#data driven modeling
Explore tagged Tumblr posts
Text
High Water Ahead: The New Normal of American Flood Risks
According to a map created by the National Oceanic and Atmospheric Administration (NOAA) that highlights ‘hazard zones’ in the U.S. for various flooding risks, including rising sea levels and tsunamis. Here’s a summary and analysis: Summary: The NOAA map identifies areas at risk of flooding from storm surges, tsunamis, high tide flooding, and sea level rise. Red areas on the map indicate more…
View On WordPress
#AI News#climate forecasts#data driven modeling#ethical AI#flood risk management#geospatial big data#News#noaa#sea level rise#uncertainty quantification
0 notes
Text
Scientists use generative AI to answer complex questions in physics
New Post has been published on https://thedigitalinsider.com/scientists-use-generative-ai-to-answer-complex-questions-in-physics/
Scientists use generative AI to answer complex questions in physics
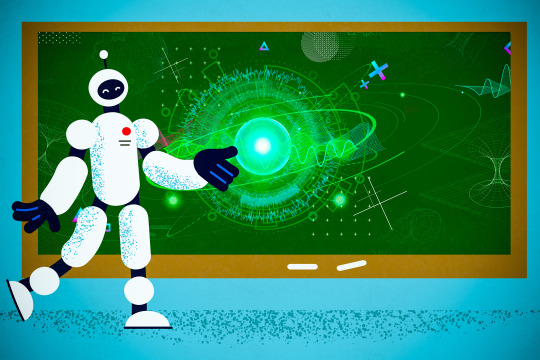
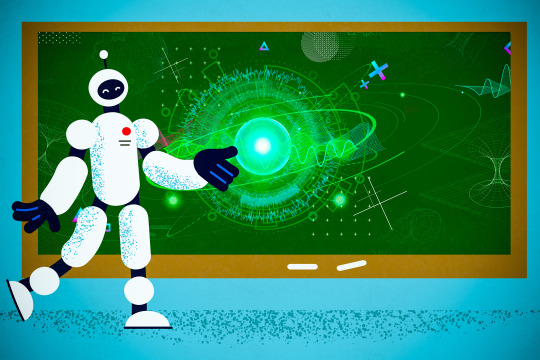
When water freezes, it transitions from a liquid phase to a solid phase, resulting in a drastic change in properties like density and volume. Phase transitions in water are so common most of us probably don’t even think about them, but phase transitions in novel materials or complex physical systems are an important area of study.
To fully understand these systems, scientists must be able to recognize phases and detect the transitions between. But how to quantify phase changes in an unknown system is often unclear, especially when data are scarce.
Researchers from MIT and the University of Basel in Switzerland applied generative artificial intelligence models to this problem, developing a new machine-learning framework that can automatically map out phase diagrams for novel physical systems.
Their physics-informed machine-learning approach is more efficient than laborious, manual techniques which rely on theoretical expertise. Importantly, because their approach leverages generative models, it does not require huge, labeled training datasets used in other machine-learning techniques.
Such a framework could help scientists investigate the thermodynamic properties of novel materials or detect entanglement in quantum systems, for instance. Ultimately, this technique could make it possible for scientists to discover unknown phases of matter autonomously.
“If you have a new system with fully unknown properties, how would you choose which observable quantity to study? The hope, at least with data-driven tools, is that you could scan large new systems in an automated way, and it will point you to important changes in the system. This might be a tool in the pipeline of automated scientific discovery of new, exotic properties of phases,” says Frank Schäfer, a postdoc in the Julia Lab in the Computer Science and Artificial Intelligence Laboratory (CSAIL) and co-author of a paper on this approach.
Joining Schäfer on the paper are first author Julian Arnold, a graduate student at the University of Basel; Alan Edelman, applied mathematics professor in the Department of Mathematics and leader of the Julia Lab; and senior author Christoph Bruder, professor in the Department of Physics at the University of Basel. The research is published today in Physical Review Letters.
Detecting phase transitions using AI
While water transitioning to ice might be among the most obvious examples of a phase change, more exotic phase changes, like when a material transitions from being a normal conductor to a superconductor, are of keen interest to scientists.
These transitions can be detected by identifying an “order parameter,” a quantity that is important and expected to change. For instance, water freezes and transitions to a solid phase (ice) when its temperature drops below 0 degrees Celsius. In this case, an appropriate order parameter could be defined in terms of the proportion of water molecules that are part of the crystalline lattice versus those that remain in a disordered state.
In the past, researchers have relied on physics expertise to build phase diagrams manually, drawing on theoretical understanding to know which order parameters are important. Not only is this tedious for complex systems, and perhaps impossible for unknown systems with new behaviors, but it also introduces human bias into the solution.
More recently, researchers have begun using machine learning to build discriminative classifiers that can solve this task by learning to classify a measurement statistic as coming from a particular phase of the physical system, the same way such models classify an image as a cat or dog.
The MIT researchers demonstrated how generative models can be used to solve this classification task much more efficiently, and in a physics-informed manner.
The Julia Programming Language, a popular language for scientific computing that is also used in MIT’s introductory linear algebra classes, offers many tools that make it invaluable for constructing such generative models, Schäfer adds.
Generative models, like those that underlie ChatGPT and Dall-E, typically work by estimating the probability distribution of some data, which they use to generate new data points that fit the distribution (such as new cat images that are similar to existing cat images).
However, when simulations of a physical system using tried-and-true scientific techniques are available, researchers get a model of its probability distribution for free. This distribution describes the measurement statistics of the physical system.
A more knowledgeable model
The MIT team’s insight is that this probability distribution also defines a generative model upon which a classifier can be constructed. They plug the generative model into standard statistical formulas to directly construct a classifier instead of learning it from samples, as was done with discriminative approaches.
“This is a really nice way of incorporating something you know about your physical system deep inside your machine-learning scheme. It goes far beyond just performing feature engineering on your data samples or simple inductive biases,” Schäfer says.
This generative classifier can determine what phase the system is in given some parameter, like temperature or pressure. And because the researchers directly approximate the probability distributions underlying measurements from the physical system, the classifier has system knowledge.
This enables their method to perform better than other machine-learning techniques. And because it can work automatically without the need for extensive training, their approach significantly enhances the computational efficiency of identifying phase transitions.
At the end of the day, similar to how one might ask ChatGPT to solve a math problem, the researchers can ask the generative classifier questions like “does this sample belong to phase I or phase II?” or “was this sample generated at high temperature or low temperature?”
Scientists could also use this approach to solve different binary classification tasks in physical systems, possibly to detect entanglement in quantum systems (Is the state entangled or not?) or determine whether theory A or B is best suited to solve a particular problem. They could also use this approach to better understand and improve large language models like ChatGPT by identifying how certain parameters should be tuned so the chatbot gives the best outputs.
In the future, the researchers also want to study theoretical guarantees regarding how many measurements they would need to effectively detect phase transitions and estimate the amount of computation that would require.
This work was funded, in part, by the Swiss National Science Foundation, the MIT-Switzerland Lockheed Martin Seed Fund, and MIT International Science and Technology Initiatives.
#ai#approach#artificial#Artificial Intelligence#Bias#binary#change#chatbot#chatGPT#classes#computation#computer#Computer modeling#Computer Science#Computer Science and Artificial Intelligence Laboratory (CSAIL)#Computer science and technology#computing#crystalline#dall-e#data#data-driven#datasets#dog#efficiency#Electrical Engineering&Computer Science (eecs)#engineering#Foundation#framework#Future#generative
2 notes
·
View notes
Text
Experience the convergence of human intuition and AI ingenuity in web development. Catapult your digital presence with an impeccable blend of creativity and technology.
#Challenges and opportunities of combining human intelligence with AI in web design#Implementing emotional intelligence in AI-driven web development#Fine-tuning AI models for natural language processing in web development#Customizing AI-driven web solutions for individual user preferences#The impact of human oversight on AI-generated web content#Empowering developers with AI-assisted human-centric web design tools#Adapting to evolving user expectations through AI-enhanced web development#Leveraging AI for data-driven insights in human-centered web design#Maintaining brand authenticity in AI-driven web development#Exploring the future of collaborative AI-human web development workflows
2 notes
·
View notes
Text
As someone who works deep in the weeds of corporate hellscape, the thing is... are you complaining?
Because if you're not complaining - ie, actually calling the phone number in the package and leaving a polite but strongly worded objection in their "feedback" channel - then... yeah, companies do think that you don't notice shrinkflation. It's not that the impacts it has are not real, it's that they're not reportable data.
One of the stupidest things about the corporate hell we live in today is that decisions are judged not by naturally observable things or even rational thought, but by data and metrics.
If someone says "we can make more money if we water down the soup", and someone tries to argue back against it by saying "customers will be mad and it will damage the brand", but after testing people don't complain or don't complain enough... yeah, as far as the corporation is concerned, people don't care.
And this is not me defending it, btw. I'm just trying to shine a light on a fucking horrid side effect of turbo capitalism: you're not allowed to exist, you have to spend time and energy constantly defending your right to receive the goods you're actually paying for, because someone else is trying to squeeze out a liiitle bit more profit out of it.
Like that's the ideal, as far as corporate goes: squeeze people and try to scam them as much as possible, until the complaining is unbearable. This is why you see stories blow up of people getting real fucking pissed and as you go down the facts, you realize they've been exploited and taken advantage of for years. Because that's what corporate considers sustainable.
"If people don't like it, they will complain."
We live in a system that expects you to complain about every aspect of your life, but if you don't do it in the correct channel or with the correct frequency, you will be assumed to be complicit in whatever new way they're looking to fuck you over. It's insane.
companies are delusional if they think consumers don't notice shrinkflation. less food in the package, less medicine in the jar, less whatever in the wherever, it doesn't matter where and it's almost always noticeable. like i just finished one box of medicine and we opened another allegedly identical one that we just bought and lo and behold, the four middle medicine segments were gone from the package. they took out four pills from the same sized box and sold it at the same price without any indication on the box other than the small number in the corner. ridiculous
#our business model revolves around pushing until we find the line our customers won't let us cross and in the meantime#profits!#shut up rie#“our business is data driven” is corpo speak for
78K notes
·
View notes
Text
Quantitative Analysis vs. Technical Chart Analysis in Cryptocurrency Markets
Cryptocurrency markets are notoriously volatile, and traders have long relied on technical chart analysis to make decisions. This method involves identifying patterns, using indicators like moving averages and RSI, and drawing trendlines to predict future price movements. However, as markets evolve, many traders are turning to quantitative analysis (quant analysis) for a more data-driven…
#automation in crypto trading#Bitcoin#chart analysis#crypto markets#crypto traders#cryptocurrency trading#data-driven models#MACD#quantitative analysis#RSI#statistical models#technical indicators#traditional analysis#trend lines
1 note
·
View note
Text
How Can Telecom Operators Turn Challenges Into Opportunities in a 5G-Driven World?
As technology speeds up, so do customer expectations, and 5G deployment, telecom operators are increasingly challenged to deliver smooth, high-quality services in the midst of increasingly complex networks. Intelligent networks in telecom—self-aware systems powered by AI and advanced analytics that adapt and optimize in real time—hold the key.
These intelligent networks are revolutionizing telecom service delivery through enhanced operational efficiency, superior customer experiences, and new revenue streams. Telecom providers can not only meet the demands of today but also future-proof their networks for a more connected world by integrating intelligence into their infrastructure.
The Shift Toward Data-Driven Business Models
The telecom operators are shifting their business models from the traditional voice and data usage revenue-generating models. They adopt more data-driven business models that rely on massive customer and network data-derived insights. This insight would help telecom providers understand and know the behavior, preferences, and usage patterns of their respective customers, thereby offering personalized services for each respective customer.
Network Data Analytics: Game Changer
Network data analytics has become a very important tool in managing and optimizing the growth of complexity in telecom networks. By analyzing real-time and historical network data, telecom operators can gain actionable insights that improve network performance, ensure reliability, and reduce operational costs.
Network data analytics is a prime area of research in fault detection and resolution. Telecom providers would be able to predict network outages or degradations that may impact their customers by utilizing machine learning algorithms to monitor performance metrics. This reduces downtime, and service quality would improve, giving users a smoother experience.
In addition, network data analytics provides resource allocation efficiently. Due to increasing requirements for bandwidth-hungry applications such as video streaming and online gaming, analytics can be applied by telecom operators to dynamically allocate resources where the most needed area exists, so customers will get proper performance, maximizing network capacity for their benefit.
The Rise of Intelligent Networks in Telecom
The integration of AI and machine learning in telecom infrastructure brought with it a whole new set of intelligent networks. These systems, self-aware, learn from real-time conditions and adapt according to their surroundings to produce remarkable efficiency and responsiveness.
A large application of intelligent networks is within 5G deployment. This is because with AI-driven automation, telecom operators can speed up the rollout of 5G infrastructure, save on operational costs, and guarantee quality. Through network slicing, several virtual networks are run on the same physical infrastructure. This provides telecom companies the opportunity to develop customized solutions to different industries; for example, low-latency services for autonomous vehicles or high-bandwidth connections for media streaming.
Another important role of intelligent networks is to enhance security. These systems can identify and mitigate cyber threats in real time by analyzing network patterns and detecting anomalies, thus safeguarding customer data and ensuring the integrity of the network.
Benefits for Telecom Providers and Customers
Data-driven business models, network data analytics, and intelligent networks are all interconnected and bring about a win-win situation for both telecom providers and their customers. The former enjoy better operational efficiency, lower costs, and the ability to scale innovation, while the latter get personalized services, greater reliability, and security.
Conclusion
The telecom industry is witnessing a sea change with the convergence of data-driven business models, network data analytics, and intelligent networks in telecom. This transformation is changing the way the telecom industry operates and also raising the bar on customer expectations and industry standards.
Brillio is a leader in intelligent networking solutions, which enables telecom providers to make the most of their data and AI power. This way, it always brings telecom companies at the top with such cutting-edge technologies that deliver new opportunities, serving them with exceptional services, and creating a more connected, intelligent future.
In this digital-transformation age, adopting new innovative strategies and technologies is not optional but inevitable. The future of telecom would focus on harnessing the potential of data and intelligence in developing networks that not only are faster, but smarter and more efficient with a focus on the customer.
#data driven decision making#intelligent network in telecom#data driven business models#intelligent networks#data driven decision making software#network data analytics#data driven strategies
0 notes
Text
Why ILRI's Kapiti Ranch in Kenya is the Ultimate Test-Bed for Digital Innovation in Livestock Research
“Learn how ILRI’s digital twin project at Kapiti Ranch is transforming livestock management in Africa, offering data-driven insights into cattle health, pasture availability, and climate adaptation.” “Explore ILRI’s innovative use of digital twin technology in Kenya, enhancing livestock research with real-time health monitoring, climate-resilient breeding, and sustainable rangeland…
#3D ranch modeling#African livestock management#Agricultural Innovation#agriculture digital tools#animal breeding research#animal phenotyping#Bodit Bluetooth collars#cattle health monitoring#CGIAR digital innovation#climate-resistant cattle#dairy farming technology#data-driven farming#digital twins in livestock#ILRI Kenya#Kapiti ranch Kenya#livestock research advancements#livestock technology#pasture monitoring#Smaxtech bolus sensors#sustainable rangeland management
0 notes
Text
The Future of Real Estate in Jamaica: AI, Big Data, and Cybersecurity Shaping Tomorrow’s Market
#AI Algorithms#AI Real Estate Assistants#AI-Powered Chatbots#Artificial Intelligence#Automated Valuation Models#Big Data Analytics#Blockchain in Real Estate#Business Intelligence#cloud computing#Compliance Regulations#Cyber Attacks Prevention#Cybersecurity#Data encryption#Data Privacy#Data Security#data-driven decision making#Digital Property Listings#Digital Transactions#Digital Transformation#Fraud Prevention#Identity Verification#Internet of Things (IoT)#Machine Learning#Network Security#predictive analytics#Privacy Protection#Property Management Software#Property Technology#Real Estate Market Trends#real estate technology
0 notes
Text
Machine Learning as a Service (MLaaS): Revolutionizing Data-Driven Decision Making
As businesses continue to generate vast amounts of data, the ability to leverage insights from that data has become a critical competitive advantage. Machine Learning as a Service (MLaaS) is an innovative cloud-based solution that allows companies to implement machine learning (ML) without the need for specialized knowledge or infrastructure. By making powerful ML tools and models accessible…
#Automation#business AI solutions#Cloud Services#Data-Driven Decision Making#Digital Transformation#Fiber Internet#Machine Learning as a Service#machine learning models#MLaaS#Predictive Analytics#scalable AI#SolveForce
0 notes
Text
The importance of predictive analytics in healthcare using big data can enhance patient care and address chronic diseases efficiently.
As someone deeply immersed in the healthcare industry, I’ve witnessed a profound transformation driven by the integration of predictive analytics and big data. The importance of predictive analytics in healthcare using big data cannot be overstated, as it offers unprecedented opportunities to improve patient care, optimize operations, and advance medical research. The vast amounts of data generated daily in healthcare settings provide the foundation for predictive analytics, enabling us to forecast future events based on historical and current data. In this blog, I’ll explore the significance of predictive analytics in healthcare, its benefits, practical applications, and the future of this technology.
#Predictive Analytics in Healthcare#Big Data in Healthcare#Healthcare Predictive Analytics#Predictive Analytics for Chronic Diseases#Patient Care Analytics#Big Data Analytics in Healthcare#Predictive Healthcare Analytics#Healthcare Data Analytics#Predictive Modeling in Healthcare#Data-Driven Healthcare Solutions
0 notes
Text
Generative AI for Startups: 5 Essential Boosts to Boost Your Business
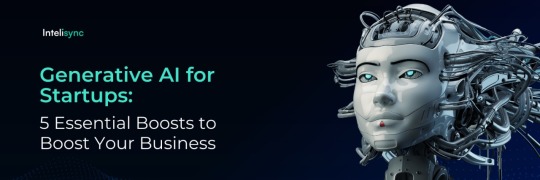
The future of business growth lies in the ability to innovate rapidly, deliver personalized customer experiences, and operate efficiently. Generative AI is at the forefront of this transformation, offering startups unparalleled opportunities for growth in 2024.
Generative AI is a game-changer for startups, significantly accelerating product development by quickly generating prototypes and innovative ideas. This enables startups to innovate faster, stay ahead of the competition, and bring new products to market more efficiently. The technology also allows for a high level of customization, helping startups create highly personalized products and solutions that meet specific customer needs. This enhances customer satisfaction and loyalty, giving startups a competitive edge in their respective industries.
By automating repetitive tasks and optimizing workflows, Generative AI improves operational efficiency, saving time and resources while minimizing human errors. This allows startups to focus on strategic initiatives that drive growth and profitability. Additionally, Generative AI’s ability to analyze large datasets provides startups with valuable insights for data-driven decision-making, ensuring that their actions are informed and impactful. This data-driven approach enhances marketing strategies, making them more effective and personalized.
Intelisync offers comprehensive AI/ML services that support startups in leveraging Generative AI for growth and innovation. With Intelisync’s expertise, startups can enhance product development, improve operational efficiency, and develop effective marketing strategies. Transform your business with the power of Generative AI—Contact Intelisync today and unlock your Learn more...
#5 Powerful Ways Generative AI Boosts Your Startup#advanced AI tools support startups#Driving Innovation and Growth#Enhancing Customer Experience#Forecasting Data Analysis and Decision-Making#Generative AI#Generative AI improves operational efficiency#How can a startup get started with Generative AI?#Is Generative AI suitable for all types of startups?#marketing strategies for startups#Streamlining Operations#Strengthen Product Development#Transform your business with AI-driven innovation#What is Generative AI#Customized AI Solutions#AI Development Services#Custom Generative AI Model Development.
0 notes
Text
Implementing Advanced Analytics in Real Estate: Using Machine Learning to Predict Market Shifts
New Post has been published on https://thedigitalinsider.com/implementing-advanced-analytics-in-real-estate-using-machine-learning-to-predict-market-shifts/
Implementing Advanced Analytics in Real Estate: Using Machine Learning to Predict Market Shifts


When it comes to the real estate industry, we have traditionally relied on local economic indicators, insights from personal networks, and comparisons of historical data to deliver market evaluations. Machine learning has disrupted many industries over the past few years, but the effects it has had in the real estate market fluctuation forecasting area have been nothing short of transformative. Drawing from my experience at Kalinka Group and Barnes International Moscow, I’ve witnessed how deep analytics has allowed us to explore massive datasets, uncover hidden patterns, and unlock predictive insights previously unimaginable. From 2025 onwards, machine learning will no longer be a utility but a strategic advantage in how real estate is approached.
The Basics of Predictive Analytics in Real Estate
Traditional real estate market analytics methods are being replaced by advanced algorithms capable of analyzing thousands of variables at once, such as property size, location, and comparable sales, which were the focus in the pre-machine learning era. Today’s variables, now addressed by machine learning, include everything from social media sentiment to infrastructure development plans, demographic shifts, neighborhood walkability scores, climate change impacts, and proximity to cultural hubs or transit lines.
For example, the team at Barnes International Moscow successfully predicted neighborhood appreciation rates using machine learning models, an accomplishment that was once unimaginable. These models synthesize data from a variety of sources, ranging from key local economic indicators to online consumer behavior, providing insights far beyond the scope of traditional human analysis.
Data Sources and Integration Challenges
Machine learning thrives on diverse qualitative data, requiring a strong data infrastructure to gather and integrate information from various sources. At Kalinka Group, we designed a roadmap for data acquisition, sourcing information from government property records, pricing trends, real-time listing platforms, and even social media sentiment to understand consumer preferences. We also utilized IoT sensors and smart home devices to measure real-time property performance metrics, enriching our forecasting models to capture everything from supply-demand dynamics to macroeconomic trends and demographic tracking.
Effective data integration is equally important. To ensure the highest degree of accuracy, we implemented rigorous validation checks, transforming raw data into actionable insights while avoiding the pitfalls of “garbage in, garbage out.”
Advanced Analytical Techniques
What excites me most about machine learning is its ability to uncover relationships that traditional statistical methods cannot identify. For instance, at Private Broker, machine learning models revealed how transit line openings influenced property values in nearby neighborhoods.
Other advanced techniques include:
These approaches provided highly accurate forecasts of market fluctuations, empowering clients to make informed investment decisions.
Practical Applications in Real Estate
Machine learning has transformed nearly every facet of real estate, offering tangible benefits to investors, developers, and property managers.
1. Predicting Market Trends
Machine learning models analyze historical data alongside external variables to forecast market conditions. For example, in a UAE-based project, we utilized ML to analyze economic data, migration patterns, and consumer preferences, uncovering a rising demand for high-end sustainable rental apartments. This insight allowed clients to capitalize on emerging opportunities.
2. Increasing Property Value
Traditional valuation methods relied on comparable sales and broker intuition. Machine learning incorporates hundreds of variables, enabling more accurate and nuanced valuations. At Kalinka Group, Automated Valuation Models (AVMs) provided transparency and speed, winning over clients with data-driven recommendations.
3. Optimizing Commercial Portfolios
Predictive analytics using machine learning forecasts vacancy rates, maintenance costs, and infrastructure impacts, guiding commercial property owners in decision-making. In a Thailand-focused project, machine learning models helped a multinational client rebalance their real estate portfolio, withdrawing from underperforming markets that previously appeared profitable on paper.
4. Identifying Emerging Markets
Machine learning identifies development hotspots by analyzing infrastructure projects, demographic trends, and local economic activities. These insights were pivotal in projects in Cyprus and Turkey, where we advised clients on confidently entering high-growth markets.
Ethical Considerations and Challenges
While machine learning offers immense potential, it also presents ethical and practical challenges.
Data Privacy
Real estate analytics often involves sensitive financial and personal data. At Kalinka Group, we implemented encryption and compliance measures to protect client data while enabling advanced analytics.
Algorithmic Bias
Machine learning models can exhibit bias, leading to discriminatory outcomes. To address this, Barnes International Moscow trained models on diverse datasets, ensuring fairness and transparency in predictive algorithms.
Conclusion
The future of machine learning in real estate is boundless. As quantum computing and more advanced AI models emerge, predictive accuracy will improve further. Real estate professionals are now equipped to anticipate market shifts with unparalleled precision, crafting bespoke strategies for each client and investment. Success will depend on strong data infrastructure, ethical practices, and the ability to combine technological knowledge with professional intuition, ensuring real estate professionals can navigate these transformative times with confidence.
#2025#acquisition#ai#AI models#Algorithms#Analysis#Analytics#applications#Artificial Intelligence#Behavior#Bias#Capture#change#climate#climate change#compliance#computing#consumer behavior#data#Data Integration#data sources#data-driven#datasets#developers#development#devices#dynamics#economic#effects#encryption
0 notes
Text
The AI Revolution: Transforming American Salespersons in the Trucking Industry
New blog alert!
Renee Williams, PresidentFreightRevCon, a Freight Revenue Consultants, LLC. company The trucking industry is experiencing a seismic shift, driven by the rapid adoption of artificial intelligence (AI) and advanced technologies. This transformation is reshaping the landscape for American salespersons, particularly in logistics and transportation jobs. As we delve into this evolution, we’ll explore…
#advanced technologies#AI#artificial intelligence#automated email response#C.H. Robinson#cash flow management#CO2E emissions tool#CRM systems#customer experience#data-driven decision making#dynamic pricing#electronic bill of lading#Freight#freight industry#Freight Revenue Consultants#future of trucking#green logistics#large language models#LLM#logistics#logistics technology.#Navisphere#Route Optimization#sales analytics#sales professionals#small carriers#Sustainability#technology adoption#touchless appointments#Transportation
1 note
·
View note
Text
How Big Data Analytics is Changing Scientific Discoveries
Introduction
In the contemporary world of the prevailing sciences and technologies, big data analytics becomes a powerful agent in such a way that scientific discoveries are being orchestrated. At Techtovio, we explore this renewed approach to reshaping research methodologies for better data interpretation and new insights into its hastening process. Read to continue
#CategoriesScience Explained#Tagsastronomy data analytics#big data analytics#big data automation#big data challenges#big data in healthcare#big data in science#big data privacy#climate data analysis#computational data processing#data analysis in research#data-driven science#environmental research#genomics big data#personalized medicine#predictive modeling in research#real-time scientific insights#scientific data integration#scientific discoveries#Technology#Science#business tech#Adobe cloud#Trends#Nvidia Drive#Analysis#Tech news#Science updates#Digital advancements#Tech trends
1 note
·
View note
Text
Creating an Effective Power BI Dashboard: A Comprehensive Guide
Introduction to Power BI Power BI is a suite of business analytics tools that allows you to connect to multiple data sources, transform data into actionable insights, and share those insights across your organization. With Power BI, you can create interactive dashboards and reports that provide a 360-degree view of your business.
Step-by-Step Guide to Creating a Power BI Dashboard
1. Data Import and Transformation The first step in creating a Power BI dashboard is importing your data. Power BI supports various data sources, including Excel, SQL Server, Azure, and more.
Steps to Import Data:
Open Power BI Desktop.
Click on Get Data in the Home ribbon.
Select your data source (e.g., Excel, SQL Server, etc.).
Load the data into Power BI.
Once the data is loaded, you may need to transform it to suit your reporting needs. Power BI provides Power Query Editor for data transformation.
Data Transformation:
Open Power Query Editor.
Apply necessary transformations such as filtering rows, adding columns, merging tables, etc.
Close and apply the changes.
2. Designing the Dashboard After preparing your data, the next step is to design your dashboard. Start by adding a new report and selecting the type of visualization you want to use.
Types of Visualizations:
Charts: Bar, Line, Pie, Area, etc.
Tables and Matrices: For detailed data representation.
Maps: Geographic data visualization.
Cards and Gauges: For key metrics and KPIs.
Slicers: For interactive data filtering.
Adding Visualizations:
Drag and drop fields from the Fields pane to the canvas.
Choose the appropriate visualization type from the Visualizations pane.
Customize the visual by adjusting properties such as colors, labels, and titles.
3. Enhancing the Dashboard with Interactivity Interactivity is one of the key features of Power BI dashboards. You can add slicers, drill-throughs, and bookmarks to make your dashboard more interactive and user-friendly.
Using Slicers:
Add a slicer visual to the canvas.
Drag a field to the slicer to allow users to filter data dynamically.
Drill-throughs:
Enable drill-through on visuals to allow users to navigate to detailed reports.
Set up drill-through pages by defining the fields that will trigger the drill-through.
Bookmarks:
Create bookmarks to capture the state of a report page.
Use bookmarks to toggle between different views of the data.
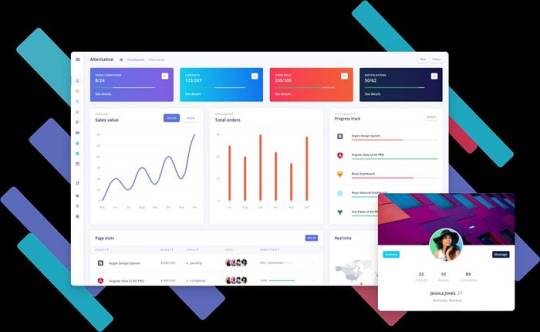
Different Styles of Power BI Dashboards Power BI dashboards can be styled to meet various business needs. Here are a few examples:
1. Executive Dashboard An executive dashboard provides a high-level overview of key business metrics. It typically includes:
KPI visuals for critical metrics.
Line charts for trend analysis.
Bar charts for categorical comparison.
Maps for geographic insights.
Example:
KPI cards for revenue, profit margin, and customer satisfaction.
A line chart showing monthly sales trends.
A bar chart comparing sales by region.
A map highlighting sales distribution across different states.
2. Sales Performance Dashboard A sales performance dashboard focuses on sales data, providing insights into sales trends, product performance, and sales team effectiveness.
Example:
A funnel chart showing the sales pipeline stages.
A bar chart displaying sales by product category.
A scatter plot highlighting the performance of sales representatives.
A table showing detailed sales transactions.
3. Financial Dashboard A financial dashboard offers a comprehensive view of the financial health of an organization. It includes:
Financial KPIs such as revenue, expenses, and profit.
Financial statements like income statement and balance sheet.
Trend charts for revenue and expenses.
Pie charts for expense distribution.
Example:
KPI cards for net income, operating expenses, and gross margin.
A line chart showing monthly revenue and expense trends.
A pie chart illustrating the breakdown of expenses.
A matrix displaying the income statement.
Best Practices for Designing Power BI Dashboards To ensure your Power BI dashboard is effective and user-friendly, follow these best practices:
Keep it Simple:
Avoid cluttering the dashboard with too many visuals.
Focus on the most important metrics and insights.
2. Use Consistent Design:
Maintain a consistent color scheme and font style.
Align visuals properly for a clean layout.
3. Ensure Data Accuracy:
Validate your data to ensure accuracy.
Regularly update the data to reflect the latest information.
4. Enhance Interactivity:
Use slicers and drill-throughs to provide a dynamic user experience.
Add tooltips to provide additional context.
5. Optimize Performance:
Use aggregations and data reduction techniques to improve performance.
Avoid using too many complex calculations.
Conclusion Creating a Power BI dashboard involves importing and transforming data, designing interactive visuals, and applying best practices to ensure clarity and effectiveness. By following the steps outlined in this guide, you can build dashboards that provide valuable insights and support data-driven decision-making in your organization. Power BI’s flexibility and range of visualizations make it an essential tool for any business looking to leverage its data effectively.
#Dynamic Data Visualization#Business Analytics#Interactive Dashboards#Data Insights#Data Transformation#KPI Metrics#Real-time Reporting#Data Connectivity#Trend Analysis#Visual Analytics#Performance Metrics#Data Modeling#Executive Dashboards#Sales Performance#Financial Reporting#Data Interactivity#Data-driven Decisions#Power Query#Custom Visuals#Data Integration
0 notes
Text
Learn how generative AI enhances data analytics, making visualization more effective and insights more powerful.
#Artificial Intelligence Systems#Generative Adversarial Networks#Transforming Data Visualization#Integrating AI-Driven Analytics#Advanced AI Techniques#AI-Driven Visualization Tools#Traditional AI Models#Generative AI Tools
0 notes