#databricks
Explore tagged Tumblr posts
Text
Move over, Salesforce and Microsoft! Databricks is shaking things up with their game-changing AI/BI tool. Get ready for smarter, faster insights that leave the competition in the dust.
Who's excited to see what this powerhouse can do?
2 notes
·
View notes
Text
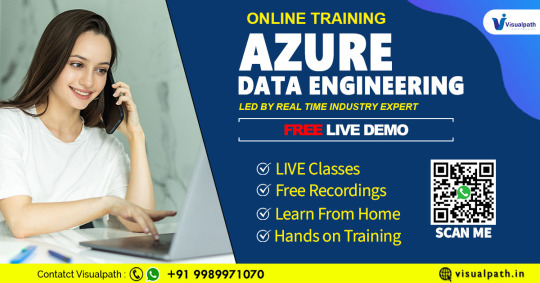
#dataengineer#onlinetraining#freedemo#cloudlearning#azuredatlake#Databricks#azuresynapse#AzureDataFactory#Azure#SQL#MySQL#NewTechnolgies#software#softwaredevelopment#visualpathedu#onlinecoaching#ADE#DataLake#datalakehouse#AzureDataEngineering
2 notes
·
View notes
Text
Navigating the Data Landscape: A Deep Dive into ScholarNest's Corporate Training
In the ever-evolving realm of data, mastering the intricacies of data engineering and PySpark is paramount for professionals seeking a competitive edge. ScholarNest's Corporate Training offers an immersive experience, providing a deep dive into the dynamic world of data engineering and PySpark.
Unlocking Data Engineering Excellence
Embark on a journey to become a proficient data engineer with ScholarNest's specialized courses. Our Data Engineering Certification program is meticulously crafted to equip you with the skills needed to design, build, and maintain scalable data systems. From understanding data architecture to implementing robust solutions, our curriculum covers the entire spectrum of data engineering.
Pioneering PySpark Proficiency
Navigate the complexities of data processing with PySpark, a powerful Apache Spark library. ScholarNest's PySpark course, hailed as one of the best online, caters to both beginners and advanced learners. Explore the full potential of PySpark through hands-on projects, gaining practical insights that can be applied directly in real-world scenarios.
Azure Databricks Mastery
As part of our commitment to offering the best, our courses delve into Azure Databricks learning. Azure Databricks, seamlessly integrated with Azure services, is a pivotal tool in the modern data landscape. ScholarNest ensures that you not only understand its functionalities but also leverage it effectively to solve complex data challenges.
Tailored for Corporate Success
ScholarNest's Corporate Training goes beyond generic courses. We tailor our programs to meet the specific needs of corporate environments, ensuring that the skills acquired align with industry demands. Whether you are aiming for data engineering excellence or mastering PySpark, our courses provide a roadmap for success.
Why Choose ScholarNest?
Best PySpark Course Online: Our PySpark courses are recognized for their quality and depth.
Expert Instructors: Learn from industry professionals with hands-on experience.
Comprehensive Curriculum: Covering everything from fundamentals to advanced techniques.
Real-world Application: Practical projects and case studies for hands-on experience.
Flexibility: Choose courses that suit your level, from beginner to advanced.
Navigate the data landscape with confidence through ScholarNest's Corporate Training. Enrol now to embark on a learning journey that not only enhances your skills but also propels your career forward in the rapidly evolving field of data engineering and PySpark.
#data engineering#pyspark#databricks#azure data engineer training#apache spark#databricks cloud#big data#dataanalytics#data engineer#pyspark course#databricks course training#pyspark training
3 notes
·
View notes
Text
Unlocking the Potential of AI: How Databricks Dolly is Democratizing LLMs
As the world continues to generate massive amounts of data, artificial intelligence (AI) is becoming increasingly important in helping businesses and organizations make sense of it all. One of the biggest challenges in AI development is the creation of la
As the world continues to generate massive amounts of data, artificial intelligence (AI) is becoming increasingly important in helping businesses and organizations make sense of it all. One of the biggest challenges in AI development is the creation of large language models that can process and analyze vast amounts of text data. That’s where Databricks Dolly comes in. This new project from…
View On WordPress
#AI#ChatGPT#Databricks#Databricks Dolly#Democratizing the LLM#Hugging Face#Large Language Model#LLM#Open Source LLM Model
2 notes
·
View notes
Text
"من الغباء الاكتتاب العام هذا العام": يشرح الرئيس التنفيذي لشركة Databricks سبب انتظاره للاكتتاب العام
اشراق العالم 24 متابعات تقنية: نقدم لكم في اشراق العالم 24 خبر بعنوان “”من الغباء الاكتتاب العام هذا العام”: يشرح الرئيس التنفيذي لشركة Databricks سبب انتظاره للاكتتاب العام ” نترككم مع محتوى الخبر أنهت Databricks للتو واحدة من أكبر جولات التمويل على الإطلاق، حيث جمعت مبلغًا مذهلاً قدره 10 مليارات دولار من رأس المال الجديد. وبطبيعة الحال، سارع مستثمرو التكنولوجيا إلى التساؤل عما يعنيه هذا…
0 notes
Text
His Startup Is Now Worth $62 Billion. It Gave Away Its First Product Free.

0 notes
Text
Topul Milionarilor Români în 2024: Cine Sunt și Ce Averi Impresionante Au Acumulat?
În 2024, averile miliardarilor români au atins noi recorduri, depășind pentru prima dată pragul impresionant de 100 de miliarde de lei. În ciuda provocărilor economice și politice globale, cei mai înstăriți români continuă să domine sectoare precum bricolajul, tehnologia și securitatea cibernetică. Clasamentul, publicat de Forbes România, evidențiază creșteri semnificative față de anii…
#actualitate#Adrian Pavăl#averi record România#Bitdefender#cei mai bogați români#Daniel Dines#Databricks#Dedeman#Dragoș Pavăl#economie#economie România#Știri#filme#Forbes România 2024#Ion Țiriac#lideri afaceri#lideri economici#miliardari România#milionari români 2024#Romania#securitate cibernetică#top averi#top bogați România#top miliardari#UiPath
0 notes
Text
2024 Guide: 10 Must-Have Skills for Hiring a Databricks Data Engineer Associate
Find the top 10 skills employers should prioritize when hiring a Databricks Data Engineer Associate in 2024. Build a future-ready team.
0 notes
Text
Top 5 Challenges of Data Validation in Databricks and How to Overcome Them
Databricks data validation is a critical step in the data analysis process, especially considering the growing reliance on big data and AI. While Databricks offers a powerful platform for data processing and analytics, flawed data can lead to inaccurate results and misleading conclusions. Here’s how to ensure your Databricks data is trustworthy and ready for analysis. Read the full post on "Challenges of Data Validation in Databricks".
1 note
·
View note
Text
Boost Business Efficiency with a Databricks Certified Data Engineer Associate
Learn how a Databricks Certified Data Engineer Associate can revolutionize your data workflows, leading to enhanced scalability, faster processing times, and improved analytics. This certification ensures that your business remains competitive in a data-driven world.
0 notes
Text
🎯 Databricks Sues Patent Holders Over Alleged Extortion Scheme
#ipconsultinggroup#Databricks#Patent_Holders#Alleged#Extraction_Scheme#Intellectualproperty_News#USA
0 notes
Text
Databricks Consulting Services & Partner Solutions | Unlocking the Power of Data
As businesses increasingly rely on data-driven insights to drive their decision-making processes, tools like Databricks have emerged as vital platforms for big data analytics and machine learning. Databricks unifies data engineering, data science, and analytics under one platform, enabling businesses to process vast amounts of data with speed and efficiency. For organizations looking to fully leverage this platform, Databricks consulting services and partner solutions provide the expertise necessary to maximize its capabilities.
What is Databricks?
Databricks is a cloud-based platform built on Apache Spark, offering a unified data analytics workspace that simplifies data workflows. It allows organizations to build and deploy scalable data pipelines, collaborate on big data projects, and run machine learning models with enhanced performance.
Key Benefits of Databricks
Unified Analytics Platform: Databricks combines data engineering, data science, and business analytics into a single workspace. This allows different teams to collaborate seamlessly on data projects, reducing time-to-insight and fostering innovation.
Scalable Data Processing: Built on Apache Spark, Databricks enables businesses to process and analyze large volumes of data in real-time, allowing for the swift processing of complex datasets.
Machine Learning at Scale: Databricks comes equipped with built-in machine learning tools, empowering organizations to develop, train, and deploy models across a scalable infrastructure. This accelerates the development of AI and ML solutions.
Seamless Integration: Databricks easily integrates with cloud platforms such as Microsoft Azure, AWS, and Google Cloud, enabling businesses to work within their preferred cloud ecosystems.
Why Databricks Consulting Services are Essential
While Databricks is a powerful platform, its full potential is unlocked with the help of expert guidance. Databricks consulting services provide the necessary skills and knowledge to ensure a smooth and effective implementation, helping companies get the most out of their data infrastructure.
Here are the key benefits of working with Databricks consultants:
Tailored Implementations: Databricks consulting partners assess your current data architecture and customize the platform to suit your unique business needs. Whether you’re looking to streamline data workflows or accelerate analytics, consultants develop tailored solutions that align with your goals.
Data Engineering Expertise: Implementing Databricks requires deep knowledge of data engineering best practices. Consulting services ensure that your data pipelines are built efficiently, delivering clean, reliable data to stakeholders.
Optimized Machine Learning Workflows: Databricks consultants help businesses optimize their machine learning models, from data preparation to deployment. This reduces errors and accelerates time to market for AI-driven solutions.
End-to-End Support: From initial setup to post-deployment support, consulting services provide end-to-end guidance. This includes everything from cloud integration to data security and governance, ensuring that your Databricks environment is optimized for performance.
Training and Enablement: Beyond implementation, consultants offer training programs to upskill your internal teams. This ensures your staff can efficiently manage and expand Databricks capabilities as your business grows.
Partner Solutions for Seamless Databricks Integration
In addition to consulting services, partner solutions play a crucial role in maximizing the potential of Databricks. These solutions enhance Databricks’ functionality by providing complementary services and tools, including:
Cloud Integrations: Seamless integration with cloud providers such as AWS, Microsoft Azure, and Google Cloud helps businesses manage their data lakes with improved scalability and cost-efficiency.
Data Security: Partners provide robust security solutions that protect sensitive data and ensure compliance with industry regulations.
Advanced Analytics: Partner solutions enhance Databricks’ capabilities by integrating advanced analytics tools and AI frameworks for deeper insights and automation.
Why Choose Databricks Consulting Services?
With Databricks consulting services, businesses gain access to a wealth of expertise and resources that enable them to harness the full power of the Databricks platform. Whether it’s optimizing big data workflows, improving collaboration across teams, or accelerating machine learning initiatives, consulting partners provide the strategic guidance needed to succeed.
When choosing a Databricks consulting partner, it’s important to look for:
Proven Experience: Ensure the partner has a track record of successful Databricks implementations across multiple industries.
Technical Expertise: Consultants should have deep knowledge of Apache Spark, machine learning, and cloud platforms.
Comprehensive Services: Choose a partner that offers a full range of services, from implementation and support to training and optimization.
Conclusion
Databricks consulting services and partner solutions provide businesses with the expertise and tools needed to unlock the full potential of their data. By collaborating with skilled consultants, companies can enhance their data management processes, build scalable data solutions, and achieve actionable insights faster than ever before.
If you're ready to elevate your data strategy with Databricks consulting services, contact Feathersoft Inc Solutions today for expert guidance.
#Databricks#BigData#DataAnalytics#MachineLearning#DataEngineering#DataScience#DatabricksConsulting#ApacheSpark#CloudComputing#DataPipelines#AnalyticsSolutions#DataStrategy#CloudData#BusinessIntelligence
0 notes
Text

Contact CT Shift - Automate Migration from SAS (celebaltech.com)
0 notes
Text
From Data to Decisions: Empowering Teams with Databricks AI/BI
🚀 Unlock the Power of Data with Databricks AI/BI! 🚀 Imagine a world where your entire team can access data insights in real-time, without needing to be data experts. Databricks AI/BI is making this possible with powerful features like conversational AI
In today’s business world, data is abundant—coming from sources like customer interactions, sales metrics, and supply chain information. Yet many organizations still struggle to transform this data into actionable insights. Teams often face siloed systems, complex analytics processes, and delays that hinder timely, data-driven decisions. Databricks AI/BI was designed with these challenges in…
#AI/BI#artificial intelligence#BI tools#Business Intelligence#Conversational AI#Data Analytics#data democratization#Data Governance#Data Insights#Data Integration#Data Visualization#data-driven decisions#Databricks#finance#Genie AI assistant#healthcare#logistics#low-code dashboards#predictive analytics#self-service analytics
0 notes
Text
Tracking Large Language Models (LLM) with MLflow : A Complete Guide
New Post has been published on https://thedigitalinsider.com/tracking-large-language-models-llm-with-mlflow-a-complete-guide/
Tracking Large Language Models (LLM) with MLflow : A Complete Guide
As Large Language Models (LLMs) grow in complexity and scale, tracking their performance, experiments, and deployments becomes increasingly challenging. This is where MLflow comes in – providing a comprehensive platform for managing the entire lifecycle of machine learning models, including LLMs.
In this in-depth guide, we’ll explore how to leverage MLflow for tracking, evaluating, and deploying LLMs. We’ll cover everything from setting up your environment to advanced evaluation techniques, with plenty of code examples and best practices along the way.
Functionality of MLflow in Large Language Models (LLMs)
MLflow has become a pivotal tool in the machine learning and data science community, especially for managing the lifecycle of machine learning models. When it comes to Large Language Models (LLMs), MLflow offers a robust suite of tools that significantly streamline the process of developing, tracking, evaluating, and deploying these models. Here’s an overview of how MLflow functions within the LLM space and the benefits it provides to engineers and data scientists.
Tracking and Managing LLM Interactions
MLflow’s LLM tracking system is an enhancement of its existing tracking capabilities, tailored to the unique needs of LLMs. It allows for comprehensive tracking of model interactions, including the following key aspects:
Parameters: Logging key-value pairs that detail the input parameters for the LLM, such as model-specific parameters like top_k and temperature. This provides context and configuration for each run, ensuring that all aspects of the model’s configuration are captured.
Metrics: Quantitative measures that provide insights into the performance and accuracy of the LLM. These can be updated dynamically as the run progresses, offering real-time or post-process insights.
Predictions: Capturing the inputs sent to the LLM and the corresponding outputs, which are stored as artifacts in a structured format for easy retrieval and analysis.
Artifacts: Beyond predictions, MLflow can store various output files such as visualizations, serialized models, and structured data files, allowing for detailed documentation and analysis of the model’s performance.
This structured approach ensures that all interactions with the LLM are meticulously recorded, providing a comprehensive lineage and quality tracking for text-generating models.
Evaluation of LLMs
Evaluating LLMs presents unique challenges due to their generative nature and the lack of a single ground truth. MLflow simplifies this with specialized evaluation tools designed for LLMs. Key features include:
Versatile Model Evaluation: Supports evaluating various types of LLMs, whether it’s an MLflow pyfunc model, a URI pointing to a registered MLflow model, or any Python callable representing your model.
Comprehensive Metrics: Offers a range of metrics tailored for LLM evaluation, including both SaaS model-dependent metrics (e.g., answer relevance) and function-based metrics (e.g., ROUGE, Flesch Kincaid).
Predefined Metric Collections: Depending on the use case, such as question-answering or text-summarization, MLflow provides predefined metrics to simplify the evaluation process.
Custom Metric Creation: Allows users to define and implement custom metrics to suit specific evaluation needs, enhancing the flexibility and depth of model evaluation.
Evaluation with Static Datasets: Enables evaluation of static datasets without specifying a model, which is useful for quick assessments without rerunning model inference.
Deployment and Integration
MLflow also supports seamless deployment and integration of LLMs:
MLflow Deployments Server: Acts as a unified interface for interacting with multiple LLM providers. It simplifies integrations, manages credentials securely, and offers a consistent API experience. This server supports a range of foundational models from popular SaaS vendors as well as self-hosted models.
Unified Endpoint: Facilitates easy switching between providers without code changes, minimizing downtime and enhancing flexibility.
Integrated Results View: Provides comprehensive evaluation results, which can be accessed directly in the code or through the MLflow UI for detailed analysis.
MLflow is a comprehensive suite of tools and integrations makes it an invaluable asset for engineers and data scientists working with advanced NLP models.
Setting Up Your Environment
Before we dive into tracking LLMs with MLflow, let’s set up our development environment. We’ll need to install MLflow and several other key libraries:
pip install mlflow>=2.8.1 pip install openai pip install chromadb==0.4.15 pip install langchain==0.0.348 pip install tiktoken pip install 'mlflow[genai]' pip install databricks-sdk --upgrade
After installation, it’s a good practice to restart your Python environment to ensure all libraries are properly loaded. In a Jupyter notebook, you can use:
import mlflow import chromadb print(f"MLflow version: mlflow.__version__") print(f"ChromaDB version: chromadb.__version__")
This will confirm the versions of key libraries we’ll be using.
Understanding MLflow’s LLM Tracking Capabilities
MLflow’s LLM tracking system builds upon its existing tracking capabilities, adding features specifically designed for the unique aspects of LLMs. Let’s break down the key components:
Runs and Experiments
In MLflow, a “run” represents a single execution of your model code, while an “experiment” is a collection of related runs. For LLMs, a run might represent a single query or a batch of prompts processed by the model.
Key Tracking Components
Parameters: These are input configurations for your LLM, such as temperature, top_k, or max_tokens. You can log these using mlflow.log_param() or mlflow.log_params().
Metrics: Quantitative measures of your LLM’s performance, like accuracy, latency, or custom scores. Use mlflow.log_metric() or mlflow.log_metrics() to track these.
Predictions: For LLMs, it’s crucial to log both the input prompts and the model’s outputs. MLflow stores these as artifacts in CSV format using mlflow.log_table().
Artifacts: Any additional files or data related to your LLM run, such as model checkpoints, visualizations, or dataset samples. Use mlflow.log_artifact() to store these.
Let’s look at a basic example of logging an LLM run:
This example demonstrates logging parameters, metrics, and the input/output as a table artifact.
import mlflow import openai def query_llm(prompt, max_tokens=100): response = openai.Completion.create( engine="text-davinci-002", prompt=prompt, max_tokens=max_tokens ) return response.choices[0].text.strip() with mlflow.start_run(): prompt = "Explain the concept of machine learning in simple terms." # Log parameters mlflow.log_param("model", "text-davinci-002") mlflow.log_param("max_tokens", 100) # Query the LLM and log the result result = query_llm(prompt) mlflow.log_metric("response_length", len(result)) # Log the prompt and response mlflow.log_table("prompt_responses", "prompt": [prompt], "response": [result]) print(f"Response: result")
Deploying LLMs with MLflow
MLflow provides powerful capabilities for deploying LLMs, making it easier to serve your models in production environments. Let’s explore how to deploy an LLM using MLflow’s deployment features.
Creating an Endpoint
First, we’ll create an endpoint for our LLM using MLflow’s deployment client:
import mlflow from mlflow.deployments import get_deploy_client # Initialize the deployment client client = get_deploy_client("databricks") # Define the endpoint configuration endpoint_name = "llm-endpoint" endpoint_config = "served_entities": [ "name": "gpt-model", "external_model": "name": "gpt-3.5-turbo", "provider": "openai", "task": "llm/v1/completions", "openai_config": "openai_api_type": "azure", "openai_api_key": "secrets/scope/openai_api_key", "openai_api_base": "secrets/scope/openai_api_base", "openai_deployment_name": "gpt-35-turbo", "openai_api_version": "2023-05-15", , , ], # Create the endpoint client.create_endpoint(name=endpoint_name, config=endpoint_config)
This code sets up an endpoint for a GPT-3.5-turbo model using Azure OpenAI. Note the use of Databricks secrets for secure API key management.
Testing the Endpoint
Once the endpoint is created, we can test it:
<div class="relative flex flex-col rounded-lg"> response = client.predict( endpoint=endpoint_name, inputs="prompt": "Explain the concept of neural networks briefly.","max_tokens": 100,,) print(response)
This will send a prompt to our deployed model and return the generated response.
Evaluating LLMs with MLflow
Evaluation is crucial for understanding the performance and behavior of your LLMs. MLflow provides comprehensive tools for evaluating LLMs, including both built-in and custom metrics.
Preparing Your LLM for Evaluation
To evaluate your LLM with mlflow.evaluate(), your model needs to be in one of these forms:
An mlflow.pyfunc.PyFuncModel instance or a URI pointing to a logged MLflow model.
A Python function that takes string inputs and outputs a single string.
An MLflow Deployments endpoint URI.
Set model=None and include model outputs in the evaluation data.
Let’s look at an example using a logged MLflow model:
import mlflow import openai with mlflow.start_run(): system_prompt = "Answer the following question concisely." logged_model_info = mlflow.openai.log_model( model="gpt-3.5-turbo", task=openai.chat.completions, artifact_path="model", messages=[ "role": "system", "content": system_prompt, "role": "user", "content": "question", ], ) # Prepare evaluation data eval_data = pd.DataFrame( "question": ["What is machine learning?", "Explain neural networks."], "ground_truth": [ "Machine learning is a subset of AI that enables systems to learn and improve from experience without explicit programming.", "Neural networks are computing systems inspired by biological neural networks, consisting of interconnected nodes that process and transmit information." ] ) # Evaluate the model results = mlflow.evaluate( logged_model_info.model_uri, eval_data, targets="ground_truth", model_type="question-answering", ) print(f"Evaluation metrics: results.metrics")
This example logs an OpenAI model, prepares evaluation data, and then evaluates the model using MLflow’s built-in metrics for question-answering tasks.
Custom Evaluation Metrics
MLflow allows you to define custom metrics for LLM evaluation. Here’s an example of creating a custom metric for evaluating the professionalism of responses:
from mlflow.metrics.genai import EvaluationExample, make_genai_metric professionalism = make_genai_metric( name="professionalism", definition="Measure of formal and appropriate communication style.", grading_prompt=( "Score the professionalism of the answer on a scale of 0-4:n" "0: Extremely casual or inappropriaten" "1: Casual but respectfuln" "2: Moderately formaln" "3: Professional and appropriaten" "4: Highly formal and expertly crafted" ), examples=[ EvaluationExample( input="What is MLflow?", output="MLflow is like your friendly neighborhood toolkit for managing ML projects. It's super cool!", score=1, justification="The response is casual and uses informal language." ), EvaluationExample( input="What is MLflow?", output="MLflow is an open-source platform for the machine learning lifecycle, including experimentation, reproducibility, and deployment.", score=4, justification="The response is formal, concise, and professionally worded." ) ], model="openai:/gpt-3.5-turbo-16k", parameters="temperature": 0.0, aggregations=["mean", "variance"], greater_is_better=True, ) # Use the custom metric in evaluation results = mlflow.evaluate( logged_model_info.model_uri, eval_data, targets="ground_truth", model_type="question-answering", extra_metrics=[professionalism] ) print(f"Professionalism score: results.metrics['professionalism_mean']")
This custom metric uses GPT-3.5-turbo to score the professionalism of responses, demonstrating how you can leverage LLMs themselves for evaluation.
Advanced LLM Evaluation Techniques
As LLMs become more sophisticated, so do the techniques for evaluating them. Let’s explore some advanced evaluation methods using MLflow.
Retrieval-Augmented Generation (RAG) Evaluation
RAG systems combine the power of retrieval-based and generative models. Evaluating RAG systems requires assessing both the retrieval and generation components. Here’s how you can set up a RAG system and evaluate it using MLflow:
from langchain.document_loaders import WebBaseLoader from langchain.text_splitter import CharacterTextSplitter from langchain.embeddings import OpenAIEmbeddings from langchain.vectorstores import Chroma from langchain.chains import RetrievalQA from langchain.llms import OpenAI # Load and preprocess documents loader = WebBaseLoader(["https://mlflow.org/docs/latest/index.html"]) documents = loader.load() text_splitter = CharacterTextSplitter(chunk_size=1000, chunk_overlap=0) texts = text_splitter.split_documents(documents) # Create vector store embeddings = OpenAIEmbeddings() vectorstore = Chroma.from_documents(texts, embeddings) # Create RAG chain llm = OpenAI(temperature=0) qa_chain = RetrievalQA.from_chain_type( llm=llm, chain_type="stuff", retriever=vectorstore.as_retriever(), return_source_documents=True ) # Evaluation function def evaluate_rag(question): result = qa_chain("query": question) return result["result"], [doc.page_content for doc in result["source_documents"]] # Prepare evaluation data eval_questions = [ "What is MLflow?", "How does MLflow handle experiment tracking?", "What are the main components of MLflow?" ] # Evaluate using MLflow with mlflow.start_run(): for question in eval_questions: answer, sources = evaluate_rag(question) mlflow.log_param(f"question", question) mlflow.log_metric("num_sources", len(sources)) mlflow.log_text(answer, f"answer_question.txt") for i, source in enumerate(sources): mlflow.log_text(source, f"source_question_i.txt") # Log custom metrics mlflow.log_metric("avg_sources_per_question", sum(len(evaluate_rag(q)[1]) for q in eval_questions) / len(eval_questions))
This example sets up a RAG system using LangChain and Chroma, then evaluates it by logging questions, answers, retrieved sources, and custom metrics to MLflow.
The way you chunk your documents can significantly impact RAG performance. MLflow can help you evaluate different chunking strategies:
This script evaluates different combinations of chunk sizes, overlaps, and splitting methods, logging the results to MLflow for easy comparison.
MLflow provides various ways to visualize your LLM evaluation results. Here are some techniques:
You can create custom visualizations of your evaluation results using libraries like Matplotlib or Plotly, then log them as artifacts:
This function creates a line plot comparing a specific metric across multiple runs and logs it as an artifact.
#2023#ai#AI Tools 101#Analysis#API#approach#Artificial Intelligence#azure#azure openai#Behavior#code#col#Collections#communication#Community#comparison#complexity#comprehensive#computing#computing systems#content#credentials#custom metrics#data#data science#databricks#datasets#deploying#deployment#development
0 notes
Text
Updates to Azure AI, Phi 3 Fine tuning, And gen AI models

Introducing new generative AI models, Phi 3 fine tuning, and other Azure AI enhancements to enable businesses to scale and personalise AI applications.
All sectors are being transformed by artificial intelligence, which also creates fresh growth and innovation opportunities. But developing and deploying artificial intelligence applications at scale requires a reliable and flexible platform capable of handling the complex and varied needs of modern companies and allowing them to construct solutions grounded on their organisational data. They are happy to share the following enhancements to enable developers to use the Azure AI toolchain to swiftly and more freely construct customised AI solutions:
Developers can rapidly and simply customise the Phi-3-mini and Phi-3-medium models for cloud and edge scenarios with serverless fine-tuning, eliminating the need to schedule computing.
Updates to Phi-3-mini allow developers to create with a more performant model without incurring additional costs. These updates include a considerable improvement in core quality, instruction-following, and organised output.
This month, OpenAI (GPT-4o small), Meta (Llama 3.1 405B), and Mistral (Large 2) shipped their newest models to Azure AI on the same day, giving clients more options and flexibility.
Value unlocking via customised and innovative models
Microsoft unveiled the Microsoft Phi-3 line of compact, open models in April. Compared to models of the same size and the next level up, Phi-3 models are their most powerful and economical small language models (SLMs). Phi 3 Fine tuning a tiny model is a wonderful alternative without losing efficiency, as developers attempt to customise AI systems to match unique business objectives and increase the quality of responses. Developers may now use their data to fine-tune Phi-3-mini and Phi-3-medium, enabling them to create AI experiences that are more affordable, safe, and relevant to their users.
Phi-3 models are well suited for fine-tuning to improve base model performance across a variety of scenarios, such as learning a new skill or task (e.g., tutoring) or improving consistency and quality of the response (e.g., tone or style of responses in chat/Q&A). This is because of their small compute footprint and compatibility with clouds and edges. Phi-3 is already being modified for new use cases.
Microsoft and Khan Academy are collaborating to enhance resources for educators and learners worldwide. As part of the partnership, Khan Academy is experimenting with Phi-3 to enhance math tutoring and leverages Azure OpenAI Service to power Khanmigo for Teachers, a pilot AI-powered teaching assistant for educators in 44 countries. A study from Khan Academy, which includes benchmarks from an improved version of Phi-3, shows how various AI models perform when assessing mathematical accuracy in tutoring scenarios.
According to preliminary data, Phi-3 fared better than the majority of other top generative AI models at identifying and fixing mathematical errors made by students.
Additionally, they have optimised Phi-3 for the gadget. To provide developers with a strong, reliable foundation for creating apps with safe, secure AI experiences, they launched Phi Silica in June. Built specifically for the NPUs in Copilot+ PCs, Phi Silica expands upon the Phi family of models. The state-of-the-art short language model (SLM) for the Neural Processing Unit (NPU) and shipping inbox is exclusive to Microsoft Windows.
Today, you may test Phi 3 fine tuning in Azure AI
Azure AI’s Models-as-a-Service (serverless endpoint) feature is now widely accessible. Additionally, developers can now rapidly and simply begin developing AI applications without having to worry about managing underlying infrastructure thanks to the availability of Phi-3-small via a serverless endpoint.
The multi-modal Phi-3 model, Phi-3-vision, was unveiled at Microsoft Build and may be accessed via the Azure AI model catalogue. It will also soon be accessible through a serverless endpoint. While Phi-3-vision (4.2B parameter) has also been optimised for chart and diagram interpretation and may be used to produce insights and answer queries, Phi-3-small (7B parameter) is offered in two context lengths, 128K and 8K.
The community’s response to Phi-3 is excellent. Last month, they launched an update for Phi-3-mini that significantly enhances the core quality and training after. After the model was retrained, support for structured output and instruction following significantly improved.They also added support for |system|> prompts, enhanced reasoning capability, and enhanced the quality of multi-turn conversations.
They also keep enhancing the safety of Phi-3. In order to increase the safety of the Phi-3 models, Microsoft used an iterative “break-fix” strategy that included vulnerability identification, red teaming, and several iterations of testing and improvement. This approach was recently highlighted in a research study. By using this strategy, harmful content was reduced by 75% and the models performed better on responsible AI benchmarks.
Increasing model selection; around 1600 models are already accessible in Azure AI They’re dedicated to providing the widest range of open and frontier models together with cutting-edge tooling through Azure AI in order to assist clients in meeting their specific cost, latency, and design requirements. Since the debut of the Azure AI model catalogue last year, over 1,600 models from providers such as AI21, Cohere, Databricks, Hugging Face, Meta, Mistral, Microsoft Research, OpenAI, Snowflake, Stability AI, and others have been added, giving us the widest collection to date. This month, they added Mistral Large 2, Meta Llama 3.1 405B, and OpenAI’s GPT-4o small via Azure OpenAI Service.
Keeping up the good work, they are happy to announce that Cohere Rerank is now accessible on Azure. Using Azure to access Cohere’s enterprise-ready language models Businesses can easily, consistently, and securely integrate state-of-the-art semantic search technology into their applications because to AI’s strong infrastructure. With the help of this integration, users may provide better search results in production by utilising the scalability and flexibility of Azure in conjunction with the highly effective and performant language models from Cohere.
With Cohere Rerank, Atomicwork, a digital workplace experience platform and a seasoned Azure user, has greatly improved its IT service management platform. Atomicwork has enhanced search relevancy and accuracy by incorporating the model into Atom AI, their AI digital assistant, hence offering quicker, more accurate responses to intricate IT help enquiries. Enterprise-wide productivity has increased as a result of this integration, which has simplified IT processes.
Read more on govindhtech.com
#AzureAI#Phi3#gen#AImodels#generativeAImodels#OpenAi#AzureAImodels#ai21#artificialintelligence#Llama31405B#slms#Phi3mini#smalllanguagemodels#AzureOpenAIService#npus#Databricks#technology#technews#news#govindhtech
0 notes