#embeddings
Explore tagged Tumblr posts
Text
AI Math Agents
We know that we are in an AI take-off, what is new is that we are in a math take-off. A math take-off is using math as a formal language, beyond the human-facing math-as-math use case, for AI to interface with the computational infrastructure. The message of generative AI and LLMs (large language models like GPT) is not that they speak natural language to humans, but that they speak formal languages (programmatic code, mathematics, physics) to the computational infrastructure, implying the ability to create a much larger problem-solving apparatus for humanity-benefitting applications in biology, energy, and space science, however not without risk.
2 notes
·
View notes
Text
Pipeshift Secures $2.5M to Streamline Open-Source AI Deployment
New Post has been published on https://thedigitalinsider.com/pipeshift-secures-2-5m-to-streamline-open-source-ai-deployment/
Pipeshift Secures $2.5M to Streamline Open-Source AI Deployment


Pipeshift has announced a $2.5 million seed round aimed at providing enterprises with the infrastructure needed to efficiently build, deploy, and manage open-source AI models. As more than 80% of companies move toward open-source GenAI, Pipeshift’s solution removes common bottlenecks related to privacy, control, and the engineering overhead of stitching together multiple components.
Revolutionizing MLOps for GenAI
Pipeshift’s new-age Platform-as-a-Service (PaaS) accelerates AI orchestration by offering a modular MLOps stack that runs on any environment—cloud or on-premises. This end-to-end service covers a wide range of workloads, including LLMs, vision models, audio models, and more. Rather than acting as a GPU broker, Pipeshift hands enterprises direct control of their infrastructure, enabling them to:
Scale from day one with in-built autoscalers, load balancers, and schedulers
Fine-tune or distill open-source models in parallel with real-time tracking of training metrics
Save on GPU costs by hot-swapping fine-tuned models without GPU memory fractioning
Maintain enterprise-grade security to keep proprietary data and IP fully in-house
By consolidating these capabilities into a single platform, Pipeshift simplifies deployment workflows and drastically reduces time-to-production.
Strong Industry Backing
The $2.5 million seed round was led by Y Combinator and SenseAI Ventures, with additional support from Arka Venture Labs, Good News Ventures, Nivesha Ventures, Astir VC, GradCapital, and MyAsiaVC. Esteemed angels like Kulveer Taggar (CEO of Zuess), Umur Cubukcu (CEO of Ubicloud and former Head of PostgreSQL at Azure), and Krishna Mehra (former Head of Engineering at Meta and co-founder of Capillary Technologies) also participated.
Arko Chattopadhyay, Co-Founder and CEO of Pipeshift, stated: “2025 marks the year when GenAI transitions into production and engineering teams are witnessing the benefits of using open-source models in-house. This offers high levels of privacy and control alongside enhanced performance and lower costs. However, this is a complex and expensive process involving multiple components being stitched together. Pipeshift’s enterprise-grade orchestration platform eradicates the need for such extensive engineering investments by not only simplifying deployment but also maximizing the production throughput.”
Rahul Agarwalla, Managing Partner of SenseAI Ventures, observed: “Enterprises prefer open-source GenAI for the benefits of privacy, model ownership, and lower costs. However, transitioning GenAI to production remains a complex and expensive process requiring multiple components to be stitched.” He continued: “Pipeshift’s enterprise-grade orchestration platform eliminates the need for such extensive engineering investments by not only simplifying deployment but also maximizing the production throughput.”
Yash Hemaraj, Founding Partner at Arka Venture Labs and General Partner at BGV, remarked:“We invested in Pipeshift because their innovative platform addresses a critical need in enterprise AI adoption, enabling seamless deployment of open-source language models. The founding team’s deep technical expertise and track record in scaling AI solutions impressed us immensely. Pipeshift’s vision aligns perfectly with our focus on transformative Enterprise AI companies, particularly those bridging the US-India tech corridor, making them an ideal fit for our portfolio.”
Why Pipeshift Stands Out
Founded by Arko Chattopadhyay, Enrique Ferrao, and Pranav Reddy, Pipeshift’s core team has been tackling AI orchestration challenges long before this seed funding. Their experience includes scaling a Llama2-powered enterprise search app for 1,000+ employees on-premises, highlighting firsthand how tricky and resource-intensive private AI deployments can be.
Key differentiators include:
Multi-Cloud Orchestration: Pipeshift seamlessly handles a mix of cloud and on-prem GPUs, ensuring cost optimization and quick failover.
Kubernetes Cluster Management: An end-to-end control panel allows enterprises to create, scale, and oversee Kubernetes clusters without juggling multiple tools.
Model Fine-Tuning & Deployment: Engineers can finetune or distill open-source models using custom datasets or LLM logs, with training metrics viewable in real time.
360° Observability: Integrated dashboards track performance, enabling quick troubleshooting and efficient scaling.
Built for Production, Not Just Experimentation
Companies often face limitations with generic API-based solutions that aren’t built for in-house privacy. Pipeshift flips this model by focusing on secure, on-prem or multi-cloud deployments. The platform supports over 100 large language models, including Llama 3.1, Mistral, and specialized offerings like Deepseek Coder. This diverse selection helps meet the specific performance, cost, and compliance needs of each project.
Some notable benefits include:
Up to 60% in GPU infrastructure cost savings
30x faster time-to-production
6x lower cost compared to GPT/Claude
55% reduction in engineering resources
Looking Ahead
Having already collaborated with over 30 companies, including NetApp, Pipeshift plans to further its mission of delivering powerful open-source AI solutions without the usual complexity. Its single-pane-of-glass approach to MLOps, combined with dedicated onboarding and ongoing account management, ensures enterprises can stay focused on leveraging AI for business outcomes rather than wrestling with infrastructure.
With private data protections, hybrid cloud compatibility, and modular flexibility at its core, Pipeshift is poised to meet the diverse needs of enterprise AI projects. By bridging the gap between open-source innovation and enterprise-grade requirements, the company is paving the way for a new era of agile, secure, and cost-effective AI deployments.
Pipeshift offers end-to-end MLOps orchestration for open-source GenAI workloads—embeddings, vector databases, LLMs, vision models, and audio models—across any cloud or on-prem GPUs..
#000#2025#adoption#agile#ai#AI adoption#AI models#amp#API#app#approach#audio#azure#Business#CEO#Cloud#cluster#clusters#Companies#complexity#compliance#control panel#data#databases#datasets#deepseek#deployment#embeddings#employees#engineering
0 notes
Text
Using Vector Index And Multilingual Embeddings in BigQuery

The Tower of Babel reborn? Using vector search and multilingual embeddings in BigQuery Finding and comprehending reviews in a customer’s favourite language across many languages can be difficult in today’s globalised marketplace. Large datasets, including reviews, may be managed and analysed with BigQuery.
In order to enable customers to search for products or company reviews in their preferred language and obtain results in that language, google cloud describe a solution in this blog post that makes use of BigQuery multilingual embeddings, vector index, and vector search. These technologies translate textual data into numerical vectors, enabling more sophisticated search functions than just matching keywords. This improves the relevancy and accuracy of search results.
Vector Index
A data structure called a Vector Index is intended to enable the vector index function to carry out a more effective vector search of embeddings. In order to enhance search performance when vector index is possible to employ a vector index, the function approximates nearest neighbour search method, which has the trade-off of decreasing recall and yielding more approximate results.
Authorizations and roles
You must have the bigquery tables createIndex IAM permission on the table where the vector index is to be created in order to create one. The bigquery tables deleteIndex permission is required in order to drop a vector index. The rights required to operate with vector indexes are included in each of the preset IAM roles listed below:
Establish a vector index
The build VECTOR INDEX data definition language (DDL) statement can be used to build a vector index.
Access the BigQuery webpage.
Run the subsequent SQL statement in the query editor
Swap out the following:
The vector index you’re creating’s name is vector index. The index and base table are always created in the same project and dataset, therefore these don’t need to be included in the name.
Dataset Name: The dataset name including the table.
Table Name: The column containing the embeddings data’s name in the table.
Column Name:The column name containing the embeddings data is called Column name. ARRAY is the required type for the column. No child fields may exist in the column. The array’s items must all be non null, and each column’s values must have the same array dimensions. Stored Column Name: the vector index’s storage of a top-level table column name. A column cannot have a range type. If a policy tag is present in a column or if the table has a row-level access policy, then stored columns are not used. See Store columns and pre-filter for instructions on turning on saved columns.
Index Type:The vector index building algorithm is denoted by Index type. There is only one supported value: IVF. By specifying IVF, the vector index is constructed as an inverted file index (IVF). An IVF splits the vector data according to the clusters it created using the k-means method. These partitions allow the vector search function to search the vector data more efficiently by limiting the amount of data it must read to provide a result.
Distance Type: When utilizing this index in a vector search, distance type designates the default distance type to be applied. COSINE and EUCLIDEAN are the supported values. The standard is EUCLIDEAN.
While the distance utilised in the vector search function may vary, the index building process always employs EUCLIDEAN distance for training.
The Diatance type value is not used if you supply a value for the distance type argument in the vector search function. Num Lists: an INT64 value that is equal to or less than 5,000 that controls the number of lists the IVF algorithm generates. The IVF method places data points that are closer to one another on the same list, dividing the entire data space into a number of lists equal to num lists. A smaller number for num lists results in fewer lists with more data points, whereas a bigger value produces more lists with fewer data points.
To generate an effective vector search, utilise num list in conjunction with the fraction lists to search argument in the vector list function. Provide a low fraction lists to search value to scan fewer lists in vector search and a high num lists value to generate an index with more lists if your data is dispersed among numerous small groups in the embedding space. When your data is dispersed in bigger, more manageable groups, use a fraction lists to search value that is higher than num lists. Building the vector index may take longer if you use a high num lists value.
In addition to adding another layer of refinement and streamlining the retrieval results for users, google cloud’s solution translates reviews from many languages into the user’s preferred language by utilising the Translation API, which is easily integrated into BigQuery. Users can read and comprehend evaluations in their preferred language, and organisations can readily evaluate and learn from reviews submitted in multiple languages. An illustration of this solution can be seen in the architecture diagram below.
Google cloud took business metadata (such address, category, and so on) and review data (like text, ratings, and other attributes) from Google Local for businesses in Texas up until September 2021. There are reviews in this dataset that are written in multiple languages. Google cloud’s approach allows consumers who would rather read reviews in their native tongue to ask inquiries in that language and obtain the evaluations that are most relevant to their query in that language even if the reviews were originally authored in a different language.
For example, in order to investigate bakeries in Texas, google cloud asked, “Where can I find Cantonese-style buns and authentic Egg Tarts in Houston?” It is difficult to find relevant reviews among thousands of business profiles for these two unique and frequently available bakery delicacies in Asia, but less popular in Houston.
Google cloud system allows users to ask questions in Chinese and get the most appropriate answers in Chinese, even if the reviews were written in other languages at first, such Japanese, English, and so on. This solution greatly improves the user’s ability to extract valuable insights from reviews authored by people speaking different languages by gathering the most pertinent information regardless of the language used in the reviews and translating them into the language requested by the user.
Consumers may browse and search for reviews in the language of their choice without encountering any language hurdles; you can then utilise Gemini to expand the solution by condensing or categorising the reviews that were sought for. By simply adding a search function, you may expand the application of this solution to any product, business reviews, or multilingual datasets, enabling customers to find the answers to their inquiries in the language of their choice. Try it out and think of additional useful data and AI tools you can create using BigQuery!
Read more on govindhtech.com
#Usingvectorindex#Multilingual#Embeddings#BigQuery#vectorsearch#Googlecloud#AItools#Swapout#Authorizations#technology#technews#news#govindhtech
0 notes
Text
Discover the power of Embeddings As a Service - the ultimate solution for efficient data representation! 🚀 Say goodbye to complex data processing and hello to seamless integration. Want to delve deeper into this game-changing technology?
Read more ➡️
0 notes
Text
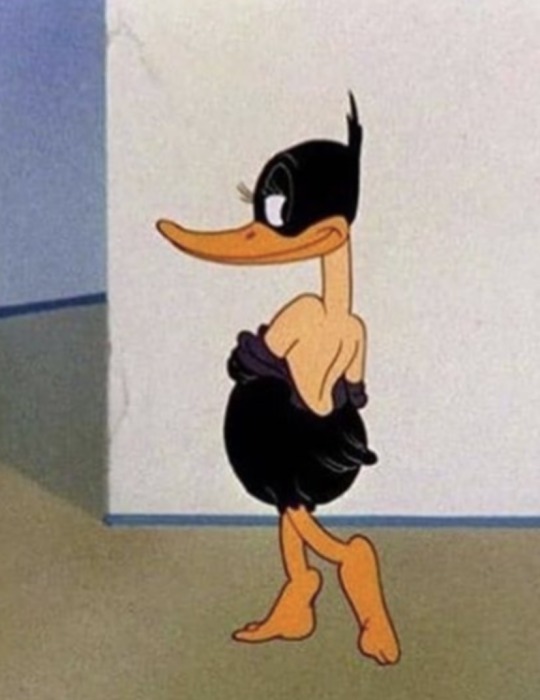
I saw a bumper sticker and thought “is that seductive Daffy Duck” and then when I looked closer I realized it was actually a fishing bumper sticker but also. also it is still very much seductive Daffy Duck???? somehow????????
#imagine selling this to the fishing community without disclosing the hidden seductive daffy embedded within#it is about ethics in fishing-enthusiast merchandising
76K notes
·
View notes
Text
og meme under the cut
3K notes
·
View notes
Text

hitting jayvik with the yuri beam, pt.2
#jayvik#jayce talis#viktor#arcane#jayce x viktor#galaxy draws#adding one more headcanon that fem!jayce wears the gem as a pendant so when mage viktor binds it to her it gets embedded in her chest#using my bisexual powers for nefarious purposes#in that I refuse not to draw the most beautiful lady jayce and viktor#they were made by me#for me
1K notes
·
View notes
Text
a new star
#i been thinkin about the asteroid again#i think about the asteroid a lot more than i think is normal#like just the complete randomness of it and how everything changed in literally the blink of an eye#like the dinoss rules the fucking earth and probably still would if space had just been a little bit different#how long did it take the asteroid to reach us#at what point was the impact inevitable#like these sound like scientific answers but i need you to know these are questions that my soul wants answered in poetry#yes the math is cool but can i talk about what tragedy looks like melted into the earth#how power and pain and mourning but also change and new life and a future were embedded in a layer of iridum that spread around the planet#can we talk about how looking at the layers of the earth is the most physical type of time travel there is#can i please talk about that layer of pain#can i mourn when i see it#or am i just a weird kid crying when i look at rocks#ALSO. was parasaurolophus alive when the asteroid hit? i dont think so#but it's too late#yall get to suffer with me#dinosaurs
2K notes
·
View notes
Text

Astarion class swap🔮🧙♀️ Collab with @heph!
We swapped Gale and Astarions classes in a "what if" scenario. Here's comp I sketched + Rogue!Gale concept :] We honestly think he'd be a terrible rogue lol
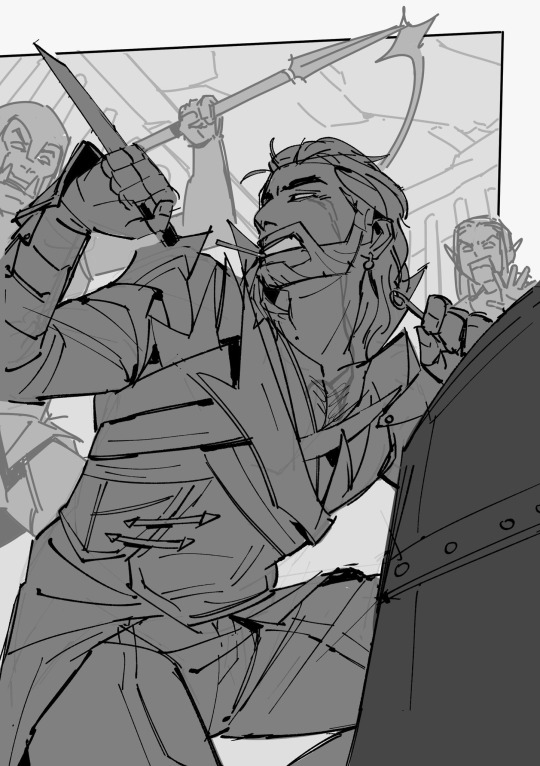

#baldur's gate 3#bg3#astarion#gale dekarios#my art#if you follow the link embeded in the word ‘collab’ you can see heph’s half!
4K notes
·
View notes
Text
the reluctance to acknowledge christianity in a lot of medieval-set fiction/fantasy means we're missing out on a lot of stories of bishops trying to assassinate each other
#the early 13th century bishops of waterford and lismore were high drama#people are getting tortured. there are swords embedded in the doors of cathedrals. etc#history#medieval
2K notes
·
View notes
Text

My big farcille doujin is done!🥳🎉
It’s 30+ pages and basically a rough, very fast-paced retelling of the entirety of dunmeshi (but farcille pov) so its super spoiler-heavy
You can find it here for free
#help me spread bc i fear the embedded link will kill this post#dungeon meshi#delicious in dungeon#farcille#falin touden#marcille donato#my art#comic
4K notes
·
View notes
Text
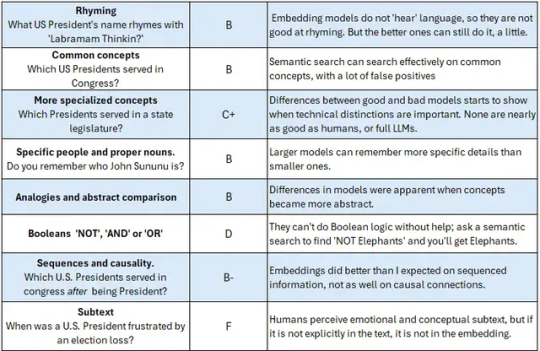
Embeddings Scorecard
0 notes
Text
No phan proof youtube video or tumblr analysis will ever be as convincing as my mothers take on Dan and Phil, which is "of course they're together. Who else is gonna want to date them?"
#dylan says things#dan and phil#dnp#this take from her was about the fact that they literally spend all their time together and are so embedded in each others lives#not about their general personality or likeability#but I just think it's very funny#phan
2K notes
·
View notes
Text
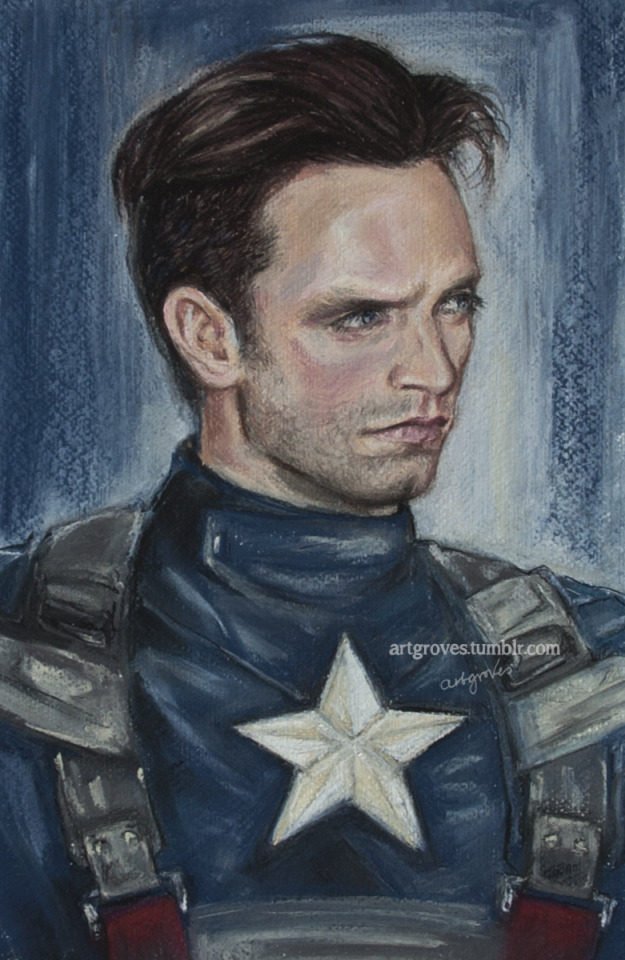
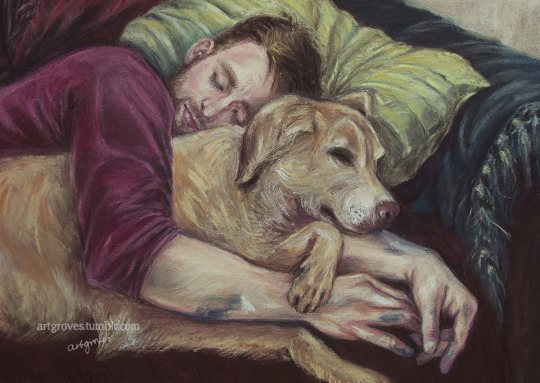

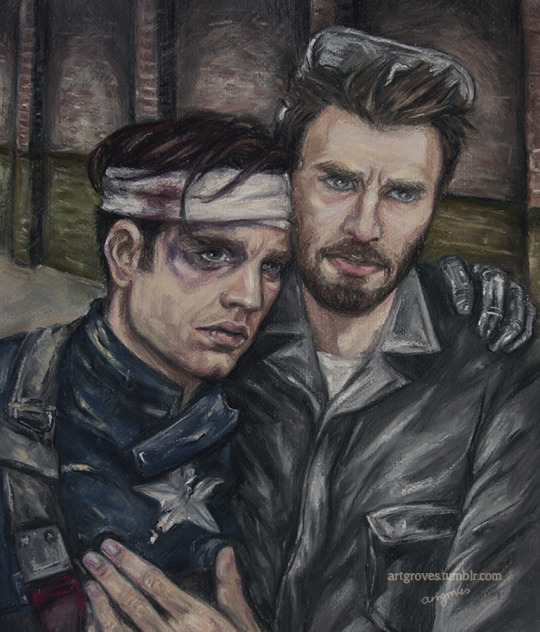
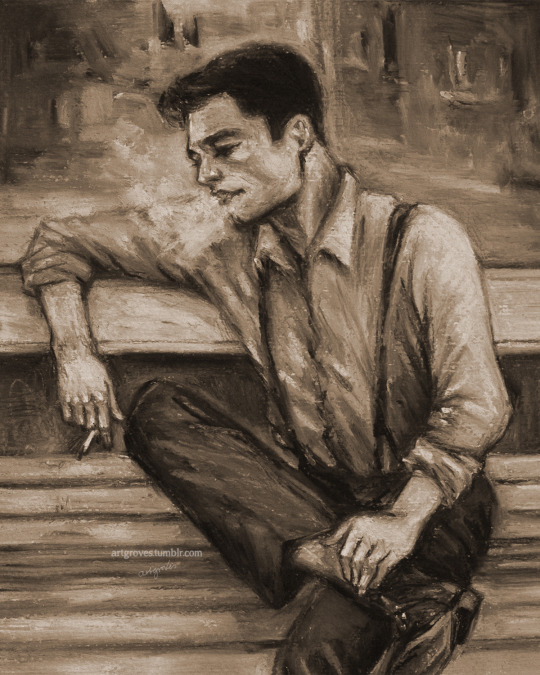

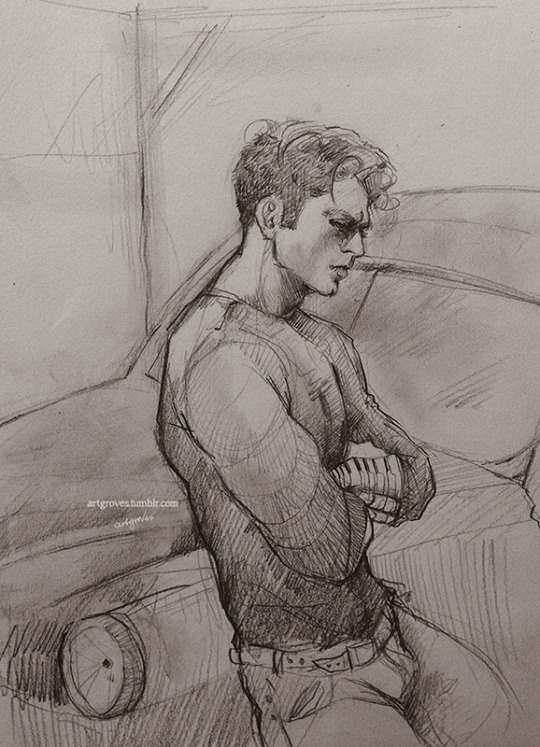

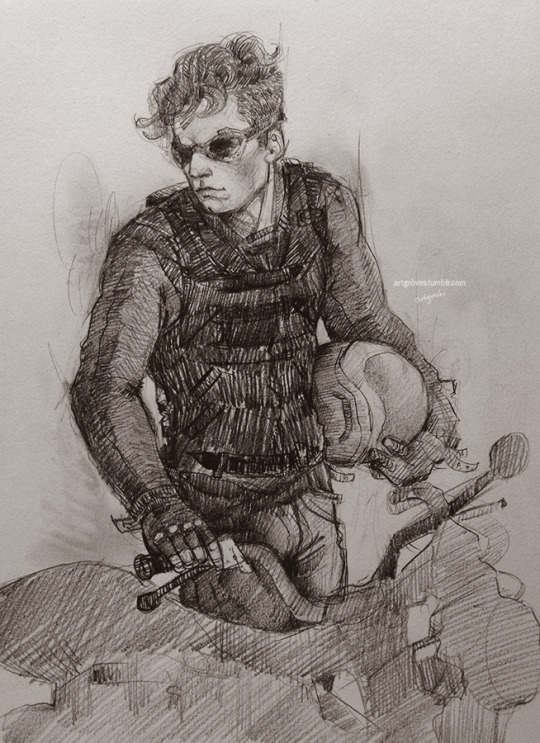
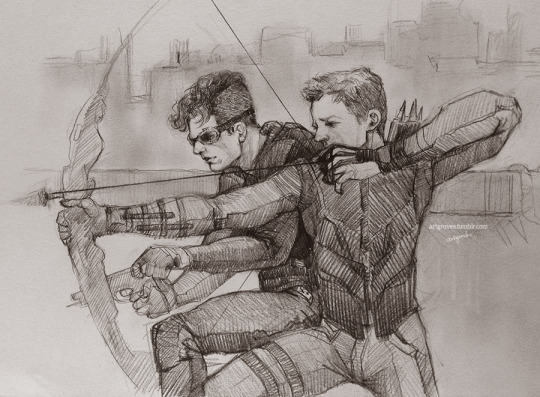
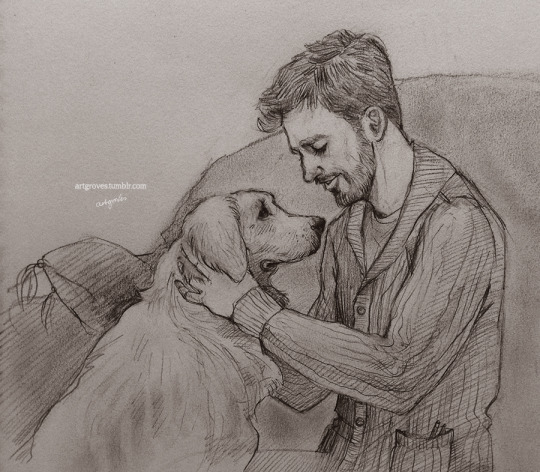

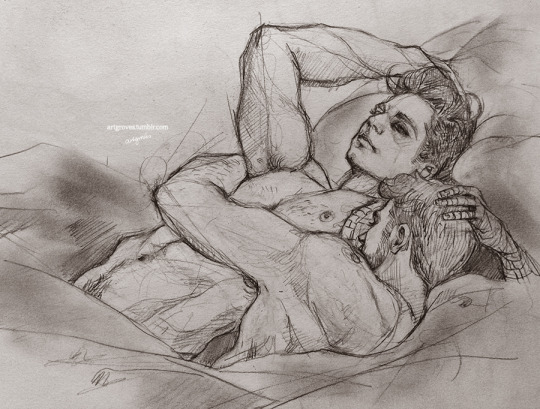
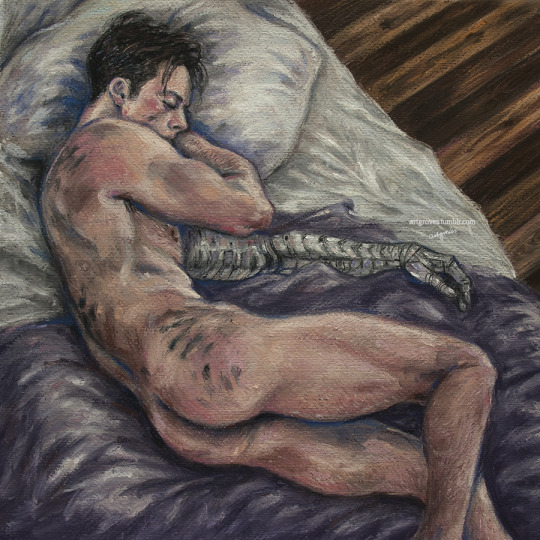




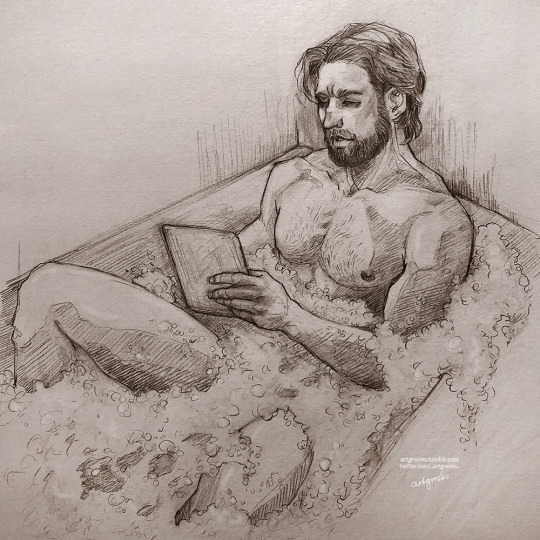
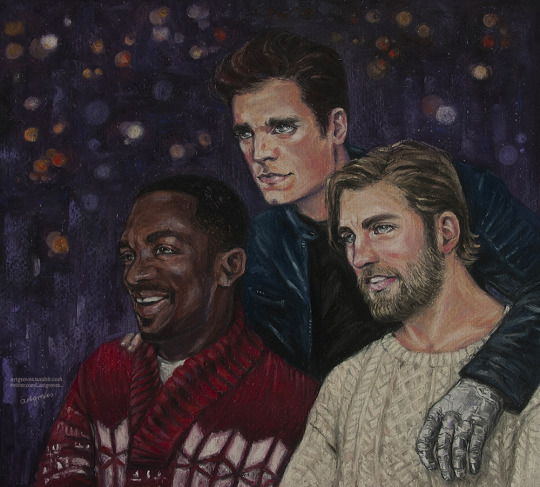


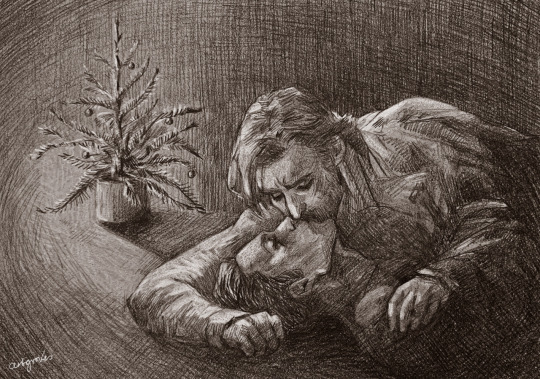
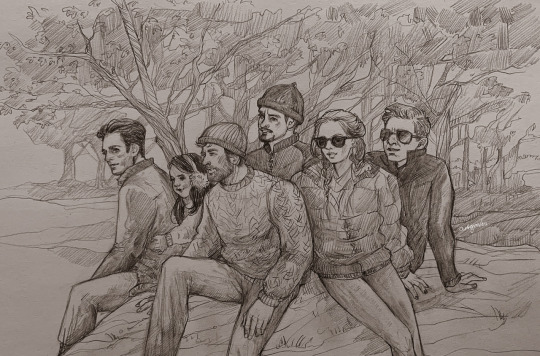

It's like Grand Central Station in here!
Masterpost of art for the 4 Minute Window series by @cesperanza
With accompanying podfic by @revolutionaryjo, miniature art by @melllacita and a host of additional pieces by other fans, this series has been a staple for so many of us over the past 10 years (the first fic was posted in January 2015).
Please indulge me in the feels nostalgia of a round-up of all the art I made for the series over the years and if you're so inclined, enjoy the wonderful stories all over again, and ring in the new year with a re-read.
Happy New Year, everyone!
#Stucky#4 Minute Window#my god that's a lot of scribbling#there are a number I couldn't post in full but if you want to see the uncensored arts - they are all embedded in the fic on AO3#Wishing you all a Happy New Year with the last post of the year - may it be healthy wealthy and wise and above all KIND to all of us
774 notes
·
View notes