#Databricks Lakehouse
Explore tagged Tumblr posts
Text
Why Can We Say Databricks Lakehouse Problem Solving Tool?
Databricks lakehouse would definitely help you in this regard since it enables you to do data processing, transformation, and analysis. Due to this convenience, you stay ahead by addressing challenges corresponding to data processing and analytics. For more information call us @ 9971900416 or mail us at [email protected]
For more details - https://gaininfotech.com/blog/2023/10/30/why-can-we-say-databricks-lakehouse-problem-solving-tool/
2 notes
·
View notes
Text
Real-World Application of Data Mesh with Databricks Lakehouse
Explore how a global reinsurance leader transformed its data systems with Data Mesh and Databricks Lakehouse for better operations and decision-making.
View On WordPress
#Advanced Analytics#Business Transformation#Cloud Solutions#Data Governance#Data management#Data Mesh#Data Scalability#Databricks Lakehouse#Delta Sharing#Enterprise Architecture#Reinsurance Industry
0 notes
Text
Databricks’ TAO method to allow LLM training with unlabeled data
Data lakehouse provider Databricks has unveiled a new large language model (LLM) training method, TAO that will allow enterprises to train models without labeling data. Typically, LLMs when being adapted to new enterprise tasks are trained by using prompts or by fine-tuning the model with datasets for the specific task. However, both these techniques have caveats. While prompting is seen as an…
0 notes
Text
Kadel Labs: Leading the Way as Databricks Consulting Partners
In today’s data-driven world, businesses are constantly seeking efficient ways to harness the power of big data. As organizations generate vast amounts of structured and unstructured data, they need advanced tools and expert guidance to extract meaningful insights. This is where Kadel Labs, a leading technology solutions provider, steps in. As Databricks Consulting Partners, Kadel Labs specializes in helping businesses leverage the Databricks Lakehouse platform to unlock the full potential of their data.
0 notes
Text
What’s New In Databricks? February 2025 Updates & Features Explained!
youtube
What’s New In Databricks? February 2025 Updates & Features Explained! #databricks #spark #dataengineering
Are you ready for the latest Databricks updates in February 2025? 🚀 This month brings game-changing features like SAP integration, Lakehouse Federation for Teradata, Databricks Clean Rooms, SQL Pipe, Serverless on Google Cloud, Predictive Optimization, and more!
✨ Explore Databricks AI insights and workflows—read more: / databrickster
🔔𝐃𝐨𝐧'𝐭 𝐟𝐨𝐫𝐠𝐞𝐭 𝐭𝐨 𝐬𝐮𝐛𝐬𝐜𝐫𝐢𝐛𝐞 𝐭𝐨 𝐦𝐲 𝐜𝐡𝐚𝐧𝐧𝐞𝐥 𝐟𝐨𝐫 𝐦𝐨𝐫𝐞 𝐮𝐩𝐝𝐚𝐭𝐞𝐬. / @hubert_dudek
🔗 Support Me Here! ☕Buy me a coffee: https://ko-fi.com/hubertdudek
🔗 Stay Connected With Me. Medium: / databrickster
==================
#databricks#bigdata#dataengineering#machinelearning#sql#cloudcomputing#dataanalytics#ai#azure#googlecloud#aws#etl#python#data#database#datawarehouse#Youtube
1 note
·
View note
Text
Enhance Data Management with Databricks: A Comprehensive Approach to Lakehouse Architecture
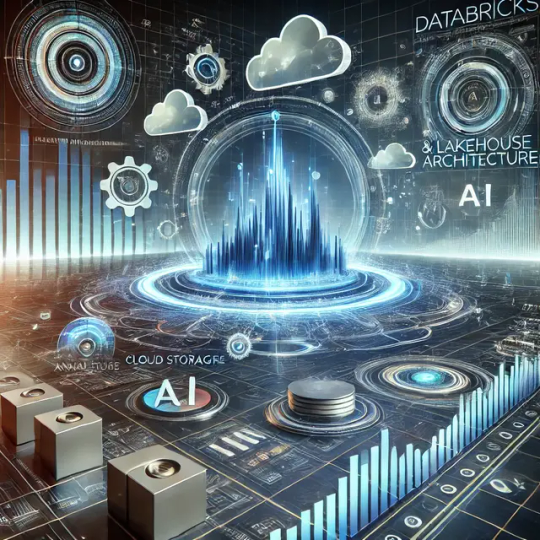
In the modern data landscape, organizations are faced with increasing data complexity and the need for efficient operations to stay ahead of the competition. This calls for innovative solutions that streamline data management while providing scalability and performance. One such solution is Databricks, a unified data analytics platform that accelerates data-driven decision-making. With the power of lakehouse architecture, Databricks offers a comprehensive approach to managing and analyzing large-scale data, blending the best of data lakes and data warehouses.
What is Lakehouse Architecture?
Before diving into how Databricks accelerates data operations with lakehouse architecture, it’s important to understand the concept of lakehouse itself. Traditional data architectures typically rely on either data lakes or data warehouses. While data lakes are ideal for storing large volumes of unstructured data, and data warehouses are optimized for structured data and high-performance queries, each of these models has its limitations.
Lakehouse architecture bridges this gap by combining the best features of both. It allows organizations to store massive amounts of raw, unstructured data in a data lake while enabling the management, performance, and analytics capabilities typically found in a data warehouse. The result is a highly flexible, cost-effective platform for managing both structured and unstructured data.
Lakehouse architecture facilitates real-time analytics and machine learning, providing unified governance and security controls while offering powerful tools for querying, transforming, and processing data. Databricks, built with lakehouse principles at its core, provides an environment where businesses can gain insights quickly, irrespective of data complexity or format.
The Role of Databricks in Accelerating Data Operations
Databricks has emerged as one of the leading platforms in the field of big data analytics. It simplifies the complexities of working with vast datasets by offering an integrated environment that leverages the lakehouse architecture. The key features of Databricks that contribute to faster and more efficient data operations include:
Unified Data Platform: Databricks offers a unified platform that allows organizations to manage their data across multiple environments. Whether the data is structured, semi-structured, or unstructured, Databricks ensures that all of it can be processed, analyzed, and stored in a single framework. This eliminates the need for disparate systems and the complexity of managing multiple data sources, making operations more streamlined and faster.
Scalable Performance: One of the key advantages of lakehouse architecture is its scalability, and Databricks ensures this by offering a highly scalable environment. With cloud-native capabilities, Databricks provides elastic compute resources, allowing organizations to scale their data operations up or down based on demand. This dynamic resource allocation helps maintain optimal performance, even as data volumes grow exponentially.
Delta Lake for Reliable Data Management: Delta Lake, an open-source storage layer developed by Databricks, enhances lakehouse architecture by adding transactional integrity to data lakes. It provides ACID (Atomicity, Consistency, Isolation, Durability) properties to your data, which ensures that data operations such as inserts, updates, and deletes are reliable and consistent. Delta Lake simplifies data engineering workflows by enabling schema enforcement, time travel (data versioning), and easy auditing, ensuring data accuracy and quality.
Real-Time Data Analytics: Databricks empowers organizations to perform real-time analytics on streaming data, which is essential for fast decision-making. The platform enables the continuous ingestion of data and facilitates real-time processing, so organizations can gain actionable insights without delay. This capability is critical for industries such as finance, retail, and manufacturing, where timely data analysis can lead to competitive advantages.
Collaborative Data Science Environment: Another key benefit of Databricks is its collaborative nature. The platform allows data scientists, data engineers, and business analysts to work together on the same projects in real-time. With shared notebooks and integrated workflows, teams can streamline the development of machine learning models and data pipelines, fostering collaboration and enhancing productivity.
Simplified Machine Learning Operations (MLOps): Databricks provides a robust environment for deploying, monitoring, and maintaining machine learning models. This is where the lakehouse architecture shines, as it enables data scientists to work with large datasets, conduct training in parallel across multiple clusters, and deploy models quickly into production. By integrating machine learning with the unified data lakehouse environment, Databricks accelerates the deployment of AI-driven insights.
Benefits of Databricks and Lakehouse Architecture
Databricks, with its integration of lakehouse architecture, offers several benefits that enhance data operations. These benefits include:
Cost Efficiency: Traditional data warehouses often come with high storage and compute costs, especially when handling large datasets. Databricks reduces the cost of data management by utilizing the storage capabilities of data lakes, which are more cost-effective. It optimizes data processing workflows, making it easier to analyze large datasets without incurring hefty infrastructure costs.
Improved Data Governance and Security: As organizations manage larger and more complex datasets, maintaining strong governance and security becomes crucial. Lakehouse architecture, supported by Databricks, ensures that data is properly governed with built-in security features such as role-based access control (RBAC), auditing, and data lineage tracking. These features help businesses comply with regulations and manage sensitive data securely.
Faster Time to Insights: The integration of real-time analytics and machine learning within Databricks ensures that organizations can gain insights faster than traditional data systems allow. By leveraging lakehouse principles, Databricks enables the processing of both historical and streaming data simultaneously, which accelerates the decision-making process. This is particularly beneficial for organizations aiming to stay ahead in rapidly changing industries.
Seamless Integration with Cloud Providers: Databricks supports major cloud platforms, including Amazon Web Services (AWS), Microsoft Azure, and Google Cloud. This makes it easier for businesses to leverage their existing cloud infrastructure and take advantage of the scalability and flexibility that cloud computing offers. With seamless cloud integration, organizations can ensure that their data operations are highly available, resilient, and globally distributed.
Conclusion
In an era where data-driven decision-making is crucial, organizations need platforms that allow them to operate more efficiently and effectively. Databricks, combined with lakehouse architecture, is a powerful solution for transforming how businesses manage, analyze, and gain insights from their data. By combining the scalability of data lakes with the performance of data warehouses, Databricks enables organizations to accelerate their data operations, reduce costs, and ensure data quality and governance.
Whether you're looking to streamline data engineering workflows, implement real-time analytics, or accelerate machine learning model deployment, Databricks offers the tools and capabilities needed to optimize data management. With its robust, unified platform and focus on lakehouse architecture, Databricks empowers businesses to stay competitive in an increasingly data-driven world.
0 notes
Text
Introduction to Data Lakes and Data Warehouses
Introduction
Businesses generate vast amounts of data from various sources.
Understanding Data Lakes and Data Warehouses is crucial for effective data management.
This blog explores differences, use cases, and when to choose each approach.
1. What is a Data Lake?
A data lake is a centralized repository that stores structured, semi-structured, and unstructured data.
Stores raw data without predefined schema.
Supports big data processing and real-time analytics.
1.1 Key Features of Data Lakes
Scalability: Can store vast amounts of data.
Flexibility: Supports multiple data types (JSON, CSV, images, videos).
Cost-effective: Uses low-cost storage solutions.
Supports Advanced Analytics: Enables machine learning and AI applications.
1.2 Technologies Used in Data Lakes
Cloud-based solutions: AWS S3, Azure Data Lake Storage, Google Cloud Storage.
Processing engines: Apache Spark, Hadoop, Databricks.
Query engines: Presto, Trino, Amazon Athena.
1.3 Data Lake Use Cases
✅ Machine Learning & AI: Data scientists can process raw data for model training. ✅ IoT & Sensor Data Processing: Real-time storage and analysis of IoT device data. ✅ Log Analytics: Storing and analyzing logs from applications and systems.
2. What is a Data Warehouse?
A data warehouse is a structured repository optimized for querying and reporting.
Uses schema-on-write (structured data stored in predefined schemas).
Designed for business intelligence (BI) and analytics.
2.1 Key Features of Data Warehouses
Optimized for Queries: Structured format ensures faster analysis.
Supports Business Intelligence: Designed for dashboards and reporting.
ETL Process: Data is transformed before loading.
High Performance: Uses indexing and partitioning for fast queries.
2.2 Technologies Used in Data Warehouses
Cloud-based solutions: Snowflake, Amazon Redshift, Google BigQuery, Azure Synapse.
Traditional databases: Teradata, Oracle Exadata.
ETL Tools: Apache Nifi, AWS Glue, Talend.
2.3 Data Warehouse Use Cases
✅ Enterprise Reporting: Analyzing sales, finance, and marketing data. ✅ Fraud Detection: Banks use structured data to detect anomalies. ✅ Customer Segmentation: Retailers analyze customer behavior for personalized marketing.
3. Key Differences Between Data Lakes and Data Warehouses
4. Choosing Between a Data Lake and Data Warehouse
Use a Data Lake When:
You have raw, unstructured, or semi-structured data.
You need machine learning, IoT, or big data analytics.
You want low-cost, scalable storage.
Use a Data Warehouse When:
You need fast queries and structured data.
Your focus is on business intelligence (BI) and reporting.
You require data governance and compliance.
5. The Modern Approach: Data Lakehouse
Combines benefits of Data Lakes and Data Warehouses.
Provides structured querying with flexible storage.
Popular solutions: Databricks Lakehouse, Snowflake, Apache Iceberg.
Conclusion
Data Lakes are best for raw data and big data analytics.
Data Warehouses are ideal for structured data and business reporting.
Hybrid solutions (Lakehouses) are emerging to bridge the gap.
WEBSITE: https://www.ficusoft.in/data-science-course-in-chennai/
0 notes
Text
Scaling Your Data Mesh Architecture for maximum efficiency and interoperability
View On WordPress
#Azure Databricks#Big Data#Business Intelligence#Cloud Data Management#Collaborative Data Solutions#Data Analytics#Data Architecture#Data Compliance#Data Governance#Data management#Data Mesh#Data Operations#Data Security#Data Sharing Protocols#Databricks Lakehouse#Delta Sharing#Interoperability#Open Protocol#Real-time Data Sharing#Scalable Data Solutions
0 notes
Link
[ad_1] Join our daily and weekly newsletters for the latest updates and exclusive content on industry-leading AI coverage. Learn More German software giant SAP is pushing the bar on the data front to power next-gen AI use cases. The company today introduced Business Data Cloud (BDC), a new SaaS product that embraces lakehouse architecture to help teams enrich their SAP ecosystem data with external data assets from different source systems and drive long-term value. The product is the outcome of a landmark collaboration with data ecosystem major Databricks. Essentially, SAP BDC natively integrates capabilities and data from Databricks’ data intelligence platform. This removes the need for creating and maintaining complex pipelines and creates a harmonized data foundation for advanced AI agents and analytical workloads. Several enterprises, including Henkel, are using BDC to power their AI projects. SAP itself is using the enriched BDC to power a new era of Joule agents focused on specific domains like finance, service and sales. The development makes SAP another notable player, much like Microsoft and Salesforce, bolstering its data platform to lay down the foundation for AI. SAP’s revamped data foundation Over the years, SAP has established itself as one of the leading players in enterprise resource planning (ERP) with S4/HANA cloud and several mission-critical applications for finance, supply chain and human capital management. These apps produce petabyte-scale data with business context and have been powering AI and analytical value for teams, via the company’s business technology platform (BTP). So far, SAP BTP has had a ‘datasphere’ that allows enterprises to connect data from SAP with information from non-SAP systems and eventually link it with SAP analytics cloud and other internal tools for downstream applications. Now, the company is evolving this experience into the unified BDC, natively powered by Databricks. What SAP business data cloud has on offer What this means is that SAP is embracing lakehouse architecture, creating a unified foundation that combines all SAP data products — from finance, spend and supply chain data in SAP S/4HANA and SAP Ariba, to learning and talent data in SAP SuccessFactors — with structured and unstructured data from other varied yet business-critical systems, stored in Databricks. Once the data is unified (via zero-copy, bi-directional sharing), SAP BDC can leverage Databricks-specific capabilities for workloads like data warehousing, data engineering and AI, all governed by Databricks unity catalog. “We take all of these different data products, which are provisioned and managed by SAP…and we will persist them into the lakehouse of SAP business data cloud, in a harmonized data model,” Irfan Khan, president and CPO for SAP data and analytics, told VentureBeat. “This lakehouse will have Databricks capabilities for users to build upon.” Previously, said Khan, users who had a large percentage of their data in Databricks and SAP data in S4 or BW had to build and manage complex pipelines and replicate all the data assets to the SAP platform while rebuilding the entire semantics and the core data model at the same time. The approach took time and required them to keep their pipelines updated with changing data. However, with Databricks’ native integration, users have access to everything in one place and can directly do data engineering, data science and other tasks on top of the BDC. “In Datasphere, you had a means of doing a similar thing, but they were all customer-managed data products,” Khan explained. “So, you had to go into the data platform, select the data sources and build the data pipelines. Then, you had to figure out what to replicate. Here, it’s all managed by SAP.” What this means for enterprises At its core, this Databricks-powered product gives teams a faster, simpler way to unify and mobilize their business data assets locked within SAP and Databricks environments. The combined, semantically-enhanced data will pave the way for building next-gen AI applications aimed at different use cases. For instance, a team could use Databricks’ Mosaic AI capabilities to develop domain-specific AI agents that could use context from SAP’s business data as well as external Databricks-specific data to automate certain human capital management or supply chain functions. Notably, SAP itself is tapping this enhanced data foundation to power ready-to-use Joule agents aimed at automating tasks and accelerating workflows across sales, service and finance functions. These agents deeply understand end-to-end processes and collaborate to solve complex business problems. Beyond this, BDC will have an “insight apps” capability, which will allow users to connect their data products and AI models with external real-time data to deliver advanced analytics and planning across business functions. More data partners to come While the partnership underscores a big move for both Databricks and SAP, it is important to note that the Ali Ghodsi-led data major won’t be the only one bolstering BDC. According to Khan, data sharing and ecosystem openness are the company’s first design principles — and they will expand to other data platforms through their partner connect capabilities. This means an enterprise user will be able to choose the platform they prefer (or that they are locked into) and bi-directionally share data for targeted use cases. Daily insights on business use cases with VB Daily If you want to impress your boss, VB Daily has you covered. We give you the inside scoop on what companies are doing with generative AI, from regulatory shifts to practical deployments, so you can share insights for maximum ROI. Read our Privacy Policy Thanks for subscribing. Check out more VB newsletters here. An error occured. [ad_2] Source link
0 notes
Text
Databricks’ new updates aim to ease gen AI app and agent development
Data lakehouse provider Databricks is introducing four new updates to its portfolio to help enterprises have more control over the development of their agents and other generative AI-based applications. One of the new features launched as part of the updates is Centralized Governance, which is designed to help govern large language models, both open and closed source ones, within Mosaic AI…
0 notes
Text
Kadel Labs: Leading the Way as Databricks Consulting Partners
In today’s data-driven world, businesses are constantly seeking efficient ways to harness the power of big data. As organizations generate vast amounts of structured and unstructured data, they need advanced tools and expert guidance to extract meaningful insights. This is where Kadel Labs, a leading technology solutions provider, steps in. As Databricks Consulting Partners, Kadel Labs specializes in helping businesses leverage the Databricks Lakehouse platform to unlock the full potential of their data.
0 notes
Text
SAP TAPS DATABRICK to enhance the willingness of artificial intelligence with the new business cloud
Join daily and weekly newsletters to obtain the latest updates and exclusive content to cover the leading artificial intelligence in the industry. Learn more German software giant Bait The tape is pushed on the front of the data to run cases of artificial intelligence from the next generation. The company today foot Business Data Cloud (BDC), the new Saas product that embraces Lakehouse’s…
0 notes
Text
Databrick consulting services
Discover the transformative potential of Databricks with Xorbix Technologies, a leading Databricks consulting services provider. From AI and machine learning to data modernization and cloud migration, our certified Databricks engineers specialize in delivering custom solutions tailored to your unique business needs. Partner with us to leverage the Databricks Lakehouse Platform, Genie, and AutoML for streamlined analytics, seamless data governance, and actionable insights. Let us be your Databricks service provider company of choice!
0 notes
Text
两个甲骨文的“叛徒”,砸烂了数仓的旧世界
为什么Snowflake能够击败AWS Redshift、Google BigQuery这些“出道即王炸”的玩家?它的技术和策略到底有多高明?而面对Databricks以Lakehouse杀入市场的挑战,Snowflake的护城河是否足够坚固?后来,Snowflake又为什么有一段时间被资本所“抛弃”?
0 notes
Text
Databricks Certified Data Engineer Professional Practice Exam For Best Preparation
Are you aspiring to become a certified data engineer with Databricks? Passing the Databricks Certified Data Engineer Professional exam is a significant step in proving your advanced data engineering skills. To simplify your preparation, the latest Databricks Certified Data Engineer Professional Practice Exam from Cert007 is an invaluable resource. Designed to mimic the real exam, it provides comprehensive practice questions that will help you master the topics and build confidence. With Cert007’s reliable preparation material, you can approach the exam with ease and increase your chances of success.
Overview of the Databricks Certified Data Engineer Professional Exam
The Databricks Certified Data Engineer Professional exam evaluates your ability to leverage the Databricks platform for advanced data engineering tasks. You will be tested on a range of skills, including:
Utilizing Apache Spark, Delta Lake, and MLflow to manage and process large datasets.
Building and optimizing ETL pipelines.
Applying data modeling principles to structure data in a Lakehouse architecture.
Using developer tools such as the Databricks CLI and REST API.
Ensuring data pipeline security, reliability, and performance through monitoring, testing, and governance.
Successful candidates will demonstrate a solid understanding of Databricks tools and the capability to design secure, efficient, and robust pipelines for data engineering.
Exam Details
Number of Questions: 60 multiple-choice questions
Duration: 120 minutes
Cost: $200 per attempt
Primary Coding Language: Python (Delta Lake functionality references are in SQL)
Certification Validity: 2 years from the date of passing
Exam Objectives and Weightage
The exam content is divided into six key objectives:
Databricks Tooling (20%) Proficiency in Databricks developer tools, including the CLI, REST API, and notebooks.
Data Processing (30%) Deep understanding of data transformation, optimization, and real-time streaming tasks using Databricks.
Data Modeling (20%) Knowledge of structuring data effectively for analysis and reporting in a Lakehouse architecture.
Security and Governance (10%) Implementation of secure practices for managing data access, encryption, and auditing.
Monitoring and Logging (10%) Ability to use tools and techniques to monitor pipeline performance and troubleshoot issues.
Testing and Deployment (10%) Knowledge of building, testing, and deploying reliable data engineering solutions.
Preparation Tips for Databricks Certified Data Engineer Professional Exam
1. Leverage Cert007 Practice Exams
The Databricks Certified Data Engineer Professional Practice Exam by Cert007 is tailored to provide a hands-on simulation of the real exam. Practicing with these questions will sharpen your understanding of the key concepts and help you identify areas where additional study is needed.
2. Understand the Databricks Ecosystem
Develop a strong understanding of the core components of the Databricks platform, including Apache Spark, Delta Lake, and MLflow. Focus on how these tools integrate to create seamless data engineering workflows.
3. Study the Official Databricks Learning Pathway
Follow the official Data Engineer learning pathway provided by Databricks. This pathway offers structured courses and materials designed to prepare candidates for the certification exam.
4. Hands-On Practice
Set up your own Databricks environment and practice creating ETL pipelines, managing data in Delta Lake, and deploying models with MLflow. This hands-on experience will enhance your skills and reinforce theoretical knowledge.
5. Review Security and Governance Best Practices
Pay attention to secure data practices, including access control, encryption, and compliance requirements. Understanding governance within the Databricks platform is essential for this exam.
6. Time Management for the Exam
Since you’ll have 120 minutes to answer 60 questions, practice pacing yourself during the exam. Aim to spend no more than 2 minutes per question, leaving time to review your answers.
Conclusion
Becoming a Databricks Certified Data Engineer Professional validates your expertise in advanced data engineering using the Databricks platform. By leveraging high-quality resources like the Cert007 practice exams and committing to hands-on practice, you can confidently approach the exam and achieve certification. Remember to stay consistent with your preparation and focus on mastering the six key objectives to ensure your success.
Good luck on your journey to becoming a certified data engineering professional!
0 notes