#contrastive learning
Explore tagged Tumblr posts
Text
Machine Learning Periodic Table: Unifying AI Algorithms
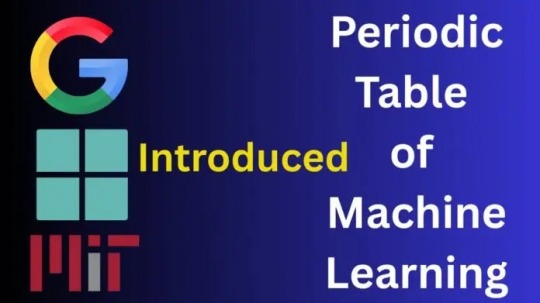
Researchers from MIT, Microsoft, and Google created Information Contrastive Learning (I-Con), the “machine learning periodic table,” to unite machine learning methodologies. The periodic table organises elements, whereas this table organises machine learning algorithms by learning data point correlations.
I-Con presents a single paradigm to show how classification, regression, big language modelling, clustering, dimensionality reduction, and spectral graph theory are all mathematically similar.
Machine learning periodic table importance
New machine learning algorithms and methods are being developed. To comprehend the essential ideas and links across methods. Benefits of the machine learning periodic table:
Unification: It shows how a single mathematical framework links numerous prominent machine learning approaches. Researchers and practitioners can benefit from understanding algorithm similarities.
The I-Con structure emphasises machine learning technique relationships, like the chemical periodic table defines element interactions. It organises the enormous array of algorithms into a clear style.
Discovery: Its ability to spark fresh discoveries is most exciting. Gaps in the chemical periodic table anticipated unknown elements. The Periodic Table of Machine Learning features “empty spaces” that imply undeveloped algorithms.
Innovation: I-Con helps researchers experiment, redefine “neighbors,” change connection confidence, and mix tactics from various algorithms to build new ways. It encourages creativity and the blending of previously unrelated methods.
Efficiency: This framework lets academics create new machine learning algorithms without “reinventing past ideas”. Understanding the ideas and algorithms in the table helps them strategically explore new methods.
How was the machine learning periodic table created?
The atomic table was an unplanned study outcome. Shaden Alshammari, an MIT Freeman Lab researcher, studied clustering, a technique to group related data elements. She connected contrastive learning with grouping. Contrastive machine learning compares positive and negative data.
Alshammari discovered that both techniques could be expressed by the same fundamental equation by studying their mathematics. Following this turning point, the Information Contrastive Learning (I-Con) paradigm was developed to illustrate that machine learning algorithms imitate real-world data linkages while minimising errors.
The researchers created a periodic table using these findings. The table distinguishes algorithms by two main factors:
Point relationships in actual datasets: Data linkages including visual likeness, shared class labels, and cluster membership are involved. These “connections” may not be 100% trustworthy.
The main ways algorithms approximate those connections: These relationships are acquired and reflected internally via algorithms.
By categorising various existing machine learning techniques inside this framework using these two criteria, the researchers found that many popular algorithms line neatly within defined “squares”. They also observed “gaps” where framework-logical algorithms have not yet been built.
How to Fill Gaps
This method helped researchers construct a current system for detecting photographs without human labelling. Combining debiased contrastive representation learning connection concepts with clustering approximation connections helped them “fill a gap” in their periodic table. This new method improved ImageNet-1K picture categorisation accuracy by 8%. They also found that contrastive learning data debiasing might increase clustering accuracy.
I-Con Learning
I-Con redefines machine learning as a tool for understanding complex data interactions. Consider a bustling party where data points, or visitors, meet at tables representing clusters and discuss shared hobbies or hometowns. Consider machine learning techniques as methods guests find friends and settle in.
I-Con simplifies real-world data point connections to make them easier to work with in algorithms. The concept of “connection” might entail appearing alike, sharing labels, or being in the same group. All algorithms try to close the gap between the connections they learn to imitate and the true connections in the training data.
Researchers Use the Periodic Table
The I-Con-based machine learning periodic table has various functions beyond organisation. This gives academics a toolkit for developing unique algorithms. When various machine learning approaches are defined in I-Con's conceptual language, experimenting with variants is easier:
Redefining neighbourhoods entails testing different ways to organise data points into “neighbours”.
Adjusting uncertainty requires varying trust in learnt connections. integrating strategies entails integrating approaches from different algorithms in unique ways.
Every modification might lead to a new periodic table entry. The table may easily be modified to include rows and columns to show more data points' relationships.
Looking Ahead
As artificial intelligence advances and its uses develop, frameworks like I-Con help us understand the area. They help researchers find hidden patterns and enable purposeful innovation. For non-AI professionals, it's a reminder that even in complex fields, basic patterns and structures are waiting to be identified.
Sorting algorithms by how they understand and estimate data point relationships is the basic notion. A full chart that lists all algorithms and their connection and approximation techniques would require more information than this. A basic table to illustrate the notion may look like this:
#technology#technews#govindhtech#news#technologynews#machine learning periodic table#machine learning#periodic table#Information Contrastive Learning#Contrastive Learning#machine learning algorithms
0 notes
Text





it got turned into a 17" x14" pillow instead haha
#rug hooking#artists on tumblr#fiber art#hnnnndgf the way the phone photo colors don't match up with how I see it is driving me a little nuts#every time but oh well >:'D#like the greens aren't as dull looking or as sharply contrasted in the detail photos#I'm glad I hoarded/saved the yarn that I precut for latchhook (which I abandoned after learning about rug hooking lmao)#because it makes for a very squishy pillow#(but also I think I def. could've stuffed in a litttle bit more before sewing it closed + didn't have a lot left)#blocked like...three other things when I was blocking this one#and the ones with non wool/funky materials were completely fine so that's nice to know haha#the back is an old flannel topsheet that nobody was using#anyways -knocks the fuck out-#edit: bb nephew recognized it as a cat so all is well/it passed hahah#also whoever said in tags that it looks great sensory wise to touch (or something like that my brain's a little fried still hhshs)#they are correct lMAO (even in general/ non pillow form I keep scrubbing my hands over them haha)#and when I was making the pillow I kept squishing it#muffled laugh
10K notes
·
View notes
Text
AI discovers that not every fingerprint is unique
0 notes
Text
drew some book!husbands. they feel like they've taken more traits from each other than the show.
#decided book!az has hints of elton john. its the old queen energy#book!crowley is nice looking average white man who learns how to wear a suit but half the reason he's interesting is bc he's trailing aroun#an old bookseller who looks maybe 30 years his senior and giving him babygirl eyes#its the stark contrast in the looks between the “bland” (cr) and “interesting” (az) that im going for with these guys#god im trying to make them not look like butch lesbians purely from an art skill pov but i cant beat the allegations#good omens#ineffable husbands#crowley#aziraphale
20K notes
·
View notes
Note
i think a lot about your mimic sometimes.... .... .








The way I draw the mimic as just the guy ever
#ask reply#guys this might be shocking but#I love the mimic#like a lot BAHA#the concept of them PLUS the story attached to them#dare I say peak#so I’m just letting y’all know now#as soon as SOTM drops imma be drawing this dude way more#it’s funny drawing the mimic casual and nonchalant#cause that dude is very much not chill BAHA#they are crazy violent but pairing that with them vibing#Hilarious always funny#I hope more people can learn to love the mimic#cause I think they can be as good of a villian as William#or at the very least a nice contrast to him#I love you mimic I won’t stop drawing you till everyone else likes you too
2K notes
·
View notes
Text
confessions of affection
chapter 4 of Like the Moon Haunts the Sun is out now!! we hope u enjoy the chapter <3 some... stuff happens lmao twirls hair cutely read from the beginning here ...or don't ...haha...unless? lmhs blog: @lmhsfic sam my love: @philosophiums
#my art#jujutsu kaisen#jjk#jjk fanart#jjk fanfic#jjk atla!au#atla!au: art#lmhs#atla!au: fic#yuji itadori#fushiguro megumi#nobara kugisaki#itafushi#lmao the contrast of th chapter title and the art is so funny#confessions of affection-FIRE#anyway dw abt it smile everything is surely fine smile#this angle choice came out of nowhere u know looking back through my old documents i think this might have been th catalyst#bc ive been playing around w angles a Lot more since i drew this#is it perfect no have any of them been perfect no but KARUCCHI APPEARANCE so it is special To Me!!!!!!!#this has been another instalment of hina refuses to learn csp perspective tool so don't look too close#READ TH CHAPTER THO READ IT I AM HOLDING U AT GUNPOINT PLS
494 notes
·
View notes
Text
50+ deaths at 5 am got me yelling absolute nonsense to the bosses kicking my whole entire ass
#ultrakill#v1#gabriel#doodles#art#ultrakill fanart#fastest death was like. 6 seconds. maybe less. i was playing on normal#and then my brother told me the secrets of Spamming Slide Like Your Life Depends On It and i got him in like. 15 tries#max0r wasnt kidding this guys entire strat is Teleporting Behind You#so the contrast of nearly killing him first try and then beating his stupid gay ass real fast in his second fight was REALLY funny#i learned bitch#i dont think i've ever yapped and screamed and yelled so much during a videogame before. dont ask me about the noise i made when#the mannequins started moving like coked up little spiders#''i dont believe people are genuinely this loud when playing lethal company they're making this up'' me playing ultrakill:
857 notes
·
View notes
Text
This season has been absolutely full of rabbits. Akilah cradling rabbits; rabbits surrounding a doomed Ben; rabbits slaughtered repeatedly onscreen. We know there’s a strong symbolic link between Jackie and rabbits in Yellowjackets, but an important distinction is that they don’t represent Jackie as a person (she didn’t even like rabbits!) so much as the idea of Jackie, something ascribed to her against her will: a warped, disingenuous version of her.
It also doesn’t escape my notice that in the very same episode that Kodiak shoots a rabbit through the heart with his crossbow he also shoots Melissa in the chest. Melissa, who Shauna uses to fill a Jackie-shaped hole inside her. Who is pinned down with a knife, hyperventilating like a cornered prey animal when she first gets with Shauna. Who approaches Shauna tentatively, timidly. Who lays flowers on Shauna’s son’s grave and gifts her a handmade sheath wrapped in bark and berries. Who is fearful yet exhilarated when encouraged to embrace her own darkness.
There’s a strange innocence to her interactions with Shauna, but it’s also the death of innocence; the relinquishment of innocence; innocence taken advantage of. When she’s struck with the arrow she cries out to Shauna, but Shauna is like a wolf hot on the hunt, and she can’t offer Melissa the unconditional love she craves and gives freely.
All of which makes me wonder: who is the rabbit?
#yellowjackets#yellowjackets spoilers#melissa yellowjackets#shauna shipman#shaunahat#jackie taylor#jackieshauna#I think the answer is both and neither#Jackie and Melissa are so tethered and I love how they reflect and contrast one another#Shauna is also the rabbit in her own way#and she’s going to learn that everything with teeth will bite back#mine#meta#yellowjackets meta
386 notes
·
View notes
Text
Mirror Twins
#emmet#ingo#submas#pokemon#pla#apparently there was an anniversary- happy not-so-happy 3 years in hisui ingo! hope you find the man that looks like you!#anyway i had an idea to draw a mirror meeting and thought 'that's way above my skill level' especially with pov but!#that's how you fucking learn so i learnt how to do a kinda reflection (mostly the hand which you can't even see)#but I KNOW IT'S THERE so it counts#partway through drawing this with weird layer visibility (intentionally since i wanted to sample stuff from layers i wanted to hide after)#emmet's glove looked like his sleeve and so now i have in my head he wears short sleeves#alongside the fact that i did the fandom thing and made his shirt colour black#to contrast ingo who would've had long sleeves#something about ingo doing bad with the cold (very horrible for hisui) and emmet doing bad with the heat (maybe not the best when overworke
290 notes
·
View notes
Text

2024/25 winter outfit practice ❄️🧣
#mollie#orla#pokemon horizons#pokeani#anipoke#drawn with references because I suck at drawing different material thickness#it’s also a good way of learning silhouettes with size contrast#I tend to draw clothing too close to the body#pokemon#mollie pokemon#pokemon orla#pokemon mollie
202 notes
·
View notes
Text

(I put a version with no gold frame under the cut because unfortunately I think it looks better without the frame)

#not entirely happy with the gold frame and I'm probably so tired there's something super important missing#buuuut it's only juneteenth for two more hours here and I'm tired and I've got work tomorrow so. we're just posting#strongly debating whether I want to do ten of these for each lesson he learned . . .#httyd#httyd books#book hiccup#books hiccup#httyd hiccup#hiccup#hiccup horrendous haddock the third#hiccup horrendous haddock iii#juneteenth#my art#I will say though that except for the frame I'm SUPER happy with the colors on this one#also the. the dilemma of how to make hiccup look kingy without making him look ridiculous#and also while keeping it like#as historically accurate and realistic as httyd ever is#mostly just like he wouldn't bedeck himself with the things all the time and would not dress that expensively because he's HICCUP#but he needed to be a little bedecked in this one for the contrast. dilemma . . .#anyway I totally drew the sword and arrow too but they got covered by the divider in the middle :sobs: so what can you do#whatever no one except me is going to notice#highlights reel
124 notes
·
View notes
Note
I've been researching and I cannot for the life of me figure out how to replicate the animation for the show. I know the fps and stuff but for some reason it isn't registering in my mind when I draw. I'm probably being dumb but how do you do it? (Also I apologize for consistently asking u stuff you are just a big inspiration😅)
Pretty much a lot of patience and practice. 2-3 years later I’m still learning how to do the art style because without a style guide all I can do is observe and pray.
the same draft re-animated 2 years later :1 Just a whole lot of patience and practice.
#I know I’m not really much help but all my learning has been is drawing and letting time run its course.#it’s not an overnight thing cos you can see the stark contrast even here. lol#this shot rotted in my drive for 2 years and then I did it in one day lmao#ramble
113 notes
·
View notes
Text
some people may see this cassian/bix content as an obstacle for rebelcaptain... but as a rebelcaptain truther i only see more jyncass material to work with 😌
#andor series#andor spoilers#rogue one#rebelcaptain#they gonna break up again alsdkjf#or if they don't it literally won't last sorry#but the contrast of how little he trusts her to do things on her own/ be healthy and stable enough for work#with how he never has to really worry about jyn at all#not necessarily bc jyn is more competent but more like. he has grown and learned how to have Faith™#her faith carried him with her etc etc#idk i'm not very articulate rn#and otherwise we get to see what it's like to date rebel spy cas 😌#and i get to cherry pick 😌
82 notes
·
View notes
Text
Thinking about the parallels between Ed’s and Mary’s violence. Stede hardly even questions Mary’s attempt to kill him, he’s mostly outraged at the method. The story punishes violence stemming from racism or enforcement of harmful social norms, but Mary’s violence to ensure she can continue to live a life where she can be herself is completely unpunished. Instead it’s an open action of self protection and expression that leads to understanding and love and a better outcome for everyone. She’s rewarded! She gets to end the marriage while also reconciling with Stede and knowing that they will both be free and happy. Also she gets Doug and the money (free real estate!) What more do we need to reinforce the message that it’s not violence that’s the problem, it’s why you use it
Ed’s violence against Izzy was for freedom too, but even more justified because it wasn’t just freedom from an unsuitable and stifling marriage, it was freedom from targeted abuse and control
Mary’s skewer more than validates Ed’s leg shot and I will die on this hill
#ed did nothing wrong#if anything part of his journey is to learn that sometimes violence is justified and worth it#and the contrast between mary’s violence for freedom and izzy’s violence of control#aaargh
181 notes
·
View notes
Text
Shadow
#ok this is all i have for this week unless i get super art cracked tonight and managed to draw some shit#shadow the hedgehog#shadow#sonic fanart#sth fanart#mono art#illustration#i need to learn how to do more contrast damn...
2K notes
·
View notes
Text

Hollow No More.
#beep boop you want fries with that#kingdom hearts#namine#kairi#sorry im back on my mythological presense of namine bullshit again#ive just been rotating so many thoughts about her and also kairi interacting with her and i love the breif moment they have together in kh2#GGGHHG. i also love how namine also has this light and darkness duality thing going on because even though she has this whole white motif#she uses darkness a lot and literally helped riku learn that it isn’t inherently bad. also have you ever thouht abt the narrative parallels#(or contrasts?? sorry im tired im probably using words wrong.)#where diz uses darkness to appear as someone riku fears to make him hate darkness#while namine uses darkness (presumably) to appear as someone riku loves to make him understand darkness???#(yeah i think its called narrative contrasts. whoopsies.)#Yuuuup. Its pretty fucking insane.#also caption uses hollow on purpose. get it?? becau
88 notes
·
View notes