innovation.
According to industry reports, job opportunities in IT will continue to grow, with the most substantial demand focused on software development, data analysis, and cloud architecture.
It’s essential for jobseekers to stay updated on these trends to remain competitive and tailor their skills to current market needs.
Recruitment efforts have also become more digitized, with many companies adopting virtual hiring processes and online job fairs.
This creates both challenges and opportunities for job seekers to showcase their talents and secure interviews through online platforms.
NOTE: Visit Now
Remote Work and IT
The surge in remote work opportunities has transformed the job market. Many IT companies now offer fully remote or hybrid roles, which appeal to professionals seeking greater flexibility.
While remote work has increased access to job opportunities, it has also intensified competition, as companies can now hire from a global talent pool.
Section 2: Choosing the Right Keywords for Your IT Resume
Keyword Optimization: Why It Matters
With more employers using Applicant Tracking Systems (ATS) to screen resumes, it’s essential for jobseekers to optimize their resumes with relevant keywords.
These systems scan resumes for specific words related to the job description and only advance the most relevant applications.
To increase the chances of your resume making it through the initial screening, jobseekers must identify and incorporate the right keywords into their resumes.
When searching for jobs in IT, it’s important to tailor your resume for specific job titles and responsibilities. Keywords like “software engineer,” “cloud computing,” “data security,” and “DevOps” can make a huge difference.
By strategically using keywords that reflect your skills, experience, and the job requirements, you enhance your resume’s visibility to hiring managers and recruitment software.
Step-by-Step Keyword Selection Process
Analyze Job Descriptions: Look at several job postings for roles you’re interested in and identify recurring terms.
Incorporate Specific Terms: Include technical terms related to your field (e.g., Python, Kubernetes, cloud infrastructure).
Use Action Verbs: Keywords like “developed,” “designed,” or “implemented” help demonstrate your experience in a tangible way.
Test Your Resume: Use online tools to see how well your resume aligns with specific job postings and make adjustments as necessary.
Section 3: Customizing Your Resume for Each Job Application
Why Customization is Key
One size does not fit all when it comes to resumes, especially in the IT industry. Jobseekers who customize their resumes for each job application are more likely to catch the attention of recruiters. Tailoring your resume allows you to emphasize the specific skills and experiences that align with the job description, making you a stronger candidate. Employers want to see that you’ve taken the time to understand their needs and that your expertise matches what they are looking for.
Key Areas to Customize:
Summary Section: Write a targeted summary that highlights your qualifications and goals in relation to the specific job you’re applying for.
Skills Section: Highlight the most relevant skills for the position, paying close attention to the technical requirements listed in the job posting.
Experience Section: Adjust your work experience descriptions to emphasize the accomplishments and projects that are most relevant to the job.
Education & Certifications: If certain qualifications or certifications are required, make sure they are easy to spot on your resume.
NOTE: Read More
Section 4: Reviewing and Testing Your Optimized Resume
Proofreading for Perfection
Before submitting your resume, it’s critical to review it for accuracy, clarity, and relevance. Spelling mistakes, grammatical errors, or outdated information can reflect poorly on your professionalism.
Additionally, make sure your resume is easy to read and visually organized, with clear headings and bullet points. If possible, ask a peer or mentor in the IT field to review your resume for content accuracy and feedback.
Testing Your Resume with ATS Tools
After making your resume keyword-optimized, test it using online tools that simulate ATS systems. This allows you to see how well your resume aligns with specific job descriptions and identify areas for improvement.
Many tools will give you a match score, showing you how likely your resume is to pass an ATS scan. From here, you can fine-tune your resume to increase its chances of making it to the recruiter’s desk.
Section 5: Trends Shaping the Future of IT Recruitment
Embracing Digital Recruitment
Recruiting has undergone a significant shift towards digital platforms, with job fairs, interviews, and onboarding now frequently taking place online.
This transition means that jobseekers must be comfortable navigating virtual job fairs, remote interviews, and online assessments.
As IT jobs increasingly allow remote work, companies are also using technology-driven recruitment tools like AI for screening candidates.
Jobseekers should also leverage platforms like LinkedIn to increase visibility in the recruitment space. Keeping your LinkedIn profile updated, networking with industry professionals, and engaging in online discussions can all boost your chances of being noticed by recruiters.
Furthermore, participating in virtual job fairs or IT recruitment events provides direct access to recruiters and HR professionals, enhancing your job hunt.
FAQs
1. How important are keywords in IT resumes?
Keywords are essential in IT resumes because they ensure your resume passes through Applicant Tracking Systems (ATS), which scans resumes for specific terms related to the job. Without the right keywords, your resume may not reach a human recruiter.
2. How often should I update my resume?
It’s a good idea to update your resume regularly, especially when you gain new skills or experience. Also, customize it for every job application to ensure it aligns with the job’s specific requirements.
3. What are the most in-demand IT jobs?
Some of the most in-demand IT jobs include software developers, cloud engineers, cybersecurity analysts, data scientists, and DevOps engineers.
4. How can I stand out in the current IT job market?
To stand out, jobseekers should focus on tailoring their resumes, building strong online profiles, networking, and keeping up-to-date with industry trends. Participation in online forums, attending webinars, and earning industry-relevant certifications can also enhance visibility.
Conclusion
The IT job market continues to offer exciting opportunities for jobseekers, driven by technological innovations and changing work patterns.
By staying informed about current trends, customizing your resume, using keywords effectively, and testing your optimized resume, you can improve your job search success.
Whether you are new to the IT field or an experienced professional, leveraging these strategies will help you navigate the competitive landscape and secure a job that aligns with your career goals.
NOTE: Contact Us
2 notes
·
View notes
Text
Cloud Agnostic: Achieving Flexibility and Independence in Cloud Management
As businesses increasingly migrate to the cloud, they face a critical decision: which cloud provider to choose? While AWS, Microsoft Azure, and Google Cloud offer powerful platforms, the concept of "cloud agnostic" is gaining traction. Cloud agnosticism refers to a strategy where businesses avoid vendor lock-in by designing applications and infrastructure that work across multiple cloud providers. This approach provides flexibility, independence, and resilience, allowing organizations to adapt to changing needs and avoid reliance on a single provider.
What Does It Mean to Be Cloud Agnostic?
Being cloud agnostic means creating and managing systems, applications, and services that can run on any cloud platform. Instead of committing to a single cloud provider, businesses design their architecture to function seamlessly across multiple platforms. This flexibility is achieved by using open standards, containerization technologies like Docker, and orchestration tools such as Kubernetes.
Key features of a cloud agnostic approach include:
Interoperability: Applications must be able to operate across different cloud environments.
Portability: The ability to migrate workloads between different providers without significant reconfiguration.
Standardization: Using common frameworks, APIs, and languages that work universally across platforms.
Benefits of Cloud Agnostic Strategies
Avoiding Vendor Lock-InThe primary benefit of being cloud agnostic is avoiding vendor lock-in. Once a business builds its entire infrastructure around a single cloud provider, it can be challenging to switch or expand to other platforms. This could lead to increased costs and limited innovation. With a cloud agnostic strategy, businesses can choose the best services from multiple providers, optimizing both performance and costs.
Cost OptimizationCloud agnosticism allows companies to choose the most cost-effective solutions across providers. As cloud pricing models are complex and vary by region and usage, a cloud agnostic system enables businesses to leverage competitive pricing and minimize expenses by shifting workloads to different providers when necessary.
Greater Resilience and UptimeBy operating across multiple cloud platforms, organizations reduce the risk of downtime. If one provider experiences an outage, the business can shift workloads to another platform, ensuring continuous service availability. This redundancy builds resilience, ensuring high availability in critical systems.
Flexibility and ScalabilityA cloud agnostic approach gives companies the freedom to adjust resources based on current business needs. This means scaling applications horizontally or vertically across different providers without being restricted by the limits or offerings of a single cloud vendor.
Global ReachDifferent cloud providers have varying levels of presence across geographic regions. With a cloud agnostic approach, businesses can leverage the strengths of various providers in different areas, ensuring better latency, performance, and compliance with local regulations.
Challenges of Cloud Agnosticism
Despite the advantages, adopting a cloud agnostic approach comes with its own set of challenges:
Increased ComplexityManaging and orchestrating services across multiple cloud providers is more complex than relying on a single vendor. Businesses need robust management tools, monitoring systems, and teams with expertise in multiple cloud environments to ensure smooth operations.
Higher Initial CostsThe upfront costs of designing a cloud agnostic architecture can be higher than those of a single-provider system. Developing portable applications and investing in technologies like Kubernetes or Terraform requires significant time and resources.
Limited Use of Provider-Specific ServicesCloud providers often offer unique, advanced services—such as machine learning tools, proprietary databases, and analytics platforms—that may not be easily portable to other clouds. Being cloud agnostic could mean missing out on some of these specialized services, which may limit innovation in certain areas.
Tools and Technologies for Cloud Agnostic Strategies
Several tools and technologies make cloud agnosticism more accessible for businesses:
Containerization: Docker and similar containerization tools allow businesses to encapsulate applications in lightweight, portable containers that run consistently across various environments.
Orchestration: Kubernetes is a leading tool for orchestrating containers across multiple cloud platforms. It ensures scalability, load balancing, and failover capabilities, regardless of the underlying cloud infrastructure.
Infrastructure as Code (IaC): Tools like Terraform and Ansible enable businesses to define cloud infrastructure using code. This makes it easier to manage, replicate, and migrate infrastructure across different providers.
APIs and Abstraction Layers: Using APIs and abstraction layers helps standardize interactions between applications and different cloud platforms, enabling smooth interoperability.
When Should You Consider a Cloud Agnostic Approach?
A cloud agnostic approach is not always necessary for every business. Here are a few scenarios where adopting cloud agnosticism makes sense:
Businesses operating in regulated industries that need to maintain compliance across multiple regions.
Companies require high availability and fault tolerance across different cloud platforms for mission-critical applications.
Organizations with global operations that need to optimize performance and cost across multiple cloud regions.
Businesses aim to avoid long-term vendor lock-in and maintain flexibility for future growth and scaling needs.
Conclusion
Adopting a cloud agnostic strategy offers businesses unparalleled flexibility, independence, and resilience in cloud management. While the approach comes with challenges such as increased complexity and higher upfront costs, the long-term benefits of avoiding vendor lock-in, optimizing costs, and enhancing scalability are significant. By leveraging the right tools and technologies, businesses can achieve a truly cloud-agnostic architecture that supports innovation and growth in a competitive landscape.
Embrace the cloud agnostic approach to future-proof your business operations and stay ahead in the ever-evolving digital world.
2 notes
·
View notes
Text
Is cPanel on Its Deathbed? A Tale of Technology, Profits, and a Slow-Moving Train Wreck
Ah, cPanel. The go-to control panel for many web hosting services since the dawn of, well, web hosting. Once the epitome of innovation, it’s now akin to a grizzled war veteran, limping along with a cane and wearing an “I Survived Y2K” t-shirt. So what went wrong? Let’s dive into this slow-moving technological telenovela, rife with corporate greed, security loopholes, and a legacy that may be hanging by a thread.
Chapter 1: A Brief, Glorious History (Or How cPanel Shot to Stardom)
Once upon a time, cPanel was the bee’s knees. Launched in 1996, this software was, for a while, the pinnacle of web management systems. It promised simplicity, reliability, and functionality. Oh, the golden years!
Chapter 2: The Tech Stack Tortoise
In the fast-paced world of technology, being stagnant is synonymous with being extinct. While newer tech stacks are integrating AI, machine learning, and all sorts of jazzy things, cPanel seems to be stuck in a time warp. Why? Because the tech stack is more outdated than a pair of bell-bottom trousers. No Docker, no Kubernetes, and don’t even get me started on the lack of robust API support.
Chapter 3: “The Corpulent Corporate”
In 2018, Oakley Capital, a private equity firm, acquired cPanel. For many, this was the beginning of the end. Pricing structures were jumbled, turning into a monetisation extravaganza. It’s like turning your grandma’s humble pie shop into a mass production line for rubbery, soulless pies. They’ve squeezed every ounce of profit from it, often at the expense of the end-users and smaller hosting companies.
Chapter 4: Security—or the Lack Thereof
Ah, the elephant in the room. cPanel has had its fair share of vulnerabilities. Whether it’s SQL injection flaws, privilege escalation, or simple, plain-text passwords (yes, you heard right), cPanel often appears in the headlines for all the wrong reasons. It’s like that dodgy uncle at family reunions who always manages to spill wine on the carpet; you know he’s going to mess up, yet somehow he’s always invited.
Chapter 5: The (Dis)loyal Subjects—The Hosting Companies
Remember those hosting companies that once swore by cPanel? Well, let’s just say some of them have been seen flirting with competitors at the bar. Newer, shinier control panels are coming to market, offering modern tech stacks and, gasp, lower prices! It’s like watching cPanel’s loyal subjects slowly turn their backs, one by one.
Chapter 6: The Alternatives—Not Just a Rebellion, but a Revolution
Plesk, Webmin, DirectAdmin, oh my! New players are rising, offering updated tech stacks, more customizable APIs, and—wait for it—better security protocols. They’re the Han Solos to cPanel’s Jabba the Hutt: faster, sleeker, and without the constant drooling.
Conclusion: The Twilight Years or a Second Wind?
The debate rages on. Is cPanel merely an ageing actor waiting for its swan song, or can it adapt and evolve, perhaps surprising us all? Either way, the story of cPanel serves as a cautionary tale: adapt or die. And for heaven’s sake, update your tech stack before it becomes a relic in a technology museum, right between floppy disks and dial-up modems.
This outline only scratches the surface, but it’s a start. If cPanel wants to avoid becoming the Betamax of web management systems, it better start evolving—stat. Cheers!
#hosting#wordpress#cpanel#webdesign#servers#websites#webdeveloper#technology#tech#website#developer#digitalagency#uk#ukdeals#ukbusiness#smallbussinessowner
14 notes
·
View notes
Text
Elevating Your Full-Stack Developer Expertise: Exploring Emerging Skills and Technologies
Introduction: In the dynamic landscape of web development, staying at the forefront requires continuous learning and adaptation. Full-stack developers play a pivotal role in crafting modern web applications, balancing frontend finesse with backend robustness. This guide delves into the evolving skills and technologies that can propel full-stack developers to new heights of expertise and innovation.
Pioneering Progress: Key Skills for Full-Stack Developers
1. Innovating with Microservices Architecture:
Microservices have redefined application development, offering scalability and flexibility in the face of complexity. Mastery of frameworks like Kubernetes and Docker empowers developers to architect, deploy, and manage microservices efficiently. By breaking down monolithic applications into modular components, developers can iterate rapidly and respond to changing requirements with agility.
2. Embracing Serverless Computing:
The advent of serverless architecture has revolutionized infrastructure management, freeing developers from the burdens of server maintenance. Platforms such as AWS Lambda and Azure Functions enable developers to focus solely on code development, driving efficiency and cost-effectiveness. Embrace serverless computing to build scalable, event-driven applications that adapt seamlessly to fluctuating workloads.
3. Crafting Progressive Web Experiences (PWEs):
Progressive Web Apps (PWAs) herald a new era of web development, delivering native app-like experiences within the browser. Harness the power of technologies like Service Workers and Web App Manifests to create PWAs that are fast, reliable, and engaging. With features like offline functionality and push notifications, PWAs blur the lines between web and mobile, captivating users and enhancing engagement.
4. Harnessing GraphQL for Flexible Data Management:
GraphQL has emerged as a versatile alternative to RESTful APIs, offering a unified interface for data fetching and manipulation. Dive into GraphQL's intuitive query language and schema-driven approach to simplify data interactions and optimize performance. With GraphQL, developers can fetch precisely the data they need, minimizing overhead and maximizing efficiency.
5. Unlocking Potential with Jamstack Development:
Jamstack architecture empowers developers to build fast, secure, and scalable web applications using modern tools and practices. Explore frameworks like Gatsby and Next.js to leverage pre-rendering, serverless functions, and CDN caching. By decoupling frontend presentation from backend logic, Jamstack enables developers to deliver blazing-fast experiences that delight users and drive engagement.
6. Integrating Headless CMS for Content Flexibility:
Headless CMS platforms offer developers unprecedented control over content management, enabling seamless integration with frontend frameworks. Explore platforms like Contentful and Strapi to decouple content creation from presentation, facilitating dynamic and personalized experiences across channels. With headless CMS, developers can iterate quickly and deliver content-driven applications with ease.
7. Optimizing Single Page Applications (SPAs) for Performance:
Single Page Applications (SPAs) provide immersive user experiences but require careful optimization to ensure performance and responsiveness. Implement techniques like lazy loading and server-side rendering to minimize load times and enhance interactivity. By optimizing resource delivery and prioritizing critical content, developers can create SPAs that deliver a seamless and engaging user experience.
8. Infusing Intelligence with Machine Learning and AI:
Machine learning and artificial intelligence open new frontiers for full-stack developers, enabling intelligent features and personalized experiences. Dive into frameworks like TensorFlow.js and PyTorch.js to build recommendation systems, predictive analytics, and natural language processing capabilities. By harnessing the power of machine learning, developers can create smarter, more adaptive applications that anticipate user needs and preferences.
9. Safeguarding Applications with Cybersecurity Best Practices:
As cyber threats continue to evolve, cybersecurity remains a critical concern for developers and organizations alike. Stay informed about common vulnerabilities and adhere to best practices for securing applications and user data. By implementing robust security measures and proactive monitoring, developers can protect against potential threats and safeguard the integrity of their applications.
10. Streamlining Development with CI/CD Pipelines:
Continuous Integration and Deployment (CI/CD) pipelines are essential for accelerating development workflows and ensuring code quality and reliability. Explore tools like Jenkins, CircleCI, and GitLab CI/CD to automate testing, integration, and deployment processes. By embracing CI/CD best practices, developers can deliver updates and features with confidence, driving innovation and agility in their development cycles.
#full stack developer#education#information#full stack web development#front end development#web development#frameworks#technology#backend#full stack developer course
2 notes
·
View notes
Text
Microsoft Azure Fundamentals AI-900 (Part 5)
Microsoft Azure AI Fundamentals: Explore visual studio tools for machine learning
What is machine learning? A technique that uses math and statistics to create models that predict unknown values
Types of Machine learning
Regression - predict a continuous value, like a price, a sales total, a measure, etc
Classification - determine a class label.
Clustering - determine labels by grouping similar information into label groups
x = features
y = label
Azure Machine Learning Studio
You can use the workspace to develop solutions with the Azure ML service on the web portal or with developer tools
Web portal for ML solutions in Sure
Capabilities for preparing data, training models, publishing and monitoring a service.
First step assign a workspace to a studio.
Compute targets are cloud-based resources which can run model training and data exploration processes
Compute Instances - Development workstations that data scientists can use to work with data and models
Compute Clusters - Scalable clusters of VMs for on demand processing of experiment code
Inference Clusters - Deployment targets for predictive services that use your trained models
Attached Compute - Links to existing Azure compute resources like VMs or Azure data brick clusters
What is Azure Automated Machine Learning
Jobs have multiple settings
Provide information needed to specify your training scripts, compute target and Azure ML environment and run a training job
Understand the AutoML Process
ML model must be trained with existing data
Data scientists spend lots of time pre-processing and selecting data
This is time consuming and often makes inefficient use of expensive compute hardware
In Azure ML data for model training and other operations are encapsulated in a data set.
You create your own dataset.
Classification (predicting categories or classes)
Regression (predicting numeric values)
Time series forecasting (predicting numeric values at a future point in time)
After part of the data is used to train a model, then the rest of the data is used to iteratively test or cross validate the model
The metric is calculated by comparing the actual known label or value with the predicted one
Difference between the actual known and predicted is known as residuals; they indicate amount of error in the model.
Root Mean Squared Error (RMSE) is a performance metric. The smaller the value, the more accurate the model’s prediction is
Normalized root mean squared error (NRMSE) standardizes the metric to be used between models which have different scales.
Shows the frequency of residual value ranges.
Residuals represents variance between predicted and true values that can’t be explained by the model, errors
Most frequently occurring residual values (errors) should be clustered around zero.
You want small errors with fewer errors at the extreme ends of the sale
Should show a diagonal trend where the predicted value correlates closely with the true value
Dotted line shows a perfect model’s performance
The closer to the line of your model’s average predicted value to the dotted, the better.
Services can be deployed as an Azure Container Instance (ACI) or to a Azure Kubernetes Service (AKS) cluster
For production AKS is recommended.
Identify regression machine learning scenarios
Regression is a form of ML
Understands the relationships between variables to predict a desired outcome
Predicts a numeric label or outcome base on variables (features)
Regression is an example of supervised ML
What is Azure Machine Learning designer
Allow you to organize, manage, and reuse complex ML workflows across projects and users
Pipelines start with the dataset you want to use to train the model
Each time you run a pipelines, the context(history) is stored as a pipeline job
Encapsulates one step in a machine learning pipeline.
Like a function in programming
In a pipeline project, you access data assets and components from the Asset Library tab
You can create data assets on the data tab from local files, web files, open at a sets, and a datastore
Data assets appear in the Asset Library
Azure ML job executes a task against a specified compute target.
Jobs allow systematic tracking of your ML experiments and workflows.
Understand steps for regression
To train a regression model, your data set needs to include historic features and known label values.
Use the designer’s Score Model component to generate the predicted class label value
Connect all the components that will run in the experiment
Average difference between predicted and true values
It is based on the same unit as the label
The lower the value is the better the model is predicting
The square root of the mean squared difference between predicted and true values
Metric based on the same unit as the label.
A larger difference indicates greater variance in the individual label errors
Relative metric between 0 and 1 on the square based on the square of the differences between predicted and true values
Closer to 0 means the better the model is performing.
Since the value is relative, it can compare different models with different label units
Relative metric between 0 and 1 on the square based on the absolute of the differences between predicted and true values
Closer to 0 means the better the model is performing.
Can be used to compare models where the labels are in different units
Also known as R-squared
Summarizes how much variance exists between predicted and true values
Closer to 1 means the model is performing better
Remove training components form your data and replace it with a web service inputs and outputs to handle the web requests
It does the same data transformations as the first pipeline for new data
It then uses trained model to infer/predict label values based on the features.
Create a classification model with Azure ML designer
Classification is a form of ML used to predict which category an item belongs to
Like regression this is a supervised ML technique.
Understand steps for classification
True Positive - Model predicts the label and the label is correct
False Positive - Model predicts wrong label and the data has the label
False Negative - Model predicts the wrong label, and the data does have the label
True Negative - Model predicts the label correctly and the data has the label
For multi-class classification, same approach is used. A model with 3 possible results would have a 3x3 matrix.
Diagonal lien of cells were the predicted and actual labels match
Number of cases classified as positive that are actually positive
True positives divided by (true positives + false positives)
Fraction of positive cases correctly identified
Number of true positives divided by (true positives + false negatives)
Overall metric that essentially combines precision and recall
Classification models predict probability for each possible class
For binary classification models, the probability is between 0 and 1
Setting the threshold can define when a value is interpreted as 0 or 1. If its set to 0.5 then 0.5-1.0 is 1 and 0.0-0.4 is 0
Recall also known as True Positive Rate
Has a corresponding False Positive Rate
Plotting these two metrics on a graph for all values between 0 and 1 provides information.
Receiver Operating Characteristic (ROC) is the curve.
In a perfect model, this curve would be high to the top left
Area under the curve (AUC).
Remove training components form your data and replace it with a web service inputs and outputs to handle the web requests
It does the same data transformations as the first pipeline for new data
It then uses trained model to infer/predict label values based on the features.
Create a Clustering model with Azure ML designer
Clustering is used to group similar objects together based on features.
Clustering is an example of unsupervised learning, you train a model to just separate items based on their features.
Understanding steps for clustering
Prebuilt components exist that allow you to clean the data, normalize it, join tables and more
Requires a dataset that includes multiple observations of the items you want to cluster
Requires numeric features that can be used to determine similarities between individual cases
Initializing K coordinates as randomly selected points called centroids in an n-dimensional space (n is the number of dimensions in the feature vectors)
Plotting feature vectors as points in the same space and assigns a value how close they are to the closes centroid
Moving the centroids to the middle points allocated to it (mean distance)
Reassigning to the closes centroids after the move
Repeating the last two steps until tone.
Maximum distances between each point and the centroid of that point’s cluster.
If the value is high it can mean that cluster is widely dispersed.
With the Average Distance to Closer Center, we can determine how spread out the cluster is
Remove training components form your data and replace it with a web service inputs and outputs to handle the web requests
It does the same data transformations as the first pipeline for new data
It then uses trained model to infer/predict label values based on the features.
2 notes
·
View notes