#w. 2427
Explore tagged Tumblr posts
Text
1810s dashboard but it's niche drama

💛 heartofanna Following
imagine cancelling someone for saying war is bad
🧵 sharethewoe Follow
#didn't expect better from w*rdsworth but some people i rly thought i could count on…… #anyway we will live to see this empire fall. can't stop history lol (via @heartofanna)
speaking as someone who was press ganged at the age of 17 to serve in his majesty's royal navy i couldn't be more grateful for your poem. young men like me are cannon fodder and you spoke for so many of us. fuck napoleon but fuck parliament even more.
86 notes

chatterpwned-deactivated78345629743
stable forgiving virtuous flourishing in my lane definitely not buying poison moisturized unbothered never been better
chatterpwned-deactivated78345629743
me when i lie
179302 notes

🏛 mynoseisfine Follow
Settling this once and for all. What does the public actually think about the Parthenon marbles debate:
🦉 realminerva Follow
lol i know it’s you lord elgin
🦉 realminerva Follow
like we joke and all but fully aside from the fact that removing the sculptures from greek soil was vulturine and opportunistic etc, it’s really just the tip of a frankly gigantic mountain of imperialist bullshit. let’s not pretend we haven’t been brutally killing hundreds who resisted oppression in india, LITERALLY BOMBED A NEUTRAL EUROPEAN CAPITAL, and embarrassed ourselves in the charge against napoleon for years now. pathetic ass empire & evil as hell to boot. @mynoseisfine the greeks who carved your marbles millennia ago would kick your tory ass so hard
3661 notes

🎀 emmawoodhousestan Follow
how do i still keep seeing thomas chatterton's final post being reblogged, wtf is wrong with you freaks??? he was seventeen it was tragic and horrible and happened ages ago. he was a kid just let him rest
294 notes

🍎 masque-off Following
callout post for @castleyeah @lordsidmouth @officialcoe @parliamentofficial: they oppress, murder and famish the british working people & also suck majorly
⛪ castleyeah Follow
sour cuz you’re unfit to have custody of your own kids huh
🍎 masque-off Following
proud to be the dad of a newborn who could already rend your pudding spine asunder with a mere glance
187 notes

🦆 mallardturner Following
finished this today 😊

44 notes

😎 chadeharold Follow
why is it always “you’re risking your life and legacy & will get yourself killed before the age of five and twenty” and never how was swimming the hellespont the hellespont looked fun was it fun
🎭 loved-joanna Mutuals
ohhh my god you swam the hellespont five years ago?? wooow should we tell everyone?? should we throw a party?? should we invite famous hero of greek myth leander who swam the hellespont
😎 chadeharold Follow
@loved-joanna look we never had any beef & don’t have to start this now. it’s cool that you’re sticking up for my ex, you guys were friends first, but just know that i’ve always trusted your opinion on my work & genuinely respect and admire you & would still be up for a collab whenever.
🎭 loved-joanna Mutuals
yea sure why don’t your lips collab with my ass
😎 chadeharold Follow
on it boss
1009 notes
#literally call me. down if you are

🍂 endymion Follow
sorry is it me or is the assassin who stabbed german bootleg wordsworth kinda…… 🥵
💄 biprincesscharlotte Mutuals
JOHN KEATS????????
2427 notes
#i'm p sure this is the author of lamia thirstposting on main??? help

🌾 huntsmanx Follow
romanticism this romanticism that why don’t you romanticise universal suffrage and rights for labouring people
🌾 huntsmanx Follow
anyone else in jail for seditious libel
🏹 axelaidtotheroot Mutuals
lmao i'm one of the “anyone else”s and i know you’re enjoying family visits and apparently some kind of cushy armchair situation, plus tons of books. try being in here as a spencean dude they won’t even let me learn how to write. worst of all some evangelical came by yesterday just to proselytize & put me “on the right path” fml
8341 notes

🗻 mounttambora Follow
y'all i don't feel so good :/
59 notes
#if you use dark mode...........rip i guess#this was the most fun i've had writing anything in so long. also. if you see the timeline not check out no you don't#(wedderburn was only imprisoned in the 1820s i think & the lamia volume was also published in 1820)#(also up to you whether he's responding to henry or leigh hunt. it's the 1810s everyone's in jail esp if they're a hunt)#romanticism#and just to be sure.#long post
611 notes
·
View notes
Text
wedding organizer akad islami surabaya Call Us: 0812–2427–2825
Pernikahan adalah momen paling istimewa dalam kehidupan seseorang, terutama bagi pasangan Muslim yang ingin melangsungkan pernikahan dalam balutan nilai-nilai Islami. Akad nikah, sebagai inti dari pernikahan, adalah prosesi yang penuh makna dan harus dilakukan dengan tata cara yang benar sesuai syariat Islam. Dalam hal ini, sebuah wedding organizer yang memahami pentingnya tata cara akad Islami dapat menjadi pilihan terbaik.
Rajata Wedding Organizer hadir untuk Anda yang menginginkan pernikahan yang khidmat dan penuh makna dengan mengedepankan nilai-nilai Islam. Kami berfokus untuk menciptakan pengalaman pernikahan yang tidak hanya berkesan, tetapi juga diberkahi. Dengan dukungan tim profesional yang paham akan esensi pernikahan Islami, kami siap membantu Anda mewujudkan pernikahan yang elegan, sakral, dan tentunya sesuai dengan tuntunan agama.
Untuk informasi lebih lanjut, silakan hubungi kami di More Information Call Us: 0812–2427–2825 atau kunjungi situs kami di Visit our Website: www.rajata.co.id. Anda juga dapat datang langsung ke kantor kami di Location: Jl. Gn. Anyar Tambak IV №50, Gn. Anyar Tambak, Kec. Gn. Anyar, Surabaya, Jawa Timur 60294.
Mengapa Memilih Wedding Organizer Akad Islami? 1. Memahami Pentingnya Nilai-Nilai Islami Setiap elemen dalam akad nikah Islami memiliki makna yang mendalam. Rajata Wedding Organizer memahami bahwa pernikahan bukan hanya tentang perayaan, tetapi juga tentang membangun fondasi keluarga yang kuat. Kami menghadirkan layanan yang tidak hanya memperhatikan estetika, tetapi juga mencakup pemahaman mendalam tentang nilai-nilai Islami. Setiap prosesi, mulai dari akad hingga doa-doa yang dipanjatkan, dirancang agar penuh dengan keberkahan.
2. Dekorasi Sederhana namun Elegan Dekorasi dalam akad Islami tidak perlu berlebihan. Dengan konsep minimalis namun elegan, kami menghadirkan suasana yang menenangkan, dengan warna-warna lembut yang menonjolkan kesakralan momen akad. Kami menggunakan elemen alami seperti bunga segar, kain berwarna netral, serta pencahayaan yang hangat. Semua ini dipadukan agar menciptakan atmosfer yang damai dan penuh cinta, sesuai dengan nilai-nilai kesederhanaan dalam Islam.
3. Tata Acara yang Tertib dan Teratur Tata cara dalam akad nikah Islami sangatlah penting, mulai dari prosesi ijab kabul hingga doa-doa yang dibacakan. Dengan pengalaman panjang dalam menyelenggarakan pernikahan Islami, tim kami menyusun acara secara tertib dan teratur, memastikan bahwa setiap tahapan berjalan lancar. Kami memastikan bahwa prosesi ijab kabul dilaksanakan dengan khidmat, dihadiri oleh wali, saksi, dan para tamu undangan dalam suasana yang tenang dan berkesan.
4. Layanan Fotografi dan Videografi Islami Momen akad nikah merupakan bagian yang sangat penting untuk diabadikan. Oleh karena itu, Rajata Wedding Organizer menyediakan layanan dokumentasi dengan pendekatan Islami, yang menjaga etika dan kesopanan. Tim kami adalah fotografer dan videografer berpengalaman yang mampu mengabadikan momen tanpa menghilangkan esensi kesakralan prosesi akad. Setiap foto dan video dibuat dengan cara yang tidak hanya estetik, tetapi juga menghormati privasi serta tata cara Islami.
Layanan yang Ditawarkan oleh Rajata Wedding Organizer untuk Akad Islami Kami menawarkan berbagai layanan lengkap yang dirancang khusus untuk prosesi akad nikah Islami:
1. Konsultasi dan Perencanaan Pernikahan Islami Setiap pasangan memiliki visi yang unik mengenai pernikahan mereka. Oleh karena itu, kami menyediakan sesi konsultasi untuk membantu Anda merencanakan akad nikah sesuai keinginan dan impian Anda. Tim kami akan membantu dalam memberikan saran tentang tema, dekorasi, serta susunan acara yang sejalan dengan syariat Islam. Setiap detail dirancang untuk menciptakan momen yang tidak hanya indah, tetapi juga bermakna dan penuh berkah.
2. Dekorasi Eksklusif yang Islami Dekorasi merupakan elemen yang memperkuat kesan dari suatu acara pernikahan. Kami menggunakan warna-warna lembut seperti putih, krem, atau hijau pastel yang melambangkan ketenangan dan kesucian. Selain itu, kami memilih elemen-elemen dekorasi yang sederhana namun elegan, yang mencerminkan nilai-nilai Islam tanpa perlu berlebihan. Tujuan kami adalah menciptakan suasana yang damai dan khusyuk, agar akad nikah dapat berjalan dalam nuansa yang sakral.
3. Pengaturan Tempat yang Sesuai Pada pernikahan Islami, penting untuk memperhatikan privasi antara tamu laki-laki dan perempuan. Rajata Wedding Organizer sangat memperhatikan detail ini, dengan pengaturan tempat duduk dan alur tamu yang terpisah namun tetap nyaman. Selain itu, kami menyediakan ruangan khusus untuk keluarga dan kerabat terdekat, sehingga mereka dapat menikmati momen istimewa dengan penuh khusyuk.
4. Layanan Dokumentasi dengan Etika Islami Kami paham bahwa akad nikah merupakan momen yang sangat istimewa yang perlu diabadikan dengan baik. Tim dokumentasi kami tidak hanya handal dalam menghasilkan gambar dan video berkualitas, tetapi juga menjaga etika Islami. Dalam proses pengambilan gambar, kami menghindari pose-pose yang tidak sesuai dan menjaga batas-batas kesopanan. Dokumentasi yang kami hasilkan tidak hanya indah secara visual, tetapi juga menghormati nilai-nilai Islam.
Keunggulan Rajata Wedding Organizer dalam Menyelenggarakan Akad Islami Rajata Wedding Organizer menghadirkan berbagai keunggulan yang menjadikan kami sebagai pilihan utama bagi Anda yang ingin melangsungkan akad nikah Islami di Surabaya:
Profesional dan Berpengalaman Dengan pengalaman panjang dalam menyelenggarakan pernikahan Islami, tim kami memiliki kemampuan untuk mengelola setiap detail acara secara profesional. Kami memastikan bahwa semua persiapan dilakukan dengan teliti, sehingga prosesi akad dapat berjalan lancar dan sesuai rencana. Menjaga Nilai-Nilai Syariah dalam Setiap Layanan Kami memahami bahwa pernikahan Islami harus dilaksanakan dengan menjaga syariat Islam dalam setiap aspeknya. Mulai dari susunan acara, dekorasi, hingga dokumentasi, semuanya dirancang agar sesuai dengan tuntunan agama. Pelayanan yang Ramah dan Responsif Kepuasan Anda adalah prioritas kami. Tim kami siap mendengarkan setiap kebutuhan dan permintaan Anda dengan sabar dan profesional. Dengan pelayanan yang ramah, kami berkomitmen untuk memberikan pengalaman terbaik dalam hari bahagia Anda. Pengaturan Acara yang Detail dan Terstruktur Kami menyusun setiap segmen acara dengan sangat terstruktur, mulai dari prosesi akad hingga doa penutup. Ini memastikan bahwa setiap tahap dalam akad Islami berlangsung sesuai jadwal dan menciptakan suasana yang khusyuk dan penuh doa. Katering Halal dan Beragam Makanan adalah bagian penting dalam pernikahan, dan Rajata Wedding Organizer menyediakan katering halal yang memenuhi standar kehalalan dan kualitas. Kami menyediakan berbagai menu, dari masakan tradisional hingga internasional, yang dapat disesuaikan dengan selera tamu undangan. Layanan Dokumentasi Islami Dengan tim fotografer dan videografer yang berpengalaman dalam dokumentasi Islami, kami memastikan bahwa setiap momen diabadikan dengan penuh etika. Kami menjaga batas-batas syariah dalam dokumentasi, sehingga kenangan Anda tetap indah dan sesuai dengan kaidah agama. Hubungi Kami untuk Informasi Lebih Lanjut Rajata Wedding Organizer siap membantu Anda mewujudkan akad nikah Islami yang berkesan dan penuh makna. Untuk informasi lebih lanjut mengenai layanan kami, silakan hubungi kami di nomor More Information Call Us: 0812–2427–2825 atau kunjungi situs web kami di Visit our Website: www.rajata.co.id. Anda juga dapat mengunjungi kantor kami di Location: Jl. Gn. Anyar Tambak IV №50, Gn. Anyar Tambak, Kec. Gn. Anyar, Surabaya, Jawa Timur 60294 untuk berkonsultasi langsung dengan tim profesional kami.
Akad Nikah Islami: Mewujudkan Pernikahan Berkah dengan Rajata Wedding Organizer Menjalani akad nikah Islami bukan hanya tentang mengucapkan ijab kabul, namun juga tentang membangun fondasi rumah tangga yang kokoh dan sesuai dengan tuntunan agama. Dengan bantuan Rajata Wedding Organizer, Anda tidak hanya akan mendapatkan pernikahan yang cantik, tetapi juga penuh dengan keberkahan.
Pernikahan Islami memberikan kebahagiaan yang hakiki, dan momen tersebut akan semakin indah jika diabadikan dengan tata cara yang benar dan layanan yang profesional. Rajata Wedding Organizer berkomitmen untuk menghadirkan pengalaman pernikahan yang tidak hanya berkesan di mata, tetapi juga di hati. Kami senantiasa memastikan bahwa setiap elemen dalam akad nikah Islami berjalan sesuai syariat, sehingga momen sakral Anda menjadi momen yang penuh doa dan kebahagiaan.
Rajata Wedding Organizer memahami pentingnya menjaga kesucian dan makna dari setiap detik yang terlewati dalam akad nikah. Kami ada di sini untuk mendampingi Anda, memastikan bahwa segala sesuatu berjalan lancar dan sempurna dalam bingkai Islami.
Hubungi kami sekarang juga untuk mendapatkan informasi lebih lanjut. Kami dengan senang hati akan membantu Anda menyempurnakan hari istimewa dalam hidup Anda, dan memastikan bahwa akad nikah Islami Anda di Surabaya berlangsung dengan penuh keindahan dan keberkahan
0 notes
Text
19.5: Kizami Backstory [true ending ★2] (pt 2)
Corpse Party Hub, < prev, next >
This is the continuation of true ending ★2 from Book of Shadows Episode #6, Mire. Sorry for breaking it up into two chapters, but between being sick and class and all my other updates, this was just too long to have done for last week.
So here's the rest! Enjoy my favorite part of the backstory!
Pairing: Wilbur!Kizami, Tommy!Ryosuke, Crumb!Urabe, Ranboo!Tohko, Tubbo!Ohkawa
Word Count: 2427
Chapter TWs: Implied/Referenced Character Death, Major Character Death, Blood and Injury, Violence
--
“Man, I've gotta pull myself together…” Ranboo mumbled, sliding down the wall to the floor. Wilbur had long since disappeared up the stairs to go after Crumb, leaving him alone and panicked. “I've gotta focus... What's the most important thing to do right now?”
Suddenly, Ranboo heard Crumb yelling from the top of the stairs, horrified. “No… No! NOOO!!”
“Shoot, Crumb!” Ranboo shot to his feet, concern quickly overtaking his steadily growing panic.
What's going on?! Why's she screaming?!
Straining a little to listen as he jogged towards the bottom of the steps, he finally got close enough to hear Tommy and Crumb having a heated argument at the top of the stairs.
“I'm so sorry, but we can't do that!” Crumb was desperate, panickedly yelling, “We have to run, we'll get caught if we don't!”
“No! We can't just leave him behind! We have to…” Tommy ignored her, his voice breaking a little. “We've gotta get him to a fucking hospital!”
“But he's…” Crumb started to trail off, but Tommy was already yelling to cut her off before she was finished. “No, he's not!”
“Tommy, he's dead!” Crumb exploded, audibly sobbing as Ranboo turned and darted up the first flight of stairs.
He needed to get into the middle of this before it got any worse.
“HE'S NOT!” Tommy was screaming, refusing to budge even a little. “He… can't be…”
‘We have to run'? 'We'll get caught'? Is… Is that ghost girl chasing them right now?! Crap!
“This is bad… this is so bad… gotta… hurry…!” Ranboo panted as he hit the middle landing, taking a moment to catch his breath before starting up the next set only to stop as he heard a disgusting thud, his heart shooting uo his throat as it sounded like someone hit the next landing. “Ahh! What the—?!”
“W—Wilbur…” Crumb whimpered, a tense silence falling until Tommy broke it with a fresh scream.
“MURDERER!!”
There was loud running followed by a sickening crack, Ranboo shuddering at the image of broken bones as he finally made it to the top of the stairs and rounded the corner.
Tommy and Tubbo were on the landing next to each other, Tubbo's body bent awkwardly and bloody as Tommy—with a freshly broken arm—tried to hug his friend’s dead body. Crumb was cowering at the top of the steps, both hands covering her mouth as she stood beside an impassive Wilbur who was watching over the whole scene with dark eyes.
What… What just happened?
“Murderer… You're a fucking murderer!!” Tommy cried, cradling both his ruined arm and Tubbo’s body with a broken sob.
“Crumb! Are you okay—?” Ranboo cut himself off as he stared at everyone one by one, trying to take it all in. “What happened? What's going on?!”
Crumb carefully hurried down the steps to get behind Ranboo, fearfully looking around as Wilbur stared on, still silent.
“Get away from here before he kills you too!” Tommy yelled at Ranboo and Crumb, still crying over Tubbo’s body.
“Crumb, what…?” Ranboo stumbled out, struggling to follow what had happened.
“Wilbur, he… he kicked him down the stairs, and Tommy, he…” Crumb fought back tears, grabbing Ranboo’s arm and starting to pull him towards the steps to get away.
Wilbur… kicked Tubbo down the stairs? Why?!
Upon closer inspection, Wilbur didn't look completely normal, his eyes murky and lips pulled back into a faint scowl.
“Wilbur…? That’s not true, is it?” Ranboo asked, Crumb ducking further behind him as Wilbur didn't answer and took a slow step down the stairs. The two watched as he slowly made his way down to Tubbo and Tommy, sinking to his knees in front of the two and reaching out for Tubbo’s bloody face with a slowly stretching grin that had Ranboo stepping forward to stop him. “Wilbur… Wilbur, what are you—?!”
“Ranboo! Get away from him!” Crumb cried, grabbing Ranboo around the waist from behind as Wilbur curiously lifted one of Tubbo’s arms only to let it drop back to the floor with a barely suppressed giggle.
“What, no!” Ranboo protested as Crumb started pulling him backwards, watching Wilbur’s strange actions before the two turned and ran down the stairs back to the second floor.
Crumb was wild, hurriedly glancing around for anywhere to go as she pushed in doors and guided Ranboo further down the hall and away from Tommy, Wilbur, and what was left of Tubbo. Despite his wariness Ranboo joined Crumb in her hunt, checking rooms for places to hide and running after her.
“Schlatt took the key and lost it, so this room's off limits…” Ranboo panted out, pulling once on the locked infirmary doors as the two stopped in front of it to check.
“Hiding in there wouldn't do us much good, anyway…” Crumb nodded, before yanking Ranboo along to keep running towards the next flight of stairs. “We need to keep going down, to the first floor, or—!”
She's scared out of her mind right now… I mean, I can't really blame her for being freaked out about all this, but…
“Crumb… I'm still not sure we need to run.” Ranboo tried gently as he stopped both of them long enough to catch their breath, Crumb staring at him incredulously.
“What?!” She blinked, waiting for a moment before demanding, “Are you insane?”
Ranboo looked away, embarrassed, lowering his voice to mumble, “I just don't believe that Wilbur… would do something like that.”
“But… he really did kick him down the stairs! It happened!” Crumb shook her head, fresh tears springing to her eyes as Ranboo sighed.
“He kicked Tubbo’s body?” Ranboo asked, trying to keep the disbelief out of his voice. “I just— That really doesn't seem right…”
“F—Fine! You can do whatever you want, Ranboo, but I don't wanna stay here knowing that he's nearby!” Crumb shoved him away, turning and running towards the stairs that led down to the first floor without looking back.
“Wait, Crumb—!”
“I don't know anymore, Ranboo! If you don't want to believe me, then— then I'll just go and hide on my own!” Shaking her head, Crumb quickly disappeared down the steps with one last terrified glance at her friend.
Great. Nice one, idiot. You're completely separated from all of your friends in this awful place.
Am I really in danger, though? Well— I am, there's these stupid crazy ghosts everywhere, but from Wilbur? That really just doesn't seem right.
Or maybe… maybe I just don't want to believe it.
Ranboo only shook out of his swirling thoughts at the sound of footsteps behind him, glancing over his shoulder to see Wilbur directly behind him with both hands clasped around—Is that Schlatt’s knife? Did he…?!
No, no no. It's fine. It's okay. It's totally fine. It's not over yet, and there's no need to run. None at all. There's no need for us to be alone right now, and we really shouldn't be separated in a place like this. It's fine. He'll understand that.
…but what the hell am I supposed to say to him right now? I'm shaking like crazy…
I just… I can't believe he'd do something like that. There's no way. It had to have been a mistake, right? Maybe I can still get through to him, if he did. Maybe he's just freaking out, in his own… super creepy and awful and terrifying way.
He's only human, so he's gotta be just as scared as the rest of us. Maybe… maybe I could help him get through it. Just need to get him to talk about what's on his mind and help him out. Easy, right?
“W—Wilbur… Are you alright?” Ranboo finally managed, turning all the way around to face Wilbur and cursing internally as his voice shook.
“Ranboo, do you know where Crumb and Tommy went?” Wilbur asked, head slowly tipping to one side as he spoke in an unusually monotonous tone.
“Huh? No, I… Why?” Confused and concerned, Ranboo watched as Wilbur muttered something to himself and started to just walk past him without saying anything else. “Wilbur! What are you doing?” Ranboo ran to get in front of Wilbur, stopping him in his tracks with a loud, “Seriously, what's going on? What are you holding?”
“Oh, just something I figured I could put to good use… in a lot of ways.” A small smile broke out across Wilbur’s darkened face, Ranboo suppressing a shiver.
“Uh, that’s ominous. But really, you should get rid of that thing.”
I can't let him leave—!
Grabbing onto Wilbur’s arm, Ranboo rooted himself in place to keep Wilbur from walking away and nearly yanking them both down to the floor.
I can't let him do this… There's no way he's a bad person.
“Ranboo, what are you doing?” Wilbur asked, not making any moves to pull out of Ranboo’s grip. He simply turned his head to the side with a chilling stare.
“Talk to me, Wilbur. What’s going on?” Ranboo tried, doing his best to maintain eye contact in an attempt to convey his sincerity. “What happened with Tubbo and Tommy?”
WIlbur scoffed lightly. “I’m sure Crumb already told you.”
“But I just… you aren’t like that!” Ranboo shook his head, slowly starting to let go of Wilbur as he made no efforts to move—or use the knife he was clutching. “Why would you do something like that, it’s not like you!”
“And how would you know?” The question was simple, but Ranboo froze at his friend’s dull tone. “What if I am?”
“No, you’re… you’re not different than any of the rest of us.” Unable to shake off his fear but unwilling to quit, Ranboo tried again with, “You’re freaked out, too, right? This is just… your way of showing it.”
“This place… has an influence.” Wilbur shrugged, his movements a little too slow to ease Ranboo’s nerves. “I haven’t decided whether or not I mind.”
“You’re not making any sense, Wil. Seriously, just talk to me!” Ranboo stumbled and fell as Wilbur suddenly swung wide and his fist connected with the side of his face, knocking him to the floor as his brain rattled around inside of his skull. “Agh!”
“It's the same in here, you know.” Wilbur supplied unhelpfully, dropping to his knees beside Ranboo only to grab him by the front of his shirt and pull him up enough to punch him hard across the face for the second time. “Getting killed by this school…” He continued, Ranboo’s head spinning from the pain and keeping him from being able to fully focus on his friend’s words, distracted by a third blossom of pain as Wilbur hit him again. “...and getting killed by me…”
WIlbur’s expression was conflicted, Ranboo watching it quickly shift between a muted remorse and a giddy excitement, his breaths from above heavy and ragged as his visible emotions refused to settle. Ranboo was too terrified to move, his head spinning and vision blurry from the pain pounding from seemingly every inch of his skull.
“Let's test this out on you, yeah? You can be the first.” Any remaining hesitance Wilbur had disappeared in an instant, reaching out for Ranboo with Schlatt’s knife in hand and a crazed grin.
“Ahhh… AHHH!” Ranboo just managed to slip away from Wilbur, wobbly legs barely getting underneath him as he turned and ran as fast as he could down the hallway. “He was gonna kill me… He was seriously gonna kill me! What the fuck?!”
He skidded to a stop by the top of the stairs, mouth full of blood that he had to lean over and spit out with a gasping breath. He glanced up in time to see Wilbur charging after him, losing his balance in his haste to straighten back up and toppling over and down a few of the stairs going down to the first floor.
“Shit!” He caught himself fast enough that the stairs didn’t injure him too badly, mostly concerned with the approaching form of Wilbur as he tried to get back up to his feet without falling again. “No no no… God, please no!”
In getting back to his feet one of his hands brushed against something hard on the floor, turning and looking to see a key wedged between the wooden boards of the staircase.
It's…! It's the infirmary key!
Shaking hands tried to grasp the metal, Ranboo taking far longer than he would have liked to pick it up and slip it into the front pocket of his hoodie as he got to his feet and tried to run up the stairs past where Wilbur was descending them. However he wasn’t quite fast enough, Wilbur grabbing one of his legs and knocking him over—his hands coming up just fast enough to catch himself. Wilbur flipped him over and dragged him back down the stairs as Ranboo let out another terrified scream, watching as his friend ran his fingers through the blood collecting on his face and took a long moment to lick it off of his fingers.
Ranboo felt nauseous.
Using the moment of distraction he shook free from Wilbur’s grip and ran back up the stairs, looking back to see that Wilbur was only watching him with what seemed to be curiosity as he bolted down the hall and through the infirmary doors that he could finally get open.
Unfortunately, the infirmary doors didn’t seem to lock from the inside, not giving Ranboo any actual feelings of safety as he made it inside. He stumbled backwards away from the door, frantically looking around for a place to hide before Wilbur inevitably decided to come after him since the doors wouldn’t be of any help. There was a bed that he could reasonably fit under and several corners with cabinets, but as he was looking he felt his eyes catch on a large pair of medical shears sitting on one of the shelves.
Scissors! Thank god, there are scissors in there! He thought, picking them up with a quickly dissipating wave of relief. But what the hell am I gonna do with scissors? It's not like I could actually take him in a fight, anyway…
Leaving the scissors within reach on the desk, Ranboo dropped to a couch behind the partition stretched across the room in hopes of making himself small enough that if Wilbur came by again he wouldn’t find him. Covering his head with his hands and trying to suppress his shaking, there was nothing Ranboo could do except wait and desperately pray for everything to go away.
#corpse party au#corpse party#fanfiction#fanfic#writing#cross posted on ao3#whump#angst#ranboolive#ranboo#ranboo fanfic#wilbur soot#wilbur soot fanfiction#crumb cuptoast#cuptoast fanfic#tubbo#tommyinnit#tommyinnit fanfic
0 notes
Text
TODAY'S SHOPPING TREASURY IN SAGAMINO CITY--July 3rd Lay-Wa 5 Daytime( Rates at 07/16/2023 11AM--UTC+9)
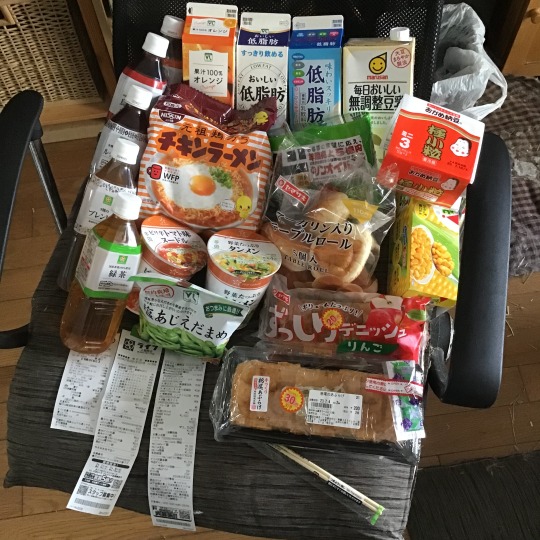
Life Super Market [Call-me-True-Motton]
¥58 $0.42 -- SMILE LIFE Rooibos Tea 500cc pet bottle ¥58 $0.42 -- SMILE LIFE Blended Tea 500cc pet bottle ¥48 $0.35 -- SMILE LIFE Barley Tea 500cc pet bottle ¥48 $0.35 -- SMILE LIFE Green Tea 500cc pet bottle ¥48 $0.35 -- SMILE LIFE Oolong Tea 500cc pet bottle ¥98 $0.71 -- STAR SELECT Vegetable Tanmin vertical cup noodle) ¥98 $0.71 -- STAR SELECT Bit Spicy Tomato Noodles vertical cup noodle ¥458 $3.30 -- Chicken Noodles( 1 package of 5 bags) ¥992 $7.15 -- total ¥73 $0.53 -- sales tax( calculating ¥914 at 8%, ¥5 at 10%)
Super Market LIFE [Kamitsuruma Store] ADDRESS: 1-16-2 Kamitsuruma Sagamihara Minami-ku, Kanagawa 252-0302 JAPAN TEL: 042-747-7720( 9:00a.m-9:00p.m) OPEN: 9:00a.m-3:00a.m, everyday PAYMENT: cash; credit card( VISA / MasterCard/ JCB/ AMEX / Diners; LaCuCa( Life Super Market official electronic money); smartphone payment( Mɛrpay, PayPay, LINE Pay)SHOULD BE CHARGED BEFORE CHECK & CANNOT BE USED TOGETHER WITH CASH, CREDIT CARD, NOR LaCuCa. PARKING LOT: 86 cars, free parking SERVICE: food, drinks, daily use items, homemade bread, drugs, clothes; LIFE café, same-day delivery, utility bill payment; point cards( LaCuCa / LC JCB / Ponta card/ d POINT); recycle box, pure water, ATM, rest space, kids space, multi-purpose toilet, delivery boxes, AEDs
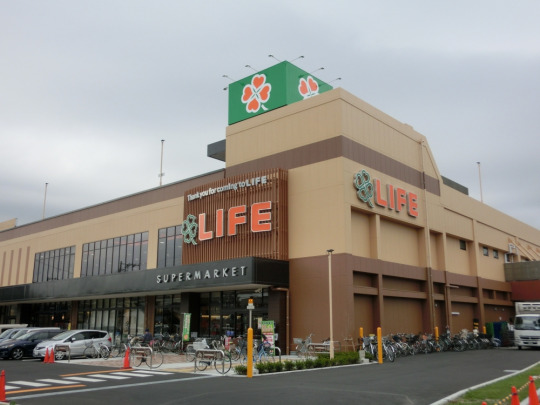
MEGA DONKI [Call-me-True-Mutton]
¥298 $2.15 -- Canned Crisp Corn( 1 pack of 3 tiny cans) ¥148 $X1.07 -- Plain Soy Milk 1qt. carton ¥108 $0.78 -- Low Fat Milk ¥798 $5.75 -- Non-Oil Tuna( 1 pack of 10 hockey puck cans) ¥58 $0.42 -- Okame Natto w/ smallest-size beans( 1 pack of 3 tiny trays) ¥5 $0.04 -- L-size Yellow Plastic Bag -¥2 -$X.XX -- Don Quijote Check Peace Discount*1 ¥1525 $11.00 -- total ¥112 $X.XX -- sales tax( calculating ¥1410 at 8%, ¥5 at 10%)
MEGA Don Quijote [Kamitsuruma Store] ADDRESS: 9-47-30 Kamitsuruma-Honcho Sagamihara Minami-ku, Kanagawa 252-0318 JAPAN TEL: 0570-048-711 OPEN: 9:00a.m-3:00a.m, everyday PAYMENT: cash; credit card; UnionPay; transportation IC card( nimoca, はやかけん, manaca, toica, ICOCA, kitaca, PASMO, Suica, SUGOCA); majica( Don Quijote official electronic money); electronic money( QUICPay+, R Edy, iD) PARKING LOT: 363 cars SERVICE: alcohol, Premier Tax Free, tobacco

Fit Care DEPOT [Sagami Oh-No Store]
¥176 $1.27 -- Big Sauce Yakisoba( 2 Yakisobas, 1 for ¥88) ¥718 $5.18 -- Retorted Cup Rice( 1 pack of 10 cups) ¥384 $2.77 -- PEPSI ZERO( 3 pet bottles, 1 pet bottle ¥128) ¥178 $1.28 -- Tissue Box in vinyl packages ¥108 $0.78 -- DANON Three Berries Yogurt( 4-in-1 pack) ¥78 $0.56 -- 3-Joined Puddings( 3-in-1 pack) ¥248 $1.79 -- Home Run Bar Vanilla & Chocolate Ice Creams( 10-in-1 box) ¥138 $1.00 -- Ah-Gee-Why Milk-based Drink ¥88 $0.63 -- O-Come-A Doe-Who Mo'-Men ¥58 $0.42 -- Ko-Tubu Natto ¥108 $0.78 -- Light-Flavored Curry Cup Noodle ¥15 $0.11 -- LL-size Clear Plastic Bag( 3 bags, 1 bag ¥5) ¥2833 $X.XX -- total ¥213 $X.XX -- sales tax( calculating ¥2427 at 8%, ¥194 at 10%)
Kamegaya Fit Care DEPOT [Sagami-Ono Store] ADDRESS: 14-5 Yutaka-cho Sagamihara Minami-ku, Kanagawa 252-0305 JAPAN TEL: 042-702-2333 OPEN: 9:00a.m-10:30p.m, CLOSED New Years PAYMENT: cash; Kamigaya money, d Point Card; transportation IC card( Suica, PASMO); iD, WAON, QUICPay, R Edy, nanaco, credit card( JCB, Union Pay International, AMERICAN EXPRESS, Diners Club INTERNATIONAL, SAISON CARD, VISA, MasterCard, DISCOVER); R Pay, PayPay, LINE Pay, Alipay, WeChat Pay, d Pay, Mɛrpay, au PAY, Smart Code, Hama Pay, Fami Pay, atone, pring; EPOS Pay, K Plus, etc. PARKING LOT: free parking for purchases SERVICE: drugs, alcohol, tobacco, parcel receival
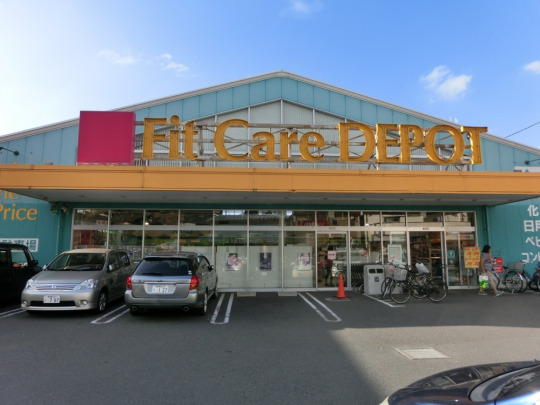
LAWSON STORE 100 [He-Got-She Lincoln Store]
¥192 $1.38 -- 100% Orange Juice 1qt. carton ¥108 $0.78 -- Low Fat Milk 1qt. carton ¥119 $0.86 -- Table Rolls w/ Trapped Margarine, 5 rolls in 1 bag ¥108 $0.78 -- Salted Green Soybeans ¥99 $0.71 -- Zoo-Siri Danish Apple Pie( originally ¥119, with -¥20 discount) ¥130 $0.94 -- Natural Cheese-in Croquette( 2 in 1 pack) ¥186 $1.34 -- Fried Tofu Pouch ¥5 $0.04 -- L-size White Plastic Bag ¥947 $6.83 -- total*2 ¥69 $0.50 -- sales tax( calculating ¥942 at 8%, ¥5 at 10%)
LAWSON STORE 100 [Higashi-Rinkan Store] ADDRESS: 4-12-7 Higashi-Rinkan Sagamihara Minami-ku, Kanagawa 252-0311 JAPAN TEL: 042-701-6699 OPEN: 5:00a.m-2:00a.m PAYMENT: cash; barcode settlement( auPAY, d Pay, PayPay, R Pay, LINE Pay, Mɛrpay, QUO Card Pay, Smart Code, COIN+, J-Coin Pay, NAVER PAY, Alipay + PARTNER); electronic money( Apple Pay, Google Pay, iD, QUICPay, R Edy, WAON); transportation IC card( Suica, Kitaca, TOICA, ICOCA, SUGOCA, PASMO, nimoca, HAYAKAKEN, manaca); credit card( VISA, MasterCard, JCB, AMERICAN EXPRESS, Diners Club INTERNATIONAL, TS Cubic, Rakuten Card, Cedyna, SAISON CARD, MUFG CARD, JACCS, UnionPay International, DISCOVER PARKING LOT: NO parking lot SERVICE: fried ready-made food, alcohol, tobacco, multi-copy
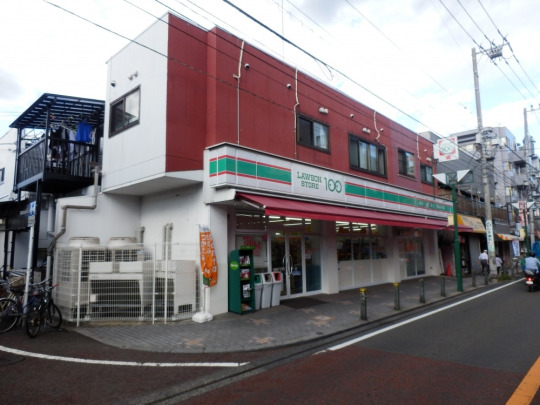
Additional Info( ticket broker)
Access Ticket [Sagami Oh-No Store]( voucher shop, ticket outlet) ¥990 $X.XX -- ODAKYU DEPARTMENT ¥1000 Gift Card ¥480 $X.XX -- Mister Donut/MOS BURGER ¥500 voucher ¥1470 $XX.XX -- total( w/o sales tax, probably because they're vouchers, not like merchandise)
ACCESS TICKET [Sagami-Ono Store] ADDRESS: 3-15-16 Sagami-Ono Sagamihara Minami-ku, Kanagawa 252-0303 JAPAN TEL: 042-701-0433 OPEN: 10:00a.m-7:00p.m( momentarily closed between 3:00p.m-4:00p.m) CLOSED: Wednesdays PAYMENT: cash( few shops allow credit card at ACCESS TICKET)
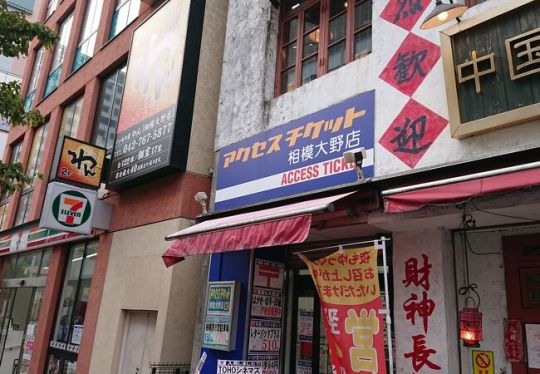
*1 Don Quijote takes care of your 1-4 yen above 0, or above 5( at the last digit of your check) by rounding it down, which is awesome. *2 Lawson 100 is not as cheap as I think it should be--at the present time( ・´ー・`).
#SagamiOno#Sagami-Ono#Sagamihara#Sagamino#City#Kanagawa#Prefecture#Japan#shopping#area#arcade#center#Higashi-Rinkan#Yutaka-Cho#Kami-Tsuruma#shopping treasury
0 notes
Text
Top 10 Must-Visit Indoor Water Parks in the United States: Ultimate Family Fun Destinations

Discover a world of thrilling aquatic adventures at the top 10 indoor water parks in the United States! These ultimate family fun destinations offer year-round excitement, regardless of the weather outside. From adrenaline-pumping slides to relaxing lazy rivers, there's something for everyone in these amazing water wonderlands.
Get ready to make a splash as you explore our carefully curated list of the most popular indoor water parks across the nation, complete with their addresses, phone numbers, websites, and opening hours. Dive into endless fun and make unforgettable memories with your loved ones at these must-visit attractions!
Kalahari Resort - Wisconsin Dells, Wisconsin Address: 1305 Kalahari Dr, Wisconsin Dells, WI 53965 Phone: (877) 525-2427 Website: kalahariresorts.com Opening Hours: Vary by season, check the website for details.
Aquatopia Indoor Waterpark - Tannersville, Pennsylvania Address: 193 Resort Dr, Tannersville, PA 18372 Phone: (570) 629-1665 Website: camelbackresort.com Opening Hours: Vary by season, check the website for details.
Schlitterbahn Waterpark Galveston - Galveston, Texas Address: 2026 Lockheed St, Galveston, TX 77554 Phone: (409) 770-9283 Website: schlitterbahn.com Opening Hours: Vary by season, check the website for details.
Massanutten Resort - McGaheysville, Virginia Address: 1822 Resort Dr, McGaheysville, VA 22840 Phone: (540) 289-9441 Website: massresort.com Opening Hours: Vary by season, check the website for details.
Avalanche Bay Indoor Waterpark - Boyne Falls, Michigan Address: 1 Boyne Mountain Rd, Boyne Falls, MI 49713 Phone: (231) 549-7979 Website: avalanchebay.com Opening Hours: Vary by season, check the website for details.
Splash Lagoon Indoor Water Park Resort - Erie, Pennsylvania Address: 8091 Peach St, Erie, PA 16509 Phone: (814) 217-1111 Website: splashlagoon.com Opening Hours: Vary by season, check the website for details.
Sahara Sam's Oasis - West Berlin, New Jersey Address: 535 NJ-73, West Berlin, NJ 08091 Phone: (856) 767-7580 Website: saharasams.com Opening Hours: Vary by season, check the website for details.
Water Park of America - Bloomington, Minnesota Address: 1700 American Blvd E, Bloomington, MN 55425 Phone: (952) 854-8700 Website: waterparkofamerica.com Opening Hours: Vary by season, check the website for details.
Castaway Bay - Sandusky, Ohio Address: 2001 Cleveland Rd W, Sandusky, OH 44870 Phone: (419) 627-2106 Website: castawaybay.com Opening Hours: Vary by season, check the website for details.
#water parks#Kalahari Resort#Great Wolf Lodge#Aquatopia Indoor Waterpark#Schlitterbahn Waterpark Galveston#KalahariResort#GreatWolfLodge#AquatopiaIndoorWaterpark#SchlitterbahnWaterparkGalveston#MassanuttenResort#AvalancheBayIndoorWaterpark#SplashLagoonIndoorWaterParkResort#SaharaSamsOasis#WaterParkofAmerica#CastawayBay
0 notes
Video
LT trams, MET 130 (LT 2466) & LT 2427 (MET 95) by Frederick McLean Via Flickr: An old photograph taken at Hendon Depot in 1934 of London Transport (LT) trams No. 130 and No. 2427, showing '66 - Canons Park' and '66 - Edgware' destination blinds respectively. It would appear this only being the year after London Passenger Transport Board (LPTB) took over local tramways companies that the renumbering of trams had not finished. The rear tram is showing it's new LT number, but the front tram still shows it's old Metropolitan Electric Tramways (MET) number. The photo reverse is annotated with "type A, Hendon Depot, 1934", and stamped with the photographer and/or negative owner name of D. W. K. Jones. Both were MET 'A' class open top unvestibuled cars built in 1904 by Brush seating 32/38, each ran on a pair of Brush BB maximum traction bogies. They had top covers fitted in 1928/9, one source says they were 'reconstructed' at this time but I cannot find that mentioned elsewhere. Their ownership changed from MET to the LPTB in 1933, No. 130 was renumbered as LT No. 2466 (still showing its old number in this photo), and No. 95 being renumbered as LT No. 2427. No. 2466 was withdrawn Dec 1935 and No. 2427 in Jan 1936, both were disposed of (broken up or sold) at the LT Hampstead Depot. The parts of the London tramways system that had not already been withdrawn or transferred to trolleybus/bus operation closed in Jul 1952. If there are any errors in the above description please let me know. Thanks. 📷 Any photograph I post on Flickr is an original in my possession, nothing is ever copied/downloaded from another location. 📷 -------------------------------------------------
#London Transport#London Passenger Transport Board#London Tram#London Transport Executive#Metropolitan Electric Tramways#D W K Jones#tram depot#Hendon depot#old photograph#old transport#old tramway#old tram#old tramcar#vintage transport#vintage photograph#vintage tram#vintage tramway#vintage tramcar#transport photograph#transport#tramway#tram#tramcar#streetcar#old streetcar#London#LT tram#flickr
1 note
·
View note
Photo

↬ 𝐖𝐎𝐎𝐒𝐔𝐍𝐆. “ 𝒚𝒐𝒖𝒓 𝒎𝒐𝒐𝒏𝒍𝒊𝒕 𝒅𝒓𝒆𝒂𝒎 𝒔𝒕𝒂𝒓𝒕𝒔 𝒉𝒆𝒓𝒆. ”
Welcome to Wanderlust. Please prepare your account and add the admins (Artemis, Apollo & Athena) within the next 48 hours. Additional information will be sent once this step has been completed. Thank you for joining, and we look forward to the start of your journey!
0 notes
Note
will you pls write maddy peeez x male reader angst where maddy is w nate and leading r on and when r confronts her she says things she shouldn't have
thank you❕️❕️❕️
𝐭𝐡𝐢𝐬 𝐢𝐬 𝐰𝐡𝐚𝐭 𝐬𝐚𝐝𝐧𝐞𝐬𝐬 𝐟𝐞𝐞𝐥𝐬 𝐥𝐢𝐤𝐞 || 𝐌𝐚𝐝𝐝𝐲 𝐏𝐞𝐫𝐞𝐳
“𝘚𝘰, 𝘐'𝘮 𝘫𝘶𝘴𝘵 𝘨𝘰𝘯' 𝘱𝘶𝘵 𝘰𝘯 𝘢 𝘴𝘢𝘥 𝘴𝘰𝘯𝘨 𝘈𝘯𝘥 𝘴𝘪𝘵 𝘪𝘯 𝘮𝘺 𝘳𝘰𝘰𝘮 𝘸𝘪𝘵𝘩 𝘵𝘩𝘦 𝘭𝘪𝘨𝘩𝘵𝘴 𝘰𝘧𝘧 𝘈𝘯𝘥 𝘱𝘶𝘵 𝘰𝘯 𝘢 𝘧𝘢𝘤𝘦 𝘵𝘩𝘢𝘵 𝘐 𝘸𝘰𝘯'𝘵 𝘴𝘩𝘰𝘸 𝘢𝘯𝘺𝘰𝘯𝘦“
Inspo: JVKE - this what sadness feels like
Pairing: Maddy Perez x Male!reader
Summary: She was lost a long time ago...

Warnings: Angst
Words: 2427
Love was a complicated subject for Maddy. She’d experienced so many relationships and so many things that felt and were similar to what love would be like, but none of them were real. Well, that was from what you had told her during the times you two hung out. Explaining everything to her and what she had been through and giving her valid points as to why you believed Nate was toxic and not good enough for her. Although she was thankful for you being there for her, her heart said otherwise as to what you were saying.
And it was painfully obvious with how she held onto Nate’s arm as they both conversed with some of his friends. The kitchen overflowing with football players gave Nate their every second to hear the man talk. Almost like he was speaking inspirational words from the creation of alcohol flowing through his system. But he wasn’t and he never would. You had your own personal reasons to hate Nate that everyone already knew. And sadly, you didn’t think Maddy was willing to put herself through this pain again and again and again, but here she was proving you wrong and making you feel worthless in the process.
You had been seated by the pool outside with the perfect line of sight to the kitchen. A deep and confused scowl on your face as you stared at how affectionate Maddy was with Nate. Although you felt things for her, it disgusted you. How could she still love him after everything he’s done? And most importantly, how could she love you after everything the two of you have been through? It just didn’t make sense and your mind couldn’t comprehend whatever reasoning she had for it.
“She’s still with him?” Elliot asked, sitting beside you on one of the lawn chairs in the backyard that wasn’t as crowded as the house. It felt nicer out here even though you could smell the familiar scent of a possible storm brewing. Judging by the clouds beginning to block out the moon, the night was going to get darker the longer you stared at Maddy. “Thought you two had shit going on.”
His words made you clench your jaw, parting your lips for a moment to take a drag off your cigarette. “I thought so too,” you muttered, lips pushing out into a purse. Propping your arms onto your knees where you leaned forward and rested your chin on your arms, staring down at the grass.
Over the past few months, Maddy had relied on you to bring some light to her life. Having had some sort of soft spot for the girl, you willingly gave your time, mind, and energy to her. Giving her your words that could help her mull over whatever she may have been ranting about or stressing over. She always said your company was better than any other guy could provide, and you took that with a warm heart. But currently, you were beginning to see the cracks in her words. The truth masked beneath that you had wanted to ignore and hopefully forget. Except for seeing her here with Nate, you couldn’t ignore it.
A bitter taste was on your tongue, causing your face to scrunch up in disgust. “I need to go to the bathroom.” Elliot could barely get a word out before you were already walking away. Cigarette hanging lazily in the corner of your lips as you entered the house where the once muffled music grew in volume. Parties hadn’t been your thing before you met Maddy. She’d forced you out to every single one she could go to and soon enough, the atmosphere of it became bearable. It wasn’t your ideal place to be, but you mainly went to them to see your friends.
Moving through the crowd urgently with a permanent scowl on your face, finally reaching the stairs where you climbed to the top floor. Searching for an empty bedroom with your frustration growing with each dead end. Until finally, you found a room that you slammed the door shut and moved towards the window. Placing your cigarette on a nearby dresser before unlocking the latch on the window and forcing it up. Leaving just enough room for you to have one leg out and to lean against the frame. A similar position you always did at Maddy’s place.
“Y/n?” You turned your gaze, finding Maddy peeking around the half-closed door, smiling. “What’s up?”
Her casual tone made you stare at her incredulously. Of course, she would act like nothing was wrong. Continue to dance around the fact that you were played and she was always going to go back to Nate. It should’ve been painfully obvious, but you had hoped she would grow up and act mature. But, you were so fucking dumb to even think that.
Seeing the rather angry look on your face, her smile slightly faltered. “What?” She questioned.
“I see you’re with Nate, again,” you scoffed, taking a long drag off your cigarette. Inhaling deeply before chuckling. “What happened to him being a worthless cunt?” You asked, snickering to yourself.
Why the hell was it so funny?
Smudging out your cigarette from the frame of the window, you tossed the filter out. “Am I a joke to you, Maddy?” You inquired painfully quietly. “Because I sure feel like I am.”
“What the fuck do you mean?” Maddy spat, beginning to feel a sense of confusion and annoyance build.
“What I mean is that you have been parading me around town and school like your next boyfriend,” you exclaimed, voice trembling as you waved your hand in hysterics. “Making me actually believe-” You scoffed at the thought. Of course, she wouldn’t consider you as a possible boyfriend or hold the same feelings. Why would she? “God, of course not! Because you care more about a toxic, selfish, piece of shit than someone who’s always cared about you. And you know what? That’s fine!”
Tears had begun to break through the resistance Maddy had performed. Now falling down her cheeks as she glared at you. “You don’t get to make me feel like shit because I want to be happy,” Maddy wept defensively. Your face was flushed, tears brimming in your eyes as you shook your head. “I thought out of everyone, you would be the last person to talk to me about Nate or to try and tell me who I should or shouldn’t be with.”
“Yeah, because everyone is Maddy Perez’s enemy,” you exclaimed with a huff of a laugh. “We got that, loud and clear! Thank you!”
“Fuck you, Y/n! I’ve only wanted to be happy and you won’t leave me be!” Pursing her lips, her face scrunched up in disgust as she looked you up and down, almost like she was finally seeing something that she couldn’t have seen before. “But I can see you are just like every asshole I know. I don’t know why I even gave you the time of day when you are just a fucking dick.”
The words were forceful. She didn’t mean a single thing that came out of her mouth, and the look on your face made her regret it in an instant. Pure shock and genuine pain are found in your glassified gaze. But that pain transformed into hatred as you glared at the girl. “Then let me make this easy for you,” you shook your head, lips quivering as you sniffled. “We’re fucking done.”
Maddy was left in shock as you brushed past her. Her heart hammering against her chest like drums as she shook her head. “Y/n, wait-” The door slammed shut, silencing her plea. Her hands clasping together as she gnawed on her bottom lip, staring at the door hopefully. Maybe you would come back, take her into your arms and tell her the fight was stupid. But the longer you were gone and the more tears that fell down her cheeks, that hope was beginning to run dry.
“Y/n?”
Rain pelted against the windshield as you glared at the open street ahead of you. Your nose twitched as you furiously wiped the tears from your cheeks. Feeling dumb enough that you had believed anything Maddy had said or may have made you believe she could’ve felt. None of it was real. She wasn’t real. Nate wasn’t real. None of what she advertised to you was real. But you knew that the stains of her lipstick stuck to your cheeks slowly were washed away by the tears that slipped past your defences. Slowly ridding yourself of any semblance of Maddy Perez that was left.
Hands clenching the leather steering wheel as you roll your shoulders back. Jaw clenching as felt your arms shake before an uncontrollable, sorrowful, heartbroken yell was ripped from your throat. Body trembling as you slammed your fist into the steering wheel.
The months since that party had passed slowly. You went through school and avoided every hall and classroom that included Maddy Perez. Staying away from her friends and becoming secluded to any interactive experience that occurred inside and outside of school. You stopped going to parties. You stopped going to the diner. You stopped hanging around Rue, Lexi, Kat, or Jules. The only person you knew in your life was Elliot and that was because he had no experience with Maddy and it was best that way.
Summer was now here and it gave you more time to focus on your summer job and staying home. Both are good for your particular situation of absolute deviation from your prior activities. A knock at your door got your attention. Getting up from the couch and wandering over. “Elliot, you better have brought the pizza-” Before you could finish, your lips clamped shut upon the sight of the girl standing on your front doorstep. Her arms tightly crossed over her chest as she stared up at you, a hint of a nervous smile forming on her lips.
“Hey, Y/n.” You stared at her, once surprised now morphing into a deadpan. An unreadable expression that was enough to show Maddy nothing had changed since that night. Something she had hoped would’ve changed, but she was completely mistaken. “Can we talk?”
She didn’t deserve your time, yet, she still hoped you held something for her. Maybe just a sliver of love that still co-existed with the resentment you held towards her. Because she knew it would never disappear, but she knew the love could be evaporated in a split-second. But she was glad when you sighed, opening the door wider for her to enter.
Stepping inside, she took in her surroundings. Although much had changed, it felt emptier. Where picture frames once were, they no longer were hung. Leaving only the outline of where dust had collected. Flowers that she knew your mother had cared for were dead with the delicate flaky petals now resting around the dirty water. Paintings had been taken down and shrouded in thick sheets you had taped around each one.
Walking into the kitchen, Maddy saw the one picture of your family held up by a magnet. Your parents wore scolding looks on their faces as you had both your siblings in chokeholds. Smiles etched on their faces, and one on yours. A very rare sight nowadays that felt practically like an artifact lost to history.
“Get on with what you want to talk about.” Your voice caused the girl to look away from the picture and towards you. Finding you sat at the table, hands clasped together and eyes looking ahead, not sparing her your undivided attention.
Sitting down at the table across from you, she sighed softly. “Nate and I broke up,” she confessed. “I know you probably don’t care, but I just wanted to let you know. You were right. He was toxic and only wanted me for his own gain. That and the fact that he held a gun to my head.” She tried to laugh, hating that you were right about everything you tried to tell her. She wished she listened to you before and kept you in her life. But she hoped that you know this might be enough to bring you back to her. To have your joyful mood elevate the darkness that clouded each day she traversed through.
But the longer the seconds passed and the unaltered expression you wore, her hope was slowly dwindling. “You don’t get it, Maddy,” you breathed, sitting across from her at the table. She could see the distant look in your eyes as you shook your head, chin quivering as you looked at her. “He killed my family in that car crash a year ago. My parents, my siblings- they’re gone because of him!” You cried, staring at the guilt-ridden Maddy Perez.
She remembers that night vividly because she was there when it happened. She remembers the phone call you had received and she remembers driving you to the car accident. Your parents had been on a business trip and had taken your little brother and sister. On their way home, there had been a drunk driver in a white Dodge Ram that was racing down the street. And when your parents had been crossing the intersection, the truck hit the side of the vehicle. From the impact, it killed both your siblings instantly, but your parents had been unconscious when the vehicle went up in a ball of flames.
Nate had been singled out for it by the damages dealt to the front of his truck and the fact that his time lined up with the incident. But with Cal Jacobs having his grasp on everything and everyone, he made it disappear. Leaving you unfulfilled as your family’s death still clung to the back of your mind and the lack of justice put out for your family. And you don’t think you would ever receive the closer needed.
“He killed everyone I cared about,” you muttered. “And it feels like he’s doing it still. Taking everyone I care about away from me without a single repercussion of his actions. And I think…” You shifted in your seat, sucking in a harsh breath as you stared down at the table for a moment. Lips wobbling as Maddy awaited anxiously for you to finish. But when you met her gaze, eyes bloodshot and body trembling, she wished you didn’t.
“I think you died the day you went back to him.”
#maddy perez#maddy x reader#maddy euphoria#maddy perez euphoria#maddy perez x male reader#maddy perez x male!reader#maddy perez x reader#maddy perez x you#maddy perez x y/n#euphoria fanfiction#euphoria#euphoria imagine#euphoria angst#maddy perez imagine#maddy perez fanfiction#x male reader#x male!reader#euphoria x male!reader#euphoria x male reader#maddy perez angst
620 notes
·
View notes
Text
mmmm bebo eating good
(this is with husk buff, with he has 2637 def, w/o he has 2427 def)
#peep peep#but I still haven't gotten a better crit hat for him sobsobsob I just want a crit rate hat w/ crit damage substat and *maybe* def-#preferably flat defense though because iirc it multiplies more in the long run compared to def%#character builds#btw he does have r5 cinnabar though#u know that i was on during his second event nodnodnodnod you KNOW it
5 notes
·
View notes
Text
2427
HAPPY NEW YEAR MUNCHKIN. i been putting off and thinking about doing this for soooooooooo fking long (AS YOU KNOW) that i think this number is gonna be imprinted on my prefrontal cortex for the rest of my life. what’s funny is i LITERALLY thought abt it again right before going to sleep the other day after schitt’s creek, and then i wake up to them messages on ig 🥺
SO.
hi.
i luv u a lot a lot a lot a lottttttttt. which im sure u know. i don’t even KNOW what to say? just. i luv u a lot. and im sooooooo sosososososo happy to have met you and to know you. like, what are the odds the random girl who you happened to share a sociology major with ended up being so much like u? what are the odds that the random girl who sent me a message abt it would be so much like me? like??????? to think if i wasn’t a soci major we probably would’ve never had that first interaction is so funny fgffkjfjfkfjk ???????////??????? losing my fkin mind
okay okay ummm.
i know i tease u a lot abt being a meanie n what not but UR SO FKING NICE. so so nice, i dont even know how you do it. the stuff you tolerate......hnhghjhgkdfhg. not to mention like you guiding me through photoshop, taking the time out of your day to help me find tutorials and then hyping me up n shit, the way you’re so supportive of people, of me, other things u do that i wont mention here (hehe). you’re just so good at making people feel special, making me feel special, and i admire u a lot for it.
(dw u also meanie im not trying to Take Away from your Personality) <3
you’re also so TALENTED, so smart, so hard-working, so SKILLED. a 70 page thesis on colorism??? a bachelors degree done done done in 3 years??? your DANCE??? the gifs you’ve made with like 4 months practice??? your FICS, fic ideas????? ugh. ur so hot can’t believe we’re friends 😌
speaking of which, i know u know but like, ur so hot, so pretty, so gorgeous. your hairrrr, your smileeee, your teef, you send me random vids/pics of you n im just :0 at your face because wtf. how u do dat. n then ur abs,, thank u 10th grade you for working out one summer <3
ALSO UR SO FUNNY. SO SO FUNNY. U MAKE ME LAUGH A LOT and u make me very happy and and and i feel like im forgetting something major but ughghghgnghgnhggh. i hate u. oh, i just remembered. brain link. we should get studied. not gonna talk bout this here because like. confidential information u kno. huhuhuhuhu
i lub u n ur ‘what u dong’s n ‘where u go’s. n ur HAHAAH n ur ehehe. n ur ‘SAME 2 SAME’. n ur ‘don’t be sorry. be better’. thanks for making me smile but also checking in on me and stuff ur just so great. lub u hope u have the BEST FATTEST FUCKING YEAR n life. muah muah muah muah smooch smooch slurp.
grateful for u
gib rowdy kisses, and tell neena bestie to fkin CALL ME.
im obsessed w u 😌✨
yours truly,
mr usa
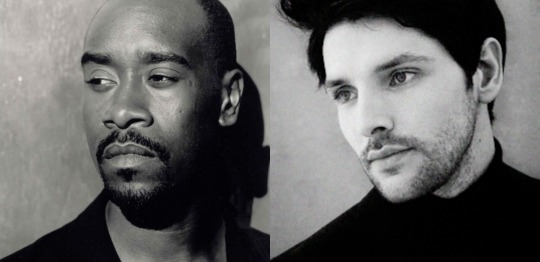
hehehehehehehehehehehe
#this got so LONG if ur not pavi this will annoy u#there's a whole picspam like. u DONT wanna click on it i swear#anyway. LOVE U PAVI. ALL. THE. KISSES. FOR. U#mutual number game#pavi
11 notes
·
View notes
Link
by ethanoliver
Harry stays at Hogwarts for the holidays. So does Draco. Shenanigans ensue, chocolate frogs are shared, and romance begins budding.
Words: 2427, Chapters: 1/1, Language: English
Fandoms: Harry Potter - J. K. Rowling
Rating: General Audiences
Warnings: No Archive Warnings Apply
Categories: M/M
Characters: Harry Potter, Draco Malfoy, Seamus Finnigan, Albus Dumbledore, Minerva McGonagall
Relationships: Draco Malfoy/Harry Potter
Additional Tags: Christmas, Christmas at Hogwarts, Hogwarts Seventh Year, maybe idk close to that probably, Alternate Universe - Canon Divergence, Gryffindor Common Room, Hogwarts Common Room, Platonic Cuddling, Cuddling & Snuggling, sort of starts platonic? who knows, Flirting, Awkward Flirting, Gay Draco Malfoy, bi harry potter, yeah I know it’s after christmas but w/e, this is self indulgent anyway, Hand & Finger Kink, kind of?? don’t judge, it’s my kink not harry’s
4 notes
·
View notes
Text
Making Data Management Decisions
import pandas as pd import numpy as np import os import matplotlib.pyplot as plt import seaborn read data and pickle it all
this function reads data from csv file
def read_data(): data = pd.read_csv('/home/data- sci/Desktop/analysis/course/nesarc_pds.csv',low_memory=False) return data
this function saves the data in a pickle "binary" file so it's faster to
deal with it next time we run the script def pickle_data(data): data.to_pickle('cleaned_data.pickle')
this function reads data from the binary .pickle file
def get_pickle(): return pd.read_pickle('cleaned_data.pickle')
def the_data(): """this function will check and read the data from the pickle file if not fond it will read the csv file then pickle it""" if os.path.isfile('cleaned_data.pickle'): data = get_pickle() else: data = read_data() pickle_data(data) return data
data = the_data()
data.shape
(43093, 3008)
data.head()
ET H R AC E2 A ET O TL C A 2 I D N U M P S U ST R A T U M W EI G HT C D A Y C M O N C Y E A R R E G I O N . . . SO L1 2A BD EP SO LP 12 AB DE P HA L1 2A BD EP HA LP 12 AB DE P M AR 12 AB DE P MA RP 12 AB DE P HE R1 2A BD EP HE RP 12 AB DE P OT HB 12 AB DE P OT HB P12 AB DE P
0 5 1 4 0 0 7 4 0 3 39 28 .6 13 50 5 1 4 8 2 0 0 1 4 . . . 0 0 0 0 0 0 0 0 0 0
1 5 0. 0 0 1 4 2 6 0 4 5 6 0 4 36 38 .6 91 84 5 1 2 1 2 0 0 2 4 . . . 0 0 0 0 0 0 0 0 0 0
2 5 3 1 2 1 2 57 79 2 3 1 1 2 0 3 . . 0 0 0 0 0 0 0 0 0 0
0 4 2 1 8 .0 32 02 5
0 1 .
3 5 4 1 7 0 9 9 1 7 0 4 10 71 .7 54 30 3 9 9 2 0 0 1 2 . . . 0 0 0 0 0 0 0 0 0 0
4 2 5 1 7 0 9 9 1 7 0 4 49 86 .9 52 37 7 1 8 1 0 2 0 0 1 2 . . . 0 0 0 0 0 0 0 0 0 0
5 rows × 3008 columns
data2 = data[['MARITAL','S1Q4A','AGE','S1Q4B','S1Q6A']] data2 = data2.rename(columns={'MARITAL':'marital','S1Q4A':'age_1st_mar', 'AGE':'age','S1Q4B':'how_mar_ended','S1Q6A':'edu'})
selecting the wanted range of values
THE RANGE OF WANTED AGES
data2['age'] = data2[data2['age'] < 30]
THE RANGE OF WANTED AGES OF FISRT MARRIEGE
convert to numeric so we can subset the values < 25
data2['age_1st_mar'] = pd.to_numeric(data2['age_1st_mar'], errors='ignor')
data2 = data2[data2['age_1st_mar'] < 25 ] data2.age_1st_mar.value_counts()
21.0 3473 19.0 2999 18.0 2944 20.0 2889 22.0 2652 23.0 2427 24.0 2071 17.0 1249 16.0 758 15.0 304 14.0 150 Name: age_1st_mar, dtype: int64
for simplisity will remap the variable edu to have just 4 levels below high school education == 0 high school == 1 collage == 2 higher == 3 edu_remap ={1:0,2:0,3:0,4:0,5:0,6:0,7:0,8:1,9:1,10:1,11:1,12:2,13:2,14:3} data2['edu'] = data2['edu'].map(edu_remap) print the frquancy of the values
def distribution(var_data): """this function will print out the frequency distribution for every variable in the data-frame """
var_data = pd.to_numeric(var_data, errors='ignore')
print("the count of the values in {}".format(var_data.name)) print(var_data.value_counts()) print("the % of every value in the {} variable ".format(var_data.name)) print(var_data.value_counts(normalize=True)) print("-----------------------------------")
def print_dist():
this function loops though the variables and print them out
for i in data2.columns: print(distribution(data2[i]))
print_dist() the count of the values in marital 1 13611 4 3793 3 3183 5 977 2 352 Name: marital, dtype: int64 the % of every value in the marital variable 1 0.621053 4 0.173070 3 0.145236 5 0.044579 2 0.016061
Name: marital, dtype: float64
None the count of the values in age_1st_mar 21.0 3473 19.0 2999 18.0 2944 20.0 2889
22.0 2652 23.0 2427 24.0 2071 17.0 1249 16.0 758 15.0 304 14.0 150 Name: age_1st_mar, dtype: int64 the % of every value in the age_1st_mar variable 21.0 0.158469 19.0 0.136841 18.0 0.134331 20.0 0.131822 22.0 0.121007 23.0 0.110741 24.0 0.094497 17.0 0.056990 16.0 0.034587 15.0 0.013871 14.0 0.006844
Name: age_1st_mar, dtype: float64
None the count of the values in age 1.0 1957 4.0 207 5.0 153 2.0 40 3.0 7 Name: age, dtype: int64 the % of every value in the age variable 1.0 0.827834 4.0 0.087563 5.0 0.064721 2.0 0.016920 3.0 0.002961
Name: age, dtype: float64
None the count of the values in how_mar_ended 10459 2 8361 1 2933 3 154 9 9 Name: how_mar_ended, dtype: int64 the % of every value in the how_mar_ended variable 0.477231 2 0.381502 1 0.133829 3 0.007027 9 0.000411 Name: how_mar_ended, dtype: float64
None the count of the values in edu 1 13491 0 4527 2 2688 3 1210 Name: edu, dtype: int64 the % of every value in the edu variable 1 0.615578 0 0.206561 2 0.122650 3 0.055211
Name: edu, dtype: float64
None summery
In [1]:
##### marital status
Married 0.48 % |
Living with someone 0.22 % |
Widowed 0.12 % |
Divorced 0.1 % |
Separated 0.03 % |
Never Married 0.03 % |
|
-------------------------------------|
-------------------------------------|
|
##### AGE AT FIRST MARRIAGE FOR THOSE
WHO MARRY UNDER THE AGE OF 25 |
AGE % |
21 0.15 % |
19 0.13 % |
18 0.13 % |
20 0.13 % |
22 0.12 % |
23 0.11 % |
24 0.09 % |
17 0.05 % |
16 0.03 % |
15 0.01 % |
14 0.00 % |
|
-------------------------------------|
-------------------------------------|
|
##### HOW FIRST MARRIAGE ENDED
Widowed 0.65 % |
Divorced 0.25 % |
Other 0.09 % |
Unknown 0.004% |
Na 0.002% |
|
-------------------------------------|
-------------------------------------|
|
##### education
high school 0.58 % |
lower than high school 0.18 % |
collage 0.15 % |
ms and higher 0.07 % |
|
1- recoding unknown values from the variable "how_mar_ended" HOW FIRST MARRIAGE ENDED will code the 9 value from Unknown to NaN
data2['how_mar_ended'] = data2['how_mar_ended'].replace(9, np.nan) data2['age_1st_mar'] = data2['age_1st_mar'].replace(99, np.nan)
data2['how_mar_ended'].value_counts(sort=False, dropna=False)
1 4025 9 98 3 201 2 10803 27966 Name: how_mar_ended, dtype: int64
0 notes
Text
Making Data Management Decisions
import pandas as pd import numpy as np import os import matplotlib.pyplot as plt import seaborn read data and pickle it all
In [2]:
#this function reads data from csv file def read_data(): data = pd.read_csv('/home/data- sci/Desktop/analysis/course/nesarc_pds.csv',low_memory=False) return data
In [3]: #this function saves the data in a pickle "binary" file so it's faster to deal with it next time we run the script def pickle_data(data): data.to_pickle('cleaned_data.pickle') #this function reads data from the binary .pickle file
def get_pickle(): return pd.read_pickle('cleaned_data.pickle')
In [4]:
def the_data(): """this function will check and read the data from the pickle file if not fond it will read the csv file then pickle it""" if os.path.isfile('cleaned_data.pickle'): data = get_pickle() else: data = read_data() pickle_data(data) return data
In [20]:
data = the_data()
In [21]:
data.shape
Out[21]:
(43093, 3008)
In [22]:
data.head()
Out[22]:
ET H R AC E2 A ET O TL C A 2 I D N U M P S U ST R A T U M W EI G HT C D A Y C M O N C Y E A R R E G I O N . . . SO L1 2A BD EP SO LP 12 AB DE P HA L1 2A BD EP HA LP 12 AB DE P M AR 12 AB DE P MA RP 12 AB DE P HE R1 2A BD EP HE RP 12 AB DE P OT HB 12 AB DE P OT HB P12 AB DE P
0 5 1 4 0 0 7 4 0 3 39 28 .6 13 50 5 1 4 8 2 0 0 1 4 . . . 0 0 0 0 0 0 0 0 0 0
1 5 0. 0 0 1 4 2 6 0 4 5 6 0 4 36 38 .6 91 84 5 1 2 1 2 0 0 2 4 . . . 0 0 0 0 0 0 0 0 0 0
2 5 3 1 2 1 2 57 79 2 3 1 1 2 0 3 . . 0 0 0 0 0 0 0 0 0 0
0 4 2 1 8 .0 32 02 5
0 1 .
3 5 4 1 7 0 9 9 1 7 0 4 10 71 .7 54 30 3 9 9 2 0 0 1 2 . . . 0 0 0 0 0 0 0 0 0 0
4 2 5 1 7 0 9 9 1 7 0 4 49 86 .9 52 37 7 1 8 1 0 2 0 0 1 2 . . . 0 0 0 0 0 0 0 0 0 0
5 rows × 3008 columns
In [102]:
data2 = data[['MARITAL','S1Q4A','AGE','S1Q4B','S1Q6A']] data2 = data2.rename(columns={'MARITAL':'marital','S1Q4A':'age_1st_mar', 'AGE':'age','S1Q4B':'how_mar_ended','S1Q6A':'edu'}) In [103]:
#selecting the wanted range of values #THE RANGE OF WANTED AGES data2['age'] = data2[data2['age'] < 30] #THE RANGE OF WANTED AGES OF FISRT MARRIEGE #convert to numeric so we can subset the values < 25 data2['age_1st_mar'] = pd.to_numeric(data2['age_1st_mar'], errors='ignor') In [105]:
data2 = data2[data2['age_1st_mar'] < 25 ] data2.age_1st_mar.value_counts()
Out[105]:
21.0 3473 19.0 2999 18.0 2944 20.0 2889 22.0 2652 23.0 2427 24.0 2071 17.0 1249 16.0 758 15.0 304 14.0 150 Name: age_1st_mar, dtype: int64
for simplisity will remap the variable edu to have just 4 levels below high school education == 0 high school == 1 collage == 2 higher == 3
In [106]: edu_remap ={1:0,2:0,3:0,4:0,5:0,6:0,7:0,8:1,9:1,10:1,11:1,12:2,13:2,14:3} data2['edu'] = data2['edu'].map(edu_remap) print the frquancy of the values
In [107]:
def distribution(var_data): """this function will print out the frequency distribution for every variable in the data-frame """ #var_data = pd.to_numeric(var_data, errors='ignore') print("the count of the values in {}".format(var_data.name)) print(var_data.value_counts()) print("the % of every value in the {} variable ".format(var_data.name)) print(var_data.value_counts(normalize=True)) print("-----------------------------------")
def print_dist(): # this function loops though the variables and print them out for i in data2.columns: print(distribution(data2[i]))
print_dist() the count of the values in marital 1 13611 4 3793 3 3183 5 977 2 352 Name: marital, dtype: int64 the % of every value in the marital variable 1 0.621053 4 0.173070 3 0.145236 5 0.044579 2 0.016061 Name: marital, dtype: float64 ----------------------------------- None the count of the values in age_1st_mar 21.0 3473 19.0 2999 18.0 2944 20.0 2889
22.0 2652 23.0 2427 24.0 2071 17.0 1249 16.0 758 15.0 304 14.0 150 Name: age_1st_mar, dtype: int64 the % of every value in the age_1st_mar variable 21.0 0.158469 19.0 0.136841 18.0 0.134331 20.0 0.131822 22.0 0.121007 23.0 0.110741 24.0 0.094497 17.0 0.056990 16.0 0.034587 15.0 0.013871 14.0 0.006844 Name: age_1st_mar, dtype: float64 ----------------------------------- None the count of the values in age 1.0 1957 4.0 207 5.0 153 2.0 40 3.0 7 Name: age, dtype: int64 the % of every value in the age variable 1.0 0.827834 4.0 0.087563 5.0 0.064721 2.0 0.016920 3.0 0.002961 Name: age, dtype: float64 ----------------------------------- None the count of the values in how_mar_ended 10459 2 8361 1 2933 3 154 9 9 Name: how_mar_ended, dtype: int64 the % of every value in the how_mar_ended variable 0.477231 2 0.381502 1 0.133829 3 0.007027 9 0.000411 Name: how_mar_ended, dtype: float64
----------------------------------- None the count of the values in edu 1 13491 0 4527 2 2688 3 1210 Name: edu, dtype: int64 the % of every value in the edu variable 1 0.615578 0 0.206561 2 0.122650 3 0.055211 Name: edu, dtype: float64 ----------------------------------- None summery
In [1]:
# ##### marital status # Married 0.48 % | # Living with someone 0.22 % | # Widowed 0.12 % | # Divorced 0.1 % | # Separated 0.03 % | # Never Married 0.03 % | # | # -------------------------------------| # -------------------------------------| # | # ##### AGE AT FIRST MARRIAGE FOR THOSE # WHO MARRY UNDER THE AGE OF 25 | # AGE % | # 21 0.15 % | # 19 0.13 % | # 18 0.13 % | # 20 0.13 % | # 22 0.12 % | # 23 0.11 % | # 24 0.09 % | # 17 0.05 % | # 16 0.03 % | # 15 0.01 % | # 14 0.00 % | # | # -------------------------------------| # -------------------------------------| # | # ##### HOW FIRST MARRIAGE ENDED # Widowed 0.65 % | # Divorced 0.25 % | # Other 0.09 % | # Unknown 0.004% |
# Na 0.002% | # | # -------------------------------------| # -------------------------------------| # | # ##### education # high school 0.58 % | # lower than high school 0.18 % | # collage 0.15 % | # ms and higher 0.07 % | # | 1- recoding unknown values from the variable "how_mar_ended" HOW FIRST MARRIAGE ENDED will code the 9 value from Unknown to NaN
In [13]:
data2['how_mar_ended'] = data2['how_mar_ended'].replace(9, np.nan) data2['age_1st_mar'] = data2['age_1st_mar'].replace(99, np.nan)
In [14]:
data2['how_mar_ended'].value_counts(sort=False, dropna=False)
Out[14]:
1 4025 9 98 3 201 2 10803 27966 Name: how_mar_ended, dtype: int64
In [23]:
#pickle the data tp binary .pickle file pickle_data(data2) Week 4 { "cells": [], "metadata": {}, "nbformat": 4, "nbformat_minor": 0 }
More from @chidujs
chidujsFollow
Making Data Management Decisions
import pandas as pd import numpy as np import os import matplotlib.pyplot as plt import seaborn read data and pickle it all
In [2]:
#this function reads data from csv file def read_data(): data = pd.read_csv('/home/data- sci/Desktop/analysis/course/nesarc_pds.csv',low_memory=False) return data
In [3]: #this function saves the data in a pickle "binary" file so it's faster to deal with it next time we run the script def pickle_data(data): data.to_pickle('cleaned_data.pickle') #this function reads data from the binary .pickle file
def get_pickle(): return pd.read_pickle('cleaned_data.pickle')
In [4]:
def the_data(): """this function will check and read the data from the pickle file if not fond it will read the csv file then pickle it""" if os.path.isfile('cleaned_data.pickle'): data = get_pickle() else: data = read_data() pickle_data(data) return data
In [20]:
data = the_data()
In [21]:
data.shape
Out[21]:
(43093, 3008)
In [22]:
data.head()
Out[22]:
ET H R AC E2 A ET O TL C A 2 I D N U M P S U ST R A T U M W EI G HT C D A Y C M O N C Y E A R R E G I O N . . . SO L1 2A BD EP SO LP 12 AB DE P HA L1 2A BD EP HA LP 12 AB DE P M AR 12 AB DE P MA RP 12 AB DE P HE R1 2A BD EP HE RP 12 AB DE P OT HB 12 AB DE P OT HB P12 AB DE P
0 5 1 4 0 0 7 4 0 3 39 28 .6 13 50 5 1 4 8 2 0 0 1 4 . . . 0 0 0 0 0 0 0 0 0 0
1 5 0. 0 0 1 4 2 6 0 4 5 6 0 4 36 38 .6 91 84 5 1 2 1 2 0 0 2 4 . . . 0 0 0 0 0 0 0 0 0 0
2 5 3 1 2 1 2 57 79 2 3 1 1 2 0 3 . . 0 0 0 0 0 0 0 0 0 0
0 4 2 1 8 .0 32 02 5
0 1 .
3 5 4 1 7 0 9 9 1 7 0 4 10 71 .7 54 30 3 9 9 2 0 0 1 2 . . . 0 0 0 0 0 0 0 0 0 0
4 2 5 1 7 0 9 9 1 7 0 4 49 86 .9 52 37 7 1 8 1 0 2 0 0 1 2 . . . 0 0 0 0 0 0 0 0 0 0
5 rows × 3008 columns
In [102]:
data2 = data[['MARITAL','S1Q4A','AGE','S1Q4B','S1Q6A']] data2 = data2.rename(columns={'MARITAL':'marital','S1Q4A':'age_1st_mar', 'AGE':'age','S1Q4B':'how_mar_ended','S1Q6A':'edu'}) In [103]:
#selecting the wanted range of values #THE RANGE OF WANTED AGES data2['age'] = data2[data2['age'] < 30] #THE RANGE OF WANTED AGES OF FISRT MARRIEGE #convert to numeric so we can subset the values < 25 data2['age_1st_mar'] = pd.to_numeric(data2['age_1st_mar'], errors='ignor') In [105]:
data2 = data2[data2['age_1st_mar'] < 25 ] data2.age_1st_mar.value_counts()
Out[105]:
21.0 3473 19.0 2999 18.0 2944 20.0 2889 22.0 2652 23.0 2427 24.0 2071 17.0 1249 16.0 758 15.0 304 14.0 150 Name: age_1st_mar, dtype: int64
for simplisity will remap the variable edu to have just 4 levels below high school education == 0 high school == 1 collage == 2 higher == 3
In [106]: edu_remap ={1:0,2:0,3:0,4:0,5:0,6:0,7:0,8:1,9:1,10:1,11:1,12:2,13:2,14:3} data2['edu'] = data2['edu'].map(edu_remap) print the frquancy of the values
In [107]:
def distribution(var_data): """this function will print out the frequency distribution for every variable in the data-frame """ #var_data = pd.to_numeric(var_data, errors='ignore') print("the count of the values in {}".format(var_data.name)) print(var_data.value_counts()) print("the % of every value in the {} variable ".format(var_data.name)) print(var_data.value_counts(normalize=True)) print("-----------------------------------")
def print_dist(): # this function loops though the variables and print them out for i in data2.columns: print(distribution(data2[i]))
print_dist() the count of the values in marital 1 13611 4 3793 3 3183 5 977 2 352 Name: marital, dtype: int64 the % of every value in the marital variable 1 0.621053 4 0.173070 3 0.145236 5 0.044579 2 0.016061 Name: marital, dtype: float64 ----------------------------------- None the count of the values in age_1st_mar 21.0 3473 19.0 2999 18.0 2944 20.0 2889
22.0 2652 23.0 2427 24.0 2071 17.0 1249 16.0 758 15.0 304 14.0 150 Name: age_1st_mar, dtype: int64 the % of every value in the age_1st_mar variable 21.0 0.158469 19.0 0.136841 18.0 0.134331 20.0 0.131822 22.0 0.121007 23.0 0.110741 24.0 0.094497 17.0 0.056990 16.0 0.034587 15.0 0.013871 14.0 0.006844 Name: age_1st_mar, dtype: float64 ----------------------------------- None the count of the values in age 1.0 1957 4.0 207 5.0 153 2.0 40 3.0 7 Name: age, dtype: int64 the % of every value in the age variable 1.0 0.827834 4.0 0.087563 5.0 0.064721 2.0 0.016920 3.0 0.002961 Name: age, dtype: float64 ----------------------------------- None the count of the values in how_mar_ended 10459 2 8361 1 2933 3 154 9 9 Name: how_mar_ended, dtype: int64 the % of every value in the how_mar_ended variable 0.477231 2 0.381502 1 0.133829 3 0.007027 9 0.000411 Name: how_mar_ended, dtype: float64
----------------------------------- None the count of the values in edu 1 13491 0 4527 2 2688 3 1210 Name: edu, dtype: int64 the % of every value in the edu variable 1 0.615578 0 0.206561 2 0.122650 3 0.055211 Name: edu, dtype: float64 ----------------------------------- None summery
In [1]:
# ##### marital status # Married 0.48 % | # Living with someone 0.22 % | # Widowed 0.12 % | # Divorced 0.1 % | # Separated 0.03 % | # Never Married 0.03 % | # | # -------------------------------------| # -------------------------------------| # | # ##### AGE AT FIRST MARRIAGE FOR THOSE # WHO MARRY UNDER THE AGE OF 25 | # AGE % | # 21 0.15 % | # 19 0.13 % | # 18 0.13 % | # 20 0.13 % | # 22 0.12 % | # 23 0.11 % | # 24 0.09 % | # 17 0.05 % | # 16 0.03 % | # 15 0.01 % | # 14 0.00 % | # | # -------------------------------------| # -------------------------------------| # | # ##### HOW FIRST MARRIAGE ENDED # Widowed 0.65 % | # Divorced 0.25 % | # Other 0.09 % | # Unknown 0.004% |
# Na 0.002% | # | # -------------------------------------| # -------------------------------------| # | # ##### education # high school 0.58 % | # lower than high school 0.18 % | # collage 0.15 % | # ms and higher 0.07 % | # | 1- recoding unknown values from the variable "how_mar_ended" HOW FIRST MARRIAGE ENDED will code the 9 value from Unknown to NaN
In [13]:
data2['how_mar_ended'] = data2['how_mar_ended'].replace(9, np.nan) data2['age_1st_mar'] = data2['age_1st_mar'].replace(99, np.nan)
In [14]:
data2['how_mar_ended'].value_counts(sort=False, dropna=False)
Out[14]:
1 4025 9 98 3 201 2 10803 27966 Name: how_mar_ended, dtype: int64
In [23]:
#pickle the data tp binary .pickle file pickle_data(data2) Week 4 { "cells": [], "metadata": {}, "nbformat": 4, "nbformat_minor": 0 }
chidujsFollow
Assignment 2
PYTHON PROGRAM:
import pandas as pd import numpy as np
data = pd.read_csv('gapminder.csv',low_memory=False)
data.columns = map(str.lower, data.columns) pd.set_option('display.float_format', lambda x:'%f'%x)
data['suicideper100th'] = data['suicideper100th'].convert_objects(convert_numeric=True) data['breastcancerper100th'] = data['breastcancerper100th'].convert_objects(convert_numeric=True) data['hivrate'] = data['hivrate'].convert_objects(convert_numeric=True) data['employrate'] = data['employrate'].convert_objects(convert_numeric=True)
print("Statistics for a Suicide Rate") print(data['suicideper100th'].describe())
sub = data[(data['suicideper100th']>12)]
sub_copy = sub.copy()
bc = sub_copy['breastcancerper100th'].value_counts(sort=False,bins=10)
pbc = sub_copy['breastcancerper100th'].value_counts(sort=False,bins=10,normalize=True)*100
bc1=[] # Cumulative Frequency pbc1=[] # Cumulative Percentage cf=0 cp=0 for freq in bc: cf=cf+freq bc1.append(cf) pf=cf*100/len(sub_copy) pbc1.append(pf)
print('Number of Breast Cancer Cases with a High Suicide Rate') fmt1 = '%s %7s %9s %12s %12s' fmt2 = '%5.2f %10.d %10.2f %10.d %12.2f' print(fmt1 % ('# of Cases','Freq.','Percent','Cum. Freq.','Cum. Percent')) for i, (key, var1, var2, var3, var4) in enumerate(zip(bc.keys(),bc,pbc,bc1,pbc1)): print(fmt2 % (key, var1, var2, var3, var4)) fmt3 = '%5s %10s %10s %10s %12s' print(fmt3 % ('NA', '2', '3.77', '53', '100.00'))
hc = sub_copy['hivrate'].value_counts(sort=False,bins=7) phc = sub_copy['hivrate'].value_counts(sort=False,bins=7,normalize=True)100 hc1=[] # Cumulative Frequency phc1=[] # Cumulative Percentage cf=0 cp=0 for freq in bc: cf=cf+freq hc1.append(cf) pf=cf100/len(sub_copy) phc1.append(pf)
print('HIV Rate with a High Suicide Rate') fmt1 = '%5s %12s %9s %12s %12s' fmt2 = '%5.2f %10.d %10.2f %10.d %12.2f' print(fmt1 % ('Rate','Freq.','Percent','Cum. Freq.','Cum. Percent')) for i, (key, var1, var2, var3, var4) in enumerate(zip(hc.keys(),hc,phc,hc1,phc1)): print(fmt2 % (key, var1, var2, var3, var4)) fmt3 = '%5s %10s %10s %10s %12s' print(fmt3 % ('NA', '2', '3.77', '53', '100.00'))
ec = sub_copy['employrate'].value_counts(sort=False,bins=10)
pec = sub_copy['employrate'].value_counts(sort=False,bins=10,normalize=True)100 ec1=[] # Cumulative Frequency pec1=[] # Cumulative Percentage cf=0 cp=0 for freq in bc: cf=cf+freq ec1.append(cf) pf=cf100/len(sub_copy) pec1.append(pf)
print('Employment Rate with a High Suicide Rate') fmt1 = '%5s %12s %9s %12s %12s' fmt2 = '%5.2f %10.d %10.2f %10.d %12.2f' print(fmt1 % ('Rate','Freq.','Percent','Cum. Freq.','Cum. Percent')) for i, (key, var1, var2, var3, var4) in enumerate(zip(ec.keys(),ec,pec,ec1,pec1)): print(fmt2 % (key, var1, var2, var3, var4)) fmt3 = '%5s %10s %10s %10s %12s' print(fmt3 % ('NA', '2', '3.77', '53', '100.00'))
------------------------------------------------------------------------------
OUTPUT:
Output with Frequency Tables at High Suicide Rate for Breast Cancer Rate, HIV Rate and Employment Rate Variables
Statistics for a Suicide Rate
count 191.000000
mean 9.640839
std 6.300178
min 0.201449
25% 4.988449
50% 8.262893
75% 12.328551
max 35.752872
Number of Breast Cancer Cases with a High Suicide Rate
# of Cases Freq. Percent Cum. Freq. Cum. Percent
6.51 6 11.32 6 11.32
15.14 14 26.42 20 37.74
23.68 5 9.43 25 47.17
32.22 7 13.21 32 60.38
40.76 2 3.77 34 64.15
49.30 4 7.55 38 71.70
57.84 5 9.43 43 81.13
66.38 1 1.89 44 83.02
74.92 3 5.66 47 88.68
83.46 4 7.55 51 96.23
NA 2 3.77 53 100.00
HIV Rate with a High Suicide Rate
Rate Freq. Percent Cum. Freq. Cum. Percent
0.03 39 73.58 6 11.32
2.64 4 7.55 20 37.74
5.23 2 3.77 25 47.17
7.81 0 0.00 32 60.38
10.40 0 0.00 34 64.15
12.98 2 3.77 38 71.70
15.56 1 1.89 43 81.13
18.15 0 0.00 44 83.02
20.73 0 0.00 47 88.68
23.32 1 1.89 51 96.23
NA 2 3.77 53 100.00
Employment Rate with a High Suicide Rate
Rate Freq. Percent Cum. Freq. Cum. Percent
37.35 2 3.77 6 11.32
41.98 2 3.77 20 37.74
46.56 7 13.21 25 47.17
51.14 8 15.09 32 60.38
55.72 16 30.19 34 64.15
60.30 4 7.55 38 71.70
64.88 5 9.43 43 81.13
69.46 2 3.77 44 83.02
74.04 3 5.66 47 88.68
78.62 3 5.66 51 96.23
NA 2 3.77 53 100.00
------------------------------------------------------------------------------
Summary of Frequency Distributions
Question 1: What is a number of breast cancer cases associated with a high suicide rate?
The high suicide rate is associated with the low number of breast cancer cases.
Question 2: How HIV rate is associated with a high suicide rate?
The high suicide rate is associated with the low HIV rate.
Question 3: How employment rate is associated with a high suicide rate?
The high suicide rate occurs at 55% of employment rate.
chidujsFollow
Assignment 1
Data set: GapMinder Data. Research question: Is a fertility rate associated with a number of breast cancer cases? Items included in the CodeBook: for fertility rate: Children per woman (total fertility) Children per woman (total fertility), with projections for breast cancer: Breast cancer, deaths per 100,000 women Breast cancer, new cases per 100,000 women Breast cancer, number of female deaths Breast cancer, number of new female cases Literature Review: From original source: http://ww5.komen.org/KomenPerspectives/Does-pregnancy-affect-breast-cancer-risk-and-survival-.html The more children a woman has given birth to, the lower her risk of breast cancer tends to be. Women who have never given birth have a slightly higher risk of breast cancer compared to women who have had more than one child. The hypothesis to explore using GapMinder data set: the higher fertility rate, the lower risk of breast cancer.
0 notes
Text
【現在画像60枚】森田涼花画像、2018年もりたすずか画像最新情報!
森田涼花(もりたすずか)画像 No.21
森田涼花(もりたすずか)画像 No.22
森田涼花(もりたすずか)画像 No.23
森田涼花(もりたすずか)画像 No.24
森田涼花(もりたすずか)画像 No.25
森田涼花(もりたすずか)画像 No.26
森田涼花(もりたすずか)画像 No.27
森田涼花(もりたすずか)画像 No.28
森田涼花(もりたすずか)画像 No.29
森田涼花(もりたすずか)画像 No.30
森田涼花(もりたすずか)画像 No.31
森田涼花(もりたすずか)画像 No.32
森田涼花(もりたすずか)画像 No.33
森田涼花(もりたすずか)画像 No.34
森田涼花(もりたすずか)画像 No.35
森田涼花(もりたすずか)画像 No.36
森田涼花(もりたすずか)画像 No.37
森田涼花(もりたすずか)画像 No.38
森田涼花(もりたすずか)画像 No.39
森田涼花(もりたすずか)画像 No.40
森田涼花(もりたすずか)画像 No.41
森田涼花(もりたすずか)画像 No.42
森田涼花(もりたすずか)画像 No.43
森田涼花(もりたすずか)画像 No.44
森田涼花(もりたすずか)画像 No.45
森田涼花(もりたすずか)画像 No.46
森田涼花(もりたすずか)画像 No.47
森田涼花(もりたすずか)画像 No.48
森田涼花(もりたすずか)画像 No.49
森田涼花(もりたすずか)画像 No.50
森田涼花(もりたすずか)画像 No.51
森田涼花(もりたすずか)画像 No.52
森田涼花(もりたすずか)画像 No.53
森田涼花(もりたすずか)画像 No.54
森田涼花(もりたすずか)画像 No.55
森田涼花(もりたすずか)画像 No.56
森田涼花(もりたすずか)画像 No.57
森田涼花(もりたすずか)画像 No.58
森田涼花(もりたすずか)画像 No.59
森田涼花(もりたすずか)画像 No.60
森田涼花画像40枚追加しました。
森田涼花画像の続きを見る。
【現在画像60枚】森田涼花画像、2018年もりたすずか画像最新情報! 【現在画像60枚】森田涼花画像、2018年もりたすずか画像最新情報! 森田涼花画像40枚追加しました。 森田涼花画像の続きを見る。
10 notes
·
View notes
Photo

↬ 𝐁𝐀𝐑𝐎𝐍. “ 𝒅𝒓𝒐𝒘𝒏𝒆𝒅 𝒊𝒏 𝒕𝒉𝒆 𝒇𝒐𝒓𝒆𝒔𝒕'𝒔 𝒅𝒓𝒆𝒂𝒎. ”
Thank you for your interest in Wanderlust! Unfortunately, you have left out your age information in your application. We hope that you would still consider joining us by submitting another application with us!
0 notes
Text
Hollow
read it on AO3 at http://bit.ly/2LQxH4L
by Disharmony (anga971)
Tekst pierwotnie był tłumaczony przez wspaniałą akken (i publikowany na snarry.hekko), jednak od prawie trzech lat nie pojawiła się aktualizacja, dlatego zdecydowałam się zająć tekstem na własną rękę, pragnąc, by pojawił się w całości po Polsku. W przeciwieństwie do pierwotnej tłumaczki postanowiłam pozostawić tytuł w oryginale.
Words: 2427, Chapters: 1/?, Language: Polski
Fandoms: Harry Potter - J. K. Rowling
Rating: Explicit
Warnings: Creator Chose Not To Use Archive Warnings
Categories: M/M
Characters: Harry Potter, Severus Snape
Relationships: Harry Potter/Severus Snape
Additional Tags: Drama, Translation
read it on the AO3 at http://bit.ly/2LQxH4L
1 note
·
View note