#DataSources
Explore tagged Tumblr posts
Text
Utilize Dell Data Lakehouse To Revolutionize Data Management
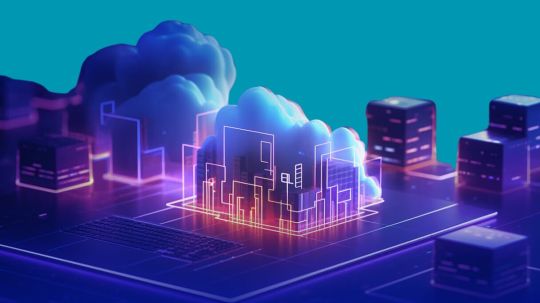
Introducing the Most Recent Upgrades to the Dell Data Lakehouse. With the help of automatic schema discovery, Apache Spark, and other tools, your team can transition from regular data administration to creativity.
Dell Data Lakehouse
Businesses’ data management plans are becoming more and more important as they investigate the possibilities of generative artificial intelligence (GenAI). Data quality, timeliness, governance, and security were found to be the main obstacles to successfully implementing and expanding AI in a recent MIT Technology Review Insights survey. It’s evident that having the appropriate platform to arrange and use data is just as important as having data itself.
As part of the AI-ready Data Platform and infrastructure capabilities with the Dell AI Factory, to present the most recent improvements to the Dell Data Lakehouse in collaboration with Starburst. These improvements are intended to empower IT administrators and data engineers alike.
Dell Data Lakehouse Sparks Big Data with Apache Spark
An approach to a single platform that can streamline big data processing and speed up insights is Dell Data Lakehouse + Apache Spark.
Earlier this year, it unveiled the Dell Data Lakehouse to assist address these issues. You can now get rid of data silos, unleash performance at scale, and democratize insights with a turnkey data platform that combines Dell’s AI-optimized hardware with a full-stack software suite and is driven by Starburst and its improved Trino-based query engine.
Through the Dell AI Factory strategy, this are working with Starburst to continue pushing the boundaries with cutting-edge solutions to help you succeed with AI. In addition to those advancements, its are expanding the Dell Data Lakehouse by introducing a fully managed, deeply integrated Apache Spark engine that completely reimagines data preparation and analytics.
Spark’s industry-leading data processing capabilities are now fully integrated into the platform, marking a significant improvement. The Dell Data Lakehouse provides unmatched support for a variety of analytics and AI-driven workloads with to Spark and Trino’s collaboration. It brings speed, scale, and innovation together under one roof, allowing you to deploy the appropriate engine for the right workload and manage everything with ease from the same management console.
Best-in-Class Connectivity to Data Sources
In addition to supporting bespoke Trino connections for special and proprietary data sources, its platform now interacts with more than 50 connectors with ease. The Dell Data Lakehouse reduces data transfer by enabling ad-hoc and interactive analysis across dispersed data silos with a single point of entry to various sources. Users may now extend their access into their distributed data silos from databases like Cassandra, MariaDB, and Redis to additional sources like Google Sheets, local files, or even a bespoke application within your environment.
External Engine Access to Metadata
It have always supported Iceberg as part of its commitment to an open ecology. By allowing other engines like Spark and Flink to safely access information in the Dell Data Lakehouse, it are further furthering to commitment. With optional security features like Transport Layer Security (TLS) and Kerberos, this functionality enables better data discovery, processing, and governance.
Improved Support Experience
Administrators may now produce and download a pre-compiled bundle of full-stack system logs with ease with to it improved support capabilities. By offering a thorough evaluation of system condition, this enhances the support experience by empowering Dell support personnel to promptly identify and address problems.
Automated Schema Discovery
The most recent upgrade simplifies schema discovery, enabling you to find and add data schemas automatically with little assistance from a human. This automation lowers the possibility of human mistake in data integration while increasing efficiency. Schema discovery, for instance, finds the newly added files so that users in the Dell Data Lakehouse may query them when a logging process generates a new log file every hour, rolling over from the log file from the previous hour.
Consulting Services
Use it Professional Services to optimize your Dell Data Lakehouse for better AI results and strategic insights. The professionals will assist with catalog metadata, onboarding data sources, implementing your Data Lakehouse, and streamlining operations by optimizing data pipelines.
Start Exploring
The Dell Demo Center to discover the Dell Data Lakehouse with carefully chosen laboratories in a virtual environment. Get in touch with your Dell account executive to schedule a visit to the Customer Solution Centers in Round Rock, Texas, and Cork, Ireland, for a hands-on experience. You may work with professionals here for a technical in-depth and design session.
Looking Forward
It will be integrating with Apache Spark in early 2025. Large volumes of structured, semi-structured, and unstructured data may be processed for AI use cases in a single environment with to this integration. To encourage you to keep investigating how the Dell Data Lakehouse might satisfy your unique requirements and enable you to get the most out of your investment.
Read more on govindhtech.com
#UtilizeDell#DataLakehouse#apacheSpark#Flink#RevolutionizeDataManagement#DellAIFactory#generativeartificialintelligence#GenAI#Cassandra#SchemaDiscovery#Metadata#DataSources#dell#technology#technews#news#govindhtech
0 notes
Text
Types of Sources
Normally Data Source is a point of Origin from where we can expect data .it might be from any Point. Inside System Outside System. Entity-Backed: if the Data For this Record Comes Directly from a Database Table or View i.e. a database entity. Normally view is seen by Appian as just another database table i.e. tables and views are both classed as entities. You can create an Appian record (or…
View On WordPress
0 notes
Text
Data preparation tools enable organizations to identify, clean, and convert raw datasets from various data sources to assist data professionals in performing data analysis and gaining valuable insights using machine learning (ML) algorithms and analytics tools.
#DataPreparation#RawData#DataSources#DataCleaning#DataConversion#DataAnalysis#MachineLearning#AnalyticsTools#BusinessAnalysis#DataCleansing#DataValidation#DataTransformation#Automation#DataInsights
0 notes
Photo
Are you struggling to manage your added data sources in your Data Studio account? Don't worry, we've got you covered! In this step-by-step guide, we'll show you exactly how to effectively manage your added data sources in a hassle-free manner: Step 1: Log in to your Data Studio account and click on the "Data Sources" tab on the left-hand side of the screen. Step 2: Once you're on the "Data Sources" page, you'll be able to see all the data sources that you've added to your account. Select the data source that you want to manage. Step 3: You'll now be taken to the data source details page where you can see all the fields that are available for this particular data source. From here, you can make any necessary edits to the data source. Step 4: If you want to remove a data source from your account, simply click on the "Remove" button at the bottom of the page. You'll be prompted to confirm your decision before the data source is permanently deleted from your account. Step 5: Congratulations, you've successfully managed your added data sources in your Data Studio account! Don't forget to check back periodically to keep your data up-to-date and accurate. If you're looking for a tool to make managing your data sources even easier, check out https://bitly.is/46zIp8t https://bit.ly/3JGvKXH, you can streamline your data management process and make informed decisions based on real-time data insights. So, what are you waiting for? Start effectively managing your added data sources today and see the impact it can have on your business!
#DataStudio#DataSources#DataManagement#RealTimeDataInsights#StreamlineYourProcess#MakeInformedDecisions#DataAnalytics#Marketing#Education
0 notes
Text
youtube
0 notes
Text
Integrate Google Sheets as a Data Source in AIV for Real-Time Data Analytics
In today’s data-driven world, seamless integration between tools can make or break your analytics workflow. For those using AIV or One AIV for data analytics, integrating Google Sheets as a data source allows real-time access to spreadsheet data, bringing powerful insights into your analysis. This guide will walk you through how to connect Google Sheets to AIV, giving you a direct pipeline for real-time analytics with AIV or One AIV. Follow this step-by-step Google Sheets data analysis guide to get started with AIV.
0 notes
Text
Data Sourcing: The Key to Informed Decision-Making
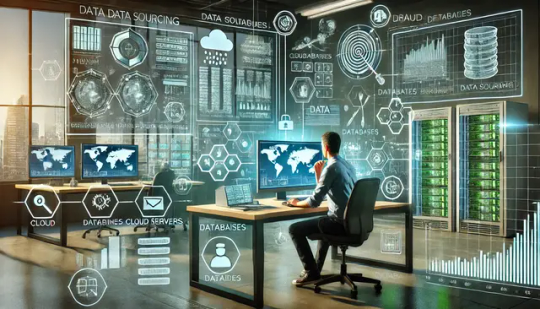
Introduction
Data sourcing in the contemporary business environment, has been known to result in gaining a competitive advantage in business. Data sourcing is a process of gathering data, processing, and managing it coming from different sources so that business people can make the right decisions. A sound data sourcing strategy will yield many benefits, including growth, increased efficiency, and customer engagement.
Data sourcing is the process of finding and assembling data from a variety of sources, including surveys, publicly available records, or third-party data sources. It's important in attaining the right amount of data that will lead strategic business decisions. Proper data sourcing can allow companies to assemble quality datasets that may provide strategic insights into market trends and consumer behavior patterns.
Types of Data Sourcing
Understanding the various forms of data sourcing will allow firms to identify the suitable type to apply for their needs:
Primary data sourcing: In this method, data sources are obtained from the primary and original source. Among the techniques are surveys, interviews, and focus groups. The benefit of using primary data is that it is unique and specifically offers a solution that meets the requirements of the business and may provide insights that are one-of-a-kind.
Secondary data sourcing: Here, data that already exists and has been collected, published, or distributed is utilized; such sources may encompass academic journals, the industry's reports, and the use of public records. Though secondary data may often be less precise, it usually goes easier to access and cheaper.
Automated Data Sourcing: Technology and tools are used when sourcing data. Sourcing can be completed faster with reduced human input errors. Businesses can utilize APIs, feeds, and web scraping to source real-time data.
Importance of Data Sourcing
Data sourcing enhances actual informed decision-making with quality data. Organizations do not assume things will become the case in the future as an assumption; they will use evidence-based decision-making. In addition, risk exposure is minimized and opportunities are exploited.
Cost Efficiencies: Effective data sourcing will always help to save money through the identification of what data is needed and utilized in analysis. This helps in optimizing resource allocation.
Market Insights: With a variety of data sourcing services, a business can gain a better understanding of its audience and thus change marketing campaigns to match that audience, which is always one aspect that will increase customer engagement and, therefore, drive sales.
Competitive Advantage: This ability can differentiate a business as it gains the advantage to access and analyze data faster than its competition in a world of data dominance. Companies that may expend more resources on robust data sourcing capabilities will have better abilities to find trends sooner and adjust accordingly.
Get more info about our data sourcing services & data building services and begin transforming your data into actionable insights Contact us now.
Data Sourcing Services
Data sourcing services could really smooth out your process of collecting data. The data sourcing providers are capable of offering you fresh, accurate, and relevant data that defines a cut above the rest in the market. Benefits of data sourcing outsourcing include:
Professional competencies: Data sourcing providers possess all the skills and tools necessary for gathering data efficiently and in good quality.
Time Saving: Outsourced management allows the organizations to focus on their core business by leaving the data collection to the experts.
Scalability: As the size of the business grows, so do its data needs. Outsourced data sourcing services can change with the evolved needs of the business.
Data Building Services
In addition to these services, data-building services help develop a specialized database for companies. This way, companies can be assured that the analytics and reporting done for them will be of high caliber because quality data comes from different sources when combined. The benefits associated with data-building services include:
Customization: They are ordered according to the needs of your company to ensure the data collected is relevant and useful.
Quality Assurance: Some data building services include quality checks so that any information gathered is the latest and accurate.
Integration: Most data building services are integrated into existing systems, thereby giving a seamless flow of data as well as its availability.
Data Sourcing Challenges
Even though data sourcing is highly vital, the process has challenges below:
Data Privacy: Firms should respect the general regulations regarding the protection of individual's data. For example, informing consumers on how firms collect as well as use their data.
Data Quality: All the data collected is not of good quality. Proper control quality measures should be installed so as not to base decisions on wrong information.
Cost: While benefits occur in outsourcing the data source, it may also incur a cost in finance. Businesses have to weigh their probable edge against the investment cost.
Conclusion
As a matter of fact, no business would function without proper data sourcing because that is what makes it competitive. True strategic growth indeed calls for the involvement of companies in overall data sourcing, which creates operational value and sets an organization up for success long-term. In the data-centric world of today, investing in quality data strategies is unavoidable if you want your business to be ahead of the curve.
Get started with our data sourcing services today and make your data building process lighter as well as a more effective decision-making. Contact us now.
Also Read:
What is Database Building?
Database Refresh: A Must for Data-Driven Success
Integration & Compatibility: Fundamentals in Database Building
Data analysis and insights: Explained
0 notes
Text
Accessing Component Policies in AEM via ResourceType-Based Servlet
Problem Statement: How can I leverage component policies chosen at the template level to manage the dropdown-based selection? Introduction: AEM has integrated component policies as a pivotal element of the editable template feature. This functionality empowers both authors and developers to provide options for configuring the comprehensive behavior of fully-featured components, including…
View On WordPress
#AEM#component behavior#component policies#datasource#datasource servlet#dialog-level listener#dropdown selection#dynamic adjustment#electronic devices#frontend developers#ResourceType-Based Servlet#Servlet#template level#user experience
0 notes
Text
🏆🌟 Unlock your dream team with our cost-effective RPO services! 🚀 Optimize hiring and secure top talent for business success. Join us today! #rpo #TalentAcquisition #BusinessSuccess 💼✨ https://rposervices.com/ #rposervices #recruitment #job #service #process #hr #companies #employee #it #hiring #recruiting #management #USA #india
#career#employment#job#bestrposervicesinindia#resumes#cvsourcing#datasourcing#offshorerecruitingservices#recruitingprocessoutsourcing
0 notes
Photo
New Jersey Municipal Retail Cannabis Laws and Licensed Businesses, May 2023
by u/MapsOfNJ
Hello all, this is a map of New Jersey's Municipal Cannabis Laws and Licensed Businesses as of May 2023.
The New Jersey Legislature passed legislation legalizing cannabis that took effect on February 22st, 2021 and created a framework where all of the state's 554 municipalities determined at a town-level whether to prohibit or allow any of six regulated classes of Cannabis licenses offered in the state. The six classes are Cultivator, Manufacturer, Wholesaler, Distributor, Retailer, and Delivery Service. Only Cultivator, Manufacturer, and Retailer have been licensed so far.
One year in, legal sales began in April 2022 with previously existing medical dispensaries converting to begin Adult-Use Recreational Sales.
By now, the state's municipalities have passed over a collective 1,150 ordinances on Cannabis, creating a patchwork of areas where businesses are allowed or prohibited.
New Jersey's Cannabis Regulatory Commission has licensed 38 Medical-Cannabis Dispensaries, 28 Adult-Use Recreational Dispensaries, and 18 Cultivators & Manufacturer's.
This is an infographic that has been created to chronicle the expansion of this industry. This update sees several new business additions, and a handful of new municipal ordinances.
Datasources: Municipal Ordinance Data: Self-collection
Permitted Businesses: Self-collection, NJ CRC
Software: QGIS, Adobe Illustrator
30 notes
·
View notes
Text
Dell CyberSense Integrated with PowerProtect Cyber Recovery
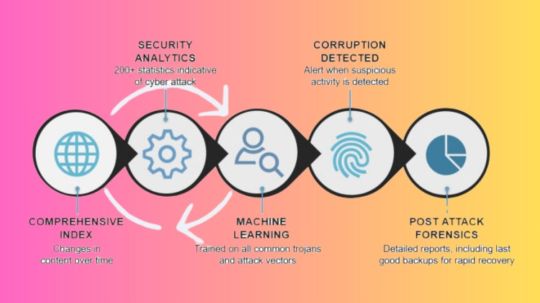
Cybersense compatibility
A smart approach to cyber resilience is represented by Dell CyberSense, which is integrated with the Dell PowerProtect Cyber Recovery platform. In order to continuously verify data integrity and offer thorough insights across the threat lifecycle, it leverages cutting-edge machine learning and AI-powered analysis, drawing on decades of software development experience. This significantly lessens the impact of an attack minimizing data loss, expensive downtime, and lost productivity and enables organisations to quickly recover from serious cyberthreats, like ransomware.
Over 7,000 complex ransomware variations have been used to thoroughly train CyberSense’s AI engine, guaranteeing accuracy over time. Up to 99.99% accuracy in corruption detection is achieved by combining more than 200 full-content-based analytics and machine learning algorithms. A sophisticated and reliable solution for contemporary cyber resilience requirements, Dell CyberSense boasts more than 1,400 commercial deployments and benefits its customers from the combined knowledge and experience acquired from real-world experiences with malware.
By keeping its defence mechanisms current and efficient, this continual learning process improves its capacity to identify and address new threats. In order for you to recover from a cyberattack as soon as possible, Dell CyberSense also uses data forensics to assist you in finding a clean backup copy to restore from.
Dell PowerProtect Cyber Recovery
The financial effects of Dell PowerProtect Cyber Recovery and Dell CyberSense for enterprises were investigated in a Forrester TEI study that Dell commissioned. According to research by Forrester, companies using Dell CyberSense and PowerProtect Cyber Recovery can restore and bring back data into production 75% faster and with 80% less time spent searching for the data.
When it comes to cybersecurity, Dell CyberSense stands out due to its extensive experience and track record, unlike the overhyped claims made by storage vendors and backup firms who have hurriedly rebranded themselves as an all-in-one solution with AI-powered cyber detection and response capabilities. The ability of more recent market entrants, which are frequently speculative and shallow, is in sharp contrast to CyberSense’s maturity and expertise.
Businesses may be sure they are selecting a solution based on decades of rigorous development and practical implementation when they invest in PowerProtect Cyber Recovery with Dell CyberSense, as opposed to marketing gimmicks.
Before Selecting AI Cyber Protection, Consider These Three Questions
Similar to the spike in vendors promoting themselves as Zero Trust firms that Dell saw a year ago, the IT industry has seen a surge in vendors positioning themselves as AI-driven powerhouses in the last twelve months. These vendors appear to market above their capabilities, even though it’s not like they lack AI or Zero Trust capabilities. The implication of these marketing methods is that these solutions come with sophisticated AI-based threat detection and response capabilities that greatly reduce the likelihood of cyberattacks.
But these marketing claims are frequently not supported by the facts. As it stands, the efficacy of artificial intelligence (AI) and generative artificial intelligence (GenAI) malware solutions depends on the quality of the data used for training, the precision with which threats are identified, and the speed with which cyberattacks may be recovered from.
IT decision-makers have to assess closely how providers of storage and data protection have created the intelligence underlying their GenAI inference models and AI analytics solutions. It is imperative to comprehend the training process of these tools and the data sources that have shaped their algorithms. If the wrong training data is used, you might be purchasing a solution that falls short of offering you the complete defence against every kind of cyberthreat that could be present in your surroundings.
Dell covered the three most important inquiries to put to your providers on their AI and GenAI tools in a recent Power Protect podcast episode:
Which methods were used to train your AI tools?
Extensive effort, experience, and fieldwork are needed to develop an AI engine that can detect cyber risks with high accuracy. In order to create reliable models that can recognise all kinds of threats, this procedure takes years to gather, process, and analyse enormous volumes of data. Cybercriminals that employ encryption algorithms that do not modify compression rates, such as the variation of the ransomware known as “XORIST,” these sophisticated threats may have behavioural patterns that are difficult for traditional cyber threat detection systems to detect since they rely on signs like changes in metadata and compression rates. Machine learning systems must therefore be trained to identify complex risks.
Your algorithms are based on which data sources?
Knowing the training process of these tools and the data sources that have influenced their algorithms is essential. AI-powered systems cannot generate the intelligence required for efficient threat identification in the absence of a broad and varied dataset. To stay up with the ever-changing strategies used by highly skilled adversaries, these solutions also need to be updated and modified on a regular basis.
How can a threat be accurately identified and a quick recovery be guaranteed?
Accurate and secure recovery depends on having forensic-level knowledge about the impacted systems. Companies run the danger of reinstalling malware during the recovery process if this level of information is lacking. For instance, two weeks after CDK Global’s customers were rendered unable to access their auto dealership due to a devastating ransomware assault, the company received media attention. They suffered another ransomware attack while they were trying to recover. Unconfirmed, but plausible, is the theory that the ransomware was reintroduced from backup copies because their backup data lacked forensic inspection tools.
Read more on govindhtech.com
#DellCyberSense#Integrated#CyberRecovery#DellPowerProtect#AIengine#AIpowered#AI#ZeroTrust#artificialintelligence#cyberattacks#Machinelearning#ransomware#datasources#aitools#aicyber#technology#technews#news#govindhtech
0 notes
Text
Datasource
(1)Goole Scholar(Search word is Polykatoikia+[ word ],)
word=['ampelokipi''ant1news' 'apartment' 'athens' 'attack' 'beirut'"buildingcentre''city''damage''dead''explosion''flattens' 'https' 'injuredisraeli''lebanon''li''man''missile''moment' 'new' 'nhttps'polykatoikia''russian''storey''thessaloniki' 'today' 'tree' 'video']]
(2)X when search word is Polykatoikia
(3)X when search word is "Athens housing"
0 notes
Text
hi
. how to implement expection handling using Spring boot/rest 2. how to configure/implement Spring cloud config server. 3. How to setup two way SSL using Java/Spring boot 4. difference between Bean factory vs Application context in spring application 5. what happens when u send a request to spring boot ? 6. How does Spring Marshall/unmarshall? 7. How to implement Transactions using Spring boot. 8. How to do load balancing using Spring boot both client side and server side. 9. How to add css/javascript/images to spring boot application UI 10. Any spring /spring boot performance issues you encountered. 11. how to implement spring boot security using OAuth 12. How to setup multiple datasources using spring boot
0 notes
Text
Einst
stark und geeint, heute ein Land, das sich selbst vernachlässigt.
Endlose Morde, Geldmangel, Inflation, Abwanderung von Unternehmen, der
Einsturz der Dresdner Carolabrücke – all das ist kein Zufall, sondern
das Ergebnis jahrelanger Versäumnisse und einer Politik des Verrats am eigenen Volk.
https://cdnjs.cloudflare.com/ajax/libs/emoji-datasource-twitter/15.0.1/img/twitter/64/203c.png
0 notes
Text
Sobre Arquitetura Limpa e inseguranças de uma dev júnior/pleno
[09/09/2024]
Acho que a pior parte de não ter tido a oportunidade de trabalhar com pessoas mais experientes é a sensação de que preciso tentar entregar tudo o mais perfeito possível, mas não sei exatamente *como*. Ou até sei, mas não o suficiente.
E essa sensação piorou significativamente quando entrei no projeto novo em que estou trabalhando. É um código em Flutter muito maduro, um aplicativo com mais de 3 anos de vida e escrito por desenvolvedores experientes.
O problema? Bem. Não existe exatamente um padrão no projeto. Um parte dele usa Arquitetura Limpa em sua melhor forma: arquivos de Repository, Datasource e UseCase bem separados.
Mas a outra parte do app (a maior parte dele) usa apenas uma versão simplificada de Repository e Datasource, sem UseCase.
Agora, toda vez que vou criar uma feature nova, perco umas 2h tentando lidar com a insegurança :P (Obrigada, devs sêniors que não estão mais no projeto e deixaram esse Frankenstein aos meus cuidados!).
Já trabalhei com Arquitetura Limpa antes, mas era muito mais fácil trabalhar com ela quando todo o aplicativo seguia um padrão só. Afinal, eu não precisava pensar muito na utilidade do UseCase antes de começar a escrever o código.
Ao invés disso, no meu trabalho atual eu fico pensando: por que ele escolheu não usar o usecase nessa outra feature? Foi uma escolha? Foi preguiça? Existem momentos em que o UseCase não é necessário? Eu estou escrevendo abstrações demais por nada?
DESCULPA, uma dev júnior que "ganhou" o cargo de pleno está surtando agora.
Esse texto meio diário, então, é uma sessão de estudo com um lembrete para mim sobre alguns conceitos importantes sobre os UseCases dentro da Arquitetura Limpa.
O papel dos UseCases
A Arquitetura Limpa preza pelas responsabilidades bem divididas entre as camadas da aplicação. O papel do UseCase é manter as operações/manipulações de dados separadas da camada de Apresentação.
"Os use cases são responsáveis por executar a lógica de negócios da aplicação, processar dados, realizar ações específicas e coordenar as operações que envolvem os repositórios ou serviços." - Por que precisamos do Use Case?
Esse artigo mitigou um pouco de uma das minhas maiores dores escrevendo UseCases: Tá, mas ele só tá chamando o Repository, por quê tô escrevendo ele? É uma abstração desnecessária?
A resposta é NÃO. Mesmo o UseCase mais simples é útil por causa de 3 fatores:
Separa a lógica de negócio da apresentação - Okay, esse é o objetivo principal do UseCase, mas isso é particularmente útil quando você precisar modificar a lógica relacionada a uma regra de negócio sem impactar negativamente a camada de Apresentação
Facilitar testes - A lógica de negócios isolada também dá mais uma vantagem: facilita na hora da criação de testes. Isso porque agora você não vai depender da implementação do Repository para implementar os testes. Você consegue escrever testes unitários para verificar se a lógica aplicadas às regras de negócio estão certas, criando mocks para simular o comportamento do Repository, por exemplo.
Manutenção de código - Se por acaso você decidir mudar a maneira como os dados são recebidos pela API, você pode fazer isso apenas mudando a implementação do Repository, sem mexer no UseCase.
Esse último tópico abriu os meus olhos para algumas coisas, inclusive. Recentemente o aplicativo passou por uma migração grande de uma parte das chamadas de API. O processo teria sido muito mais simples se tivéssemos um UseCase implementado nessa parte da aplicação!
Usei a Perplexyti.ai para conversar comigo me explicando melhor os conceitos para além das leituras, e foi muito útil! Considero que foi uma sessão de estudo bem produtiva :)
#programação#programming#mobile app developers#diário de dev#software development#clean architecture
1 note
·
View note
Text
Learning Azure Data Factory
This week I finally got a reason to play around in Data Factory and see how data gets transformed from one datasource and placed into another via automated pipelines. I managed to get a brief overview on the important functions of ADF by my senior before he had to run of. I hope I will remember most of it if I have to do more work in ADF.
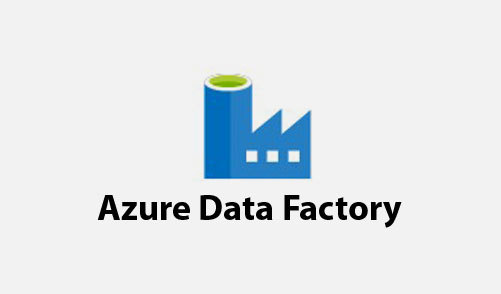
The specific task that was appointed to me was to ensure that certain pipelines use a specific amount of cores that we dictate, instead of ADF choosing a random number. Initially I did it via the Azure portal (analyzing the data in one screen and adapting the partitioning of cores in the other) but at some point my senior mentioned that I had another requirement and had to change certain partition numbers. He then advised me to do that by simply doing a find all and change all in the JSON files in the code solution. This saved a lot on time.
0 notes