#oncology data analysis
Explore tagged Tumblr posts
Text
youtube
#Global oncology#clinical trials#cancer research#international collaboration#oncology innovation#cancer care equity#globalization in oncology#emerging markets#cancer therapy development#international clinical trials#oncology infrastructure#regulatory challenges#cancer epidemiology#clinical research trends#oncology data analysis#global cancer care#medical collaboration#cancer treatment access#oncology breakthroughs#cancer disparities.#Youtube
0 notes
Text
Peter Ellman, President and CEO of Certis Oncology Solutions – Interview Series
New Post has been published on https://thedigitalinsider.com/peter-ellman-president-and-ceo-of-certis-oncology-solutions-interview-series/
Peter Ellman, President and CEO of Certis Oncology Solutions – Interview Series
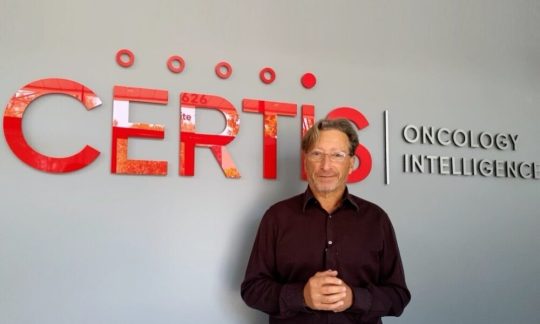
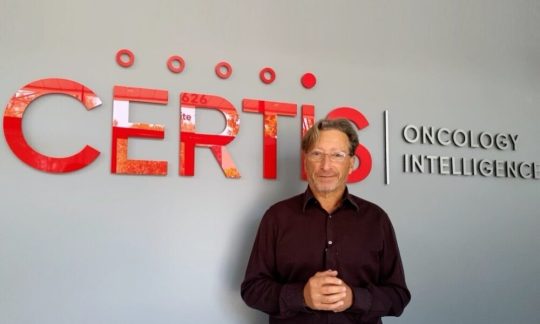
Certis Oncology Solutions, led by Peter Ellman, President and CEO, is a life science technology company dedicated to realizing the promise of precision oncology. The company’s product is Oncology Intelligence® — highly predictive therapeutic response data derived from advanced cancer models. Certis partners with physician-scientists and industry researchers to expand access to precision oncology and address the critical translation gap between preclinical studies and clinical trials.
Can you describe the broader problem in oncology research that the CertisOI Assistant is addressing?
The failure rate of oncology investigational drug candidates is high. It was recently reported that in 2023, 90% of oncology programs ultimately failed. That figure is a remarkable improvement over the historical trend, which hovered around 96% until 2022. Considering the cost of developing drugs, a 90% failure rate is not sustainable. Imagine how patients would benefit if the success rate were even 50%.
CertisOI Assistant immediately addresses two really important issues that contribute to this failure rate:
Improved preclinical model selection: Many compounds show promising results in preclinical studies but fail to demonstrate a sufficient therapeutic effect in humans.Most members of the scientific community point to preclinical models as part of the problem. Choosing preclinical models with the correct gene expression signature (and using orthotopic engraftments for pivotal studies) can improve “translation” into the clinic.
Earlier, better biomarker identification: Relying on biomarkers that do not accurately predict therapeutic response can result in failed clinical trials. CertisOI Assistant is integrated with CertisAI, our patent-pending predictive AI/ML platform, enabling the identification of predictive biomarkers early in the drug development process.
How does the CertisOI Assistant use AI to improve access to oncology data, and what sets it apart from other AI tools in the field?
The CertisOI Assistant provides advanced data analysis and predictive modeling capabilities through an easy-to-use, natural language interface. It stands out in several ways:
Comprehensive Dataset Integration: The assistant integrates a wide range of oncology data, including patient information, tumor characteristics, genetic profiles, and drug response predictions. This holistic approach allows for a more comprehensive analysis than tools focusing on isolated data types.
AI-Based Predictions: The assistant employs AI algorithms to predict drug response and resistance, offering insights into which treatments will likely be effective for specific cancer models. This predictive capability is crucial for personalized medicine and sets it apart from tools that rely solely on historical data.
User-Friendly Interface: By providing an intuitive interface for querying and analyzing complex datasets, the assistant makes it easier for researchers to access and interpret oncology data without requiring advanced technical skills.
Focus on Pre-Clinical Models: The assistant specializes in pre-clinical oncology research, particularly PDX and cell line models, offering unique insights into early-stage drug development and tumor biology.
Interactive Visualizations: The assistant supports interactive visualizations, such as pharmacology and tumor growth studies, enabling researchers to explore data more engaging and informatively.
How does the tool transform complex data into actionable insights, especially for researchers working on drug sensitivity or genomic data?
CertisOI Assistant leverages a structured workflow to transform raw data into meaningful insights. It involves querying a comprehensive oncology dataset, analyzing the data, and presenting the results in a clear and interpretable format. Here’s how it works:
Data Querying: CertisOI Assistant can access a relational database containing detailed information about oncology models, including patient data, tumor characteristics, genomic data, and drug response predictions. It uses SQL-like queries to extract relevant data based on the researcher’s specific needs.
Data Analysis: Once the data is retrieved, CertisOI Assistant can perform various analyses, such as identifying common mutations, correlating gene expression with drug sensitivity, or evaluating pharmacology study results. It can also rank and filter data to highlight the most significant findings.
Visualization: The assistant can present data in tabular formats, generate interactive charts for pharmacology and tumor growth studies, and display histology images. This visualization helps researchers quickly grasp complex data patterns and relationships.
Interpretation and Insights: By providing a clear interpretation of the data, including predictions for drug sensitivity or resistance, CertisOI Assistant helps researchers make informed decisions about potential therapeutic strategies or further experimental directions.
Customization and Flexibility: Researchers can tailor their queries to focus on specific cancer types, genetic markers, or treatment responses, allowing for a highly customized analysis that aligns with their research objectives.
How does the CertisOI Assistant enhance researchers’ ability to select cancer models, design biomarker strategies, or perform in silico validations?
I covered the first two areas – the cancer model section and biomarker strategy design – at the outset of this interview, so I’ll focus on performing in silico validations. CertisOI Assistant provides a virtual environment to test and validate hypotheses related to drug efficacy, target engagement, and biomarker discovery without the need for immediate laboratory experiments. This allows them to rapidly refine their hypotheses and focus experimental efforts on the most promising avenues.
Here are a few examples:
Drug Response Predictions: Use AI-based predictions for drug response and resistance to assess how different models are likely to respond to specific drugs. This can help validate the potential efficacy of a drug in silico before moving to in vitro or in vivo studies.
Genomic and Molecular Profiling: Analyze the genomic data, including mutations, gene expression, and copy number variations, to identify potential targets and validate their relevance to the drug’s mechanism of action. This can help in understanding the molecular basis of drug sensitivity or resistance.
Biomarker Discovery: Correlate molecular characteristics with drug response predictions to identify potential predictive biomarkers. This can guide the selection of patient populations more likely to benefit from a particular therapy.
Combination Therapy Exploration: Explore drug synergy predictions to identify promising drug combinations that may enhance therapeutic outcomes. This can provide insights into potential combination strategies that can be further validated experimentally.
Histological Analysis: Use histology images to validate the morphological effects of drugs on tumor tissues, providing additional evidence for the drug’s mechanism of action and potential efficacy.
Cross-Model Comparisons: Compare different models to understand how various genetic backgrounds influence drug response, helping to validate hypotheses about the role of specific genes or pathways in silico.
Virtual Screening: Perform virtual screening of drugs against a wide range of models to prioritize candidates for further experimental validation.
Can you share examples of how researchers are anticipated to use this tool to improve their workflows or achieve breakthroughs?
The simplest example is preclinical model selection. Every preclinical study begins with the selection of tumor models. CertisOI Assistant takes the manual effort out of this process and brings great precision to selecting the optimal models for any given study.
Another is developing a biomarker strategy. The traditional approach is to hypothesize what biomarker or biomarkers might be linked to the drug’s mechanism of action and then test those hypotheses in preclinical studies, which is usually an iterative process. If preclinical data is promising, researchers must validate predictive biomarkers in human clinical trials—and as discussed, the failure rate is high.
The CertisOI Assistant helps researchers identify and validate more precise, predictive gene expression biomarkers earlier in the development process and with less iteration than the traditional workflow—saving time, and money, and improving chances for commercial success.
What kinds of cancer models or datasets does the tool support, and how does this breadth benefit the research community?
The current version of CertisOI gives researchers access to Certis’ rapidly expanding library of PDX and PDX-derived tumor models and the entire Cancer Cell Line Encyclopedia (CCLE) of models. The platform’s algorithms also draw on data from Genomics of Drug Sensitivity in Cancer (GDSC), International Cancer Genome Consortium (ICGC), CI ALMANAC, O’Neil, and other datasets. This holistic approach to data integration allows for a more comprehensive analysis than tools that focus on isolated data types.
The CertisOI Assistant is designed to be user-friendly. How do you ensure that it is accessible to researchers who may not have extensive technical expertise?
Several features make CertisOI Assistant accessible to researchers at all levels:
Intuitive Interface: The interface is designed to be intuitive and easy to navigate, allowing users to perform complex queries and analyses without needing to understand the underlying technical details.
Guided Workflows: The assistant provides guided workflows for common research tasks, such as querying drug response predictions, analyzing genomic data, and exploring pharmacology studies. This helps users focus on their research questions without getting bogged down in technical complexities.
Natural Language Processing: Users can interact with the assistant using natural language queries, making accessing the information they need easier for those without technical expertise. The assistant interprets the queries and translates them into the appropriate database queries.
Comprehensive Documentation: Detailed documentation and tutorials help users understand how to use the assistant effectively. This includes step-by-step guides, examples, and explanations of key concepts.
Interactive Visualizations: The assistant provides interactive visualizations for data analysis, such as charts and histology images, allowing users to explore and interpret data visually without needing to write code.
Responsive Support: Users can access responsive support to assist with any questions or issues. This ensures they can get help quickly and continue their research without unnecessary delays.
Customizable Queries: While the assistant provides default workflows, it also allows for customization, enabling users to tailor queries to their specific research needs without requiring deep technical knowledge.
Collaboration is a key aspect of research. How does the CertisOI Assistant facilitate teamwork among researchers or institutions?
With CertisOI Assistant, researchers from different teams or institutions can access the same dataset and tools, allowing them to work collaboratively on shared projects or research questions. The platform also makes it easy to download and share data queries, results, and insights among team members so everyone involved in a project can contribute effectively.
What are the biggest challenges in scaling AI adoption in cancer research, and how can they be addressed?
Significant challenges include data security, data integration, and trust in AI‐based outcome predictions. I am not an expert on data security or data integration, but great minds are working to solve those challenges. With respect to trusting AI-generated predictions, we need efficient and credible ways to validate those predictions.
Certis has taken a two-pronged approach to this: in silico validation via internal, cross-validation studies, and in vivo validation—performing studies in clinically relevant mouse models to evaluate the accuracy of our platform’s predictions. Over time, these tools will also be validated clinically in human patients—but of course, that will take a great deal of time and money, as well as the willingness to change the current cancer treatment paradigm. The medical and regulatory community will have to stop relying on how things have always been done and embrace the power of computational analyses to inform decisions.
How do you envision tools like the CertisOI Assistant shaping the future of cancer treatment and precision medicine?
Modern medicine doesn’t yet have a great way to match patients to the ideal treatments. Overall, only 10% of cancer patients experience a clinical benefit from treatments matched to tumor DNA mutations. That not only hurts patients’ health, but it also harms them financially. An estimated $2.5 billion —with a B—is wasted on ineffective therapies. It is a very sad fact that 42% of cancer patients fully deplete their assets by the second year of their diagnosis.
Tools like CertisOI Assistant and CertisAI will help us realize the promise of precision medicine—getting people the optimal treatment for their unique form of cancer the first time, every time…. And to democratize access to more effective, personalized care.
Thank you for the great interview, readers who wish to learn more should visit Certis Oncology Solutions.
#2022#2023#adoption#ai#AI adoption#ai tools#AI/ML#Algorithms#almanac#analyses#Analysis#approach#assets#billion#Biology#biomarkers#Cancer#cancer treatment#cell#CEO#Certis Oncology Solutions#change#charts#clinical#code#Collaboration#Community#comprehensive#course#data
0 notes
Text
#Saudi Arabia Interventional Oncology Market#Market Size#Market Share#Market Trends#Market Analysis#Industry Survey#Market Demand#Top Major Key Player#Market Estimate#Market Segments#Industry Data
0 notes
Text
By Amy Mek March 11, 2025
Over the last few years, the National Citizen’s Inquiry (NCI) has traversed Canada, gathering sworn testimony from experts and victims alike on the disastrous consequences of COVID-19 measures, with a particular emphasis on the experimental mRNA injections.
One of the most harrowing testimonies came from Canadian oncologist Dr. William Makis, who has been at the forefront of exposing the unprecedented rise in aggressive cancers following mass inoculation campaigns. His findings have led to relentless persecution by Canadian authorities, including the Alberta government, which is now seeking a jail term against him for his defiant stance against these gene therapy injections.
The Explosion of ‘Turbo Cancer’ Among the Young
Dr. Makis, a specialist in nuclear medicine and oncology, laid out a damning case against the continued administration of COVID-19 mRNA vaccines, particularly in children. Through meticulous analysis of data from Canada’s adverse event reporting system, the World Health Organization’s VigiAccess, and the U.S. VAERS database, he revealed a horrifying trend—cancers developing at an unprecedented rate and with unparalleled aggression in vaccinated individuals, including children as young as six months old.’
6 notes
·
View notes
Text
Radiotherapy resources in Brazil (RT2030): a comprehensive analysis and projections for 2030
Background
The demand for radiotherapy in Brazil is unfulfilled, and the scarcity of data on the national network hampers the development of effective policies. We aimed to evaluate the current situation, estimate demands and requirements, and provide an action plan to ensure access to radiotherapy for those in need by 2030.
Methods
The Brazilian Society for Radiation Oncology created a task force (RT2030) including physicians, medical physicists, policy makers, patient advocates, and suppliers, all of whom were major stakeholders involved in Brazilian radiotherapy care. The group was further divided into seven working groups to address themes associated with radiotherapy care in Brazil. From March 1, 2019, to Aug 3, 2020, there were monthly meetings between the group's leaders and the Central Committee and six general meetings. First, a comprehensive search of all different national databases was done to identify all radiotherapy centres. Questionnaires evaluating radiotherapy infrastructure and human resources and assing the availability, distribution, capacity, and workload of resources were created and sent to the radioprotection supervisor of each centre. Results were analysed nationally and across the country's regions and health-care systems. A pre-planned review of available databases was done to gather data on active radiation oncology centres and the distribution of radiotherapy machines (linear accelerators [LINACs]) across Brazil. We used national population and cancer incidence projections, recommended radiotherapy usage from the medical literature, and national working patterns to project radiotherapy demands in 2030. An action plan was established with suggestions to address the gaps and meet the demands.
Findings
The database search yielded 279 centres with an active radiotherapy registry. After applying predefined exclusion criteria, 263 centres were identified that provided external beam radiotherapy machines with or without brachytherapy. All 263 operational centres answered the questionnaires sent on Dec 9, 2019, which were then returned between Jan 1 and June 30, 2020. There were 409 therapy machines, 646 radiation oncologists, 533 physicists, and 230 989 patients undergoing radiotherapy (150 628 [65·2%] in the public health-care system and 80 937 [35·0%] in private). The mean annual occupation rate was 566 patients per treatment machine (SD 250). The number of residents per treatment machine ranged from 258 333 to 1 800 000. Technology availability varied considerably among regions and systems. In 2030, 639 994 new cancer cases are expected, which will require 332 797 radiotherapy courses. Therefore, 530 LINACs, 1079 radiation oncologists, and 1060 medical physicists will be needed.
Interpretation
The expected increase in cancer incidence in the coming years will probably increase the disparities in cancer care and the burden for Brazilian patients. We provide a roadmap of the current situation and the particularities of the Brazilian radiotherapy network, which can serve as a starting point for cancer policy planning to improve this scenario.
Read the paper.
#brazil#science#politics#healthcare#brazilian politics#mod nise da silveira#image description in alt
2 notes
·
View notes
Text
Revolutionizing Health: Cancer Care Specialties Mena Transforming Patient Outcomes
Contact Us On: +971 52 747 2414
Cancer continues to be one of the most daunting health challenges across the globe, and the Middle East and North Africa (MENA) region is no exception. But recent advancements in Cancer Care Specialties Mena are not just catching up with global standards—they’re setting new benchmarks. With a combination of modern medical technology, increased awareness, and region-specific approaches, the landscape of cancer care in MENA is evolving fast.
Understanding the Cancer Burden in MENA
The MENA region, home to over 400 million people, faces a growing burden of cancer due to multiple factors: aging populations, urbanization, lifestyle shifts, and environmental risks. According to the World Health Organization, countries in MENA are witnessing rising incidences of breast, lung, colorectal, and prostate cancers.
However, what’s equally significant is how these countries are responding—with strategic investments in cancer care infrastructure, medical expertise, and specialized treatment centers.
Specialized Cancer Centers Making a Mark
Countries like the UAE, Saudi Arabia, Egypt, and Jordan are at the forefront of developing world-class oncology centers. Facilities such as the King Hussein Cancer Center in Jordan, Cleveland Clinic Abu Dhabi, and National Cancer Institute in Egypt are known for delivering comprehensive cancer treatment, including early diagnosis, surgical oncology, radiotherapy, chemotherapy, and palliative care.
What sets these centers apart is their patient-centric approach. Many of them now offer multidisciplinary treatment plans, which means oncologists, surgeons, radiologists, nutritionists, and mental health professionals collaborate to offer holistic care.
Advanced Technology Reshaping Treatment
One of the most promising developments in cancer care specialists in dubai is the adoption of advanced medical technology. Proton therapy, robotic surgery, precision medicine, and immunotherapy are being increasingly integrated into treatment protocols.
Take, for example, artificial intelligence (AI)—AI is being used to detect cancer at early stages through image recognition and data analysis. Meanwhile, genomic testing is helping tailor treatment plans based on individual patient profiles, improving success rates and reducing side effects.
Telemedicine and Remote Oncology Care
With vast geographical landscapes and underserved rural populations, telemedicine is playing a huge role in transforming cancer care access. Countries like Morocco and Tunisia are leveraging tele-oncology platforms to bridge the gap between patients and specialists.
This approach not only reduces the burden on urban hospitals but also ensures early detection and regular follow-ups, which are critical in effective cancer management. The COVID-19 pandemic accelerated this shift, making remote consultations a standard part of oncology practices.
Training and Education: Building a Stronger Workforce
Another key pillar of progress in cancer care is the focus on medical education and professional training. MENA countries are investing heavily in training local oncologists, nurses, and support staff to meet international standards. Partnerships with global cancer institutes have led to exchange programs, virtual seminars, and clinical research collaborations.
This localized expertise is crucial because it ensures culturally sensitive and linguistically appropriate care, improving patient trust and treatment adherence.
Public Awareness and Early Detection Campaigns
Awareness plays a huge role in fighting cancer. Several MENA governments and NGOs have launched campaigns to educate the public about early signs of cancer and the importance of regular screenings. The Pink Caravan initiative in the UAE, for instance, travels across cities and villages to provide free breast cancer screening and awareness sessions.
These campaigns are not just informative—they are lifesaving. When cancer is detected early, the chances of successful treatment go up significantly.
Challenges and the Way Forward
Despite these strides, the journey isn’t without hurdles. Inconsistent access to specialized care, unequal healthcare infrastructure, affordability issues, and stigma surrounding cancer are still significant challenges in some parts of the region.
To overcome these, experts recommend increasing public-private partnerships, improving health insurance coverage, and decentralizing cancer care services to reach remote communities more effectively.
Conclusion: A New Era in Cancer Care
The transformation of best breast surgeons in dubai signals a new era of hope and healing. From cutting-edge technology to specialized centers and growing awareness, the region is charting a powerful course toward better patient outcomes. The future looks optimistic, especially with a growing emphasis on personalized, patient-first care that addresses not just the disease but the person fighting it.
As governments, medical institutions, and communities continue to collaborate, MENA is set to become a hub of excellence in oncology that rivals the best in the world.
0 notes
Text
Generative AI in Healthcare: Market Trends and Opportunities in 2025
The healthcare industry is experiencing a transformative shift in 2025, driven by the rapid integration of generative artificial intelligence (AI). From accelerating drug discovery to enhancing patient care, generative AI is redefining the boundaries of what's possible in medicine. This blog delves into the current market trends, key applications, and emerging opportunities of generative AI in healthcare.

Market Overview: A Rapidly Expanding Frontier
The global generative AI in healthcare market is witnessing exponential growth. Valued at approximately USD 1.8 billion in 2023, it's projected to soar to USD 14.8 billion by 2030, reflecting a compound annual growth rate (CAGR) of 36.7% . This surge is fueled by advancements in deep learning, increasing demand for personalized treatments, and the vast availability of healthcare data.
North America currently leads the market, accounting for over 40% of global revenue in 2023. However, the Asia-Pacific region is emerging as a significant growth area, driven by large patient populations, government initiatives, and rapid technological adoption.
Key Applications of Generative AI in Healthcare
1. Drug Discovery and Development
Generative AI is revolutionizing the pharmaceutical industry by streamlining the drug discovery process. AI algorithms can analyze vast datasets to identify potential drug candidates, predict their efficacy, and optimize molecular structures. This not only accelerates the development timeline but also reduces costs associated with traditional methods.
Notably, startups like Latent Labs, founded by a former DeepMind scientist, are leveraging generative AI to design synthetic proteins, aiming to make biology "programmable" and enhance computational drug discovery.
2. Personalized Medicine
Generative AI enables the creation of individualized treatment plans by analyzing genetic, clinical, and lifestyle data. This approach is particularly impactful in fields like oncology and rare disease treatment, where tailored therapeutic strategies are crucial.
3. Medical Imaging and Diagnostics
AI-powered tools are enhancing the accuracy and speed of medical imaging analysis. For instance, the University of Pittsburgh's CPACE has developed AI tools that produce quick and precise leukemia reports, aiding in faster diagnosis and treatment planning.
4. Clinical Documentation and Workflow Optimization
Generative AI is streamlining clinical documentation by automating the generation of patient notes, reducing administrative burdens, and allowing healthcare professionals to focus more on patient care.
5. Mental Health Support
AI-driven mental health tools, such as chatbot-based interventions, have shown effectiveness in improving symptoms of anxiety and depression, especially for mild to moderate cases. These tools offer accessible and scalable mental health support, complementing traditional therapy methods.
Emerging Opportunities and Innovations
1. Integration with Telemedicine
The fusion of generative AI with telemedicine platforms is enhancing remote diagnostic capabilities. AI-driven tools can assess patient data and medical images, offering real-time insights and recommendations, thereby expanding access to high-quality care in remote and underserved areas.
2. AI-Powered Virtual Health Assistants
Startups like Doctronic are deploying AI agents as intelligent, fast, and personalized alternatives to traditional online medical searches. These AI agents provide users with possible diagnoses and action plans, including options to book real-time video consultations with licensed medical professionals.
3. Workforce Training and AI Literacy
Pharmaceutical companies are emphasizing AI literacy among their workforce. For example, Johnson & Johnson has implemented mandatory generative AI training for over 56,000 employees, while Merck has developed a proprietary platform, GPTeal, to facilitate AI usage and productivity.
Ethical, Legal, and Regulatory Considerations
The integration of generative AI in healthcare raises several ethical and legal questions. Concerns include data privacy, algorithmic biases, and the need for robust regulatory frameworks. International collaborations are essential to develop adaptive policies and regulatory sandboxes to test and refine governance in real-world settings.
Moreover, ethical principles such as governance, reliability, equity, accountability, traceability, privacy, lawfulness, empathy, and autonomy must be considered to ensure responsible deployment of generative AI in healthcare.
Conclusion
Generative AI for Healthcare is poised to transform the healthcare landscape by enhancing diagnostic accuracy, personalizing treatment plans, and streamlining clinical workflows. As the technology continues to evolve, it offers unprecedented opportunities to improve patient outcomes and operational efficiency. However, addressing ethical, legal, and regulatory challenges is crucial to ensure the responsible and equitable integration of generative AI in healthcare.
0 notes
Text
youtube
#Machine learning#colorectal cancer#prognostic signature#epitranscriptomics#m6A modification#m1A modification#m5C modification#m7G modification#bioinformatics#survival prediction#cancer biomarkers#precision oncology#AI in healthcare#omics analysis#feature selection#deep learning#big data#genomics#risk stratification#personalized medicine.#Youtube
0 notes
Text
How Machine Learning Is Revolutionizing Healthcare, Finance & Retail?
We live in an era of unprecedented data. From medical records to stock market fluctuations and shopping habits, vast oceans of information are generated every second. But raw data alone is meaningless. It's the ability to extract insights and make predictions from this data that holds the key to progress across industries. And at the forefront of this transformation lies Machine Learning (ML).
This powerful branch of Artificial Intelligence is no longer a futuristic fantasy. It's actively reshaping how we experience healthcare, manage our finances, and even how we shop. Let's delve into the exciting ways ML is revolutionizing these three crucial sectors.
Healthcare: From Diagnosis to Personalized Treatment
A future where illnesses are caught in their initial stages, therapies are designed specifically for your individual genetic code, and the creation of new medicines happens much faster. This is the promise of Machine Learning in healthcare, and it's rapidly becoming a reality.
Early Diagnosis and Prediction: ML algorithms can analyze medical images (X-rays, MRIs, CT scans) with remarkable accuracy, often surpassing human capabilities in detecting subtle anomalies indicative of diseases like cancer or diabetic retinopathy. They can also analyze patient history, genetic data, and lifestyle factors to predict the likelihood of developing certain conditions, enabling proactive interventions.
Personalized Medicine: The era of universal treatments is fading away and no longer relevant. ML can analyze individual patient data to predict how they might respond to different therapies, allowing doctors to prescribe the most effective and least harmful course of action. This is particularly impactful in areas like oncology and mental health.
Drug Discovery and Development: The traditional drug discovery process is lengthy and expensive. ML algorithms can analyze vast datasets of chemical compounds, biological interactions, and clinical trial results to identify promising drug candidates, predict their efficacy and toxicity, and even design novel molecules, significantly accelerating the development pipeline.
Remote Patient Monitoring and Wearables: ML powers sophisticated wearable devices and remote monitoring systems that track vital signs, sleep patterns, and activity levels. This data can be analyzed to detect early signs of deterioration, manage chronic conditions remotely, and empower patients to take a more active role in their health.
Operational Efficiency: ML is also optimizing hospital operations by predicting patient flow, managing resources efficiently, and automating administrative tasks, freeing up healthcare professionals to focus on patient care.
As per data, ML algorithms now detect lung cancer with 94% accuracy (Nature Medicine, 2019) while reducing drug discovery timelines by 70% (Deloitte, 2023). The precision medicine market is projected to reach $175 billion by 2028 (Grand View Research, 2021), with genetic analysis improving oncology treatment responses by 30-40% (Journal of Clinical Oncology, 2022).
Finance: Smarter Investments, Fraud Prevention, and Personalized Services
The financial industry thrives on data, making it a fertile ground for ML applications. From high-frequency trading to personalized financial advice, ML is transforming how we manage and interact with our money.
Algorithmic Trading: Sophisticated ML algorithms analyze massive amounts of market data in real-time to identify profitable trading opportunities and execute trades at lightning speed, often outperforming human traders.
Fraud Detection and Prevention: Financial institutions are leveraging ML to analyze transaction patterns and identify anomalies that could indicate fraudulent activity, protecting both the institutions and their customers from financial losses.
Credit Risk Assessment: ML models can analyze a wider range of data points than traditional credit scoring methods to provide more accurate and nuanced assessments of creditworthiness, potentially expanding access to credit for underserved populations.
Personalized Financial Advice: Robo-advisors powered by ML algorithms provide personalized investment recommendations, financial planning advice, and portfolio management services based on individual financial goals and risk tolerance, making financial planning more accessible.
Customer Service and Chatbots: ML-powered chatbots are revolutionizing customer service in finance, providing instant answers to queries, guiding users through processes, and freeing up human agents to handle more complex issues.
Algorithmic trading now accounts for 70% of U.S. equity volume (Bloomberg Finance, 2023) and improves returns by 17-23% (Journal of Financial Economics, 2022). ML systems have reduced credit card fraud by 60% (McKinsey, 2022), saving institutions $20 million annually (Gartner, 2023).
Retail: Understanding Consumers and Optimizing Experiences
In the competitive world of retail, understanding customer behavior is paramount. Machine Learning is providing retailers with unprecedented insights, leading to more personalized and efficient operations.
Personalized Recommendations: E-commerce platforms leverage ML algorithms to analyze past purchases, browsing history, and demographic data to recommend products that individual customers are likely to be interested in, driving sales and enhancing the shopping experience.
Demand Forecasting and Inventory Management: ML models can predict future demand for products based on historical sales data, seasonal trends, and external factors, allowing retailers to optimize inventory levels, reduce waste, and avoid stockouts.
Price Optimization: Retailers are using ML to dynamically adjust prices based on factors like demand, competitor pricing, and inventory levels to maximize revenue and profitability.
Customer Segmentation and Targeted Marketing: ML algorithms can segment customers into distinct groups based on their purchasing behavior and preferences, enabling retailers to deliver more targeted and effective marketing campaigns.
Supply Chain Optimization: ML can analyze vast amounts of logistics data to optimize supply chains, predict potential disruptions, and improve delivery efficiency.
Enhanced Customer Experience: From personalized product recommendations to AI-powered chatbots providing customer support, ML is helping retailers create more engaging and seamless shopping experiences.
Recommendation engines drive 35% of Amazon's revenue (Harvard Business Review, 2023), with personalization increasing sales by 15-20% (Salesforce, 2022). ML-powered forecasting reduces inventory costs by 20-30% (McKinsey, 2023), while dynamic pricing increases profit margins by 5-10% (Deloitte, 2022). Retail chatbots now handle 70% of customer inquiries without human intervention (Gartner, 2023).
The Future is Intelligent
The applications of Machine Learning in healthcare, finance, and retail are constantly evolving and expanding. As algorithms become more sophisticated and data availability continues to grow, we can expect even more transformative changes in these industries. While challenges like data privacy, algorithmic bias, and the need for skilled professionals remain, the potential of ML to improve lives, drive efficiency, and create new opportunities is undeniable.
The algorithm is not just processing data; it's learning, adapting, and ultimately, revolutionizing the way we live, work, and interact with the world around us. The future is intelligent, and Machine Learning is leading the charge.
Conclusion
To summarize, the potential power of Machine Learning is undeniably reshaping the landscapes of healthcare, finance, and retail. From enabling earlier disease detection and personalized treatments to fostering smarter financial decisions and more engaging shopping experiences, ML algorithms are extracting invaluable insights from vast datasets, driving efficiency, and creating unprecedented value. While mindful consideration of ethical implications and the need for ongoing development are crucial, the trajectory is clear: Machine Learning is not merely an emerging technology but a fundamental force driving innovation and ushering in a more intelligent future across these critical sectors and beyond. The ability of machines to learn, adapt, and predict is fundamentally altering how these industries operate and ultimately, how we experience the world around us.
0 notes
Link
#AIinmedicaleducation#clinicaltraininginnovation#GPT-4applications#healthcareAIethics#medicalsimulationtechnology
0 notes
Text
¶ … Oral Candidosis in Patients With Advanced Cancer and Nursing Intervention Andrew N. Davies, et al. (2008) in the work entitled: "Oral Candidosis in Community-Based Patients with Advanced Cancer" reports that oral candidosis is a "significant cause of morbidity in patients with advanced cancer." Oral candidosis is described as "an opportunistic infection...relatively common in patients with early cancer, particularly in those with hematological malignancies, those receiving systemic chemotherapy and those receiving head and neck radiotherapy." (Davies, et al., 2008, p. 509) Davies et al. (2008) states that oral candidosis is not "...a single clinical entity, but a spectrum of very different clinical entities." (p.509) Davies et al. (2008) reports a study that included individuals with a diagnosis of advanced cancer and who were over the age of 18 years. The study reported is a "prospective, observational study" which is a study of a qualitative nature. The study contained a clinical component which involved completion of a study questionnaire and assessment of the Eastern Cooperative Oncology Group (ECOG) "performance status, clinical examination of the oral cavity, measurement of the unstimulated whole salivary flow rate, and performance of an oral rinse." (Davies, et al., 2008, p.509) Additionally it is reported that oral swabs were taken from subjects "with appearances indicative of oral candidosis on clinical examination of the oral cavity." (Davies, et al., 2008, p.509) The laboratory component of the protocol is stated to have involved "standard procedures to isolate enumerate, and identify yeasts from the clinical specimens. Yeasts were isolated using CHROMagar Candida and indentified using the API 20 C. Aux yeast identification system, and if necessary DNA sequencing." (Davies, et al., 2008, p.509) Data analysis in the quantitative portion of the study was conducted using the software package Statistical Package for the Social Sciences version 13.0 which calculated the descriptive statistics and this is stated to have included both percentages and frequencies for various clinical and microbiological parameters." (Davies, et al., 2008, p. 509) There were a total of 396 participants in the study reported by Davies, et al. (2008). Findings in the study include that the "prevalence of oral candidosis has been reported to vary between 8% and 94% in patients with advanced cancer." (Davies, et al., 2008, p. 512) A poor ECOG performance status is reported to be "an important risk factor for oral candidosis." (Davies, et al., 2008, p. 512) Findings additionally states that candidosis is "extremely high in patients admitted to hospice." (Davies, et al., 2008, p.512) There are also indications by the study results that there is "an association between systemic corticosteroids and oral candidosis." (Davies, et al., 2008, p.512) There is a "strong association between oral candidosis and the use of topical (inhaled) corticosteroids" however the study did not find an association between "systemic antibiotics and oral candidosis." (Davies, et al., 2008, p.512) The study found that screening for oral candidosis in early cancer is important to begin early treatment before patients develop local symptoms which include those of oral discomfort, taste disturbance and prior to their developing distant complications which includes esophageal candidosis, or systemic candidosis. The work of Chang, Molassiotis, Chan and Lee (2007) entitled: "Nursing Management of Oral Mucositis in Cancer Patients" reports that reduction of oral mucositis development through promotion of patient compliance with oral care regimens "can be achieved by teaching patients meticulous oral care during chemotherapy, reinforcement of these instructions as well as regular nursing assessment of the oral cavity." (p.20) The study reported in the work of Chang, Molassiotis, Chan and Lee (2007) had the aim of determining the "current nursing oral care practice and mucositis-related outcomes for patients undergoing chemotherapy." (p.20) The study was comprised by two phases with the second phase focusing on the provision to nurses of education on oral care and prevention of mucositis and comparison of patient outcomes for those patients who received chemotherapy. Emphasized in the oral care protocol were: (1) regular oral assessment; and (2) frequent mouth rinsing using normal saline or water. (Chang, Molassiotis, Chan and Lee, 2007, p.20) The study is reported to have been conducted beginning May 2002 and ending October 2004. Study results state that there was a higher incidence of mucositis in Phase I participants as well as higher level of baseline symptoms and longer duration of cancer when compared to patients in Phase II of this study. There was stated to be low adherence of nurses to oral care principles for those patients who were undergoing chemotherapy in Phase I of the study. However, the adherence of nurses to oral care principles in Phase II of the study was "significantly higher than that in Phase I and for the experimental group it was significantly higher than that for controls." (Chang, Molassiotis, Chan and Lee, 2007, p.22) It is reported by Chang, Molassiotis, Chan and Lee (2007) that the "prevalence of mucositis at day 8 in the phase II study was greater for the experimental group receiving the oral care protocol" however it is reported additionally that there was no statistical significance "adjusted for 5FU chemotherapy, as more of the corresponding patients received such treatment. " The same is stated to be true as of day 16. Stated to be an additional factor that influencing this study's findings was "...the overall low level of mucositis. Moreover, as the assessment of mucositis occurred at fixed days post commencement of chemotherapy, there was no assessment of the pre-treatment levels of mucositis." (Chang, Molassiotis, Chan and Lee, 2007, p.23.) Also reported in the findings of Chang, Molassiotis, Chan and Lee (2007) is: "The Oral Nursing Care Monitor developed for this study indicated an improvement in the level of oral care from phase I to phase II and a significantly higher level of oral care provided by the experimental group nurses in phase II. However as the maximum possible score was 24, the mean of 15.2 shows the need for further improvement in oral care as well as the education and follow-up of nurses in the implementation of oral care for patients receiving chemotherapy." (p.23) The work of Alred, et al. (1991) entitled: "Oral Health in the Terminally Ill: A Cross-Sectional Pilot Survey" reports that patients who are terminally ill "have a number of signs and symptoms related to the mouth. These include mucosal soreness and ulceration, candidosis, glossitis and Xerostomia." (Alred, et al., 1991, p.59) Alred et al. (1991) report a study that included 20 patients/participants who were all suffering from malignant disease that was advanced and formed. Not included were patients with AIDS and cardiac disease. The group was chosen through random sampling and involved measurement of salivary flow rates. Mouth dryness was stated to have been "only ascribed to the extreme situations, in that there was almost total lack of saliva at any sites in the mouth." (Alred, et al., 1991, p. 59) Details were secured concerning the participants "edentulous/teeth present / partial denture; presence of dental care is (decayed teeth); oral hygiene using the criteria of the Debris Index, recorded form the buccal surfaces of all teeth present, gingivitis based on gingival inflammation: graded as no or minimal inflammation, mild gingivitis, moderate gingivitis, or severe gingivitis; xerostomia, mucosal abnormalities; and denture type and quality of fit (good moderate or poor)." (Alred, et al., 1991, p. 60) The study reported by Alred, et al. (1991) states that 75% of the patients "complained of at least one oral symptom, indicating a high prevalence of oral signs and symptoms among patients with terminal illness." (Alred, et al., 1991, p. 61) Alred, et al. (1991) state that 60% of denture wearers "wore their prostheses continuously...despite professional recognition of the importance of leaving dentures out at night..." (p. 63) This emphasized the importance of clearly explaining to patients "the values of leaving dentures in a denture cleaner overnight...particularly in view of their high rate of candidal carriage and candidiasis." (Alred, et al., 1991, p.63) Conclusions in the study of Alred, et al. (1991) state a prevalence of "...oral coliform carriage in a group of healthy adults (median age 62 years) has been reported as 12%" compared with 47% of the terminally ill patients." (p.63) In addition it is reported that "An increased prevalence of coliforms has also been reported in the oral flora of patients on cytotoxic therapy for malignant disease," in patients who have received radiotherapy for oral and laryngeal cancer, and in patients with acute leukemia, where their importance as a potential source of systemic infection has been implied." (Alred, et al., 1991, p. 63) The work of Davies, Brailsford and Beighton (2005) entitled: "Oral Candidosis in Patients with Advanced Cancer" reports a study with the objective of determining "the epidemiology, aetiology, clinical features and microbiological aspects of oral candidosis in a cohort of cancer patients receiving specialist palliative care." (p.698) The study reported is stated to be a collaborative study involving the Department of Palliative Medicine at the Royal Marsden Hospital and the Dental Institute at Kings College London. The inclusion criteria for the study were: (1) diagnosis of advanced cancer, i.e. incurable cancer; (2) referral to the Department of Palliative Medicine. The exclusion criteria for the study were: (1) estimated prognosis of less than 1 week; (2) significant cognitive impairment, i.e. unable to provide consent; (3) significant physical impairment, i.e. unable to complete protocol; (4) clinical evidence of dehydration; (5) current/recent (less than 2 weeks) use of antifungal medication. (Davies, Brailsford and Beighton, 2005) Davies, Brailsford and Beighton (2005) report that the clinical component of the study involved "...completion of a study questionnaire (demographic data and clinical data), completion of the Memorial Symptom Assessment Scale, clinical examination of the oral cavity, measurement of the unstimulated whole salivary flow rate (UWSFR), measurement of the stimulated whole salivary flow rate (SWSFR), and performance of an oral rinse." ( p.698) The study results states that all patients/participants "...had either locally advanced, or metastatic cancer. The prevalence of different cancer diagnoses were: carcinoma breast -- 40%; carcinoma bronchus -- 16.5%; carcinoma of prostate -- 15%; carcinoma colon/rectum -- 6%; others Read the full article
0 notes
Text
The Cancer Biomarkers Market: Accelerating Precision Oncology with Innovation
Market Overview
The cancer biomarkers market is projected to be valued at USD 21.37 billion in 2025 and is expected to grow to USD 32.54 billion by 2030, with a compound annual growth rate (CAGR) of 8.77% during the forecast period from 2025 to 2030. This growth is fueled by several key factors:
Rising Cancer Incidence: The global increase in cancer cases necessitates improved diagnostic and therapeutic solutions.
Advancements in Biomarker Discovery: Innovations in genomics, proteomics, and metabolomics are enhancing our understanding of cancer biology.
Shift Towards Personalized Medicine: There's a growing emphasis on treatments tailored to individual genetic profiles.
Key Drivers of Market Growth
Technological Advancements: The integration of next-generation sequencing (NGS), mass spectrometry, and artificial intelligence (AI) is revolutionizing biomarker discovery and validation processes.
Liquid Biopsy Technologies: Non-invasive tests analyzing circulating tumor DNA (ctDNA) are gaining popularity for early cancer detection and monitoring treatment responses.
Regulatory Approvals: The increasing number of FDA-approved biomarkers is facilitating the development of companion diagnostics and targeted therapies.
Collaborations and Investments: Partnerships between pharmaceutical companies and diagnostic firms are accelerating the development of novel biomarkers and diagnostic tools.
Technological Innovations Shaping the Industry
Artificial Intelligence and Machine Learning: AI algorithms are being employed to analyze complex biomarker data, identify novel biomarkers, and predict patient responses to treatments.
Multi-Omics Approaches: Combining genomics, proteomics, and metabolomics provides a comprehensive understanding of cancer biology, leading to the discovery of more precise biomarkers.
Imaging Technologies: Advanced imaging techniques, such as MRI and PET scans, are being integrated with biomarker analysis to enhance diagnostic accuracy.
Regional Insights
North America: Dominates the market due to a well-established healthcare infrastructure, substantial research investments, and favorable regulatory policies.
Asia-Pacific: Expected to witness the highest growth rate, driven by increasing healthcare expenditures, rising cancer incidence, and growing awareness of personalized medicine.
Europe: Exhibits steady growth, supported by robust healthcare systems and ongoing research initiatives.
Challenges and Opportunities
Challenges:
High Development Costs: The discovery and validation of new biomarkers require significant financial investments.
Regulatory Hurdles: Navigating complex regulatory pathways can delay the introduction of new biomarkers to the market.
Data Privacy Concerns: The handling of sensitive patient data necessitates stringent security measures.
Opportunities:
Emerging Markets: Expanding healthcare access in developing regions presents opportunities for biomarker-based diagnostics.
Companion Diagnostics: The rise of targeted therapies increases the demand for companion diagnostic tests.
Government Initiatives: Supportive policies and funding can accelerate biomarker research and commercialization.
Conclusion
The cancer biomarkers market is at the forefront of transforming oncology through precision medicine. As technological innovations continue to emerge and global collaborations intensify, the future holds promise for more effective and personalized cancer care solutions. Stakeholders across the healthcare ecosystem must collaborate to overcome challenges and harness the full potential of cancer biomarkers in improving patient outcomes.
For a detailed overview and more insights, you can refer to the full market research report by Mordor Intelligence
1 note
·
View note
Text
Introduction Cancer has become one of the major burdens in the healthcare system, leading to high mortality rates and significantly decreasing the quality of life of the surviving patients. In many cases, healthcare professionals manage to save their patients’ lives through surgical operations. However, there exists a considerable risk of hospital readmissions after such operations for cancer patients. According to Stitzenberg, Chang, Smith, and Nielsen (2015), such readmissions lead to higher costs and lower patient outcomes. Scholars emphasize the importance of coming up with effective interventions that could minimize the burden of readmissions among cancer patients that have undergone surgery. The focus of the present review of literature is on identifying the level of the problem and investigating the extent to which the issue has been researched. The articles under investigation come from scholarly peer-reviewed journals and contain the latest research results. The significance of the identified problem is manifested in the statistical data on post-operative cancer patients’ readmission rates and on the negative effects resulting from such readmissions. Cancer patients are defined as the individuals ill with the oncology disease that have undergone surgery. Readmission is designated as the process of repeated admission to the hospital after having been discharged. Intervention is defined as a viable solution to solve the stated problem. The purpose of this review is to investigate the PICOT question, which has been formulated in the following way: - In patients who have undergone cancer surgery (P), - does the use of the early screen for discharge planning (ESDP) intervention (I), - compared to no use of such a tool (C), - resulting in reduced readmission rates (O) - within a 30- and 90-day period (T)? Methods The search of the literature was performed with the help of such databases as Cochrane Library, EBSCOhost, MedlinePlus, and PubMed. These search engines are designed specifically for medical research and offer their users access to a vast variety of materials on healthcare subjects. The keywords that were used to limit search results included “readmission,” “cancer surgery,” “oncology surgery,” and “cancer patients.” The inclusion criteria were concerned with the type of the source (peer-reviewed scholarly articles) and the time of publication (within the past five years). With the help of these criteria, nearly fifty matches were found. Then, the articles that were the most relevant to the present paper were selected. The credibility of the sources is manifested through the authors’ experience, as well as through the sample size and research performed. Results Out of the articles located as a result of the search, several foci on post-surgery oncology patients and a few discuss the problems of readmissions. The source that covers the aspects of the PICOT question most comprehensively is the study by Socwell et al. (2018). In their research, the authors investigate the feasibility of using the ESDP in predicting the readmission risk of oncology patients. The data were collected with the help of a cohort taken from the Peter MacCallum Cancer Centre. Socwell et al. (2018) note that although the ESDP is not a significant predictor of the patients’ length of stay, this factor can be useful in forecasting discharge destination and readmission. In particular, the negative ESDP score is associated with the 14-day readmission. As a result, Socwell et al. (2018) remark that the ESDP score has the potential to predict readmissions. The major strength of this study is the analysis of the possible ways of reducing readmissions. The limitation is that the authors focus on a 14-day readmission rate whereas the PICOT question aims at analyzing the 30- and 90-day rates. In several of the located articles, the issue of hospital readmissions upon surgery in cancer patients is analyzed. The purpose of the study performed by Mays, Worley, Ackall, D’Agostino, and Waltonen (2015) is to investigate the effect of gastronomy tube (G-tube) placement timing on post-surgery outcomes for cancer patients. 793 cases were identified with the help of the retrospective review of patient records from the Wake Forest Baptist Health Otolaryngology-Head and Neck Oncology clinic (Mays et al., 2015) The major findings of the study are concerned with the connection between the information available to physicians before the operation and the surgery’s success. Mays et al. (2015) conclude that to reduce readmissions of oncology patients, it is crucial to make sure that there are no complications during the operation. The main strength of this research is the large sample size, whereas the biggest limitation is that data was mainly dependent on clinic notes. The study by Stitzenberg et al. (2015) also focuses on postoperative readmissions. The data were collected with the help of the Medicare-linked database, the Surveillance, Epidemiology, and End Results. The major findings are concerned with the readmission predictors, which are the length of stay, the discharge destination, comorbidities, long travel distance, and a higher stage at diagnosis. The strengths of the study include the investigation of readmission predictors and the 90-day readmission rate, which coincides with the PICOT question. The major limitation is that only the older population was included whereas younger patients’ characteristics may be quite different. Two other of the located sources deal with the causes of readmission following surgery and preparatory education for cancer patients undergoing such operations. The purpose of Merkow et al.’s (2015) study is to investigate the factors related to unplanned post-surgical readmissions. The data collection was performed with the help of the American College of Surgeons National Surgical Quality Improvement Program, which collects clinical readmission evidence. The major findings indicate that the most common reasons for readmissions are surgical site infection and obstruction (Merkow et al., 2015). The strength of the article is the identification of possible readmission triggers. The limitations include the impossibility to be completely sure of the reasons for readmissions. The objective of Waller et al.’s (2015) research is to analyze the effect of pre-operative education of cancer patients on readmission rates. The data for the analysis were collected from EMBASE, Medline, and PsychINFO databases. The findings indicate that educational interventions lead to improved patient satisfaction and knowledge. The strength of the study is the investigation of interventions aimed at improving the quality of health. The limitation is concerned with the inclusion criteria preferred by the authors. Discussion The reviewed articles signify that there is much interest of researchers in the issue of hospital readmissions in oncology patients. However, some studies are limited by patients’ age (Stitzenberg et al., 2015), and some focus on a shorter readmission period than included in the PICOT question (Socwell et al., 2018). Still, the authors of all articles agree that readmission rates should be reduced both for the sake of patients’ quality of life and hospitals’ expenditures. Conclusion The review of literature offered an insight into the investigated problem. The major findings allow concluding that the question of readmission rate reduction has gained substantial interest from scholars, but a viable solution for post-operative cancer patients has not been found yet. Overall, the combination of all articles’ findings provides some crucial data on the PICOT question. Further investigation should be focused on various age groups of patients and different readmission periods. References Mays, A. C., Worley, M., Ackall, F., D’Agostino, R., & Waltonen, J. D. (2015). The association between gastrostomy tube placement, poor post-operative outcomes, and hospital re-admissions in head and neck cancer patients. Surgical Oncology, 24(3), 248-257. Merkow, R. P., Ju, M. H., Chung, J. W., Hall, B. L., Cohen, M. E., Williams, M. V.,… Bilimoria, K. Y. (2015). Underlying reasons associated with hospital readmission following surgery in the United States. JAMA, 313(5), 483-495. Socwell, C. P., Bucci, L., Patchell, S., Kotowicz, E., Edbrooke, L., & Pope, R. (2018). Utility of Mayo Clinic’s early screen for discharge planning tool for predicting patient length of stay, discharge destination, and readmission risk in an inpatient oncology cohort. Supportive Care in Cancer, 26(11), 3843-3849. Stitzenberg, K. B., Chang, Y., Smith, A. B., & Nielsen, M. E. (2015). Exploring the burden of inpatient readmissions after major cancer surgery. Journal of Clinical Oncology, 33(5), 455-464. Waller, A., Forshaw, K., Bryant, J., Carey, M., Boyes, A., & Sanson-Fisher, R. (2015). Preparatory education for cancer patients undergoing surgery: A systematic review of volume and quality of research output over time. Patient Education and Counseling, 98(12), 1540-1549. Read the full article
0 notes
Text
Levorphanol Tartrate Market set to hit $551.6 million by 2035
Industry revenue for Levorphanol Tartrate is estimated to rise to $551.6 million by 2035 from $290.6 million of 2024. The revenue growth of market players is expected to average at 6.0% annually for the period 2024 to 2035.
Detailed Analysis - https://datastringconsulting.com/industry-analysis/levorphanol-tartrate-market-research-report
Levorphanol Tartrate is critical across several key applications including pain management, anesthesia and addiction treatment. The report unwinds growth & revenue expansion opportunities at Levorphanol Tartrate’s Dosage Form, Application, Manufacturing Process, Distribution Channel and End User including industry revenue forecast.
Industry Leadership and Competitive Landscape
The Levorphanol Tartrate market is characterized by intense competition, with a number of leading players such as Johnson & Johnson, Pfizer Inc., Amneal Pharmaceuticals LLC, Teva Pharmaceutical Industries Ltd., Mylan Pharmaceuticals Inc., Sun Pharmaceutical Industries Ltd., Lupin Limited, Ranbaxy Laboratories Ltd., Daiichi Sankyo Co. Ltd., Fresenius Kabi Oncology Ltd., Sandoz Inc. and Novartis AG..
The Levorphanol Tartrate market is projected to expand substantially, driven by rising prevalence of chronic pain conditions and advancement in drug delivery systems. This growth is expected to be further supported by Industry trends like Inclusion in Palliative Care.
Moreover, the key opportunities, such as untapped markets, technological advancements and strategic partnerships, are anticipated to create revenue pockets in major demand hubs including U.S., China, Germany, UK and Japan.
Regional Shifts and Evolving Supply Chains
North America and Asia-Pacific are the two most active and leading regions in the market. With challenges like stringent regulatory guidelines and risk of opioid misuse and addiction, Levorphanol Tartrate market’s supply chain from raw material procurement / api production / formulation & development to end user distribution is expected to evolve & expand further; and industry players will make strategic advancement in emerging markets including Brazil, South Africa and Thailand for revenue diversification and TAM expansion.
About DataString Consulting
DataString Consulting offers a complete range of market research and business intelligence solutions for both B2C and B2B markets all under one roof. We offer bespoke market research projects designed to meet the specific strategic objectives of the business. DataString’s leadership team has more than 30 years of combined experience in Market & business research and strategy advisory across the world. DataString Consulting’s data aggregators and Industry experts monitor high growth segments within more than 15 industries on an ongoing basis.
DataString Consulting is a professional market research company which aims at providing all the market & business research solutions under one roof. Get the right insights for your goals with our unique approach to market research and precisely tailored solutions. We offer services in strategy consulting, comprehensive opportunity assessment across various sectors, and solution-oriented approaches to solve business problems.
0 notes