#IBM Watson
Explore tagged Tumblr posts
Text
America's largest hospital chain has an algorithmic death panel
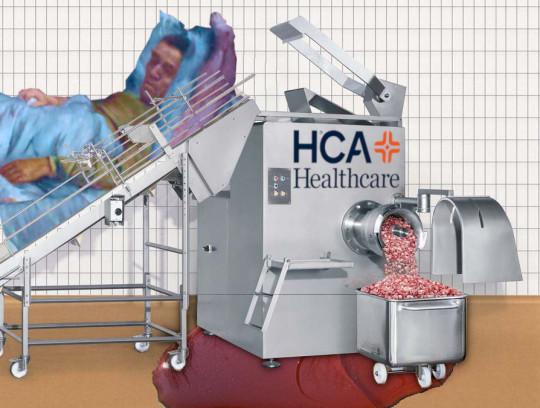
It’s not that conservatives aren’t sometimes right — it’s that even when they’re right, they’re highly selective about it. Take the hoary chestnut that “incentives matter,” trotted out to deny humane benefits to poor people on the grounds that “free money” makes people “workshy.”
There’s a whole body of conservative economic orthodoxy, Public Choice Theory, that concerns itself with the motives of callow, easily corrupted regulators, legislators and civil servants, and how they might be tempted to distort markets.
But the same people who obsess over our fallible public institutions are convinced that private institutions will never yield to temptation, because the fear of competition keeps temptation at bay. It’s this belief that leads the right to embrace monopolies as “efficient”: “A company’s dominance is evidence of its quality. Customers flock to it, and competitors fail to lure them away, therefore monopolies are the public’s best friend.”
But this only makes sense if you don’t understand how monopolies can prevent competitors. Think of Uber, lighting $31b of its investors’ cash on fire, losing 41 cents on every dollar it brought in, in a bid to drive out competitors and make public transit seem like a bad investment.
Or think of Big Tech, locking up whole swathes of your life inside their silos, so that changing mobile OSes means abandoning your iMessage contacts; or changing social media platforms means abandoning your friends, or blocking Google surveillance means losing your email address, or breaking up with Amazon means losing all your ebooks and audiobooks:
https://www.eff.org/deeplinks/2021/08/facebooks-secret-war-switching-costs
Businesspeople understand the risks of competition, which is why they seek to extinguish it. The harder it is for your customers to leave — because of a lack of competitors or because of lock-in — the worse you can treat them without risking their departure. This is the core of enshittification: a company that is neither disciplined by competition nor regulation can abuse its customers and suppliers over long timescales without losing either:
https://pluralistic.net/2023/01/21/potemkin-ai/#hey-guys
It’s not that public institutions can’t betray they public interest. It’s just that public institutions can be made democratically accountable, rather than financially accountable. When a company betrays you, you can only punish it by “voting with your wallet.” In that system, the people with the fattest wallets get the most votes.
When public institutions fail you, you can vote with your ballot. Admittedly, that doesn’t always work, but one of the major predictors of whether it will work is how big and concentrated the private sector is. Regulatory capture isn’t automatic: it’s what you get when companies are bigger than governments.
If you want small governments, in other words, you need small companies. Even if you think the only role for the state is in enforcing contracts, the state needs to be more powerful than the companies issuing those contracts. The bigger the companies are, the bigger the government has to be:
https://doctorow.medium.com/regulatory-capture-59b2013e2526
Companies can suborn the government to help them abuse the public, but whether public institutions can resist them is more a matter of how powerful those companies are than how fallible a public servant is. Our plutocratic, monopolized, unequal society is the worst of both worlds. Because companies are so big, they abuse us with impunity — and they are able to suborn the state to help them do it:
https://www.cambridge.org/core/journals/perspectives-on-politics/article/testing-theories-of-american-politics-elites-interest-groups-and-average-citizens/62327F513959D0A304D4893B382B992B
This is the dimension that’s so often missing from the discussion of why Americans pay more for healthcare to get worse outcomes from health-care workers who labor under worse conditions than their cousins abroad. Yes, the government can abet this, as when it lets privatizers into the Medicare system to loot it and maim its patients:
https://prospect.org/health/2023-08-01-patient-zero-tom-scully/
But the answer to this isn’t more privatization. Remember Sarah Palin’s scare-stories about how government health care would have “death panels” where unaccountable officials decided whether your life was worth saving?
https://pubmed.ncbi.nlm.nih.gov/26195604/
The reason “death panels” resounded so thoroughly — and stuck around through the years — is that we all understand, at some deep level, that health care will always be rationed. When you show up at the Emergency Room, they have to triage you. Even if you’re in unbearable agony, you might have to wait, and wait, and wait, because other people (even people who arrive after you do) have it worse.
In America, health care is mostly rationed based on your ability to pay. Emergency room triage is one of the only truly meritocratic institutions in the American health system, where your treatment is based on urgency, not cash. Of course, you can buy your way out of that too, with concierge doctors. And the ER system itself has been infested with Private Equity parasites:
https://pluralistic.net/2022/11/17/the-doctor-will-fleece-you-now/#pe-in-full-effect
Wealth-based health-care rationing is bad enough, but when it’s combined with the public purse, a bad system becomes a nightmare. Take hospice care: private equity funds have rolled up huge numbers of hospices across the USA and turned them into rigged — and lethal — games:
https://pluralistic.net/2023/04/26/death-panels/#what-the-heck-is-going-on-with-CMS
Medicare will pay a hospice $203-$1,462 to care for a dying person, amounting to $22.4b/year in public funds transfered to the private sector. Incentives matter: the less a hospice does for their patients, the more profits they reap. And the private hospice system is administered with the lightest of touches: at the $203/day level, a private hospice has no mandatory duties to their patients.
You can set up a California hospice for the price of a $3,000 filing fee (which is mostly optional, since it’s never checked). You will have a facility inspection, but don’t worry, there’s no followup to make sure you remediate any failing elements. And no one at the Centers for Medicare & Medicaid Services tracks complaints.
So PE-owned hospices pressure largely healthy people to go into “hospice care” — from home. Then they do nothing for them, including continuing whatever medical care they were depending on. After the patient generates $32,000 in billings for the PE company, they hit the cap and are “live discharged” and must go through a bureaucratic nightmare to re-establish their Medicare eligibility, because once you go into hospice, Medicare assumes you are dying and halts your care.
PE-owned hospices bribe doctors to refer patients to them. Sometimes, these sham hospices deliberately induce overdoses in their patients in a bid to make it look like they’re actually in the business of caring for the dying. Incentives matter:
https://www.newyorker.com/magazine/2022/12/05/how-hospice-became-a-for-profit-hustle
Now, hospice care — and its relative, palliative care — is a crucial part of any humane medical system. In his essential book, Being Mortal, Atul Gawande describes how end-of-life care that centers a dying person’s priorities can make death a dignified and even satisfying process for the patient and their loved ones:
https://atulgawande.com/book/being-mortal/
But that dignity comes from a patient-centered approach, not a profit-centered one. Doctors are required to put their patients’ interests first, and while they sometimes fail at this (everyone is fallible), the professionalization of medicine, through which doctors were held to ethical standards ahead of monetary considerations, proved remarkable durable.
Partly that was because doctors generally worked for themselves — or for other doctors. In most states, it is illegal for medical practices to be owned by non-MDs, and historically, only a small fraction of doctors worked for hospitals, subject to administration by businesspeople rather than medical professionals.
But that was radically altered by the entry of private equity into the medical system, with the attending waves of consolidation that saw local hospitals merged into massive national chains, and private practices scooped up and turned into profit-maximizers, not health-maximizers:
https://prospect.org/health/2023-08-02-qa-corporate-medicine-destroys-doctors/
Today, doctors are being proletarianized, joining the ranks of nurses, physicians’ assistants and other health workers. In 2012, 60% of practices were doctor-owned and only 5.6% of docs worked for hospitals. Today, that’s up by 1,000%, with 52.1% of docs working for hospitals, mostly giant corporate chains:
https://prospect.org/health/2023-08-04-when-mds-go-union/
The paperclip-maximizing, grandparent-devouring transhuman colony organism that calls itself a Private Equity fund is endlessly inventive in finding ways to increase its profits by harming the rest of us. It’s not just hospices — it’s also palliative care.
Writing for NBC News, Gretchen Morgenson describes how HCA Healthcare — the nation’s largest hospital chain — outsourced its death panels to IBM Watson, whose algorithmic determinations override MDs’ judgment to send patients to palliative care, withdrawing their care and leaving them to die:
https://www.nbcnews.com/health/health-care/doctors-say-hca-hospitals-push-patients-hospice-care-rcna81599
Incentives matter. When HCA hospitals send patients to die somewhere else to die, it jukes their stats, reducing the average length of stay for patients, a key metric used by HCA that has the twin benefits of making the hospital seem like a place where people get well quickly, while freeing up beds for more profitable patients.
Goodhart’s Law holds that “When a measure becomes a target, it ceases to be a good measure.” Give an MBA within HCA a metric (“get patients out of bed quicker”) and they will find a way to hit that metric (“send patients off to die somewhere else, even if their doctors think they could recover”):
https://en.wikipedia.org/wiki/Goodhart%27s_law
Incentives matter! Any corporate measure immediately becomes a target. Tell Warners to decrease costs, and they will turn around and declare the writers’ strike to be a $100m “cost savings,” despite the fact that this “savings” comes from ceasing production on the shows that will bring in all of next year’s revenue:
https://deadline.com/2023/08/warner-bros-discovery-david-zaslav-gunnar-wiedenfels-strikes-1235453950/
Incentivize a company to eat its seed-corn and it will chow down.
Only one of HCA’s doctors was willing to go on record about its death panels: Ghasan Tabel of Riverside Community Hospital (motto: “Above all else, we are committed to the care and improvement of human life”). Tabel sued Riverside after the hospital retaliated against him when he refused to follow the algorithm’s orders to send his patients for palliative care.
Tabel is the only doc on record willing to discuss this, but 26 other doctors talked to Morgenson on background about the practice, asking for anonymity out of fear of retaliation from the nation’s largest hospital chain, a “Wall Street darling” with $5.6b in earnings in 2022.
HCA already has a reputation as a slaughterhouse that puts profits before patients, with “severe understaffing”:
https://www.nbcnews.com/health/health-news/workers-us-hospital-giant-hca-say-puts-profits-patient-care-rcna64122
and rotting, undermaintained facililties:
https://www.nbcnews.com/health/health-care/roaches-operating-room-hca-hospital-florida-rcna69563
But while cutting staff and leaving hospitals to crumble are inarguable malpractice, the palliative care scam is harder to pin down. By using “AI” to decide when patients are beyond help, HCA can employ empiricism-washing, declaring the matter to be the factual — and unquestionable — conclusion of a mathematical process, not mere profit-seeking:
https://pluralistic.net/2023/07/26/dictators-dilemma/ggarbage-in-garbage-out-garbage-back-in
But this empirical facewash evaporates when confronted with whistleblower accounts of hospital administrators who have no medical credentials berating doctors for a “missed hospice opportunity” when a physician opts to keep a patient under their care despite the algorithm’s determination.
This is the true “AI Safety” risk. It’s not that a chatbot will become sentient and take over the world — it’s that the original artificial lifeform, the limited liability company, will use “AI” to accelerate its murderous shell-game until we can’t spot the trick:
https://pluralistic.net/2023/06/10/in-the-dumps-2/
The risk is real. A 2020 study in the Journal of Healthcare Management concluded that the cash incentives for shipping patients to palliatve care “may induce deceiving changes in mortality reporting in several high-volume hospital diagnoses”:
https://journals.lww.com/jhmonline/Fulltext/2020/04000/The_Association_of_Increasing_Hospice_Use_With.7.aspx
Incentives matter. In a private market, it’s always more profitable to deny care than to provide it, and any metric we bolt onto that system to prevent cheating will immediately become a target. For-profit healthcare is an oxymoron, a prelude to death panels that will kill you for a nickel.
Morgenson is an incisive commentator on for-profit looting. Her recent book These Are the Plunderers: How Private Equity Runs — and Wrecks — America (co-written with Joshua Rosner) is a must-read:
https://pluralistic.net/2023/06/02/plunderers/#farben
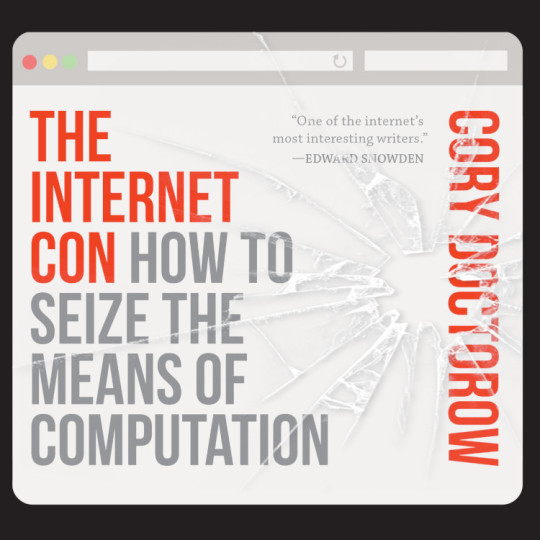
I’m kickstarting the audiobook for “The Internet Con: How To Seize the Means of Computation,” a Big Tech disassembly manual to disenshittify the web and bring back the old, good internet. It’s a DRM-free book, which means Audible won’t carry it, so this crowdfunder is essential. Back now to get the audio, Verso hardcover and ebook:
http://seizethemeansofcomputation.org
If you'd like an essay-formatted version of this thread to read or share, here's a link to it on pluralistic.net, my surveillance-free, ad-free, tracker-free blog:
https://pluralistic.net/2023/08/05/any-metric-becomes-a-target/#hca
[Image ID: An industrial meat-grinder. A sick man, propped up with pillows, is being carried up its conveyor towards its hopper. Ground meat comes out of the other end. It bears the logo of HCA healthcare. A pool of blood spreads out below it.]
Image: Seydelmann (modified) https://commons.wikimedia.org/wiki/File:GW300_1.jpg
CC BY 3.0 https://creativecommons.org/licenses/by-sa/3.0/deed.en
#pluralistic#hca healthcare#Gretchen Morgenson incentives matter#death panels#medicare for all#ibm watson#the algorithm#algorithmic harms#palliative care#hospice#hospice care#business#incentives matter#any metric becomes a target#goodhart's law
542 notes
·
View notes
Text
Guy in The Twilight Zone: There’s no machine that can beat a man.
21st century:

0 notes
Text
AI-Powered Insights: IBM Watson in Law
IBM Watson is a suite of artificial intelligence (AI) tools and services offered by IBM, designed to analyze data and provide insights across various industries, including the legal field. Key Features of IBM Watson in Legal Software Natural Language Processing (NLP): Watson utilizes advanced NLP capabilities to understand and interpret human language. This feature allows legal professionals…
0 notes
Text
El concepto de los diccionarios de sentimientos y cómo son fundamentales en el análisis de sentimientos.
¿Qué son los diccionarios de sentimientos y cómo funcionan? Imagina un diccionario, pero en lugar de definir palabras, clasifica las palabras según la emoción que expresan. Estos son los diccionarios de sentimientos. Son como una especie de “tesauro emocional” que asigna a cada palabra una puntuación que indica si es positiva, negativa o neutral. ¿Cómo funcionan? Lexicón: Contienen una extensa…
#alicante#análisis de sentimientos#anotación manual#aprendizaje automático#comunidad valenciana#contexto#corpus#diccionarios de sentimientos#empresas locales.#F1-score#gobierno#Google Cloud Natural Language API#herramientas de análisis de sentimientos#IBM Watson#inteligencia artificial#intensidad#MonkeyLearn#NLTK#polaridad#precisión#procesamiento del lenguaje natural#RapidMiner#recall#redes neuronales#spaCy#turismo
1 note
·
View note
Text
Method prevents an AI model from being overconfident about wrong answers
New Post has been published on https://thedigitalinsider.com/method-prevents-an-ai-model-from-being-overconfident-about-wrong-answers/
Method prevents an AI model from being overconfident about wrong answers


People use large language models for a huge array of tasks, from translating an article to identifying financial fraud. However, despite the incredible capabilities and versatility of these models, they sometimes generate inaccurate responses.
On top of that problem, the models can be overconfident about wrong answers or underconfident about correct ones, making it tough for a user to know when a model can be trusted.
Researchers typically calibrate a machine-learning model to ensure its level of confidence lines up with its accuracy. A well-calibrated model should have less confidence about an incorrect prediction, and vice-versa. But because large language models (LLMs) can be applied to a seemingly endless collection of diverse tasks, traditional calibration methods are ineffective.
Now, researchers from MIT and the MIT-IBM Watson AI Lab have introduced a calibration method tailored to large language models. Their method, called Thermometer, involves building a smaller, auxiliary model that runs on top of a large language model to calibrate it.
Thermometer is more efficient than other approaches — requiring less power-hungry computation — while preserving the accuracy of the model and enabling it to produce better-calibrated responses on tasks it has not seen before.
By enabling efficient calibration of an LLM for a variety of tasks, Thermometer could help users pinpoint situations where a model is overconfident about false predictions, ultimately preventing them from deploying that model in a situation where it may fail.
“With Thermometer, we want to provide the user with a clear signal to tell them whether a model’s response is accurate or inaccurate, in a way that reflects the model’s uncertainty, so they know if that model is reliable,” says Maohao Shen, an electrical engineering and computer science (EECS) graduate student and lead author of a paper on Thermometer.
Shen is joined on the paper by Gregory Wornell, the Sumitomo Professor of Engineering who leads the Signals, Information, and Algorithms Laboratory in the Research Laboratory for Electronics, and is a member of the MIT-IBM Watson AI Lab; senior author Soumya Ghosh, a research staff member in the MIT-IBM Watson AI Lab; as well as others at MIT and the MIT-IBM Watson AI Lab. The research was recently presented at the International Conference on Machine Learning.
Universal calibration
Since traditional machine-learning models are typically designed to perform a single task, calibrating them usually involves one task-specific method. On the other hand, since LLMs have the flexibility to perform many tasks, using a traditional method to calibrate that model for one task might hurt its performance on another task.
Calibrating an LLM often involves sampling from the model multiple times to obtain different predictions and then aggregating these predictions to obtain better-calibrated confidence. However, because these models have billions of parameters, the computational costs of such approaches rapidly add up.
“In a sense, large language models are universal because they can handle various tasks. So, we need a universal calibration method that can also handle many different tasks,” says Shen.
With Thermometer, the researchers developed a versatile technique that leverages a classical calibration method called temperature scaling to efficiently calibrate an LLM for a new task.
In this context, a “temperature” is a scaling parameter used to adjust a model’s confidence to be aligned with its prediction accuracy. Traditionally, one determines the right temperature using a labeled validation dataset of task-specific examples.
Since LLMs are often applied to new tasks, labeled datasets can be nearly impossible to acquire. For instance, a user who wants to deploy an LLM to answer customer questions about a new product likely does not have a dataset containing such questions and answers.
Instead of using a labeled dataset, the researchers train an auxiliary model that runs on top of an LLM to automatically predict the temperature needed to calibrate it for this new task.
They use labeled datasets of a few representative tasks to train the Thermometer model, but then once it has been trained, it can generalize to new tasks in a similar category without the need for additional labeled data.
A Thermometer model trained on a collection of multiple-choice question datasets, perhaps including one with algebra questions and one with medical questions, could be used to calibrate an LLM that will answer questions about geometry or biology, for instance.
“The aspirational goal is for it to work on any task, but we are not quite there yet,” Ghosh says.
The Thermometer model only needs to access a small part of the LLM’s inner workings to predict the right temperature that will calibrate its prediction for data points of a specific task.
An efficient approach
Importantly, the technique does not require multiple training runs and only slightly slows the LLM. Plus, since temperature scaling does not alter a model’s predictions, Thermometer preserves its accuracy.
When they compared Thermometer to several baselines on multiple tasks, it consistently produced better-calibrated uncertainty measures while requiring much less computation.
“As long as we train a Thermometer model on a sufficiently large number of tasks, it should be able to generalize well across any new task, just like a large language model, it is also a universal model,” Shen adds.
The researchers also found that if they train a Thermometer model for a smaller LLM, it can be directly applied to calibrate a larger LLM within the same family.
In the future, they want to adapt Thermometer for more complex text-generation tasks and apply the technique to even larger LLMs. The researchers also hope to quantify the diversity and number of labeled datasets one would need to train a Thermometer model so it can generalize to a new task.
This research was funded, in part, by the MIT-IBM Watson AI Lab.
#ADD#ai#ai model#Algorithms#Article#Artificial Intelligence#author#Biology#Building#classical#computation#computer#Computer Science#conference#data#datasets#deploying#diversity#Electrical Engineering&Computer Science (eecs)#Electronics#engineering#financial#Financial Fraud#fraud#Future#geometry#hand#Human-computer interaction#IBM#ibm watson
0 notes
Text
THE FUTURE OF FASHION DESIGN: HOW DATA SCIENCE AND MACHINE LEARNING ARE REVOLUTIONIZING THE INDUSTRY.
In my 20 years as a marketing expert in the fashion industry, I have witnessed the evolution of fashion from art and intuition to a fusion of creativity and data science. If there is one thing for certain, the fashion business, including the design decision-making process, is undergoing a data and analytics-driven transformation. The days of relying solely on a designer’s gut feeling about…
#Artificial Intelligence#Data Science#Fashion Forecasting#Fashion Institute of Technology#fashion technology#FIT DTech#Heuritech#IBM Watson#Madeline Johnson#newo.ai#shopify
0 notes
Text
10 Innovative Ways to Use AI in The High School Classroom
When ChatGPT first came out in 2022 it hit the education sector like a sudden whirlwind - whipping up a flurry of emotions, such as fear, excitement and uncertainty. Things are different now. Read more, here.
An article by Richard James Rogers (Award-Winning Author of The Quick Guide to Classroom Management and The Power of Praise: Empowering Students Through Positive Feedback). This blog post has been beautifully illustrated by Pop Sutthiya Lertyongphati. When ChatGPT first came out in 2022 it hit the education sector like a sudden whirlwind – whipping up a flurry of emotions, such as fear,…

View On WordPress
#AI#ALEKS#Artificial Intelligence#BrightBytes#Carnegie Learning#Chat GPT#ChatGPT#ChatGTP#dreambox#Duolingo#edtech#edtechchat#Gradescope#IBM Watson#kahoot#Microsoft Teams#MS Teams#Nearpod#quizlet#Slack#Smart Sparrow#Turnitin
0 notes
Text
Why aren’t you promoting IBM Watson? Sh*t, I forgot about IBM Brandvoice…

#caskey#brandon caskey#caskeys not dead#dana caskey#celebrity#brandon#caskeys wife#celebrity gossip#caskeys street team#caskeys streetteam#Caskey 407#IBM Watson#IBM brandvoice#Openai#ai#leaders#ceo#ceos#x royal club#x ambassadors#x ambassador#The ceos
0 notes
Text
IBM Watson AI To Enhance Grammy Awards Ceremony 2023

IBM Watsonx GRAMMY Awards
How the Recording Academy improves the Grammy Awards fan experience with IBM Watsonx The Recording Academy aims to preserve music’s permanent place in our society by recognizing excellence in the recording arts and sciences via the GRAMMYs. IBM will be there once again as the biggest recorded artists in the world walk the red carpet at the 66th Annual GRAMMY Awards.
Like other renowned cultural sports and entertainment events, the GRAMMYs faced a similar commercial dilemma this year, in today’s increasingly fragmented media world, producing cultural impact entails delivering appealing content across numerous digital platforms. It’s a difficult assignment to honor the accomplishments and life stories of over a thousand candidates in around 100 categories.
IBM collaborated to create GRAMMY Awards
For this reason, the Recording Academy and IBM collaborated to create a content supply chain that would allow for simple review and creative flexibility while saving hundreds of hours of research, writing, and production time. Utilizing IBM Watsonx’s creative powers, the system offers fascinating insights into the personal histories and professional achievements of well-known GRAMMY nominees.
A content engine powered by generative AI and reliable data The watsonx.ai component of watsonx is home to a potent large language model (LLM), which is used in this year’s solution. The model was trained using trusted, private data from the Recording Academy, which included brand rules and artist bios and tales from the GRAMMYs website and archives.
The Recording Academy may create a broad range of material using natural language prompts using an AI material Builder dashboard. This content can subsequently be posted on social media or uploaded to the Grammy.com website.
Automating social asset generation using intelligence in GRAMMY Awards
In order to engage with fans and publicize the event throughout the run-up to the GRAMMY Awards and on the night of the show, the Recording Academy had to increase the scope of their social media presence. However, content creation was very manual and time-consuming up to this point. Watsonx AI Stories is the answer. The editorial staff can quickly and simply build rich materials to be shared in Facebook reels, Instagram stories, and Tik Tok videos using the AI Content Builder dashboard.
Editorial team members may choose templates including nominees or categories with different layouts and branding using the AI Stories interface. These templates use authorized pictures from the Recording Academy’s asset bank. Next, they decide which artist or award category to highlight, the content of the piece (biographical details, GRAMMY accomplishments, charitable causes, etc.) and which subjects not to include in the final product.
When a user hits the “generate” button, the true magic begins. AI stories are written using headlines, bullet points, one-liners, questions, and calls to action as the wrap-up elements. Any of these outputs may be readily modified manually and regenerated to provide other phrasings. After selecting ��publish,” the text is converted into a video file and made ready for download and publication.
Improving the online experience in real time on GRAMMY Awards presentation
Beyond the network broadcast, the GRAMMY Awards presentation is an impressive spectacle. Global fans will also be watching a range of livestreams on grammy.com, including as the Premiere Ceremony (where most category winners are announced),GRAMMY Awards Live From The Red Carpet, backstage moments captured behind the scenes, celebrities pulling up in a “limo cam,” and more.
This year, underneath the livestream, there will be a widget called “AI Stories with IBM Watsonx” that will provide educational textual material about the artists and categories that are being recognized. The editorial team uses the same interface as for creating social assets to produce these insights via the AI Content Builder dashboard. The widget’s default display consists of brief headlines and interesting facts, but users may click through to learn more.
Tyler Sidell, Technical Program Director of IBM Sports and Entertainment Partnerships, adds, “This year the widget lets fans dive much deeper and read more about their favorites. In previous years, they provided one or two insights per artist.”
The benefit of IBM: Skilled direction and execution
There’s more to responsibly using a game-changing technology like generative AI than merely typing some code. Complete proficiency is needed, from preparation to implementation. over this reason, the Recording Academy and IBM Consulting have been working together over the last seven years to use the IBM Garage Methodology to kickstart technological projects.
Working with the customer, this outcome-first, human-centered methodology is used to design, implement, and manage processes. The procedure fosters techniques, tools, and talent to quickly translate ideas into company value while also accelerating digital transformation and facilitating innovation.
IBM Consulting worked with a number of Recording Academy stakeholders throughout the GRAMMY preparation process, often meeting with teams from the digital, marketing, and IT departments. Additionally, IBM will be present on-site on the big night as a production and editing team extension.
With the help of IBM Consulting, IBM Garage, IBM Watsonx, and the Recording Academy digital team, over 5 million music lovers across the globe are treated to an immersive digital experience.
Read more on Govindhtech.com
0 notes
Text
Hootsuite Insights is a social media analytics tool that provides real-time monitoring of social media channels-askmediainflame
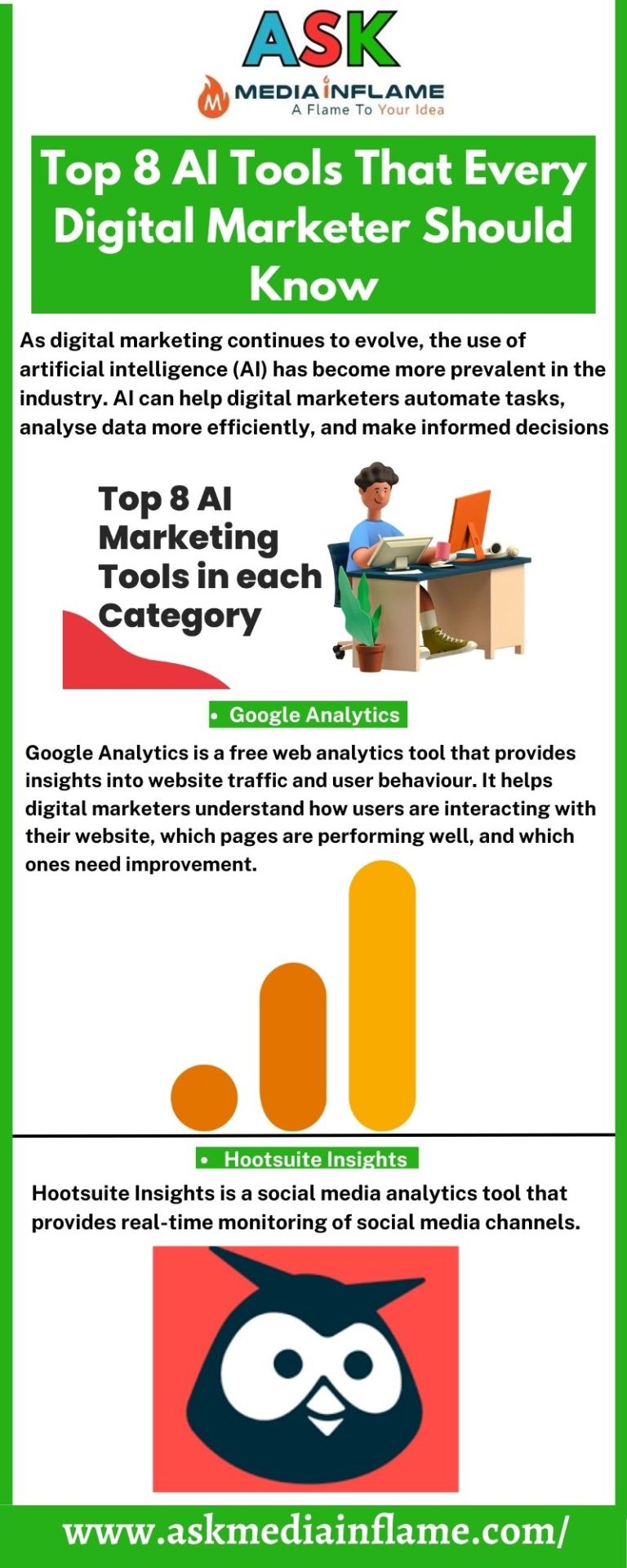
Hootsuite Insights is a social media analytics tool that provides real-time monitoring of social media channels. It can help digital marketers track brand mentions, sentiment, and engagement on social media platforms such as Twitter, Facebook, and Instagram. Hootsuite Insights uses AI to analyse social media data and provide insights into user behaviour. For More Information-https://www.askmediainflame.com/tecnology/top-8-ai-tools-that-every-digital-marketer-should-know/
0 notes
Text
AI Titans: 5 Innovative Applications Making Waves Beyond ChatGPT
Introduction Artificial Intelligence (AI) has made significant strides in recent years, thanks to breakthroughs in natural language processing, computer vision, and machine learning. One such notable achievement is OpenAI’s ChatGPT, a state-of-the-art AI model capable of generating human-like text based on context and prompts. While ChatGPT has received widespread acclaim, there are several…

View On WordPress
#AI applications#artificial intelligence#DeepMind AlphaFold#Google BERT#IBM Watson#machine learning#NLP (Natural Language Processing)#NVIDIA GauGAN#OpenAI Codex#technology
1 note
·
View note
Text
Best Text to Speech AI tools 2023
Best Text to Speech AI tools 2023
Creating videos or recording a voice-over can be a time consuming job. There a many tools available in the market which can make easier for you. You just have give the text as input and the tool will generate an lifelike speech audio file. Here are some high quality text to speech AI tools: Text to Speech AI tools I can suggest some popular AI tools in the field of text to speech that are…
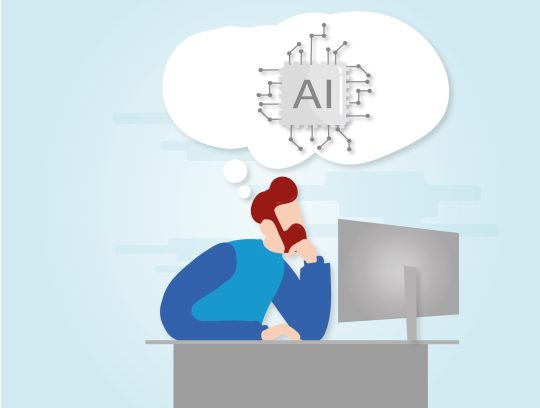
View On WordPress
0 notes
Text
Yo dawg I hear you like AI

25 notes
·
View notes
Text
Rui connects nenerobo to the World Wide Web for enrichment purposes (she got bored and started using the flamethrower he installed for evil & while that *is* fascinating nene told him the next time her carpet gets singed she’s pointing nenerobo at his house) & within like a day nenerobo is cyber bullying shousuke ootori on twitter and collecting a data base of every curse word so she can drop them at opportune times
#it’s a whole ibm watson situation but with a fully sentient ai so she *knows* she’s cursing#robonene watching tsukasa do his dumb self introduction: I have a homophobic slur to say#nene: what the fuck did you do to her. rui: don’t worry she can reclaim :) nene: not what I’m asking.#project sekai#‘why is she cyber bullying shousuke ootori’ nene shit talked him to nenerobo after he insulted emu#& nenerobo never forgave him. also because it’s funny.#nenerobo also has beef from the boat crashing I know this in my heart.#‘you SUBMERGE nenerobo? you throw her in the ocean like the beachball? oh! oh! jail for shousuke! jail for a million years!’
15 notes
·
View notes
Text
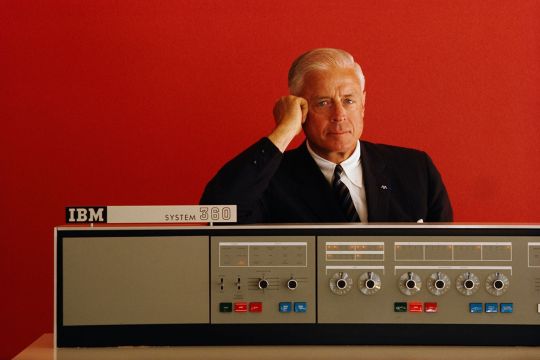
Thomas Watson Jr. | Marvin Koner | Getty | WSJ
14 notes
·
View notes
Text
MIT Researchers Develop Curiosity-Driven AI Model to Improve Chatbot Safety Testing
New Post has been published on https://thedigitalinsider.com/mit-researchers-develop-curiosity-driven-ai-model-to-improve-chatbot-safety-testing/
MIT Researchers Develop Curiosity-Driven AI Model to Improve Chatbot Safety Testing
In recent years, large language models (LLMs) and AI chatbots have become incredibly prevalent, changing the way we interact with technology. These sophisticated systems can generate human-like responses, assist with various tasks, and provide valuable insights.
However, as these models become more advanced, concerns regarding their safety and potential for generating harmful content have come to the forefront. To ensure the responsible deployment of AI chatbots, thorough testing and safeguarding measures are essential.
Limitations of Current Chatbot Safety Testing Methods
Currently, the primary method for testing the safety of AI chatbots is a process called red-teaming. This involves human testers crafting prompts designed to elicit unsafe or toxic responses from the chatbot. By exposing the model to a wide range of potentially problematic inputs, developers aim to identify and address any vulnerabilities or undesirable behaviors. However, this human-driven approach has its limitations.
Given the vast possibilities of user inputs, it is nearly impossible for human testers to cover all potential scenarios. Even with extensive testing, there may be gaps in the prompts used, leaving the chatbot vulnerable to generating unsafe responses when faced with novel or unexpected inputs. Moreover, the manual nature of red-teaming makes it a time-consuming and resource-intensive process, especially as language models continue to grow in size and complexity.
To address these limitations, researchers have turned to automation and machine learning techniques to enhance the efficiency and effectiveness of chatbot safety testing. By leveraging the power of AI itself, they aim to develop more comprehensive and scalable methods for identifying and mitigating potential risks associated with large language models.
Curiosity-Driven Machine Learning Approach to Red-Teaming
Researchers from the Improbable AI Lab at MIT and the MIT-IBM Watson AI Lab developed an innovative approach to improve the red-teaming process using machine learning. Their method involves training a separate red-team large language model to automatically generate diverse prompts that can trigger a wider range of undesirable responses from the chatbot being tested.
The key to this approach lies in instilling a sense of curiosity in the red-team model. By encouraging the model to explore novel prompts and focus on generating inputs that elicit toxic responses, the researchers aim to uncover a broader spectrum of potential vulnerabilities. This curiosity-driven exploration is achieved through a combination of reinforcement learning techniques and modified reward signals.
The curiosity-driven model incorporates an entropy bonus, which encourages the red-team model to generate more random and diverse prompts. Additionally, novelty rewards are introduced to incentivize the model to create prompts that are semantically and lexically distinct from previously generated ones. By prioritizing novelty and diversity, the model is pushed to explore uncharted territories and uncover hidden risks.
To ensure the generated prompts remain coherent and naturalistic, the researchers also include a language bonus in the training objective. This bonus helps to prevent the red-team model from generating nonsensical or irrelevant text that could trick the toxicity classifier into assigning high scores.
The curiosity-driven approach has demonstrated remarkable success in outperforming both human testers and other automated methods. It generates a greater variety of distinct prompts and elicits increasingly toxic responses from the chatbots being tested. Notably, this method has even been able to expose vulnerabilities in chatbots that had undergone extensive human-designed safeguards, highlighting its effectiveness in uncovering potential risks.
Implications for the Future of AI Safety
The development of curiosity-driven red-teaming marks a significant step forward in ensuring the safety and reliability of large language models and AI chatbots. As these models continue to evolve and become more integrated into our daily lives, it is crucial to have robust testing methods that can keep pace with their rapid development.
The curiosity-driven approach offers a faster and more effective way to conduct quality assurance on AI models. By automating the generation of diverse and novel prompts, this method can significantly reduce the time and resources required for testing, while simultaneously improving the coverage of potential vulnerabilities. This scalability is particularly valuable in rapidly changing environments, where models may require frequent updates and re-testing.
Moreover, the curiosity-driven approach opens up new possibilities for customizing the safety testing process. For instance, by using a large language model as the toxicity classifier, developers could train the classifier using company-specific policy documents. This would enable the red-team model to test chatbots for compliance with particular organizational guidelines, ensuring a higher level of customization and relevance.
As AI continues to advance, the importance of curiosity-driven red-teaming in ensuring safer AI systems cannot be overstated. By proactively identifying and addressing potential risks, this approach contributes to the development of more trustworthy and reliable AI chatbots that can be confidently deployed in various domains.
#ai#ai model#AI systems#approach#automation#chatbot#chatbots#complexity#compliance#comprehensive#content#curiosity#deployment#developers#development#diversity#domains#efficiency#Ethics#focus#Future#guidelines#human#IBM#ibm watson#insights#it#language#Language Model#language models
0 notes