#cloudsql
Explore tagged Tumblr posts
Text
Database Center, Your AI-powered Fleet Management Platform
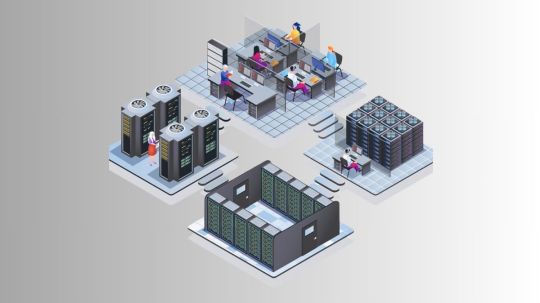
Businesses are battling an explosion of operational data dispersed over a database environment that is ever more sophisticated and varied. Their capacity to extract insightful information and provide outstanding client experiences is hampered by this complexity, which frequently leads to expensive outages, performance bottlenecks, security flaws, and compliance gaps. Google Cloud unveiled Database Center, an AI-powered, unified fleet management system, earlier this year to assist companies in overcoming these obstacles.
Many clients are using Database Center at an increased rate. For instance, Ford proactively reduces possible threats to their applications and uses Database Center to obtain answers on the health of their database fleet in a matter of seconds. Database Center is now accessible to all of Google’s clients, giving you the ability to manage and keep an eye on database fleets at scale using a single, cohesive solution. You can now manage Spanner alongside your Cloud SQL and AlloyDB deployments with its addition of support, and more databases will soon follow.
Database Center is intended to harness the full potential of your data and restore order to the disarray of your database fleet. It offers a unified, user-friendly interface where you can:
Get a thorough picture of your whole database fleet. No more searching through spreadsheets and specialized tools or information silos.
De-risk your fleet proactively with wise performance and security suggestions. With data-driven recommendations, Database Center helps you keep ahead of any issues by offering actionable insights that boost security, lower expenses, and improve performance.
Make the most of your database fleet with help from AI. Ask questions, get optimization advice, and swiftly handle fleet concerns using a natural language chat interface.
Now let’s take a closer look at each.
Take a thorough look at your database fleet
Are you sick of switching between consoles and tools to manage your databases?
With a single, cohesive view of your whole database landscape, Database Center streamlines database administration. Throughout your entire company, you may keep an eye on database resources across various engines, versions, locations, projects, and environments (or applications using labels).
Database Center is fully connected with Cloud SQL, AlloyDB, and now Spanner, allowing you to keep an eye on your inventory and proactively identify problems. With Database Center’s unified inventory view, you can:
Determine which database versions are outdated to guarantee appropriate support and dependability.
Monitor version updates, such as if PostgreSQL 14 to PostgreSQL 15 is happening at the anticipated rate.
Make that database resources are allocated properly; for example, determine how many databases support non-essential development and test environments versus vital production applications.
Track database migrations between engines or from on-premises to the cloud.
Use suggestions to proactively de-risk your fleet
Navigating through a complicated fusion of security postures, data protection settings, resource configurations, performance tweaking, and cost optimizations can be necessary when managing the health of your database fleet at scale. Database Center proactively identifies problems related to certain configurations and helps you fix them.
For instance, a Cloud SQL instance with a large transaction ID may stop accepting new queries, which could result in latency problems or even outages. Database Center identifies this proactively, gives you a thorough explanation, and guides you through the recommended procedures to troubleshoot the problem.
It can help you with a basic optimization trip and has introduced several performance recommendations to the Database Center that are related to too many tables/joins, connections, or logs.
By automatically identifying and reporting violations of a variety of industry standards, including as CIS, PCI-DSS, SOC2, and HIPAA, Database Center also makes compliance management easier. Database Center keeps an eye on your databases to look for possible infractions of the law. When a violation is found, you are given a detailed description of the issue, which includes:
The particular security or dependability problem that led to the infraction
Practical measures to assist in resolving the problem and regaining compliance
This improves your security posture, streamlines compliance checks, and lowers the possibility of expensive fines. Real-time detection of unwanted access, modifications, and data exports is now supported by Database Center as well.
Maximize your fleet with help from AI
Database Center makes optimizing your database fleet very simple when Gemini is enabled. To get accurate responses, identify problems in your database fleet, troubleshoot issues, and swiftly implement fixes, just talk with the AI-powered interface. For instance, you can easily find instances in your entire fleet that are under-provisioned, get actionable insights like how long high CPU/memory utilization conditions last, get suggestions for the best CPU/memory configurations, and find out how much those changes will cost.
The Database Center’s AI-powered conversation offers thorough information and suggestions on every facet of database administration, including availability, performance, inventory, and data security. Furthermore, enhanced security and compliance advice help improve your security and compliance posture, and AI-powered cost recommendations offer strategies for maximizing your expenditure.
Start using Database Center right now
All customers can preview the new Database Center features for Spanner, Cloud SQL, and AlloyDB today. To monitor and manage your whole database fleet, just navigate to Database Center in the Google Cloud dashboard.
Read more on Govindhtech.com
#DatabaseCenter#AI#CloudSQL#PostgreSQL#fleet#Spanner#AlloyDB#FleetManagement#News#Technews#Technology#Technologynews#Technologytrends#govindhtech
0 notes
Text
Day 016/017 - running
Today was a bit of a late day for me, I was working the whole day and I've been walking around campus, back and forth to the point where my legs are sore. It was exhausting, I finally got to enjoy a dream where I Work with a team and eat pizza with them.
We implemented the notifications for the app, it was awesome to see that realtime feature doing its work! So, in the video you'll notice a new item being added to the list. Well two services are involved in talking, the events service which is responsible for inviting a user and the notification service which is responsible for both push notifications and showing holding notifications as well. All thanks to RabbitMQ, Firebase and NodeJS! I suppose Angular as well because of how its binding works.
I'm going to finish the posts service today, I still want to make to combine the posts and other stuff.
Also we finally deployed our MySQL databases using google's CloudSQL. I'm so happy about that, we're using. Hopefully not too much money was taken from the free credits.
Well I'm still very sleepy and wanted to write more but later! I basically missed yesterday so I hope you'll forgive me on that!
Personally, I don't know how I'm feeling? I am just feeling content with everything you know, yeah I still get anxious and paranoid but normal. The only things on my mind these days is finishing the app and trying to keep up with my studies.
I'll try and make time during the break between classes to organize my notes, alright lets do this! Going back to sleep!
0 notes
Text
GCP CloudSQL Vulnerability Leads to Internal Container Access and Data Exposure
https://www.dig.security/post/gcp-cloudsql-vulnerability-leads-to-internal-container-access-and-data-exposure Comments
0 notes
Text
Google Cloud Patches Vulnerability In CloudSQL Service
http://i.securitythinkingcap.com/SpgPC2
0 notes
Video
youtube
Deploy V lang CRUD REST API with PostgreSQL on GCP Cloud Run
#youtube#gcp googlecloudplatform googlecloud cloudrun vlang vprogramming vweb buildtrigger devops cloudbuild cloudsql postgresql#gcp#googlecloudplatform#googlecloud#cloudrun#vlang#vprogramming#vweb#buildtrigger#devops#cloudbuild#cloudsql#postgresql
1 note
·
View note
Photo
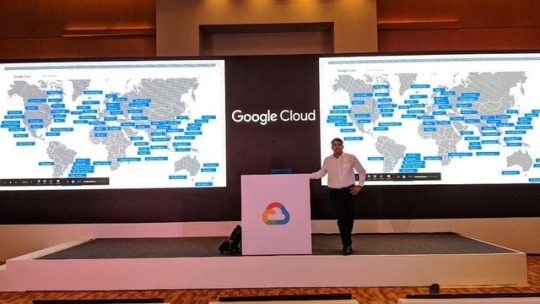
Google Cloud OnBoard Pune 2018 #GoogleCloudOnBoard #google #googlecloud #googlecloudplatform #machinelearning #pubsub #cloudsql #spanner (at WESTIN Hotel Pune) https://www.instagram.com/p/BqucmmgHXw9/?utm_source=ig_tumblr_share&igshid=1uyrpkfpkjdu5
0 notes
Photo
Google Cloud launches new Data Migration Service
#GoogleCloud has released its new Data Migration Service (DMS), with the intention of making it easier for enterprise customers to move production databases to the cloud. Currently available in Preview, customers can migrate MySQL, PostgreSQL, and SQL Server databases to Cloud SQL from on-premises environments or other clouds. Customers can start migrating with DMS at no additional charge for native like-to-like migrations to #CloudSQL. Support for #PostgreSQL is currently available for limited customers in Preview, with SQL Server coming soon.
Source: https://bit.ly/3amC5WU
1 note
·
View note
Text
Once you are in the Cloud, the opportunities for automation are endless. It is no longer viable to create resources and managing them manually because it does not scale once there are more users and the requests for cloud resources increase. The only way to reduce the work and to make the same as easy and as scalable as possible is to leverage on tools that will lighten this burden. Having said that, this is a showcase of how you can be able to reduce the work involved in giving users access to databases in CloudSQL. After much thought and deliberations, the tool that won the tender to accomplish the reduction of the boring exertion involved in granting users database access manually is terraform. This is a guide that elucidates how it was done: In this setup, we will be using three providers: Vault: Found on this link. Google Cloud: On this Link cyrilgdn/postgresql: You can get this one on this link. Also note that we will be needing the following before everything works Google Cloud Account with CloudSQL Instances Vault Server up and running Vault will be storing secrets for the “cyrilgdn/postgresql” provider. Cyrilgdn/postgresql will handle granting of rights to IAM roles which will be created by the Google provider. The following steps explain how the entire project was created and tested. Step 1: Prepare your CloudSQL Modules Since you may be having multiple instances in CloudSQL zones, it makes sense to organise them into separate modules so that it becomes easier and clearer to add new records in future as well as provide a good representation of what lies in your environment. We will be consolidating our CloudSQL Terraform files into the following folders. cd ~ mkdir -p databases/tf_cloudsql/modules cd databases/tf_cloudsql/modules mkdir geekssales-db-production,geeksfinance-db-production,geeksusers-db-production Inside each database module, we will create sub-modules that will accommodate every department such as “Engineering”, “Finance”, Sales Service“ and so forth. Finally, we should have something like this with “engineering” sub-module added. $ tree -d └── tf_cloudsql └── modules ├── geekssales-db-production │ └── engineering ├── geeksfinance-db-production │ └── engineering ├── geeksusers-db-production │ └── engineering Step 2: Adding Fresh Condiments In database_connect directory which is the root directory, create a ”main.tf” file and populate it as follows: ######################################################################## provider "vault" token = var.geeks_token alias = "geeks_vault" address = var.geeks_vault_address provider "google" project = var.project_id region = var.region alias = "geeks_gcp" #1. geekssales-db-production instance Module module "geekssales-db-production" source = "./modules/geekssales-db-production" providers = vault = vault.geeks_vault google = google.geeks_gcp #2. geeksfinance-db-production instance Module module "geeksfinance-db-production" source = "./modules/geeksfinance-db-production" providers = vault = vault.geeks_vault google = google.geeks_gcp #3. geeksusers-db-production instance Module module "geeksusers-db-production" source = "./modules/geeksusers-db-production" providers = vault = vault.geeks_vault google = google.geeks_gcp ## This is the bucket where tfstate for CloudSQL will be stored in GCP terraform backend "gcs" bucket = "geeks-terraform-state-bucket" prefix = "terraform/cloudsql_roles_state" Here we have two providers added for Vault and for Google and all of the modules we want. The reason why we didn’t include postgresql is because it does not work well with aliases like we have done for the other two. So we will have to add it in each of the modules as we will see later.
Note also that we are using GCP Bucket backend to store terraform’s state. Ensure that a bucket with the name given already exists or you can create it if it is not there yet. You will find the state in a the “terraform” sub-directory called “cloudsql_roles_state” Create a ”vars.tf” file in the same directory and fill ip us as follows: $ vim vars.tf variable "geeks_token" type = string default = "s.6fwP0qPixIajVlD3b0oH6bhy" description = “Dummy Token” variable "geeks_vault_address" type = string default = "//vault.computingforgeeks.com:8200" variable "project_id" type = string default = "computingforgeeks" variable "region" type = string default = "us-central1" The variables declared in “vars.tf” are the credentials and details that Terraform will use to connect to Vault and perform its cool operations we desire. After we have completed that, in each module, create another ”main.tf” file that has this configuration: $ cd databases/tf_cloudsql/modules/geekssales-db-production $ vim main.tf ######################################################################## #Engineering SubModule ######################################################################## terraform required_providers postgresql = source = "cyrilgdn/postgresql" version = "1.15.0" vault = source = "vault" version = "~> 3.0" google = source = "hashicorp/google" version = "4.13.0" #1. Engineering Files SubModule module "engineering" source = "./engineering" This is another file that calls the sub-modules within each module. As you can see, we are calling “engineering” sub-module and other sub-modules can be added here such as the “Finance”, Sales Service“ and so forth. Step 3: Create files we need for the sub-modules Once the “main.tf” file in each module is well done, let us now get into the “engineering” sub-module and create the files of interest here. First, we shall add the “postgresql” provider that if you remember we mentioned that does not work well with aliases and we shall also add vault connection for “postgresql” provider secrets retrieval. The secret here is a user that can connect to the CloudSQL databases and hence be able to grant permissions to other users we will be creating. So we should already have the username and password for this user created in Vault within the “cloudsql/credentials” path. This is what the (data “vault_generic_secret” “cloudsql”) will be fetching from. Once the “main.tf” file in each module is well done, we shall proceed to create the files of interest for each sub-module. Let us begin with “engineering”. $ cd databases/tf_cloudsql/modules/geekssales-db-production/engineering $ vim provider.tf data "vault_generic_secret" "cloudsql" path = "cloudsql/credentials" provider "postgresql" scheme = "gcppostgres" host = "computingforgeeks:us-central1:geekssales-db-production" # The CloudSQL Instance port = 5432 username = data.vault_generic_secret.cloudsql.data["apps_user"] # Username from Vault password = data.vault_generic_secret.cloudsql.data["apps_pass"] # Password from Vault superuser = false sslmode = "disable" terraform required_providers postgresql = source = "cyrilgdn/postgresql" version = "1.15.0" vault = source = "vault" version = "~> 3.0" google = source = "hashicorp/google" version = "4.13.0" Next, we shall create a “roles.tf” file that will hold the new users we will be creating. We will be using the Google Provider resources here so we will have to specify the instances we are connecting to explicitly. Proceed to create them as shown below within the “engineering” directory: $ cd databases/tf_cloudsql/modules/geekssales-db-production/engineering
$ vim roles.tf ###################################################### # Engineering Block ###################################################### ## Junior Engineering Team Members IAM DB Roles Creation resource "google_sql_user" "jnr_eng_member_roles" count = length(var.jnr_eng_member_roles) name = var.jnr_eng_member_roles[count.index] instance = "geekssales-db-production" type = "CLOUD_IAM_USER" ## Engineering Team Members IAM DB Roles Creation resource "google_sql_user" "eng_member_roles" count = length(var.eng_member_roles) name = var.eng_member_roles[count.index] instance = "geekssales-db-production" type = "CLOUD_IAM_USER" ## Senior Engineering Team Members IAM DB Roles Creation resource "google_sql_user" "snr_eng_member_roles" count = length(var.snr_eng_member_roles) name = var.snr_eng_member_roles[count.index] instance = "geekssales-db-production" type = "CLOUD_IAM_USER" You will immediately notice that we have separated the users into junior, senior and mid-level engineers. In conjunction with this, we will have to create a variable file that will hold the names of these users and in their respective categories. We will loop through each of the users in the variable that will be a list as we create them and we we grant them database privileges. Create a variable file and add the meat as shown below for better clarity: $ vim vars.tf ###################################################### # Engineering Block ###################################################### ## Junior Engineering Member Roles variable "jnr_eng_member_roles" description = "CloudSQL IAM Users for Engineering Members" type = list(string) default = [] ## Mid-level Engineering Member Roles variable "eng_member_roles" description = "CloudSQL IAM Users for Engineering Members" type = list(string) default = ["[email protected]", "[email protected]"] ## Senior Engineering Member Roles variable "snr_eng_member_roles" description = "CloudSQL IAM Users for Engineering Members" type = list(string) default = ["[email protected]", "[email protected]"] Step 4: Let us create the privileges file Within the same “engineering” directory, let us create a privileges files for each of the categories (senior, junior and mid-level) that will be granting users specific privies for specific databases. The junior’s file looks like below (truncated). $ vim privileges-jnr-eng.tf ###################################################### # Junior Engineering DB Privileges File ###################################################### ## Finance Database resource "postgresql_grant" "grant_jnr_eng_db_privileges_on_courier_db" count = length(var.jnr_eng_member_roles) #for_each = toset( ["table", "sequence"] ) database = "finance" role = var.jnr_eng_member_roles[count.index] schema = "public" object_type = "table" objects = [] privileges = ["SELECT"] depends_on = [ google_sql_user.jnr_eng_member_roles, ] ## Users Database resource "postgresql_grant" "grant_jnr_eng_db_privileges_on_datasync_db" count = length(var.jnr_eng_member_roles) database = "users" role = var.jnr_eng_member_roles[count.index] schema = "public" object_type = "table" objects = [] privileges = ["SELECT"] depends_on = [ google_sql_user.jnr_eng_member_roles, ] ## Sales Database resource "postgresql_grant" "grant_jnr_eng_db_privileges_on_dispatch_db" count = length(var.jnr_eng_member_roles) database = "sales" role = var.jnr_eng_member_roles[count.index] schema = "public" object_type = "table" objects = [] privileges = ["SELECT"] depends_on = [ google_sql_user.jnr_eng_member_roles, ] The senior’s file looks like below (truncated).
$ vim privileges-snr-eng.tf ###################################################### # Senior Engineering DB Privileges File ###################################################### ## Finance Database resource "postgresql_grant" "grant_snr_eng_db_privileges_on_courier_db" count = length(var.snr_eng_member_roles) #for_each = toset( ["table", "sequence"] ) database = "finance" role = var.snr_eng_member_roles[count.index] schema = "public" object_type = "table" objects = [] privileges = ["INSERT", "SELECT", "UPDATE", "DELETE"] depends_on = [ google_sql_user.snr_eng_member_roles, ] ## Sales Database resource "postgresql_grant" "grant_snr_eng_db_privileges_on_datasync_db" count = length(var.snr_eng_member_roles) database = "sales" role = var.snr_eng_member_roles[count.index] schema = "public" object_type = "table" objects = [] privileges = ["INSERT", "SELECT", "UPDATE", "DELETE"] depends_on = [ google_sql_user.snr_eng_member_roles, ] ## Users Database resource "postgresql_grant" "grant_snr_eng_db_privileges_on_dispatch_db" count = length(var.snr_eng_member_roles) database = "users" role = var.snr_eng_member_roles[count.index] schema = "public" object_type = "table" objects = [] privileges = ["INSERT", "SELECT", "UPDATE", "DELETE"] depends_on = [ google_sql_user.snr_eng_member_roles, ] You can easily get how it goes. To explain a bit, you see each resource points to a particular database in the CloudSQL “geekssales-db-production” instance. We have also ensured that the privileges files will “wait” for the roles to be created first before it runs. This is done via the “depends_on” line in each of the resources. You will also acknowledge that the “privileges” vary depending on your level of seniority. Step 5: Users addition to other roles We realised that PostgreSQL version 9.6 must have a user added to the group of the owner of the database for it to be able to actually receive the privileges added. Because of that, we have to add another file that adds the new CloudIAM users to the owners of the various databases. We shall create this file as follows within the same “engineering” directory. $ vim privileges-grant-role-eng.tf ###################################################### # Engineering DB Grant users to various roles ###################################################### ## Add Eng members to cloudsqlsuperuser role resource "postgresql_grant_role" "grant_eng_db_privileges_on_all" count = length(var.eng_member_roles) role = var.eng_member_roles[count.index] grant_role = "cloudsqlsuperuser" depends_on = [ google_sql_user.snr_eng_member_roles, ] ## Add eng members to geeks role resource "postgresql_grant_role" "grant_eng_db_privileges_on_all" count = length(var.eng_member_roles) role = var.eng_member_roles[count.index] grant_role = "geeks" depends_on = [ google_sql_user.eng_member_roles, ] ## Add senior eng members to geeks role resource "postgresql_grant_role" "grant_snr_eng_db_privileges_on_all" count = length(var.snr_eng_member_roles) role = var.snr_eng_member_roles[count.index] grant_role = "geeks" depends_on = [ google_sql_user.snr_eng_member_roles, ] In the end, we should have a general structure (truncated) that looks like below: $ tree └── tf_cloudsql ├── main.tf ├── modules │ ├── geekssales-db-production │ ├── main.tf │ │ └── engineering │ │ ├── privileges-grant-role-eng.tf │ │ ├── privileges-jnr-eng.tf │ │ ├── privileges-snr-eng.tf │ │ ├── privileges-eng.tf
│ │ ├── provider.tf │ │ ├── roles.tf │ │ └── vars.tf │ ├── geeksusers-db-production │ │ ├── main.tf │ │ └── engineering │ │ ├── privileges-grant-role-eng.tf │ │ ├── privileges-jnr-eng.tf │ │ ├── privileges-snr-eng.tf │ │ ├── privileges-eng.tf │ │ ├── provider.tf │ │ ├── roles.tf │ │ └── vars.tf By this time, we should be ready to create the resources we have been creating. Let us initialize terraform then execute a plan and if we will be good with the results, we can comfortably apply the changes. The commands are as follows: Initialize Terraform $ terraform init Initializing modules... Initializing the backend... Initializing provider plugins... - Reusing previous version of hashicorp/vault from the dependency lock file - Reusing previous version of cyrilgdn/postgresql from the dependency lock file - Reusing previous version of hashicorp/google from the dependency lock file - Using previously-installed hashicorp/google v4.13.0 - Using previously-installed hashicorp/vault v3.3.1 - Using previously-installed cyrilgdn/postgresql v1.15.0 Terraform has been successfully initialized! You may now begin working with Terraform. Try running "terraform plan" to see any changes that are required for your infrastructure. All Terraform commands should now work. If you ever set or change modules or backend configuration for Terraform, rerun this command to reinitialize your working directory. If you forget, other commands will detect it and remind you to do so if necessary. Terraform Plan $ terraform plan -out create_roles Then apply if you are good with the results $ terraform apply create_roles Closing Words There we have it. The entire project has its own challenges and we hope we shall continue to improve on it as time goes. In the end, the goal to be able to focus on code while Terraform or other tools do the bulk of the boring work. We hope the playbook was as informative as we intended .
0 notes
Text
Fwd: Job: Washington.MachineLearningEvolution
Begin forwarded message: > From: [email protected] > Subject: Job: Washington.MachineLearningEvolution > Date: 18 November 2021 at 05:19:18 GMT > To: [email protected] > > > Hello EvolDir Members: > > Hiring Now: NSF-funded Consultant or Postdoctoral Fellow (1 year, with > potential for extension), Washington, D.C., USA; remote work space > possible > > Project title: NSF Convergence Accelerator Track E: Innovative seafood > traceability network for sustainable use, improved market access, and > enhanced blue economy > > Project Overview: This proposal will build a cross-cutting traceability > network to accelerate the path towards accurate and inclusive monitoring > and management of marine bioresources, whose sustainability is vital to > feed the global population. Leveraging wide-ranging expertise in fisheries > science, marine biology, environmental anthropology, computer science, > trade policy, and the fisheries industry, we will develop a powerful > tool to achieve long-lasting & transferable solutions. Addressing the > global challenge of feeding the human population will require the ocean > as a solution. > > This NSF Convergence Accelerator project will: > > 1.Develop a prototype traceability tool that allows affordable > identification of species and area of capture for wild octopus fisheries > within the United States and abroad using our proposed machine learning > (ML) model “SeaTraceBlueNet” trained on legacy data of environmental > metadata, species occurrence and images; > > 2.Develop a community-based citizen-science network (fishers, researchers, > industry partners, students, etc.) to gather new data (images, metadata > and environmental DNA (eDNA)), train on and test the portable eDNA > kits and SeaTraceBlueNet dashboard prototype to build the collaborative > capacity to establish a standardized traceability system; and, > > 3.Set a system in place to connect traceability, sustainability and > legality to support the development of a blue economy around the octopus > value chain, incorporating the best practices and existing standards > from stakeholders. > > > Background and skills sought: > > Expertise (5+ years of combined work and/or academic experience) > preferably in one of the following fields: Bioinformatics, Machine > Learning, Computer Sciences, Biological Sciences, Molecular Biology Note: > PhD is not required, as long as applicant shows demonstrated abilities > in the following. > > Experience required: Software: Agile software i.e., Jira, GitHub, > Anaconda, pyCharm, Jupyter notebooks, OpenCV Must have languages: Python, > SQL, bash scripting, linux command-line Optional: C++, R, Spark, Hive > > Programming environments and infrastructure: Cloud, HPC, Linux, Windows > Familiar with Machine learning platform and libraries such as, TensorFlow, > PyTorch, Caffe, Keras, Scikit-learn, scipy, etc. Implementing computer > vision models such as ResNet, Deep learning models using Recurrent > Neural Networks (CNNs, LSTMs, DNNs), using Support Vector Machines > (SVMs) models, probabilistic and un/regression models, data processing > and handling activities including data wrangling, computer vision. > > Bonus skills: Using BERT NLP models, computer software like OpenVino, > targeting GPUs, familiarity with GCP tools and applications, > such as BigTable, cloudSQL, DataFlow, CloudML, DataProc, etc., > dashboard development and implementation, Compiling and configuring HPC > environments, developing applications using MPI, OpenMPI, pyMIC, using job > schedulers such as PBS, Scrum; Bioinformatics tools such as BLAST, Qiime2 > > Job Physical Location: Washington, D.C. USA; remote work station is > an option. > > Compensation: $65,000 (annual) with benefits, and some travel > > Although, US citizenship is not required, proper work status in the USA > is required. Unfortunately, we cannot sponsor a visa at this time. > > Expected start date: November/December 2021 (up to 12 months depending > on start date) > > Application Deadline: Applications will be reviewed and interviews > will take place on a rolling basis until the position is filled. > Application submission process: Please send the following documents via > email to Demian A. Willette, Loyola Marymount University (demian.willette > @ lmu.edu). > > 1.Curriculum Vitae: Including all relevant professional and academic > experience; contracts, collaborations, on-going projects, grants > funded, list of publications (URLs provided), presentations, workshops, > classes; Machine Learning and bioinformatics skills and languages; > Gitbub/bitbucket; any experience with processing molecular sequence data > (genomics and/or metabarcoding) > > 2.Reference contacts: Names, affiliations and contact information (email, > phone) for up to three professional references that we will contact in > the event that your application leads to an interview. > > 3.Transcripts: Transcript showing date of completion of your most relevant > degree(s) and grades. > > Please send all inquiries to: Demian A. Willette, Loyola Marymount > University (demian.willette @ lmu.edu) > > > Cheryl Ames > via IFTTT
0 notes
Text
メルカリShopsはマイクロサービスとどう向き合っているか
from https://engineering.mercari.com/blog/entry/20210806-3c12d85b97/
こんにちは。ソウゾウのSoftware Engineerの@napoliです。連載:「メルカリShops」プレオープンまでの開発の裏側の2日目を担当させていただきます。
メルカリShopsではマイクロサービスアーキテクチャによる開発を採用しています。ここではメルカリShopsではどのようにマイクロサービスと向き合っているかを紹介させていただきます。
メルカリShopsのマイクロサービス群
メルカリShopsはざっくりと、図のような形でマイクロサービス群が構成されています。
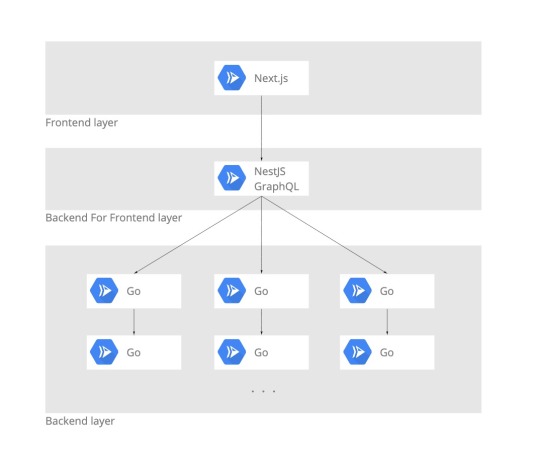
Frontendがひとつ、Backend For Frontend(BFF)がひとつ、そして(執筆時点で)約40ほどのBackendサービスが、それぞれが独立した実行環境で稼働しています。
BackendはShop(ショップ)、Product(商品)、Order(注文)、Payment(決済)といったドメインごとに独立したサービスが構築されており、ひとつのサービスはおおよそ10から20種程度のAPIを提供しています。
各サービス間の通信は基本的にgRPC / Protocol Buffersによって行われています。
マイクロサービスのメリット
システムを構築する際、マイクロサービスをアーキテクチャとして採用するメリットは何でしょうか。細かく考えると多岐にわたりますが、大きくは以下のような点が��げられると思います。
変更による影響を局所化できる
システムリソース(機能)を効率的に再利用できる
サービスごとに自由な技術選定ができる
ソウゾウは会社のValueとして「Move Fast」を掲げており、開発に置いても「スピード感」をとても重視しています。もちろんスピードだけでなく、誰もが知っているような大きなプロダクトに育てていきたいという想いもあります。
大規模なプロダクトにおいて、短期的にも長期的にも開発のスピード感を維持し続けていくという目標を掲げた時、マイクロサービスアーキテクチャの採用は必然と言えるものでした。
そしてこの「開発のスピード感を維持し続けていく」ために「変更による影響を局所化する」という点が非常に重要な要素となってきます。
日々巨大化していくシステムの中で、「いちエンジニアがシステム全体を詳細に把握してから開発をスタートさせる」ことは困難です。困難というより無理と言っても過言ではありません。「ある箇所を触ったら思いもよらぬところで不具合が出た」なんてことが日常茶飯事で起こっていたとしたら、とてもじゃないですが「スピード感のある開発」は行なえません。
かといってスピードを重視するあまり、テスト不十分のまま不具合だらけの機能を提供するわけにもいきません。開発メンバーは変更による影響を注意深く探し続け、特定できた影響範囲に対して入念なテストを実施する必要があります。テストはどのような場合でも非常に重要なステップではありますが、その量が増えれば増えるほど、機能追加や改善に掛けられる時間はどんどんと少なくなってゆきます。
また、そのような複雑なサービスはあとから見た時や新しいメンバーが開発に参加した際に全体像の把握が難しくなります。どんなに優秀なエンジニアでも人間が一度に把握できる情報量には限界があります。安心してのびのび開発するためにも、影響範囲を局所化していくことは非常に重要なのです。
「サービスごとに自由な技術選定できる」という点に関してはメリットではありますが、デメリットにもなりえます。プロダクトを構成するマイクロサービス群のなかで、各マイクロサービスごとにあまりにもバラバラな技術スタックを採用していると、いざ開発メンバーでメンテナンスを行おうとした際に、それぞれの技術による知識を習得することから入る必要があり、とても大変です。
メルカリShopsではサーバサイドはGolang、フロントエンドとBFFはTypescript、データベースはCloudSQLなどといったように、使用する言語やサービスを限���的にして開発を行っています。
マイクロサービスのデメリット
一方で、マイクロサービスアーキテクチャにはデメリットも多くあります。
設計の難易度が上がる
データの一貫性を担保するのが難しくなる
サービス間通信によるレイテンシが大きくなりやすい
一定規模以上のプロダクトでない限り、恩恵を感じることが少ない
コードや設定が冗長になりやすく、初速が出にくくなる
個人的には、「設計の難易度が上がる」ことが一番のデメリットであるかなと感じます。正直なところ、マイクロサービスアーキテクチャは「エンジニアとしてこれから頑張っていくぞ!」というフェーズの方にはおすすめできないアプローチかもしれません。ゲームで言えば初見からHARDEST Modeでプレイするようなものです。一気に設計の難易度が上がります。
幸いにしてソウゾウには歴戦のツワモノエンジニア達が揃っているので、この点についての不安はそこまではありませんでした。(ただ、それでもやっぱり開発しながら難しいな…と感じることは多々ありますが…)
しかし設計の難しさをクリアできるとしても、「コードや設定が冗長になりやすく、初速が出にくくなる」という問題は無視できるものではありませんでした。ひとつのマイクロサービスを作るためには、実行環境の設定、権限の設定、依存サービスの設定、Bootstrapコードの作成、などなど、色々と作業があります。特にインフラ系の設定周りなどは普段アプリケーションよりの開発を行っているとハマりやすく時間が吸い取られていくポイントでもあります。
サービスを起動するための初期コードの作成も地味に大変です。例えばサーバサイドであればサーバを起動するための基本となるコードです。マイクロサービスアーキテクチャではなく、既に稼働しているサーバに機能追加するのであれば基本的には必要とされない作業です。生産的な作業でない割には時間が取られますし、「似たようなコードだから」といってテストしないわけにもいきません。
Code Generation
このマイクロサービス初期構築時の負担の軽減のため、メルカリShopsでは初期コードを自動生成してくれるCode Generationの仕組みがあります。
具体的にはyamlファイルに記載された設定をもとに、goのプログラムがサーバ起動のための最低限のコードを自動生成します。
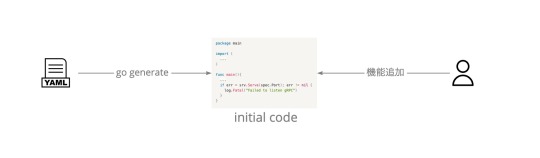
これにより開発メンバーはサーバの「機能実装」に集中することが出来るとともに、マイクロサービス構築までの時間を大きく短縮させることが出来ます。
もちろんひとつの自動生成プログラムだけで十分な自動生成ができるわけではありません。メルカリShopsでは他にもコード生成の負担を減らすためのプログラム(ツール)が用意されています。
コードが冗長になることを受け入れ、ツールによってその負担を軽減していく、というのがメルカリShops開発での基本のスタンスとなっています。
Circular dependencyの検知
マイクロサービス群が成長していくと、マイクロサービス同士がCircular Dependency(循環参照)を起こしていないかを気をつける必要があります。
Circular Dependencyが発生すると「サービスを分離しているメリットをすべて潰してしまう」、「依存されてるものからデプロイ」ができなくなる」などといった様々な問題が出てきます。
厄介なのは循環参照は短期的には問題が顕在化しないケースが多くあることです。ある問題だけを解決するには手っ取り早い解決方法に見えることがあるので、つい誘惑に負けてしまいそうになります。しかし「循環参照しているサービスはそれすなわちひとつのサービス」になってしまうので、なんのために苦労してマイクロサービスアーキテクチャを採用しているのかが分からなくなってしまいます。言い換えると循環参照が増えていくと、そのシステムはそう遠くない未来に「マイクロサービスのメリットを潰してデメリットだけ残した悪いところどりのシステム」になってしまいます。
循環参照を防ぐにはどうしたら良いでしょうか。「各サービスが循環しないように各エンジニアが気をつける」のももちろん良いのですが、サービスの数が多くなってくるとかなりきつくなります。どのサービスがどのサービスに依存しているのかすべて把握しつづけるのは難しいため、システムに慣れていてもついうっかり発生させてしまうことがあります。
メルカリShopsではCI(Continuous Integration)によるBuild時に、Circular Dependencyを検知したら自動的にFailさせるというアプローチを取っています。もちろん自動的に依存関係を解消してくれるわけではありませんが、暗黙的にマイクロサービス間のCircular Dependencyができてしまうことを防いでいます。
Service Dependencies Graph
Circular dependency検知プログラムの副産物として、Service Dependencies GraphもBuildのたびに自動生成されるようになっています。
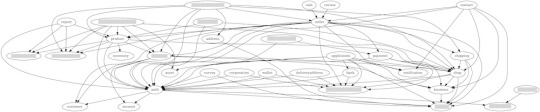
この図だと依存の線が多くなってやや分かりにくくなっていますが、ざっくりとどれが「多く依存されているサービス」や「上位のサービス」なのかが視覚的に分かりやすくなっています。
さらに図だけでは依存関係が正確に分かりづらいため、以下のような依存/非依存をを示すテーブルも自動的に生成されるようになっています。
Shop
Depends on Depends on indirectly auth business account customer
Depended from Depended from indirectly application contact product shipping order payment report sale review
mono-repository
メルカリShopsではMonorepoを採用しています。個人的にはMonorepoはマイクロサービスととても相性が良いと感じます。通常、サービスを分ける場合、Repositoryも分けることが多いですが、規模が大きくなりRepositoryがあまりに多く分かれてくるとそれぞれの連携が難しくなってきます。
一例ではありますが、例えばサービス全体をローカル環境で動かしたい場合、Repositoryが分かれているとそれぞれのRepositoryをCloneして、Buildして…といった作業が必要になってきます。数個のサービスなら手作業でもなんとかなりますが、数十個といった単位となると、動くサービスを構築するだけでも一苦労です。
(詳細な説明はここでは省きますが)メルカリShopsではMonorepoの構成にBazelを採用しているので、数多くのマイクロサービスをコマンド一発でBuildすることが可能です。
さらにdocker-composeと組み合わせることでこちらも一発でローカル開発環境を立ち上げることも出来ます。
開発環境をストレスなく継続的に構築できることは開発のスピードに大きく貢献するので、マイクロサービスを設計する際はそのあたりも意識してゆくと良いでしょう。
おわりに
いかがだったでしょうか。メルカリShopsでのマイクロサービスとどう向き合っているか、その一部を紹介させていただきました。これからマイクロサービスに挑戦していきたいエンジニアの方たちとって少しでも参考になれば幸いです。
マイクロサービスは難しさもありますが、うまく採用していくことで、拡張性、メンテナンス性をもったサステナビリティのあるシステムを構築することが可能なアーキテクチャであると思います。
本文でも言及していますが、設計の肝となるところは「いかに影響範囲、関心事を局所化していくか」であると言えるでしょう。ぜひみなさんもチャレンジしてみて頂ければと思います。
なお、メルカリShopsではメンバーを募集中です。メルカリShopsの開発に興味を持ったり、マイクロサービスによる開発にチャレンジしてみたいという方がいれば、ぜひこちらも覗いてみてください。またカジュアルに話だけ聞いてみたい、といった方も大歓迎です。こちらの申��込みフォームよりぜひご連絡ください!
https://storage.googleapis.com/prd-engineering-asset/2021/08/7fa73106-mercarishops-microservices.jpg
0 notes
Text
Database Migration Service GCP Migrates SQL Server Database
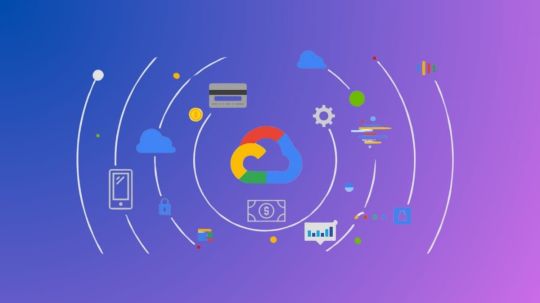
Database Migration Service GCP
Businesses are using managed cloud services to update their IT infrastructure in order to increase cost-effectiveness, security, and dependability. For these kinds of modernization initiatives, Cloud SQL for SQL Server, a fully managed database that offers both AI-powered Google Cloud innovations and native SQL Server features, is a popular option. With great excitement, Google Cloud announce today that Cloud SQL for SQL Server migrations from on-premises and other clouds are now generally accessible as a component of Database Migration Service (DMS).
Database migrations are frequently difficult and call for specialized knowledge. Customers that have used Database Migration Service GCP to move their SQL Server workloads to Cloud SQL for SQL Server have given us great feedback since it first introduced SQL Server migrations in April. Database conversion Service reduces the complexity of database migrations by utilizing continuous native backup and restoration technologies. This minimizes downtime while maintaining high fidelity, allowing your vital SQL Server databases to continue to function throughout the conversion process.
Google Cloud Database Migration Service
Your SQL Server database migrations will benefit from the following advantages with Database Migration Service‘s distinctive methodology:
Reduced system overhead and downtime: Use continuously available native backup and restoration technologies to maintain the functionality of your source databases during the migration process.
Serverless simplicity: Free up your teams to concentrate on strategic tasks by doing away with the requirement to manage infrastructure.
Prioritizing security: Encrypted SQL Server backup support guarantees strong protection during the migration.
No extra cost: obtainable at no additional expense, expanding the accessibility of cloud migration.
Plarium Global Ltd., a gaming firm, was able to transfer to Google Cloud with ease because to Database Migration Service’s SQL Server migration capabilities.
Using Database Migration Service
Here’s how to use Database Migration Service GCP to begin moving your SQL Server workloads right now:
Open the Google Cloud dashboard, navigate to Databases, Database Migration, and create a Connection Profile pointing to a Cloud Storage bucket where your SQL Server backup files will be uploaded.
In a similar manner, make another Connection Profile that points to the SQL Server instance you have selected in Cloud SQL.
To connect both connection profiles, create a migration job and choose the databases you want to move.
Verify that your migration job tested successfully by looking at the results shown below. When you’re ready, begin working on the project.
Upload a complete backup and ongoing backups of each database’s transaction logs to the Cloud Storage bucket as often as you’d like.
Following the restoration of the complete backups, the migration phase shifts from “Initial Load” to “Incremental Load.” Database Migration Service GCP regularly recovers transaction log backup files and searches for new ones.
Use the integrated metrics and logs to track the status of your migration jobs and determine when it is best to finish the migration.
To restore the databases, make them available, and finish the migration, click “Promote” for Database Migration Service when you’re ready.
And that’s it! You can now use your newly created Cloud SQL for SQL Server database.
Database Migration Service pricing
Database Migration Service is provided free of charge for homogeneous migrations, or migrations in which the source and destination databases use the same database engine. This involves moving sources like MySQL, PostgreSQL, and SQL Server to destinations like Cloud SQL and AlloyDB.
On a per-migration job basis, heterogeneous migrations between various database engines are paid in increments of one byte. Based on raw (uncompressed) data, bytes are counted.
The cost of the Database Migration Service is detailed on this page.
Get started migrating to SQL Server right now
Prepare to update your SQL Server database infrastructure and realize the full cloud potential with Database Migration Service GCP ease of use, security features, and low downtime capabilities.
Read more on govindhtech.com
#DatabaseMigrationService#GCPMigrates#SQLServer#Database#CloudSQL#DMS#CloudStorage#gcp#GoogleCloudDatabase#sql#technology#technews#news#govindhtech
1 note
·
View note
Text
Logical replication and decoding for Cloud SQL for PostgreSQL
https://cloud.google.com/blog/products/databases/you-can-now-use-cdc-from-cloudsql-for-postgresql Comments
0 notes
Text
DBeaverからcloud sqlに繋ぐ
Cloud SQL Auth Proxyを使ってDBeaverからCloudSQLにアクセスする。
前提
Cloud SQL Admin APIが有効になっている
CloudSQLのインスタンスが立っている
サービスアカウントを作成済み
CloudSQLのクライアントってroleを持っている
json keyを作成してダウンロード済み(credential.json)
Cloud SQL Auth Proxy
インストールする。
# install $ wget https://dl.google.com/cloudsql/cloud_sql_proxy.linux.amd64 -O cloud_sql_proxy # 動作可能にする $ chmod +x cloud_sql_proxy
起動する。
# credential_fileを別指定して起動 $ ./cloud_sql_proxy -instances=INSTANCE_CONNECTION_NAME=tcp:13306 -credential_file=credential.json
ここでいう、INSTANCE_CONNECTION_NAMEは、GCP_PROJECT:REGION:DB_INSTANCE_NAMEって形式の接続名です。 プロキシはlocalhost:13306に起動します。
DBeaver
接続を追加する。ドライバはMySQLなりPostgreSQLなり、よしなに。
接続設定にて以下を設定
Server Host: localhost
Port: 13306
Database: あれば任意で設定
User Name: root | 他にあれば
Password: UserNameに対応したパスワード
これで接続テストしたら繋がる。
ref
Cloud SQL Auth Proxy を使用して接続する
Cloud SQLをローカルのDBeaver(クライアントツール)にプロキシを使用して接続する方法
0 notes
Text
Retention settings for Cloud SQL
#CloudSQL now allows configuring backup retention settings to protect against data loss. You can retain between 1 and 365 days’ worth of automated backups and between 1 and 7 days’ worth of transaction logs for point-in-time recovery.

0 notes
Text
Google Cloud Platform Console Walkthrough
GCP Console provides a web-based, graphical user interface that one can use to manage their Google Cloud projects and resources.
The GCP Console can be gotten to through the GCP Console landing page. On the off chance that you are new to Google Cloud, first pursue a Google Cloud free preliminary record.
1.) Home
Google Cloud Platform Console Homepage
The HOME tab has data about dynamic assets and charging data.
There are 3 different tabs accessible; viz Dashboard, Activity, and Recommendations.
2.) Dashboard
Dashboard
After the main use, the Dashboard is set as the default presentation page when you open the GCP Console. The dashboard shows the data about the at present dynamic undertaking that the client is working on, and furthermore refreshes on different boundaries like APIs, Resources, Instances, Billing, and so forth
3.) Activity and Recommendations
The Activity tab shows a background marked by all activities performed by the client on the GCP support. This incorporates things like making or erasing an occasion, making another task, sending/altering/erasing another bunch, or setting up another Virtual Machine (VM).
The Recommendations tab houses ideas from Google’s side, to additionally improve the client experience, in light of their work design on the Google Cloud Platform.
Toolbar
The Google Cloud Platform includes a toolbar at the top, which has simple access catches to get to warnings, settings, and other such utilities.
1.) Search
To get going, there is a flat hunt bar in the toolbar to help search for any assistance in the GCP Console.
Search
2.) Cloud Shell
The Activate Cloud Shell button opens up a little window at the lower part of the screen, from where the client can enter orders to interface with the GCP climate by means of CLI
Cloud Shell
3.) Help
The Help button appears the different accessible assistance choices, similar to the Documentation joins, console alternate ways, opening the Support place, and so forth
GCP Help Button
4.) Notifications
The notices for all assignments performed on GCP Console can be found here. These incorporate the creation or cancellation of a VM, occasion, group, or some other substance.
GCP Notifications Button
5.) Settings
This catch permits the client to set their inclinations, and alter project settings
GCP Settings ButtonNavigation Menu
The Navigation Menu has fast connects to all significant administrations offered by the Google Cloud Platform. Aside from the HOME area talked about above, there are different alternate routes like MarketPlace, Billing, Support, Security, and so on There is an element called “Beginning”, which contains all capacities that another client may require. It very well may be gotten to as follows
Beginning
This will lead you to the Getting Started page, which has the accompanying choices prepared as brisk access.
One can likewise stick certain administrations for simpler access, by tapping on the pin button close to their name.
Pin
Peruse more about Google Cloud Services and Tools in our blog.
1.) Compute Products
The Google Compute Engine is an adjustable and secure figure administration that allows one to make, run and oversee virtual machines on the Google Cloud. One can make formats for simple access for the future, and save their inclinations there.
Aside from the Compute Engine, the Compute Products tab likewise has other process alternatives like Kubernetes Engine, Google App Engine, Cloud Run, and Google Cloud Functions.
Figure Engine
2.) Storage and Database Products
Google Cloud Storage is perhaps the most well known stockpiling administrations available at this point. The Cloud Storage offered under GCP is an immediate likeness Amazon’s S3 administration. Among the best 3 cloud specialist co-ops, Google Cloud stockpiling is additionally the most savvy choice ($/GB).
Notwithstanding capacity administrations, GCP likewise has a few DataBase administrations. These incorporate CloudSQL, Cloud Firestore, and Cloud Spanner — each altered by the necessities of the client concerning size.
Find out about them in our blog Google Cloud stockpiling And Database Services.
Distributed storage
3.) Other Services
Moreover, GCP has administrations spreading over a great deal of different regions too. These can be recorded as follows.
APIs and ML: APIs are Application Programming Interfaces that permit correspondence with Google Services and their combination with different administrations. The Machine Learning API is one of the more famous APIs accessible. The administrations offered under this are:
Guides Platform
Cloud Translation
Cloud Speech to Text
Systems administration: This has choices that permit the client to alter and redo the different parts of the systems administration — like IP Address determination, directing, firewalls, VPC organizations, and so on The accompanying administrations fall under this class:
Google Cloud Load Balancing
Cloud DNS
Cloud VPN
Enormous Data: The administrations accessible under this title take into consideration simple administration of information that is too huge to be dealt with physically. Google offers an administration framework, alongside ongoing examination and information bits of knowledge to help the client or association.
0 notes
Text
In case you intend to use CloudSQL to host your application’s databases in GCE, then there will be a need for you to have Google Cloud Compute Engine SQL Proxy installed and working. This will allow you to connect to your Databases securely and beautifully. The Cloud SQL Auth proxy provides secure access to your instances without a need for Authorized networks or for configuring SSL. It works by having a local client running in the local environment. Your application communicates with the Cloud SQL Auth proxy running locally with the standard database protocol used by your database. Benefits of Cloud SQL Auth proxy Secure connections: The proxy automatically encrypts traffic to and from the database using TLS with a 128-bit AES cipher. IAM database authentication Easier connection authorization Prerequisites For this to work till the end, we need to have the following: Google Cloud authentication credentials. You will have to create a service account credential file (JSON) specifically for the Cloud SQL Auth proxy. It will be explicitly and permanently linked to the Cloud SQL Auth proxy as long as it is running. A valid database user account and password for your instance. Step 1: Install Cloud SQL Auth proxy To begin the installation process, we will first download the Cloud SQL Auth proxy: cd ~ wget https://dl.google.com/cloudsql/cloud_sql_proxy.linux.amd64 -O cloud_sql_proxy Step 2: Make it executable After you have fetched the file of interest, we will have to make the Cloud SQL Auth proxy executable as follows. chmod +x cloud_sql_proxy Step 3: Create SystemD service At this point, we can use the application by executing it as follows ./cloud_sql_proxy -version Even though you can use the Cloud Compute Engine SQL Proxy as it is now, we can do better. We can create a systemd service so that we can have it easy to start, stop , enable or disable the service. It will afford us a lot os convenience and we can manage it just like we are used to managing other daemons and applications within your servers. We can do this, so let us get to it right away. First, add cloud_sql_proxy, the executable, to PATH as follows sudo cp ~/cloud_sql_proxy /usr/local/bin Then let us create a systemd service file thus. You can call the service a name that works for you: $ sudo vim /lib/systemd/system/proxy.service [Install] WantedBy=multi-user.target [Unit] Description=Google Cloud Compute Engine SQL Proxy Requires=networking.service After=networking.service [Service] Type=simple WorkingDirectory=/usr/local/bin ExecStart=/usr/local/bin/cloud_sql_proxy -instances=your_gcp_project:region_of_instace:cloudsql_instance_name=tcp:3307 -credential_file=/var/credential.json Restart=always StandardOutput=journal User=root The “credential.json” file is the service account we need having the requisite permissions to access CloudSQL. Replace the following with your details: your_gcp_project region_of_instace e.g us-central1 cloudsql_instance_name port e.g 3307 or something else not used in the server. Do a daemon reload so that the new file can be read and loaded sudo systemctl daemon-reload Then start and enable the proxy sudo systemctl start proxy sudo systemctl enable proxy Check its status to confirm that everything is okay $ systemctl status proxy ● proxy.service - Google Cloud Compute Engine SQL Proxy Loaded: loaded (/lib/systemd/system/proxy.service; enabled; vendor preset: enabled) Active: active (running) since Wed 2022-02-02 13:52:01 UTC; 21h ago Main PID: 9411 (cloud_sql_proxy) Tasks: 10 (limit: 4915) Memory: 10.3M CGroup: /system.slice/proxy.service └─9411 /usr/local/bin/cloud_sql_proxy -instances This is the part that you smile! Step 4: Connecting to your instance via the Auth Proxy When you connect to your instance using the Cloud SQL Auth proxy, you provide a user account that is used to log in to the instance. You can use any database user account for this purpose.
An example of connecting to a MySQL instance is as follows via the CLI. mysql -u your_user —-host=127.0.0.1 —-port=3307 -p your_database In this example, we are connecting to port 3307 as we configured our “proxy.service” to listen from. It is also connecting to localhost. So the Google Cloud Auth SQL Proxy will receive the command and route it all the way to your GCP CloudSQL instance. However, because the Cloud SQL Auth proxy always connects from a hostname that cannot be accessed except by the Cloud SQL Auth proxy, you can create a user account that can be used only by the Cloud SQL Auth proxy. The advantage of doing this is that you can specify this account without a password without compromising the security of your instance or your data. To create a user account for Cloud SQL Auth proxy connections, specify the hostname as ‘cloudsqlproxy~[IP_ADDRESS]‘. You can also use the IP address wildcard, which would result in ‘cloudsqlproxy~%‘. You can do it as follows using “gcloud” command: gcloud sql users create user \ --host=cloudsqlproxy~24.123.4.142 \ --instance=cloudsql-instance \ --password=password Or gcloud sql users create user \ --host=cloudsqlproxy~% \ --instance=cloudsql_instance_name \ --password=your_password After that, you can now connect to the Auth proxy from your application and it will authenticate against the new user without any qualms. And that is how we will end our guide today. References Google Cloud Docs Concluding Remarks We hope that the information provided will be helpful and any improvements we can make are always welcome. Thank you for reading through and we continue to appreciate your enormous support that we continue to receive.
0 notes