#logestic
Explore tagged Tumblr posts
Text
remember when we thought we would get videos every day in june? yeah we were fucking stupid.
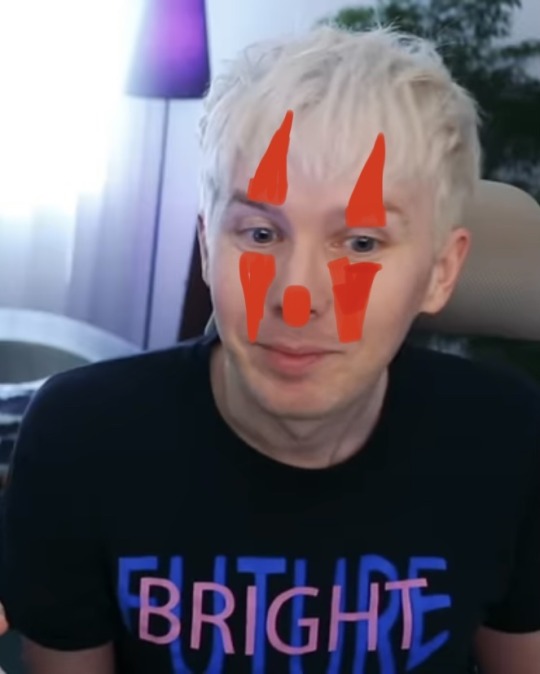
#do you all like my drawing of clown phil? i worked very hard on it. clearly.#also just logestically- monetization wise- gaymingmas just never made sense. it would never be worth the amount of effort#however i love that we were all dreamers 💗#phan#dan and phil#dnp#dan and phil games#amazingphil#danisnotonfire#daniel howell
219 notes
·
View notes
Photo
A very important shot out to amazing animatics I’ve managed to find for some of my favorite Rise fics!
Links to the animatics and fics under the cut. Check out these creators!
Oyasumi [Rottmnt fic Animatic] Every Night the Longest Day - Animatic by Kryptid Krackers
Every Night the Longest Day by ashtreelane
TRAUMA | Animation meme | ROTTMNT FIC - There must be something under the water | - Animatic by 『 Sherbet • Studios 』
There Must be Something in the Water by Filsamek
Treehouse [ by Alex G | rottmnt animatic] - Animatic by Chiren_Exe
Rotten Reflections by @nicoforlifetrue
And a bonus shout out to Pieces - I May Be Invisible PMV (ROTTMNT AU) by Javaskulls, detailing a scene from I May be Invisible but I Still Look Good by @dandylovesturtles
(Spoilers in the animatics for the fics. Be safe and read tags carefully! Love you all!)
#rottmnt#this is so so important guys#save rottmnt#unpause rise of the tmnt#animatics#fics#meme#every night the logest day#there must be something in the water#rotten reflections#my loves#i may be invisible but i still look good#and of course check out those wonderful LFLS stuff#chefs kiss#those two full length animatics are gorgeous
282 notes
·
View notes
Text
Me: Agreed. You should love your friends—
Friends: *get the wrong idea and ghost you*
Me: ...
Me: *tears up* *starts singing the chorus of Carried Away by Shawn Mendes*
Reblog if you think it’s okay to platonically say “I Love You” to your friends
745K notes
·
View notes
Text

May 22 2023
Lunch for everyone!
Rice | Pepper and corn mixed in
Pickle chicken | Canned chicken with garlic, a little bbq sauce, and a splash of pickle juice. Seasoned with old bay and a few other things
Green beans | garlic oil and bacon bits
0 notes
Text
Well, this will probably end up being the logest chapter in the fic once I'm finished. This ones a fun one after the angst :> it'll probably be posted soonish so yay! Sorry for how long it took though, writers block is a BITCH, but hey, got there eventually.
#amangela#amangela fic <3#you know im such a fool for you#ykisaffy#it HOPEFULLY wont be too ling before this posted :>#typed 5k words in one night after having writers block for 5 months....#what ever works i guess LMAO
11 notes
·
View notes
Text
Ask game time!
Thank you @heavensblessing-official for the tag <3
Favourite colour: I don't have one, but I love black, yellow, and pink.
Last song: Not a song, a piece, Piano Concerto in A minor, Op. 7 Allegro Maestroso by Clara Schumann.
Last Movie: The World to Come (2021.)
Currently watching: Tian Guan Ci Fu S2 and Killing Eve.
Currently working on: embroidery, and oh so many fics, (Trying to finish this weekend the logest fic I've ever written; it's a hellcheer fic!! this couple has taking my attention, but I know it's not going to last long, it's a thing of one time. My heart and attention will always belong to danmei <3)
Current obsession: ALWAYS TGCF and 2ha!! and for the last month hellcheer.
And now 😈 tagging the last 5 blogs who left a note in this blog 🔥 Feel free to ignore.
@thereshallbespringagain // @thepizzamanisaneva // @nightwalking-fae // @rquartz94 // @half-eaten-mantou //
3 notes
·
View notes
Text
little doest thou know, I consume locaine thrice daily! I am immune to thoustest sillyest poison. Thousust shallest logest offest insteadicus!

Tumblr, I propose a battle of wits!
I have put Iocaine powder in one of these two goblets. You choose, then we both drink.
55K notes
·
View notes
Text
youtube
Tony and susan alamo "christian" foundation episode. Logest ive ever made
0 notes
Text
dirdiri Logestic Binary Regression On Pima Data
Dealing with PIMA -Diabetes Data through Logistic Regression
Edlirdiri Fadol Ibrahim
30 May 2019
Introduction
The Pima are a group of Native Americans living in Arizona. A genetic predisposition allowed this group to survive normally to a diet poor of carbohydrates for years. In the recent years, because of a sudden shift from traditional agricultural crops to processed foods, together with a decline in physical activity, made them develop the highest prevalence of type 2 diabetes and for this reason they have been subject of many studies.
Dataset
The dataset includes data from 768 women with 8 characteristics, in particular:
1-Number of times pregnant
2-Plasma glucose concentration a 2 hours in an oral glucose tolerance test
3-Diastolic blood pressure (mm Hg)
4-Triceps skin fold thickness (mm)
5-Hour serum insulin (mu U/ml)
6-Body mass index (weight in kg/(height in m)^2) 7-Diabetes pedigree function 8-Age (years)
(Binary):
The last column of the dataset indicates if the person has been diagnosed with diabetes (1) or not (0)
Source
The original dataset is available at UCI Machine Learning Repository and can be downloaded from this address: http://archive.ics.uci.edu/ml/datasets/Pima+Indians+Diabetes
The problem
The type of dataset and problem is a classic supervised binary classification. Given a number of elements all with certain characteristics (features), we want to build a machine learning model to identify people affected by type 2 diabetes.
To solve the problem we will have to analyse the data, do any required transformation and normalisation, apply a machine learning algorithm, train a model, check the performance of the trained model and iterate with other algorithms until we find the most performant for our type of dataset.
###Imports and configuration
In [74]:
import pandas
import numpy as np
import pandas as pd
import matplotlib.pyplot as plt
import os
import pandas as pd
import numpy as np
# render the plot inline, instead of in a separate window
%matplotlib inline
Imports and configuration
In [75]:
import os
import pandas as pd
import numpy as np
In [76]:
DATASET_PATH = 'datasets/'
Load the dataset
In [77]:
# We read the data from the CSV file
data_path = os.path.join(DATASET_PATH, 'pima-indians-diabetes (1).csv')
dataset = pd.read_csv('pima-indians-diabetes (1).csv', header=None)
In [78]:
dataset
Out[78]:012345678
061487235033.60.627501
11856629026.60.351310
28183640023.30.672321
318966239428.10.167210
40137403516843.12.288331
55116740025.60.201300
637850328831.00.248261
71011500035.30.134290
82197704554330.50.158531
9812596000.00.232541
104110920037.60.191300
1110168740038.00.537341
1210139800027.11.441570
131189602384630.10.398591
145166721917525.80.587511
15710000030.00.484321
160118844723045.80.551311
177107740029.60.254311
18110330388343.30.183330
19111570309634.60.529321
203126884123539.30.704270
21899840035.40.388500
227196900039.80.451411
2391198035029.00.263291
2411143943314636.60.254511
2510125702611531.10.205411
267147760039.40.257431
27197661514023.20.487220
2813145821911022.20.245570
295117920034.10.337380
..............................
738299601716036.60.453210
7391102740039.50.293421
74011120803715042.30.785481
741310244209430.80.400260
7421109581811628.50.219220
7439140940032.70.734451
74413153883714040.61.174390
74512100843310530.00.488460
74611479441049.30.358271
74718174415746.31.096320
7483187702220036.40.408361
7496162620024.30.178501
7504136700031.21.182221
751112178397439.00.261280
75231086224026.00.223250
7530181884451043.30.222261
75481547832032.40.443451
7551128883911036.51.057371
75671379041032.00.391390
7570123720036.30.258521
7581106760037.50.197260
7596190920035.50.278661
76028858261628.40.766220
76191707431044.00.403431
762989620022.50.142330
76310101764818032.90.171630
76421227027036.80.340270
7655121722311226.20.245300
7661126600030.10.349471
7671937031030.40.315230
768 rows × 9 columns
In [79]:
dataset.columns = [
"NumTimesPrg", "PlGlcConc", "BloodP",
"SkinThick", "TwoHourSerIns", "BMI",
"DiPedFunc", "Age", "HasDiabetes"]
Inspect the Dataset
In [80]:
# Check the shape of the data: we have 768 rows and 9 columns:
# the first 8 columns are features while the last one
# is the supervised label (1 = has diabetes, 0 = no diabetes)
dataset.shape
Out[80]:
(768, 9)
In [81]:
# Visualise a table with the first rows of the dataset, to
# better understand the data format
dataset.head()
Out[81]:NumTimesPrgPlGlcConcBloodPSkinThickTwoHourSerInsBMIDiPedFuncAgeHasDiabetes
061487235033.60.627501
11856629026.60.351310
28183640023.30.672321
318966239428.10.167210
40137403516843.12.288331
Data correlation matrix
The correlation matrix is an important tool to understand the correlation between the different characteristics. The values range from -1 to 1 and the closer a value is to 1 the bettere correlation there is between two characteristics. Let's calculate the correlation matrix for our dataset.
In [82]:
corr = dataset.corr()
corr
Out[82]:NumTimesPrgPlGlcConcBloodPSkinThickTwoHourSerInsBMIDiPedFuncAgeHasDiabetes
NumTimesPrg1.0000000.1294590.141282-0.081672-0.0735350.017683-0.0335230.5443410.221898
PlGlcConc0.1294591.0000000.1525900.0573280.3313570.2210710.1373370.2635140.466581
BloodP0.1412820.1525901.0000000.2073710.0889330.2818050.0412650.2395280.065068
SkinThick-0.0816720.0573280.2073711.0000000.4367830.3925730.183928-0.1139700.074752
TwoHourSerIns-0.0735350.3313570.0889330.4367831.0000000.1978590.185071-0.0421630.130548
BMI0.0176830.2210710.2818050.3925730.1978591.0000000.1406470.0362420.292695
DiPedFunc-0.0335230.1373370.0412650.1839280.1850710.1406471.0000000.0335610.173844
Age0.5443410.2635140.239528-0.113970-0.0421630.0362420.0335611.0000000.238356
HasDiabetes0.2218980.4665810.0650680.0747520.1305480.2926950.1738440.2383561.000000
If you are not a doctor
and you don't have any knowledge of medicine, but from the data you can guess that the greater the age or the BMI of a patient is, the greater probabilities are the patient can develop type 2 diabetes.
In [83]:
%matplotlib inline
import seaborn as sns
sns.heatmap(corr, annot = True)
Out[83]:
<matplotlib.axes._subplots.AxesSubplot at 0x174dcea2358>
Visualise the Dataset
Visualising the data is an important step of the data analysis. With a graphical visualisation of the data we have a better understanding of the various features values distribution: for example we can understand what's the average age of the people or the average BMI etc...
We could of course limit our inspection to the table visualisation, but we could miss important things that may affect our model precision.
In [84]:
import matplotlib.pyplot as plt
dataset.hist(bins=50, figsize=(20, 15))
plt.show()
An important thing I notice in the dataset (and that wasn't obvious at the beginning) is the fact that some people have null (zero) values for some of the features: it's not quite possible to have 0 as BMI or for the blood pressure.
How can we deal with similar values? We will see it later during the data transformation phase.
Data cleaning and transformation
We have noticed from the previous analysis that some patients have missing data for some of the features. Machine learning algorithms don't work very well when the data is missing so we have to find a solution to "clean" the data we have.
The easiest option could be to eliminate all those patients with null/zero values, but in this way we would eliminate a lot of important data.
Another option is to calculate the median value for a specific column and substitute that value everywhere (in the same column) we have zero or null. Let's see how to apply this second method.
In [85]:
# Calculate the median value for BMI
median_bmi = dataset['BMI'].median()
# Substitute it in the BMI column of the
# dataset where values are 0
dataset['BMI'] = dataset['BMI'].replace(
to_replace=0, value=median_bmi)
In [86]:
# Calculate the median value for BloodP
median_bloodp = dataset['BloodP'].median()
# Substitute it in the BloodP column of the
# dataset where values are 0
dataset['BloodP'] = dataset['BloodP'].replace(
to_replace=0, value=median_bloodp)
In [87]:
# Calculate the median value for PlGlcConc
median_plglcconc = dataset['PlGlcConc'].median()
# Substitute it in the PlGlcConc column of the
# dataset where values are 0
dataset['PlGlcConc'] = dataset['PlGlcConc'].replace(
to_replace=0, value=median_plglcconc)
In [88]:
# Calculate the median value for SkinThick
median_skinthick = dataset['SkinThick'].median()
# Substitute it in the SkinThick column of the
# dataset where values are 0
dataset['SkinThick'] = dataset['SkinThick'].replace(
to_replace=0, value=median_skinthick)
In [89]:
# Calculate the median value for TwoHourSerIns
median_twohourserins = dataset['TwoHourSerIns'].median()
# Substitute it in the TwoHourSerIns column of the
# dataset where values are 0
dataset['TwoHourSerIns'] = dataset['TwoHourSerIns'].replace(
to_replace=0, value=median_twohourserins)
I haven't transformed all the columns, because for some values can make sense to be zero (like "Number of times pregnant").
plitting the Dataset
Now that we have transformed the data we need to split the dataset in two parts: a training dataset and a test dataset. Splitting the dataset is a very important step for supervised machine learning models. Basically we are going to use the first part to train the model (ignoring the column with the pre assigned label), then we use the trained model to make predictions on new data (which is the test dataset, not part of the training set) and compare the predicted value with the pre assigned label.
In [90]:
# Split the training dataset in 80% / 20%
from sklearn.model_selection import train_test_split
train_set, test_set = train_test_split(
dataset, test_size=0.2, random_state=42)
In [91]:
# Separate labels from the rest of the dataset
train_set_labels = train_set["HasDiabetes"].copy()
train_set = train_set.drop("HasDiabetes", axis=1)
test_set_labels = test_set["HasDiabetes"].copy()
test_set = test_set.drop("HasDiabetes", axis=1)
Feature Scaling
One of the most important data transformations we need to apply is the features scaling. Basically most of the machine learning algorithms don't work very well if the features have a different set of values. In our case for example the Age ranges from 20 to 80 years old, while the number of times a patient has been pregnant ranges from 0 to 17. For this reason we need to apply a proper transformation.
In [92]:
# Apply a scaler
from sklearn.preprocessing import MinMaxScaler as Scaler
scaler = Scaler()
scaler.fit(train_set)
train_set_scaled = scaler.transform(train_set)
test_set_scaled = scaler.transform(test_set)
Scaled Values
In [93]:
df = pd.DataFrame(data=train_set_scaled)
df.head()
Out[93]:01234567
00.1176470.2580650.4897960.2727270.0198320.2822090.0964990.000000
10.5294120.4387100.5918370.2909090.0198320.2044990.5140910.483333
20.0588240.6129030.2244900.2000000.0829330.2147240.2459440.016667
30.0000000.7548390.2653060.2727270.0198320.0756650.0751490.733333
40.3529410.5806450.5714290.5272730.4278850.5725970.0683180.416667
Select and train a model
It's not possible to know in advance which algorithm will work better with our dataset. We need to compare a few and select the one with the "best score".
Comparing multiple algorithms
To compare multiple algorithms with the same dataset, there is a very nice utility in sklearn called model_selection. We create a list of algorithms and then we score them using the same comparison method. At the end we pick the one with the best score.
In [94]:
# Import all the algorithms we want to test
from sklearn.linear_model import LogisticRegression
from sklearn.neighbors import KNeighborsClassifier
from sklearn.naive_bayes import GaussianNB
from sklearn.svm import SVC
from sklearn.svm import LinearSVC
from sklearn.ensemble import RandomForestClassifier
from sklearn.tree import DecisionTreeRegressor
In [95]:
# Import the slearn utility to compare algorithms
from sklearn import model_selection
In [96]:
# Prepare an array with all the algorithms
models = []
models.append(('LR', LogisticRegression()))
models.append(('KNN', KNeighborsClassifier()))
models.append(('NB', GaussianNB()))
models.append(('SVC', SVC()))
models.append(('LSVC', LinearSVC()))
models.append(('RFC', RandomForestClassifier()))
models.append(('DTR', DecisionTreeRegressor()))
In [97]:
# Prepare the configuration to run the test
seed = 7
results = []
names = []
X = train_set_scaled
Y = train_set_labels
In [98]:
# Every algorithm is tested and results are
# collected and printed
for name, model in models:
kfold = model_selection.KFold(
n_splits=10, random_state=seed)
cv_results = model_selection.cross_val_score(
model, X, Y, cv=kfold, scoring='accuracy')
results.append(cv_results)
names.append(name)
msg = "%s: %f (%f)" % (
name, cv_results.mean(), cv_results.std())
print(msg)
LR: 0.755632 (0.045675) KNN: 0.740984 (0.049627) NB: 0.739450 (0.062140) SVC: 0.757271 (0.037642) LSVC: 0.763802 (0.042701) RFC: 0.749180 (0.039811) DTR: 0.719778 (0.048670)
In [99]:
# boxplot algorithm comparison
fig = plt.figure()
fig.suptitle('Algorithm Comparison')
ax = fig.add_subplot(111)
plt.boxplot(results)
ax.set_xticklabels(names)
plt.show()
It looks like that using this comparison method, the most performant algorithm is SVC.
Find the best parameters for SVC
The default parameters for an algorithm are rarely the best ones for our dataset. Using sklearn we can easily build a parameters grid and try all the possible combinations. At the end we inspect the bestestimator property and get the best ones for our dataset.
In [100]:
from sklearn.model_selection import GridSearchCV
param_grid = {
'C': [1.0, 10.0, 50.0],
'kernel': ['linear', 'rbf', 'poly', 'sigmoid'],
'shrinking': [True, False],
'gamma': ['auto', 1, 0.1],
'coef0': [0.0, 0.1, 0.5]
}
model_svc = SVC()
grid_search = GridSearchCV(
model_svc, param_grid, cv=10, scoring='accuracy')
grid_search.fit(train_set_scaled, train_set_labels)
Out[100]:
GridSearchCV(cv=10, error_score='raise', estimator=SVC(C=1.0, cache_size=200, class_weight=None, coef0=0.0, decision_function_shape='ovr', degree=3, gamma='auto', kernel='rbf', max_iter=-1, probability=False, random_state=None, shrinking=True, tol=0.001, verbose=False), fit_params=None, iid=True, n_jobs=1, param_grid={'C': [1.0, 10.0, 50.0], 'kernel': ['linear', 'rbf', 'poly', 'sigmoid'], 'shrinking': [True, False], 'gamma': ['auto', 1, 0.1], 'coef0': [0.0, 0.1, 0.5]}, pre_dispatch='2*n_jobs', refit=True, return_train_score='warn', scoring='accuracy', verbose=0)
In [101]:
# Print the bext score found
grid_search.best_score_
Out[101]:
0.76872964169381108
Apply the parameters to the model and train it
In [102]:
# Create an instance of the algorithm using parameters
# from best_estimator_ property
svc = grid_search.best_estimator_
# Use the whole dataset to train the model
X = np.append(train_set_scaled, test_set_scaled, axis=0)
Y = np.append(train_set_labels, test_set_labels, axis=0)
# Train the model
svc.fit(X, Y)
Out[102]:
SVC(C=10.0, cache_size=200, class_weight=None, coef0=0.0, decision_function_shape='ovr', degree=3, gamma=0.1, kernel='rbf', max_iter=-1, probability=False, random_state=None, shrinking=True, tol=0.001, verbose=False)
Make a Prediction
In [103]:
# We create a new (fake) person having the three most correated values high
new_df = pd.DataFrame([[6, 168, 72, 35, 0, 43.6, 0.627, 65]])
# We scale those values like the others
new_df_scaled = scaler.transform(new_df)
In [104]:
# We predict the outcome
prediction = svc.predict(new_df_scaled)
In [105]:
# A value of "1" means that this person is likley to have type 2 diabetes
prediction
Out[105]:
array([1], dtype=int64)
Conclusion
We finally find a score of 76% using SVC algorithm and parameters optimisation. Please note that there may be still space for further analysis and optimisation, for example trying different data transformations or trying algorithms that haven't been tested yet. Once again I want to repeat that training a machine learning model to solve a problem with a specific dataset is a try / fail / improve process.
In [ ]:
0 notes
Text
me writign the logest essay ive ver voluntarily written abt my poor widdle broken heart n my feelings for this girl in my drafts and receiving the most beautulf ask ever from my computer friend right when i conclude. is like looking up into the beautiful sky
#and then immediately going back to my drafts and seeing all the other drafts i made abt it in little bits. lol#the post was called my feelings. should i post it lol. i am desperate for understanding and connection! but i am meeting my friend tmr mayb#well talk abt it
1 note
·
View note
Text
The popular comedy show Taarak Mehta Ka Ooltah Chashmah has created a new record. The show completed 13 years on Wednesday, July 28. With this, the show to be aired on the small screen has become the most-running show in television history.
0 notes
Video
tumblr
International Logistics Companies Australia | TSL Australia
TSL is one of the best international logistics companies in Australia. We provide all kinds of services like freight forwarding including third-party logistics, customs brokerage, imports, exports, value-added services, inland transport, first-time customer care, and door-to-door delivery.
For more info visit - https://www.tslaustralia.com/
0 notes
Text
Yes, Excel does this but it also has functions you can use to do linear regression directly: LINEST/LOGEST/TREND/GROWTH!
“We use a circular reference in Excel to do linear regression.” My mind was blown. I had thought, naively perhaps, that circular references in Excel simply created an error. But this data scientist showed me that Excel doesn’t error on circular references—if the computed value of the cell converges. You see, when formulas create a circular reference, Excel will run that computation up to a number of times. If, in those computations, the magnitude of the difference between the most recent and previous computed values for the cell falls below some pre-defined epsilon value (usually a very small number, like 0.00001), Excel will stop recomputing the cell and pretend like it finished successfully. Yeah, really.
624 notes
·
View notes
Text
Logestic & warehousing in Aurangabd with largest serviceability at Shreejee Logestics.
Fastest and safest Logestic & warehousing in Aurangabd with largest serviceability at Shreejee Logestics.
#Logestic & warehousing in Aurangabd#Logestic & warehousing#Logestic in Aurangabd#warehousing in Aurangabd#Aurangabd#Logestic#warehousing
0 notes